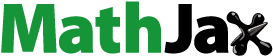
ABSTRACT
Recent development of monoclonal antibodies as mainstream anticancer agents demands further optimization of their safety for use in humans. Potent targeting and/or effector activities on normal tissues is an obvious toxicity concern. Optimization of specific tumor targeting could be achieved by taking advantage of the extracellular acidity of solid tumors relative to normal tissues. Here, we applied a structure-based computational approach to engineer anti-human epidermal growth factor receptor 2 (Her2) antibodies with selective binding in the acidic tumor microenvironment. We used an affinity maturation platform in which dual-pH histidine-scanning mutagenesis was implemented for pH selectivity optimization. Testing of a small set of designs for binding to the recombinant Her2 ectodomain led to the identification of antigen-binding fragment (Fab) variants with the desired pH-dependent binding behavior. Binding selectivity toward acidic pH was improved by as much as 25-fold relative to the parental bH1-Fab. In vitro experiments on cells expressing intact Her2 confirmed that designed variants formatted as IgG1/k full-size antibodies have high affinity and inhibit the growth of tumor spheroids at a level comparable to that of the benchmark anti-Her2 antibody trastuzumab (Herceptin®) at acidic pH, whereas these effects were significantly reduced at physiological pH. In contrast, both Herceptin and the parental bH1 antibody exhibited strong cell binding and growth inhibition irrespective of pH. This work demonstrates the feasibility of computational optimization of antibodies for selective targeting of the acidic environment such as that found in many solid tumors.
Introduction
Antibody-based anti-cancer therapeutics are intended to target antigens present on tumor cells. Specific tumor-targeting can be accomplished via antigens exclusively found on cancer cells and not present at all on normal cells, such as epidermal growth factor receptor (EGFR)vIII resulting from a deletion in the EGFR gene specific to glioma cells. In most cases, however, the target antigen overexpressed by cancer cells is also present at lower concentrations in normal tissues. In order to reduce antibody toxicity in these cases, one strategy is to take advantage of the higher antigen density on tumor cells relative to normal cells.Citation1–Citation4 This approach requires modulation of antibody-antigen affinity, e.g., by mutagenesis of the complementarity-determining region (CDR), to an optimal range where binding to the low-density antigen on normal cells is reduced while a reasonable level of binding to the high-density antigen present on tumor cells is retained. This results from the avidity of bridged binding that can be achieved by typical bivalent antibodies and related constructs. The optimal range of monovalent-binding selectivity is found empirically and is system dependent; too little or too much affinity weakening can lead to maintained binding of antigens at low density, or loss of binding at high density, respectively. The avidity-based approach can only be applied when there is a significant antigen overexpression on tumor cells and their surrounding stroma.
Here, we propose a completely different optimization strategy for specific tumor targeting, which exploits the slightly higher acidity of the tumor relative to normal tissues pH.Citation5,Citation6 Due to factors such as poor vascular perfusion, regional hypoxia, and fermentative glycolysis,Citation7 the extracellular pH in most solid tumors is in the 6.0–6.8 range.Citation8–Citation16 Recently, marking of acidic regions with pH below 6.5 overlapped with highly proliferative, invasive regions at the tumor-stroma interface.Citation17 However, non-cancerous cells maintain the extracellular pH at physiological levels (7.3–7.4). In order to take advantage of this differential pH to reduce antibody toxicity on normal cells, CDR mutagenesis can be used to introduce a certain level of pH dependence into the antibody binding affinity to the antigen, such that binding is significantly weakened at physiological pH relative to the acidic pH. Since the pKa (negative logarithm of acid ionization constant, Ka) of histidine residues present on protein surface is ~6.4,Citation18 histidine-scanning mutagenesis is as a logical approach for accomplishing this type of design.
De novo engineering of pH-dependent antibody binding has overwhelmingly focused on weakening binding at acidic pH relative to the physiological pH. When antibody CDRs were mutated in order to generate so-called recycling or sweeping antibodies, the motivation was mainly to direct overexpressed antigens to lysosomal degradation following dissociation in the acidic endosomes from their antibody complexes.Citation19–Citation27 A similar approach for engineering pH-dependent dissociation was also applied to antibody regions outside of the CDR or to non-antibody protein-protein complexes.Citation28–Citation30 From a completely different perspective, protein domains were engineered against non-CDR antibody surfaces as binding reagents at neutral pH from which antibodies can be eluted at acidic pH.Citation31–Citation34 Engineered selectivity toward the acidic pH was rarely reported, aimed at extending half-lives in blood. Examples include de novo engineering of an affibody protein for binding to the recycling neonatal Fc receptor (FcRn) at the acidic pH of early endosomes,Citation35 and modulating the already present pH-dependent binding of Fc to FcRn to further improve binding selectivity toward acidic pH.Citation36
Expectedly, histidine mutagenesis has been the workhorse for most of these pH-dependent binding engineering efforts, either by screening of recombinant variants or selection from combinatorial display libraries. While computational design has been successfully applied to antibody-antigen affinity maturation,Citation37,Citation38 successfully predicting pH-dependent antigen-binding CDRs of antibodies has been limited thus far. To our knowledge, only two previous computational structure-based design studies reported successful prospective engineering of pH-dependent binding proteins, both aimed at weakening binding at acidic pH.Citation28,Citation33 A computational framework for structure-based design of pH-dependent binding was also proposed and used to retrospectively recapitulate previous Fc-FcRn pH-dependent binding data.Citation39
In this study, bH1, a Fab targeting human epidermal growth factor receptor 2 (Her2), was selected as the starting point for structure-based de novo engineering of pH-dependent antigen binding. In addition to its available crystal structure in complex with the antigen, bH1 binds Her2 with reduced affinity relative to the related antibody trastuzumab (Herceptin®).Citation40 As mentioned earlier, this is a desired characteristic that can be used to reduce toxicity to normal cells via avidity. Here, we first implemented dual-pH histidine-scanning mutagenesis into the Assisted Design of Antibody and Protein Therapeutics (ADAPT) platform previously used for antibody-antigen affinity maturation at physiological pH.Citation38,Citation41 The extended computational platform was then applied to the structure of the bH1-Her2 complex aiming at improved binding selectivity toward acidic pH versus normal pH. Rational designs were first tested as Fabs at two pHs, for in vitro binding to the soluble recombinant Her2 ectodomain and then for binding to intact Her2 expressed at cell surface. Full-size antibody (FSA) versions of successfully designed mutants were then tested for binding to Her2 expressing cells by scanning the pH within the 5.2–7.3 range. Finally, pH selectivity and functional efficacy were tested using an in vitro tumor spheroid growth inhibition assay. Rationally designed FSA variants displayed marked selectivity toward the extracellular pH of solid tumors versus that of normal tissues.
Results
Computational design of pH dependence
The concept of free energy optimization of a parent antibody-antigen system via mutagenesis for improved binding at acidic pH (tumor microenvironment) relative to physiological pH (normal cells) is presented schematically in . The main objective is to widen the binding free energy gap, between the mutant and parent variants in the acidic relative to physiological environments. This binding free energy gap must be as negative as possible in order to enhance the selectivity for binding at acidic versus physiological pH. At the same time, the mutant must maintain a reasonable level of binding in the acidic environment relative to the parent molecule. For example, a mutant that binds 1000-fold better at acidic versus physiological pH, but has a 100 μM affinity under acidic conditions, has high pH selectivity but is of limited practical use. Hence, a filter was applied to step (3) in to ensure that
is not too overly positive (e.g., not more than 2.7 kcal/mol, or 100-fold increase in KD). Moreover, since the designed mutants must be stable in the intended acidic environment, another filter was applied to the folding free energy associated with step (3). This ensures that the stability of the mutant is comparable with that of the parent, and hence the predicted
should typically be less than 2.7 kcal/mol.Citation38 For manufacturing and in vivo delivery reasons, stability at physiological pH should also be maintained, and so ∆∆Gfolding at that pH associated with step (4) in should not be overly positive either.
Figure 1. Definition of relative binding free energy function for optimization of pH dependence. The main property to be optimized is ∆∆∆Gbinding, the binding free energy gap between the Acidic and Physiological pH environments of a Mutant relative to the Parent, which has to be as negative as possible. This is shown in the upper diagram as the difference given by (1) – (2). Computationally, we simulate (3) – (4) instead, which from the thermodynamic cycle yields the same quantity. The bottom diagram provides an illustrative example for a possible distribution of free energies for the four states shown in the thermodynamic cycle, and how ∆∆∆Gbinding can be calculated based on their free energies
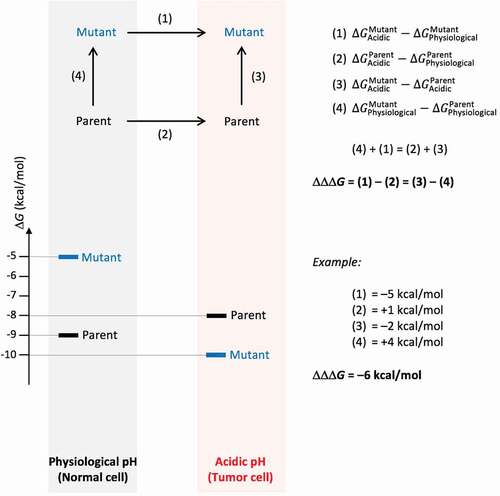
We have implemented a version of ADAPTCitation38,Citation41 capable of handling dual-pH His-scanning mutagenesis, and applied it to the bH1 Fab in complex with Her2 ectodomain.Citation40 A total of 68 positions (non-His, non-Pro, non-Cys) forming the CDR loops of bH1 (Figure S1) were screened for single mutations to His with three protocols for mutant generation and relative binding affinity scoring, and with one protocol for relative stability scoring (). We excluded 21 mutants based on folding stability criteria. From the remaining 47 mutations, the top 10 in average ranks in terms of ∆∆∆Gbinding binding affinity gap were retained. Ranks 1 (L-R30a), 2 (H-R58), 6 (H-N28), 8 (H-Y100a) and 10 (H-Y56) were pursued further. The other five mutations were excluded after visual examination of the modeled 3D structures, namely rank 3 (H-G97) due to steric clashes, rank 4 (L-Y92) due to packing of protonated His in a hydrophobic environment, and ranks 5 (H-N54), 7 (L-S30d) and 9 (H-K64) for which the introduced His residues made no direct contacts with the antigen. Rank 26 (L-S30b) was included based on its second-best stability in acidic conditions. Rank 30 (H-Y33) was also included as another example of a non-highly-ranked mutant. These two mutants from the bottom half of the ranked list passed visual inspection in terms of good steric and electrostatic interactions in the complex. Lastly, H-R50 was included as another control, having the best average rank for binding affinity change if the folding stability filter at acidic conditions was not considered.
Table 1. Computational predictions of relative binding affinities and stabilities under slightly acidic and physiological pH conditions
These selected residues for single-point mutations to histidine are distributed over four of the six CDR loops (Figure S1). Having widely separated mutations at the antibody–antigen interface () can be beneficial for combining them into higher-order mutants using the ADAPT protocol, based on reasonable additivity of contributions from spatially separated mutations to binding affinity.Citation38,Citation41
Figure 2. Structural location of selected histidine mutations. Shown is the crystal structure of the parental bH1-Her2 complex (PDB code 3BE1)Citation40 as prepared for molecular simulations. Only the antigen-binding Fv domains of the antibody are shown, and colored cyan and magenta for the heavy and light chains, respectively. Selected positions for mutation to histidine are shown as Cα-sphere models and are labeled. Domain IV (residues C489-N607) of the Her2 antigen including the epitope is rendered as a black ribbon inside a translucent gray molecular surface
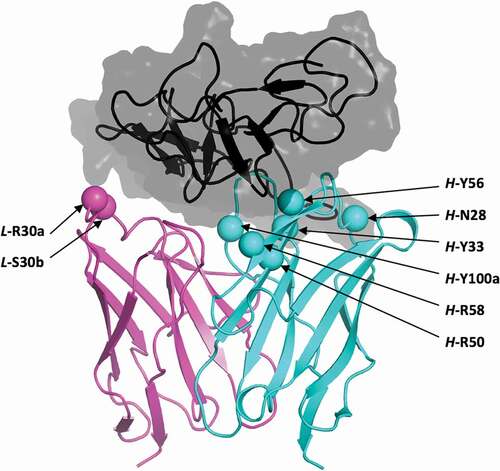
pH-dependent binding to recombinant Her2 ectodomain
The parental and selected bH1-Fab variants were first tested for binding to recombinant Her2 ectodomain by surface plasmon resonance (SPR) at the physiological pH of 7.4 as well as at pH 5.0, whereby the Fab samples were flowed over the antigen ectodomain immobilized at a sensor-chip surface. Interestingly, parental bH1-Fab bound to the Her2 ectodomain about 4 times weaker at acidic pH (KD = 13 ± 4 nM) versus physiological pH (KD = 3 ± 1 nM) due to a combination of a slower on-rate and a faster off-rate (). In contrast, our rank-2 computational structure-based designed variant bH1-P5 having the H-R58 amino-acid residue mutated to histidine showed preferential antigen binding at acidic pH (KD = 98 ± 30 nM) versus physiological pH (KD = 310 ± 8 nM). In free energy terms, reversal by the new mutant of the undesired binding phenotype of the parental Fab to the desired pH dependence resulted from a negative value for ∆∆∆Gbinding of −1.54 kcal/mol. This indicates a successful design in the desired direction for pH dependence, and is clearly apparent in the iso-affinity plot in . Here, we see the opposite directions of binding free energy change by shifting the pH from physiological to acidic, and comparing the parental bH1-Fab to the bH1-P5 and other variants.
Table 2. SPR data for Fab binding to recombinant human Her2 ectodomain
Figure 3. Iso-affinity plots from SPR data. Acidic pH data are plotted with red symbols, physiological pH data with black symbols. Arrows indicate moving the data point on the iso-affinity plot from physiological to acidic environments for various variants (labeled, mean data from )
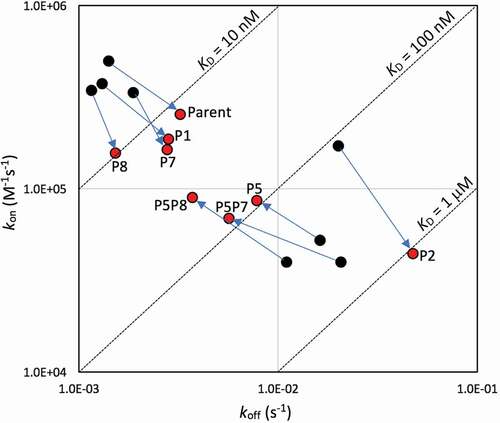
By the ∆∆∆Gbinding objective metric, other successful designs were bH1-P7 (L-R30aH) and bH1-P8 (L-S30bH), although in these cases the undesired binding phenotype of the parent was not reversed but merely weakened. These are also apparent in the iso-affinity plot (). Variant bH1-P1 (H-N28H), ranked 6 computationally, was similar to the parent, whereas bH1-P6 (H-Y100aH, ranked 8) had complex binding and poor fit at acidic pH. For variant bH1-P2 (H-Y33H, ranked 30) the parental phenotype was actually accentuated, and hence this variant had a positive value for ∆∆∆Gbinding (). Finally, binding was so weak that it could not be detected at one or both pHs for variants bH1-P4 (H-Y56H, ranked 10) and bH1-P3 (H-R50H, control for testing a case with high destabilization predicted at acidic pH). Because this SPR binding analysis was carried out at 25°C, we repeated the experiments at 37°C and obtained similar trends (Table S1), with almost identical equilibrium constants and slightly higher rate constants as expected from the Arrhenius equation.
Based on the SPR data for single mutants, three designed bH1 variants, bH1-P5, -P7 and -P8, were selected for generation of double mutants bH1-P5P7 (H-R58H, L-R30aH) and bH1-P5P8 (H-R58H, L-S30bH). These double mutants combine well-spaced mutations present on distinct chains, aiming at introducing additivity of mutation effects. The SPR-based ∆∆∆Gbinding values listed in confirm that additivity was achieved. Both double mutants had ~sixfold stronger antigen binding at acidic pH than at physiological pH. This behavior was significantly driven by faster dissociations (larger koff) at physiological pH than at acidic pH, in sharp contrast to the parent, as is also apparent in the iso-affinity plot (). By comparing the variant bH1-P5P8 with the parental bH1-Fab, we see that antigen binding in the acidic environment has only marginally weakened (KD of 50 ± 20 nM versus 13 ± 4 nM, respectively) whereas binding affinity in the physiological environment has been weakened by 2 orders of magnitude (KD of 290 ± 50 nM versus 3 ± 1 nM, respectively). This is also readily seen by visual inspection of the corresponding SPR sensorgrams shown in .
Figure 4. Representative SPR sensorgrams for select Fab variants. Interaction of the parent bH1-Fab, the lead single mutant bH1-P5 (H-R58H) and the double mutant bH1-P5P8 (H-R58H,L-S30bH) with immobilized Her2 ectodomain. The black lines represent raw data and the red lines are global fits to a 1:1 bimolecular interaction model. See the Materials and Methods section for experimental details
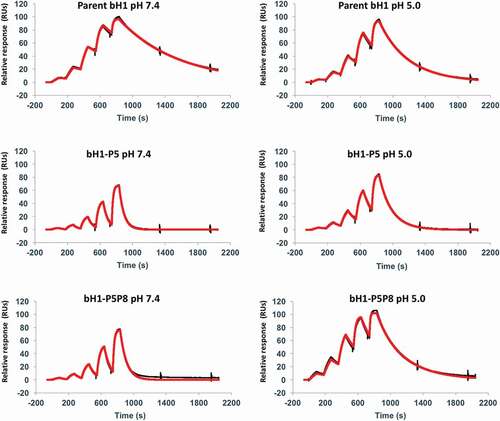
pH-dependent binding to cells expressing intact Her2
We next sought to examine the most promising designed bH1-Fab variants P5, P5P7 and P5P8 for pH dependence of binding to cells expressing intact Her2. At the high Her2 cell-surface density of the SKOV3 cells, the parent bH1-Fab binding was ~twofold weaker at acidic pH (KD of ~41 nM) than at physiological pH (KD of 21 nM) (). In contrast, while weak binding of the designed Fab mutants to SKOV3 cells could be detected at pH 5.2 (KD range ~100–200 nM), their binding, if any, was weaker than the sensitivity of our detection method at pH 7.3 (). The double mutant bH1-P5P8 Fab was found to have ~twofold stronger affinity than bH1-P5 and bH1-P5P7 at acidic pH. Similar results were obtained on the JIMT-1 cell line expressing Her2 at lower density than SKOV3 cells (data not shown). The viability of various cell lines under acidic (pH 5.2) and physiological (pH 7.3) conditions was tested and shown to be unaffected by the conditions used in the binding assay (Figure S2).
Table 3. Apparent binding affinities to SKOV3 cells for bH1 variants formatted as Fabs
Figure 5. pH dependence of Fab variants binding to Her2-expressing SKOV3 cells. Selected anti-Her2 Fab variants were analyzed for cell-based binding by flow cytometry under acidic and physiological pH conditions (using Method A, Materials and Methods section). Error bars represent standard deviations between technical replicates
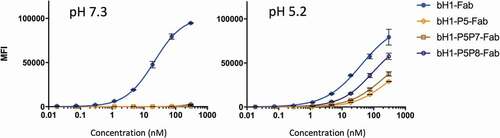
The designed variants were then reformatted into human IgG1/k FSAs for re-testing on the high-density Her2 cells. We first verified that the designed His mutations do not introduce protein folding instability or aggregation relative to the parental bH1 antibody. Differential scanning calorimetry (DSC) and sedimentation velocity (SV) analytical ultracentrifugation analyses showed similar biophysical properties for all bH1-FSA variants at both pH 5.1 and pH 7.2 (Figures S3 and S4, and Table S2). We then performed a pH scan of SKOV3 cell binding within the 5.2–7.3 range for designed FSA variants and control antibodies (). The parental bH1-FSA displayed low-nM apparent affinity similar to the related antibody Herceptin at physiological pH (apparent KD of 4 nM and 3 nM, respectively, ), suggesting that the weaker cell-based binding previously seen with the bH1-Fab (KD ~20 nM, , ) could be improved by avidity binding. Both parental bH1-FSA and Herceptin displayed practically no pH-dependent cell binding, with apparent KD values relatively stable within the 2–4 nM range between pH 5.2 and pH 7.3. The designed FSA mutants also maintained significant cell-surface Her2 binding in the acidic range of pHs, from pH 5.2 up to pH 6.0 (KD range 8–12 nM), with some weakening of affinity in the pH 6.4–6.8 range to 13–30 nM. Importantly, further increasing the pH to 7.3 led to a marked drop of binding capacity of the designed variants well above an apparent KD of 100 nM (), which is in sharp contrast to the pH-independent binding observed with the parental bH1-FSA and Herceptin. Hence, we were successful in designing Her2 directed antibodies that showed more than 10-fold pH-selectivity for SKOV3 cell binding at pH 6.8 over pH 7.3. The observed cell binding dependence on pH for the designed mutants () is consistent with the histidine pKa value of ~6.4.Citation18
Table 4. Apparent binding affinities to SKOV3 cells for bH1 variants formatted as full-size antibodies (FSAs)
Figure 6. pH dependence of FSA variants binding to cells expressing Her2. (A) High-density Her2 cells (SKOV3) were tested in environments with varying pH between 5.2 and 7.3 and cell binding was analyzed by flow cytometry (using Method A, Materials and Methods section). Error bars represent standard deviations between technical replicates. (B) Apparent dissociation constants (KD) of tested FSA variants from binding experiments to high-density Her2 cells (SKOV3) at various pHs
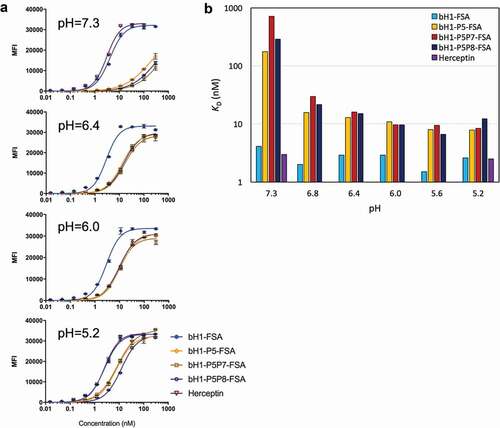
Our multi-well plate binding assay (see Method A, Materials and Methods section) requires an antibody dilution series in small and equal volumes (0.1 mL) to be performed. This volume constraint resulted in molar amounts of antibodies in the lower dose range (0.02 to 1.2 nM) that were less than the calculated total number of surface Her2 receptors on the SKOV3 cells (which express ~6 × 10Citation5 Her2 receptors per cellCitation42) (Table S3, Method A). This may have caused stoichiometric limitations and affected KD measurements. To assess this possibility, we tested binding of the parental bH1-FSA antibody and a representative mutant, bH1-P5P8-FSA, to SKOV3 cells at pH 6.0 and pH 7.3, comparing Method A with a manual dilution regimen performed in tubes. In the latter case, sample volumes were varied such that antibody was always in excess relative to Her2 for every dose (Table S3, Method B). Our analysis showed that with both methods, bH1-FSA had similar binding to cells at pH 7.3 and pH 6.0 (KD ~1–4 nM), whereas bH1-P5P8-FSA showed significantly improved binding at pH 6.0 compared to pH 7.3 (KD ~10 nM versus ˃ 130 nM, respectively) (Figure S5, Table S4). We note the caveat that, although Method A was informative for screening and ranking our mutant antibodies, low-nanomolar binding affinities measured by this assay are somewhat reduced, likely due to antibody depletion at the lower concentration range (e.g., at pH 6.0, bH1-FSA KDs are 2.9 nM versus 0.7 nM using Method A versus Method B, respectively).
To further determine the tumor selectivity of these antibodies, we evaluated their binding on Her2-expressing JIMT-1 cells (i.e., ~10Citation4 Her2 receptors per cell, representing a lower Her2 density similar to Her2-expressing cardiac cellsCitation43) at physiological pH. As it can be seen in the left panel of , Herceptin and bH1-FSA bind very well to the surface of these cells at pH 7.3. In sharp contrast, the designed antibodies, and especially the double-point His mutants, completely lost their binding in these normal cell conditions even at the highest tested concentration of 300 nM (~45 μg/mL). Here, the pH-dependent binding mechanism works in concert with weakened avidity effects to completely eliminate off-tumor targeting and increase the selectivity for tumor versus normal tissue. pH-dependent binding of the His-mutant bH1-FSAs was also present on the low-Her2 JIMT-1 cells, with estimated KDs greater than 50 nM at pH 6.0 (, right panel), albeit it more closely mirrored the pH-dependent binding of Fabs on high-Her2 cells due to lack of avidity effects. The data also suggest reduced off-tumor targeting of normal tissues with acidic pH (e.g., Her2 expressed on normal gastric epitheliaCitation44) relative to Herceptin and parental bH1-FSA.
Figure 7. pH dependence of FSA variants binding to cells expressing Her2 at low-density. Low-density Her2 expressing JIMT-1 cells were tested under acidic and physiological pH conditions and cell binding was analyzed by flow cytometry (using Method A, Materials and Methods section). The left panel representing binding to the normal cell model (low-density Her2 and physiological pH) is to be compared with binding of the same variants to the tumor cell model consisting of high-density Her2 (SKOV3 cells) within a pH range of 6.0–6.8 (). Error bars represent standard deviations between technical replicates
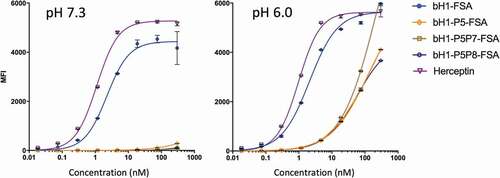
pH-dependent inhibition of tumor spheroid growth
We tested the pH-dependent function of the designed variant bH1-P5P8-FSA by evaluating its effect on the growth of the BT474 spheroids in vitro. Human breast invasive ductal primary carcinoma BT474 cells expressing high levels of Her2 were selected since they readily responded to the benchmark Herceptin antibody in spheroid assays.Citation45,Citation46 We asked if the spheroids grown under normal or low-pH conditions respond differently to antibody treatment. We first established that the growth of the spheroids without antibody treatment in response to low pH conditions was not affected (Figure S6). To assess the pH selectivity of the designed antibody relative to Herceptin, spheroids were treated with different concentrations of antibodies either in physiological pH or a lower pH of 6.4 typical for solid tumor microenvironment (). As expected, Herceptin as well as the parental bH1 antibody performed similarly in both pHs. The antibody mutant with engineered pH-sensitive Her2 binding only inhibited the growth when spheroids were treated in low-pH conditions (). This suggests that the engineered pH selectivity for Her2 binding is also manifested functionally. The tumor growth inhibition efficacy of the engineered bH1-P5P8-FSA antibody was statistically indistinguishable from those of the benchmark Herceptin and parental bH1 antibodies when the extracellular pH was acidified artificially ().
Figure 8. Tumor spheroid growth inhibition. Human breast primary carcinoma BT474 spheroids were cultured in physiological pH and in acidic pH of 6.4 in the presence of antibodies Herceptin, bH1-FSA, bH1-P5P8-FSA or Ctrl-hIgG (human IgG1 isotype antibody, negative control), and spheroid size monitored over time (see Materials and Methods section). (A) Dose-response effect of antibody variants in different pH conditions at the 192 h time point. Spheroid size is normalized to time zero and Ctrl-hIgG. (B) Spheroid growth over time for different antibody variants in different pH conditions. Spheroid size is normalized to time zero and Ctrl-hIgG. The p-values for each treatment relative to the control were calculated using Student’s t-test (*, p ≤ 0.05; **, p ≤ 0.01). (C) Representative images of the spheroids at 100 μg/mL antibody concentration in different pH conditions at the end of experiment. Error bars in (A) and (B) represent standard deviations between biological replicates
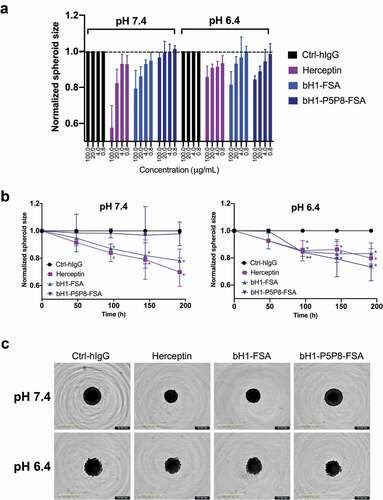
Discussion
Previous histidine mutagenesis studies directed to the CDRs of antibodies have generally focused on maintaining or enhancing antigen binding at physiological pH while reducing binding at acidic pH.Citation20–Citation26 In most cases, the motivation for that type of pH-dependent binding is lysosomal degradation of targeted receptors and soluble ligands via recycling or sweeping antibodies.Citation19 Here, a novel dual-pH computational His-scanning mutagenesis based on the previous ADAPT platformCitation38,Citation41 was applied to undertake the opposite, perhaps more challenging, task of improving binding at acidic relative to physiological pH. The practical relevance of this pH dependency is reduction of antibody toxicity via selective targeting of the acidic tumor microenvironment.Citation5–Citation7 In this case, a positively charged and highly solvated His residue is introduced at the interface with the antigen, which is a desolvated environment. While this is challenging from a molecular design perspective, naturally evolved systems exist that rely on histidine switches in order to achieve this type of pH-dependent binding selectivity. A classic example is antibody recycling, where several His residues from the antibody Fc are used for pH-dependent binding to FcRn at the acidic pH of early endosomes and dissociation from it at extracellular physiological pH.Citation47,Citation48
For designing the type of pH-dependent binding pursued in this study, the ideal scenario is to weaken binding in the physiological environment (negative design) and strengthen binding in the acidic environment (positive design) relative to the parent, as exemplified in . Scenarios based only on positive designs or only on negative designs in both environments are also viable. It is likely that available optimization routes will be system dependent. In the bH1-Her2 system investigated here, the pH selectivity of the P5 mutant H-R58H arises from negative designs at both pHs, with the impact being marginal at acidic pH and large at physiological pH (see , –). Molecular modeling suggests that replacing arginine for histidine at position H-58 may incur some modest cost in nonpolar packing in both environments due to a relatively crowded location at the antibody–antigen interface. From an electrostatic viewpoint, this mutation may have minimal impact in the acidic environment where the positive charge is maintained and favorable interaction with the negatively charged E558 could be established, but it may incur a larger cost in the physiological environment that eliminates the positive charge (, left panel). A similar mechanism underlies the P7 mutant L-R30aH, but the negative design is attenuated relative to P5 due to its more exposed location. Hence, for P7, the small effect (see ) of negative design at physiological pH is predicted to arise from removal of positive charge and loss of electrostatic interactions with the negatively charged E598 (, middle panel). The case of P8 mutation L-S30bH seems to be different, as the SPR and cell-binding data for the P8 mutant versus parent, as well as between the P5P8 and P5 mutants (see and ), appear to signal a small degree of positive design under acidic conditions. A charged His residue at this position can interact more favorably with E598 than the parental neutral residue Ser (, right panel).
Figure 9. Structural details of pH-sensitive histidine mutations. Antibody chains of the parental bH1 antibody are colored as in , and the antigen is rendered as gray tube. At each mutated position, the parental side chain and its histidine side chain substitutions in the acidic conditions (dark-green C atoms) and physiological conditions (bright-green C atoms) are overlaid and rendered as sticks model. The main interacting side chains of the antigen are shown in sticks models and labeled. Rosetta models for histidine mutants shown
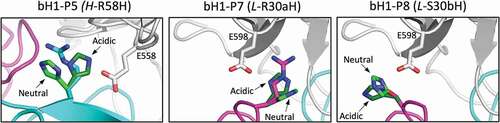
The ability of Herceptin and the related bH1-FSA to equally engage Her2-expressing cells at the slightly acidic pH typical of solid tumors as well as at physiological pH typical of normal tissues () may result in unwanted systemic toxicity to the host. Histidine mutants derived from the bH1-FSA designed in this study have more than 10-fold weaker binding at pH 7.3 than at pH 6.0 while still possessing fairly strong binding within the pH 6.0–6.8 range (KD below 30 nM) to high Her2-density tumor cells. At physiological pH, these variants lose their binding, even at the highest tested concentration of 300 nM (~45 μg/mL), to cells expressing Her2 at a level typical to normal cells (). Therefore, it is anticipated that these pH-sensitive variants will serve as safer treatment modalities. We tested the effect of the most promising designed pH-sensitive variant bH1-P5P8-FSA in a spheroid growth inhibition assay adapted to mimic tumor acidic conditions, and found that it inhibited spheroid growth with similar efficacy relative to the benchmark Herceptin and parental bH1-FSA antibodies (). In contrast to Herceptin and the bH1-FSA, which exerted their effects on spheroid growth independently of the extracellular pH, we observed a highly pH-selective effect for bH1-P5P8-FSA. This further supports the success of our design strategy to generate a pH-selective antibody that can efficiently bind and function under tumor acidic conditions. It remains to be established whether the observed in vitro functional efficacy on spheroid growth inhibition will be maintained on tumors in vivo. An important aspect in vivo is the pH heterogeneity of solid tumors. Our designed pH-sensitive variants seem to maintain cell-binding capacity within a relatively broad range of pH relevant to the tumor microenvironment, i.e., from 6.0 to 6.8 (, ), while their efficacies will likely be lower in tumor pockets of physiological pH. Minimal binding of pH-sensitive antibodies to cells under normal conditions (low-Her2 density at physiological pH, ) suggests that the effect of tumor heterogeneity on efficacy could be overcome by adjusting the dosing regimen. Overall, we anticipate that pH-sensitive antibodies will be better at mitigating the balance between efficacy on tumor cells versus safety on normal cells, relative to non-pH-sensitive antibodies.
A structure-based approach such as that adopted here adds to the design toolbox for pH-dependent binding, which includes screening and display-based directed evolution approaches. A requirement of the rational approach is the availability of a crystal structure for the antibody-antigen complex. Provided that the computational ADAPT algorithm can rapidly suggest a short list of histidine mutants with the desired phenotype. Hence, it appears as less laborious than screening or selection methods, with the added benefit of molecular structure-based rationalization of the observed pH dependency. Currently, the ADAPT protocol is limited to histidine mutagenesis, whereas other methods such as directed evolution may access a wider sequence space that sometimes include non-histidine mutations, possibly via conformational effects on pH dependence.Citation30
Anti-Her2 antibodies with pH-dependent binding selectivity toward slightly acidic pH such as those disclosed here can be adapted to other formats suitable for various therapeutic modalities. In particular, antibody-drug conjugate versions are needed because they carry toxic payloads with potential for widespread cytotoxicity.Citation49,Citation50 Another format that would benefit from pH-selective antibodies is that used in the radioimmunotherapy (RIT) of solid tumors, especially when a compartmental route of administration is not feasible and systemic application leads to radiation exposure to non-target organs.Citation51 The fact that Fabs of the designed variants possess monovalent binding at acidic pH with no binding at physiological pH () suggests that they may be used as targeting moieties in bispecific antibodies. Furthermore, a heterodimeric IgG framework can be augmented by multivalent presentation of the pH-sensitive Fab arm for increased potency through avidity (e.g., 1Fab-IgG format).Citation1,Citation52 Another exciting application would be their reformatting as single-chain variable domains for generation of chimeric antigen receptors (CARs).Citation53 The 2D-tethering of CARs on the T-cell membrane may also complement their pH-dependent binding with avidity-driven selectivity toward tumor cells. The promising results obtained in this study not only warrant further development of these improved variants toward the clinic, but they also open an exciting perspective that computational structure-based engineering of pH-selectivity can be successfully applied to other high-profile cancer targets with the aim of delivering safer immunotherapies in oncology.
Materials and methods
Computational design
The Her2-bound crystal structure of bH1-Fab was retrieved from the Protein Data Bank (entry 3BE1),Citation40 and structurally prepared for molecular design as described previously at physiological pH.Citation38 At slightly acidic pH of 5–6, visual examination was used in order to decide whether a His side chain can be protonated based on its structural environment protonation. This led to protonation of three His residues in the antigen epitope: H497, H537 and H567, whereas the other two His residues in the antigen epitope (H490 and H542), as well as the two His residues of the Fv fragment (L-H95 and H-H35), were treated in the neutral state in the slightly acidic conditions.
Computational His scanning was carried out with the ADAPT protocolCitation38 to generate and score single-point mutations to histidine of non-proline, non-cysteine and non-histidine residues in the CDR region covering three loops in the light chain: CDR-L1 (R24-A34), CDR-L2 (W50-S56), CDR-L3 (Q89-T97), and three loops in the heavy chain: CDR-H1 (G26-H35), CDR-H2 (R50-G65) and CDR-H3 (R94-Y104) of bH1-Fv (Figure S1). Histidine mutations were built and evaluated energetically at these positions using the three programs, SCWRL,Citation54–Citation56 FoldX,Citation57,Citation58 and Rosetta,Citation59,Citation60 currently implemented in ADAPT.Citation38 From an operational perspective, since scoring functions for computational mutagenesis provide meaningful results when taken as scores relative to the parent system for which the crystal structure has been determined, the implementation of these methods in ADAPT adopts a conservative approach that limits conformational flexibility of the mutants as much as possible, and especially at the level of the protein backbone atoms.Citation59,Citation61
In this work, histidine mutations were generated at physiological pH and also in slightly acidic conditions (e.g., pH 6). For mutations at physiological pH, all programs were forced to mutate to a neutral histidine. For mutations at slightly acidic pH, each program had a different implementation of a protonated histidine. Rosetta had the simplest method in that mutations to histidine were forced to use the protonated form. In the case of FoldX, protonated histidine takes two different forms, protonated delta (called “o”) and protonated epsilon (called “e”). Therefore, the mutation with the lowest FoldX stability score was retained and used for ranking. Lastly, for SCWRL, the option to enable the protonated form to compete with neutral histidine tautomers was chosen. While this does not force mutation to the protonated form, if the resulting mutation is neutral it is assumed that the protonated form would be destabilizing, and vice-versa. Binding scores of the constructed His mutants relative to the parent were then calculated at each of the two pHs, and
, with the three scoring functions in ADAPT: SIE,Citation55,Citation56 FoldX-FOLDEF,Citation57 and Rosetta-Interface.Citation59 The double-referenced binding free energy scores, ∆∆∆Gbinding (), were then derived as the difference in the scores obtained in acidic conditions relative to physiological conditions. A consensus ranking was finally derived from these individual ∆∆∆Gbinding scores calculated with each scoring functions. The FoldX-FOLDEF energy functionCitation57 was also used to estimate the effect of His substitutions on the internal stability of the Fv structure at each of the two pHs. Additional technical and implementation details of ADAPT and its component methods can be found in earlier reports.Citation38,Citation41,Citation61
Protein production and purification
cDNA for the heavy and light chains of Fab and full-size human IgG1/k antibody variants were ordered from commercial vendors (ThermoFisher Scientific/Life Technologies Inc., Burlington, ON; GENEART, Regensburg, Germany). These contained signal peptide sequences, but no His-tags. Productions were carried out by co-transfection of CHO-3E7 cells as described previously,Citation38 at 200-mL scale. Transfections were performed at a cell density around 2.0 × 10Citation6 cells/mL with viability greater than 98%. Cells were distributed in 1.0 L shaker flasks and transfected with 1 μg of total DNA per 1 mL of production (50–80% of the total DNA contained heavy chain and light chain constructs) using PEI MAXTM (Polysciences, Inc., Warrington, PA). Cell cultures were incubated for 24 h on an orbital shaking platform at an agitation rate of 110 rpm at 37°C in a humidified 5% CO2 atmosphere. Twenty-four hours later, the cultures were fed with Tryptone N1 at 1% w/v, transferred to 32°C and harvested 7–10 days post-transfection. Cell density and cell viability were determined by direct counting of cell samples with a Cedex automated cell counting system (Roche Innovatis, Bielefeld, Germany) using the trypan blue dye exclusion method.
Purifications from cell-culture supernatants were performed by protein-A affinity chromatography for Fabs and the IgG1/k full-size antibodies (FSAs). Purifications of cell-culture supernatants were performed by loading the Fabs onto MabSelect resin column (GE Healthcare Life Sciences, Mississauga, ON) and FSAs onto HiTrap MabSelect column (GE Healthcare), equilibrated in HyCloneTM Dulbecco’s phosphate-buffered saline (DPBS; GE Healthcare Life Sciences). Columns were washed with PBS, and Fabs or FSAs were eluted with 100 mM citrate buffer pH 3.6 and pH 3.0, respectively. Fractions containing Fabs or FSAs were pooled and the citrate buffer was exchanged against DPBS on CentriPure P100 columns (EMP Biotech, Howell, NJ) or ZebaSpin TK MWCO columns (ThermoFisher Scientific, Waltham, MA). Purified Fabs and FSAs were sterilized by filtration through 0.2 μm filters. Ultra-high performance liquid chromatography-size exclusion chromatography (UPLC-SEC) was used to assess the purity of all eluates. Variants with less than 95% purity (Fabs bH1-P4, bH1-P6, bH1-P5P7, and bH1-P5P8) were further purified by preparative SEC on Superdex-200 increase columns (GE Healthcare Life Sciences). Selected peak fractions were concentrated by ultrafiltration using Vivaspin® Turbo 4 or 15 (depending on the volume to concentrate) centrifugal concentrator with a membrane molecular weight cut off of 10 kDa (GE Healthcare Life Sciences) at room temperature following the manufacturer’s instructions. During the process, the protein concentration was monitored on a NanoDrop™ 2000 spectrophotometer (ThermoFisher Scientific) using absorbance at 280 nm and the calculated specific extinction coefficient of each variant. UPLC-SEC chromatograms of purified Fabs are shown in Figure S7.
Differential scanning calorimetry analysis
DSC was used to determine the thermal transition midpoints (Tm) of bH1-FSA variants using a VP-Capillary DSC system (Malvern Instruments Ltd, Malvern, UK). Samples in DPBS buffer were diluted in the DPBS buffer to a final concentration of 0.4 mg/mL. Aliquots of each variant were buffer exchanged to 20 mM sodium acetate, 150 mM NaCl, pH 5.1 using 0.5 mL, 7 kDa MWCO ZebaSpin columns (ThermoFisher Scientific) according to the manufacturer’s instructions. Samples in acetate buffer were diluted in the acetate buffer to concentrations of 0.15–0.25 mg/mL to accommodate sample availability. Thermal denaturation was carried out by increasing the temperature from 20°C to 100°C at a rate of 60°C/h, with feedback mode/gain set at “low”, filtering period of 8 s, pre-scan time of 3 min, and under 70 psi of nitrogen pressure. All data were analyzed with Origin 7.0 software (OriginLab Corporation, Northampton, MA). Thermograms were corrected by subtraction of corresponding buffer blank scans and normalized to the protein molar concentration. The Tm were determined using a manual fit to three transitions.
Sedimentation velocity analysis
SV analytical ultracentrifugation experiments were performed on a Beckman XL-I analytical ultracentrifuge monitoring absorbance at 280 nm. Full-size antibodies, with the exception of bH1-FSA, were diluted to an A280 of 0.5 with a pathlength of 0.3 cm. Material availability required that bH1-FSA be diluted to an A280 of 0.3. Two sector charcoal-filled epon centerpieces were used with the appropriate buffer loaded into the reference sector. Samples were sedimented at 40,000 rpm using an eight-hole rotor at 20°C with absorbance scans collected every 4 min. The c(s) distributions were obtained from scans 1–63 using SEDFITCitation62 and were integrated using GUSSI.Citation63
Surface plasmon resonance binding method
Fab-Her2 interactions were analyzed using a Biacore T200 (GE Healthcare, Mississauga, ON) SPR instrument. Samples were assayed at 25°C or 37°C using PBS containing 0.05% Tween 20 (Teknova, Hollister, CA) with added 3.4 mM ethylenediaminetetraacetic acid (EDTA) as running buffer or 150 mM citrate-phosphate buffer pH 5 with added 3.4 mM EDTA, 135 mM NaCl and 0.05% Tween 20. Recombinant human Her2 extracellular domain (ThermoFisher Scientific, Burlington, ON) was immobilized onto a CM-5 sensorchip along with a mock-activated blank control surface for referencing. Her2 was diluted to 10 μg/mL in 10 mM NaOAc immobilization buffer pH 4.5 (Biacore) and immobilized to ~200 RUs using the Biacore control software Immobilization Wizard with standard NHS/EDC amine coupling. The Her2 interaction was determined using single-cycle kinetics analysis for each variant with five concentrations using threefold dilutions from the top concentration between 100 and 900 nM depending on the affinity of the variant. Fab samples were injected at 100 μL/min with a contact time of 90 s and a 1200-s dissociation using either pH 5.0 or 7.4 running buffer. Sensorgrams were double referenced to the mock-activated blank sensor surface and analyzed for kinetic determination using a 1:1 binding model in BiaEvaluation software v3.1 (GE Healthcare).
Flow cytometry binding methods
SKOV3 and JIMT-1 cells (ATCC) were cultured in McCoy’s 5A and DMEM media, respectively, supplemented with 10% fetal bovine serum (FBS).
Method A. Cells in T-75 flasks were washed twice with DPBS and then dissociated using Cell Dissociation buffer (Sigma, C5914) at 37ºC. The cells were centrifuged and resuspended in the appropriate pH binding buffer; RPMI-1640 media, 2% FBS, 50 mM BES (Sigma), at the indicated pH ranging from pH 5.2 to 7.3, and then dispensed at 1 × 10Citation5 cells/well in a 96-well polypropylene (PP) v-bottom plate (Costar) at 4ºC. Pre-diluted Fab or full-size antibody samples were then added to cells to give concentrations ranging from 0.02 to 300 nM (eight-point dilution series) in a final volume of 100 μL/well, followed by incubation at 4°C, 2 h. The cells were then washed twice by centrifugation at 233 × g, removal of supernatant by aspiration and resuspending the cells in 200 μL binding buffer at 4°C. Detection reagent, either anti-human Fab or Fc AlexaFluor488-(Fab’)Citation2 (Jackson Immunochemicals, West Groove, PA; Cat# 109-546-097 and 709-546-098, respectively), was then added at a final concentration of 10 μg/mL and samples were incubated at 4°C, 1 h. The cells were washed twice in 200 μL binding buffer, followed by addition of 120 μL 1.0% propidium iodide and samples were then transferred to Multiscreen 96-well plates (60 μm Nylon Mesh, Millipore, Etobicoke, ON) and filtered by centrifugation. The filtrate samples were collected from the Multiscreen receiver plate and transferred to a new V-bottom polypropylene plate at 4°C. Flow cytometric analysis was performed on a BD LSR-Fortessa instrument (BD Biosciences, San Jose, CA). The AlexaFluor488 fluorescence was measured using a 488 nm laser as excitation source and a 530/30 nm bandpass filter. Median fluorescence intensity (MFI) was reported by analyzing 3000 alive cells per sample with the gating strategy: all cells/singlets/alive cells (PI negative) using BD FACSDiva software (BD Biosciences).
Method B. To rule out stoichiometric limitations, binding experiments were also performed where antibody was kept in excess over the total number of Her2 receptors on the cells. In this case, after dissociation from the flasks, SKOV3 cells were resuspended and aliquoted into separate polypropylene tubes at different cell numbers (2 × 10Citation4 or 4 × 10Citation4 cells) and volumes of pH binding buffer (0.1, 6.7 or 10.0 mL), and then incubated with various doses of antibody at 4°C for 2 h, as shown in Table S3. The cells were then washed, centrifuged, resuspended and treated with anti-human Fc AlexaFluor488-(Fab’)Citation2, as described above in Method A.
Spheroid cultures
BT474 cells (ATCC) were grown in McCoy’s 5A medium supplemented with 10% FBS. Cells were seeded at 1000 cells per well in 96-well PrimeSurface 3D Culture Spheroid plates (S-BIO, Hudson, NH) 72 h prior to the addition of antibodies. Spheroids were then supplemented with adjusted concentrations of antibodies diluted in the culture media at pH 7.4 or pH 6.4. The pH of the medium was altered by adjusting the sodium bicarbonate concentration in the base medium to achieve the desired pH. Culture media were equilibrated at 37°C and 5% CO2 for at least 12 h prior to use. Spheroid growth was monitored over 8 days and images were captured every 6 h using IncuCyte S3 (Essen BioScience, Ann Arbor, MI). Spheroid segmentation and size measurements were conducted using the IncuCyte software, following instrument guidelines. Spheroid sizes were then normalized to time zero and to the human IgG1 isotype control antibody (BioXCell, West Lebanon, NH, Cat# BE0297) treated spheroids.
Abbreviations
ADAPT | = | assisted design of antibody and protein therapeutics |
CAR | = | chimeric antigen receptor |
CDR | = | complementarity-determining region |
Fab | = | fragment antigen-binding |
FSA | = | full-size antibody |
MFI | = | median fluorescence intensity |
SPR | = | surface plasmon resonance |
Author Contributions
TS, JCZ, MLJ, JB, CRC, and EOP contributed to the conception and the study design. CD performed software implementation. TS and CRC carried out computer simulations and analyzed the data. JB performed SPR binding studies and analyzed the data. NR carried out spheroid growth inhibition studies and analyzed the data. YCD and AR performed flow cytometry binding studies, and together with JCZ and MLJ analyzed the data. MP supervised protein purification. MD carried out protein production. JDS performed biophysical characterization of full-size antibodies. TS wrote the manuscript text. All authors contributed to editing.
Disclosure of potential conflicts of interest
The authors declare that they have no conflicts of interest with the contents of this article.
Supplemental Material
Download Zip (6.7 MB)Acknowledgments
We thank Nathalie Bousquet-Gagnon, Pierre Plante, Hongtao Qi, Marc Sasseville, and Louise Thauvette for help with protein purification.
Supplementary material
Supplemental data for this article can be accessed on the publisher’s website.
References
- Slaga D, Ellerman D, Lombana TN, Vij R, Li J, Hristopoulos M, Clark R, Johnston J, Shelton A, Mai E, et al. Avidity-based binding to Her2 results in selective killing of HER2-overexpressing cells by anti-HER2/CD3. Sci Transl Med. 2018;10:eaat5775. doi:https://doi.org/10.1126/scitranslmed.aat5775.
- Rudnick SI, Lou J, Shaller CC, Tang Y, Klein-Szanto AJ, Weiner LM, Marks JD, Adams GP. Influence of affinity and antigen internalization on the uptake and penetration of anti-HER2 antibodies in solid tumors. Cancer Res. 2011;71:2250–15. doi:https://doi.org/10.1158/0008-5472.CAN-10-2277.
- Harms BD, Kearns JD, Su SV, Kohli N, Nielsen UB, Schoeberl B. Optimizing properties of antireceptor antibodies using kinetic computational models and experiments. Methods Enzymol. 2012;502:67–87.
- Zhou Y, Goenaga AL, Harms BD, Zou H, Lou J, Conrad F, Adams GP, Schoeberl B, Nielsen UB, Marks JD. Impact of intrinsic affinity on functional binding and biological activity of EGFR antibodies. Mol Cancer Ther. 2012;11:1467–76. doi:https://doi.org/10.1158/1535-7163.MCT-11-1038.
- Tannock IF, Rotin D. Acid pH in tumors and its potential for therapeutic exploitation. Cancer Res. 1989;49:4373–84.
- Stubbs M, McSheehy PM, Griffiths JR, Bashford CL. Causes and consequences of tumour acidity and implications for treatment. Mol Med Today. 2000;6:15–19. doi:https://doi.org/10.1016/S1357-4310(99)01615-9.
- Kato Y, Ozawa S, Miyamoto C, Maehata Y, Suzuki A, Maeda T, Baba Y. Acidic extracellular microenvironment and cancer. Cancer Cell Int. 2013;13:89. doi:https://doi.org/10.1186/1475-2867-13-89.
- Zhang X, Lin Y, Gillies RJ. Tumor pH and its measurement. J Nucl Med. 2010;51:1167–70. doi:https://doi.org/10.2967/jnumed.109.068981.
- Damaghi M, Wojtkowiak JW, Gillies RJ. pH sensing and regulation in cancer. Front Physiol. 2013;4:370. doi:https://doi.org/10.3389/fphys.2013.00370.
- Hashim AI, Zhang X, Wojtkowiak JW, Martinez GV, Gillies RJ. Imaging pH and metastasis. NMR Biomed. 2011;24:582–91. doi:https://doi.org/10.1002/nbm.1644.
- Gillies RJ, Raghunand N, Karczmar GS, Bhujwalla ZM. MRI of the tumor microenvironment. J Magn Reson Imaging. 2002;16:430–50. doi:https://doi.org/10.1002/(ISSN)1522-2586.
- Estrella V, Chen T, Lloyd M, Wojtkowiak J, Cornnell HH, Ibrahim-Hashim A, Bailey K, Balagurunathan Y, Rothberg JM, Sloane BF, et al. Acidity generated by the tumor microenvironment drives local invasion. Cancer Res. 2013;73:1524–35. doi:https://doi.org/10.1158/0008-5472.CAN-12-2796.
- Gerweck LE, Seetharaman K. Cellular pH gradient in tumor versus normal tissue: potential exploitation for the treatment of cancer. Cancer Res. 1996;56:1194–98.
- Corbet C, Feron O. Tumour acidosis: from the passenger to the driver’s seat. Nat Rev Cancer. 2017;17:577–93. doi:https://doi.org/10.1038/nrc.2017.77.
- Harguindey S, Reshkin SJ. The new pH-centric anticancer paradigm in oncology and medicine. Semin Cancer Biol. 2017;43:1–4. doi:https://doi.org/10.1016/j.semcancer.2017.02.008.
- Damaghi M, Tafreshi NK, Lloyd MC, Sprung R, Estrella V, Wojtkowiak JW, Morse DL, Koomen JM, Bui MM, Gatenby RA, et al. Chronic acidosis in the tumour microenvironment selects for overexpression of LAMP2 in the plasma membrane. Nat Commun. 2015;6:8752. doi:https://doi.org/10.1038/ncomms9752.
- Rohani N, Hao L, Alexis MS, Joughin BA, Krismer K, Moufarrej MN, Soltis AR, Lauffenburger DA, Yaffe MB, Burge CB, et al. Acidification of tumor at stromal boundaries drives transcriptome alterations associated with aggressive phenotypes. Cancer Res. 2019;79:1952–66. doi:https://doi.org/10.1158/0008-5472.CAN-18-1604.
- Tanokura M. 1H-NMR study on the tautomerism of the imidazole ring of histidine residues. I. Microscopic pK values and molar ratios of tautomers in histidine-containing peptides. Biochim Biophys Acta. 1983;742:576–85. doi:https://doi.org/10.1016/0167-4838(83)90276-5.
- Igawa T, Mimoto F, Hattori K. pH-dependent antigen-binding antibodies as a novel therapeutic modality. Biochim Biophys Acta. 2014;1844:1943–50. doi:https://doi.org/10.1016/j.bbapap.2014.08.003.
- Schroter C, Gunther R, Rhiel L, Becker S, Toleikis L, Doerner A, Becker J, Schonemann A, Nasu D, Neuteboom B, et al. A generic approach to engineer antibody pH-switches using combinatorial histidine scanning libraries and yeast display. MAbs. 2015;7:138–51. doi:https://doi.org/10.4161/19420862.2014.985993.
- Konning D, Zielonka S, Sellmann C, Schroter C, Grzeschik J, Becker S, Kolmar H. Isolation of a pH-sensitive IgNAR variable domain from a yeast-displayed, histidine-doped master library. Mar Biotechnol (NY). 2016;18:161–67. doi:https://doi.org/10.1007/s10126-016-9690-z.
- Tillotson BJ, Goulatis LI, Parenti I, Duxbury E, Shusta EV. Engineering an anti-transferrin receptor scFv for pH-sensitive binding leads to increased intracellular accumulation. PLoS One. 2015;10:e0145820. doi:https://doi.org/10.1371/journal.pone.0145820.
- Igawa T, Ishii S, Tachibana T, Maeda A, Higuchi Y, Shimaoka S, Moriyama C, Watanabe T, Takubo R, Doi Y, et al. Antibody recycling by engineered pH-dependent antigen binding improves the duration of antigen neutralization. Nat Biotechnol. 2010;28:1203–07. doi:https://doi.org/10.1038/nbt.1691.
- Murtaugh ML, Fanning SW, Sharma TM, Terry AM, Horn JR. A combinatorial histidine scanning library approach to engineer highly pH-dependent protein switches. Protein Sci. 2011;20:1619–31. doi:https://doi.org/10.1002/pro.696.
- Chaparro-Riggers J, Liang H, DeVay RM, Bai L, Sutton JE, Chen W, Geng T, Lindquist K, Casas MG, Boustany LM, et al. Increasing serum half-life and extending cholesterol lowering in vivo by engineering antibody with pH-sensitive binding to PCSK9. J Biol Chem. 2012;287:11090–97. doi:https://doi.org/10.1074/jbc.M111.319764.
- Devanaboyina SC, Lynch SM, Ober RJ, Ram S, Kim D, Puig-Canto A, Breen S, Kasturirangan S, Fowler S, Peng L, et al. The effect of pH dependence of antibody-antigen interactions on subcellular trafficking dynamics. MAbs. 2013;5:851–59. doi:https://doi.org/10.4161/mabs.26389.
- Bonvin P, Venet S, Fontaine G, Ravn U, Gueneau F, Kosco-Vilbois M, Proudfoot AE, Fischer N. De novo isolation of antibodies with pH-dependent binding properties. MAbs. 2015;7:294–302. doi:https://doi.org/10.1080/19420862.2015.1006993.
- Sarkar CA, Lowenhaupt K, Horan T, Boone TC, Tidor B, Lauffenburger DA. Rational cytokine design for increased lifetime and enhanced potency using pH-activated “histidine switching”. Nat Biotechnol. 2002;20:908–13. doi:https://doi.org/10.1038/nbt725.
- Heinzelman P, Krais J, Ruben E, Pantazes R. Engineering pH responsive fibronectin domains for biomedical applications. J Biol Eng. 2015;9:6. doi:https://doi.org/10.1186/s13036-015-0004-1.
- Traxlmayr MW, Lobner E, Hasenhindl C, Stadlmayr G, Oostenbrink C, Ruker F, Obinger C. Construction of pH-sensitive Her2-binding IgG1-Fc by directed evolution. Biotechnol J. 2014;9:1013–22. doi:https://doi.org/10.1002/biot.201300483.
- Bailey LJ, Sheehy KM, Hoey RJ, Schaefer ZP, Ura M, Kossiakoff AA. Applications for an engineered protein-G variant with a pH controllable affinity to antibody fragments. J Immunol Methods. 2014;415:24–30. doi:https://doi.org/10.1016/j.jim.2014.10.003.
- Gera N, Hill AB, White DP, Carbonell RG, Rao BM. Design of pH sensitive binding proteins from the hyperthermophilic Sso7d scaffold. PLoS One. 2012;7:e48928. doi:https://doi.org/10.1371/journal.pone.0048928.
- Strauch EM, Fleishman SJ, Baker D. Computational design of a pH-sensitive IgG binding protein. Proc Natl Acad Sci U S A. 2014;111:675–80. doi:https://doi.org/10.1073/pnas.1313605111.
- Tsukamoto M, Watanabe H, Ooishi A, Honda S. Engineered protein A ligands, derived from a histidine-scanning library, facilitate the affinity purification of IgG under mild acidic conditions. J Biol Eng. 2014;8:15. doi:https://doi.org/10.1186/1754-1611-8-15.
- Seijsing J, Lindborg M, Hoiden-Guthenberg I, Bonisch H, Guneriusson E, Frejd FY, Abrahmsen L, Ekblad C, Lofblom J, Uhlen M, et al. An engineered affibody molecule with pH-dependent binding to FcRn mediates extended circulatory half-life of a fusion protein. Proc Natl Acad Sci U S A. 2014;111:17110–15. doi:https://doi.org/10.1073/pnas.1417717111.
- Ghetie V, Popov S, Borvak J, Radu C, Matesoi D, Medesan C, Ober RJ, Ward ES. Increasing the serum persistence of an IgG fragment by random mutagenesis. Nat Biotechnol. 1997;15:637–40. doi:https://doi.org/10.1038/nbt0797-637.
- Lippow SM, Wittrup KD, Tidor B. Computational design of antibody-affinity improvement beyond in vivo maturation. Nat Biotechnol. 2007;25:1171–76. doi:https://doi.org/10.1038/nbt1336.
- Vivcharuk V, Baardsnes J, Deprez C, Sulea T, Jaramillo M, Corbeil CR, Mullick A, Magoon J, Marcil A, Durocher Y, et al. Assisted design of antibody and protein therapeutics (ADAPT). PLoS One. 2017;12:e0181490. doi:https://doi.org/10.1371/journal.pone.0181490.
- Spassov VZ, Yan L. pH-selective mutagenesis of protein-protein interfaces: in silico design of therapeutic antibodies with prolonged half-life. Proteins. 2013;81:704–14. doi:https://doi.org/10.1002/prot.v81.4.
- Bostrom J, Yu SF, Kan D, Appleton BA, Lee CV, Billeci K, Man W, Peale F, Ross S, Wiesmann C, et al. Variants of the antibody herceptin that interact with HER2 and VEGF at the antigen binding site. Science. 2009;323:1610–14. doi:https://doi.org/10.1126/science.1165480.
- Sulea T, Hussack G, Ryan S, Tanha J, Purisima EO. Application of assisted design of antibody and protein therapeutics (ADAPT) improves efficacy of a Clostridium difficile toxin A single-domain antibody. Sci Rep. 2018;8:2260. doi:https://doi.org/10.1038/s41598-018-20599-4.
- DeFazio-Eli L, Strommen K, Dao-Pick T, Parry G, Goodman L, Winslow J. Quantitative assays for the measurement of HER1-HER2 heterodimerization and phosphorylation in cell lines and breast tumors: applications for diagnostics and targeted drug mechanism of action. Breast Cancer Res. 2011;13:R44. doi:https://doi.org/10.1186/bcr2866.
- Onsum MD, Geretti E, Paragas V, Kudla AJ, Moulis SP, Luus L, Wickham TJ, McDonagh CF, MacBeath G, Hendriks BS. Single-cell quantitative HER2 measurement identifies heterogeneity and distinct subgroups within traditionally defined HER2-positive patients. Am J Pathol. 2013;183:1446–60. doi:https://doi.org/10.1016/j.ajpath.2013.07.015.
- Press MF, Cordon-Cardo C, Slamon DJ. Expression of the HER-2/neu proto-oncogene in normal human adult and fetal tissues. Oncogene. 1990;5:953–62.
- Rodriguez CE, Reidel SI, de Kier Joffe EDB, Jasnis MA, Fiszman GL. Autophagy protects from trastuzumab-induced cytotoxicity in HER2 overexpressing breast tumor spheroids. PLoS One. 2015;10:e0137920. doi:https://doi.org/10.1371/journal.pone.0137920.
- Gangadhara S, Smith C, Barrett-Lee P, Hiscox S. 3D culture of Her2+ breast cancer cells promotes AKT to MAPK switching and a loss of therapeutic response. BMC Cancer. 2016;16:345. doi:https://doi.org/10.1186/s12885-016-2377-z.
- Roopenian DC, Akilesh S. FcRn: the neonatal Fc receptor comes of age. Nat Rev Immunol. 2007;7:715–25. doi:https://doi.org/10.1038/nri2155.
- Burmeister WP, Huber AH, Bjorkman PJ. Crystal structure of the complex of rat neonatal Fc receptor with Fc. Nature. 1994;372:379–83. doi:https://doi.org/10.1038/372379a0.
- Beck A, Goetsch L, Dumontet C, Corvaia N. Strategies and challenges for the next generation of antibody-drug conjugates. Nat Rev Drug Discov. 2017;16:315–37. doi:https://doi.org/10.1038/nrd.2016.268.
- Masters JC, Nickens DJ, Xuan D, Shazer RL, Amantea M. Clinical toxicity of antibody drug conjugates: A meta-analysis of payloads. Invest New Drugs. 2018;36:121–35. doi:https://doi.org/10.1007/s10637-017-0520-6.
- Bartholoma MD. Radioimmunotherapy of solid tumors: approaches on the verge of clinical application. J Labelled Comp Radiopharm. 2018;61:715–26. doi:https://doi.org/10.1002/jlcr.3619.
- Brinkmann U, KontermannRE. The making of bispecific antibodies. MAbs. 2017;9:182–212. doi:https://doi.org/10.1080/19420862.2016.1268307.
- Dotti G, Gottschalk S, Savoldo B, Brenner MK. Design and development of therapies using chimeric antigen receptor-expressing T cells. Immunol Rev. 2014;257:107–26. doi:https://doi.org/10.1111/imr.2013.257.issue-1.
- Krivov GG, Shapovalov MV, Dunbrack RL Jr. Improved prediction of protein side-chain conformations with SCWRL4. Proteins. 2009;77:778–95. doi:https://doi.org/10.1002/prot.v77:4.
- Naim M, Bhat S, Rankin KN, Dennis S, Chowdhury SF, Siddiqi I, Drabik P, Sulea T, Bayly CI, Jakalian A, et al. Solvated interaction energy (SIE) for scoring protein-ligand binding affinities. 1. Exploring the parameter space. J Chem Inf Model. 2007;47:122–33. doi:https://doi.org/10.1021/ci600406v.
- Sulea T, Purisima EO. The solvated interaction energy method for scoring binding affinities. Methods Mol Biol. 2012;819:295–303.
- Guerois R, Nielsen JE, Serrano L. Predicting changes in the stability of proteins and protein complexes: a study of more than 1000 mutations. J Mol Biol. 2002;320:369–87. doi:https://doi.org/10.1016/S0022-2836(02)00442-4.
- Schymkowitz J, Borg J, Stricher F, Nys R, Rousseau F, Serrano L. The FoldX web server: an online force field. Nucl Acids Res. 2005;33:W382–388. doi:https://doi.org/10.1093/nar/gki387.
- O’Conchuir S, Barlow KA, Pache RA, Ollikainen N, Kundert K, O’Meara MJ, Smith CA, Kortemme T. A web resource for standardized benchmark datasets, metrics, and Rosetta protocols for macromolecular modeling and design. PLoS One. 2015;10:e0130433. doi:https://doi.org/10.1371/journal.pone.0130433.
- Rohl CA, Strauss CE, Misura KM, Baker D. Protein structure prediction using Rosetta. Methods Enzymol. 2004;383:66–93.
- Sulea T, Vivcharuk V, Corbeil CR, Deprez C, Purisima EO. Assessment of solvated interaction energy function for ranking antibody-antigen binding affinities. J Chem Inf Model. 2016;56:1292–303. doi:https://doi.org/10.1021/acs.jcim.6b00043.
- Schuck P. Size-distribution analysis of macromolecules by sedimentation velocity ultracentrifugation and lamm equation modeling. Biophys J. 2000;78:1606–19. doi:https://doi.org/10.1016/S0006-3495(00)76713-0.
- Brautigam CA. Calculations and publication-quality illustrations for analytical ultracentrifugation data. Methods Enzymol. 2015;562:109–33.