ABSTRACT
Antibody drugs should exhibit not only high-binding affinity for their target antigens but also favorable physicochemical drug-like properties. Such drug-like biophysical properties are essential for the successful development of antibody drug products. The traditional approaches used in antibody drug development require significant experimentation to produce, optimize, and characterize many candidates. Therefore, it is attractive to integrate new methods that can optimize the process of selecting antibodies with both desired target-binding and drug-like biophysical properties. Here, we summarize a selection of techniques that can complement the conventional toolbox used to de-risk antibody drug development. These techniques can be integrated at different stages of the antibody development process to reduce the frequency of physicochemical liabilities in antibody libraries during initial discovery and to co-optimize multiple antibody features during early-stage antibody engineering and affinity maturation. Moreover, we highlight biophysical and computational approaches that can be used to predict physical degradation pathways relevant for long-term storage and in-use stability to reduce the need for extensive experimentation.
Introduction
Antibodies and antibody-derived proteins are immensely successful as therapeutics. In 2021, we witnessed the 100th market approval of an antibody drug by the U.S. Food and Drug Administration (FDA).Citation1 It is expected that this number will expand quickly in the coming years fueled by the hundreds of antibodies in clinical development.Citation1 Impressively, there are also 800 antibody-based molecules with international nonproprietary names (INNs), approximately half of which were listed in the past 5 years.Citation2 Most of these drugs have a conventional immunoglobulin G (IgG) structure, but there is an increasing interest in modalities such as bispecifics, antibody-drug conjugates, nanobodies and other immunoglobulin classes.Citation2–6
Owing to decades of research, the antibody technology has matured to a stage where new monoclonal antibodies (mAbs) can be developed and manufactured at unprecedented speed.Citation7 For instance, several new antibody therapeutics for the treatment of COVID-19 became available to patients only 10–24 months after the start of the pandemic.Citation8
Notwithstanding these successes, there are still many outstanding challenges in antibody development. For example, antibodies with desired antigen specificity can exhibit poor physicochemical properties and low stability that hinder their development as therapeutics.Citation9,Citation10 Some issues (e.g., poor solubility, high viscosity) can sometimes be solved by formulation development,Citation11 while others (e.g., non-specific binding) can only be mitigated by modifying the antibody molecule.Citation12 Therefore, it is crucial that, in addition to functionality, the right antibody candidate with suitable drug-like biophysical properties is selected as early as possible. As a response to this requirement, the concepts of developability assessment and drug-like antibodies emerged.Citation13–17
Traditional antibody development starts with the isolation of many mAbs with desired antigen specificity, followed by candidate characterization to identify leads that should move forward to lead validation or optimization.Citation18–20 Often, an optimization step is necessary – either to increase the binding affinity or to mitigate physicochemical issues intrinsic to the lead candidates.Citation21 The challenge is to identify and optimize mAbs that combine desired binding affinity, high specificity, excellent stability, and other favorable physicochemical properties.Citation22
The toolbox used in developability assessment and optimization of antibodies has rapidly expanded over the past decade.Citation18,Citation19 However, standard approaches to antibody drug development still have drawbacks. For example, it is possible that none of the isolated candidates exhibits all desired drug-like properties and that optimization efforts result in iterative cycles where mutations in the molecule improve one property but hamper another.Citation22,Citation23 In addition, it is still impossible to measure all physicochemical and stability issues in mAbs with the scarce material available during early-stage development. This aspect is further challenged by the fact that all the physiochemical and stability problems are not intrinsic properties of the protein sequence but strongly depend on the formulation and the different stresses experienced during the production and life cycle of the drug. It is therefore highly desirable to integrate new methods into antibody development.
Here, we summarize a selection of emerging techniques that can complement the conventional toolbox used in antibody therapeutic development. These approaches can bring several improvements such as (i) increasing the likelihood of discovering target-specific antibodies that also have favorable physicochemical properties, (ii) rational co-optimization of multiple antibody features (i.e., binding affinity, specificity, stability), (iii) prediction of physical degradation pathways relevant for long-term storage and in-use stability, and (iv) reduction of the number of lab experiments by using rapid computational methods that have no material requirements.
Drug-like properties of antibodies
Drug-like antibodies exhibit suitable features for development, manufacturing, and administration in patients. Some desired physicochemical properties will depend on the target product profile (TPP).Citation24 For example, an antibody that requires subcutaneous administration of large doses to achieve therapeutic effect must exhibit excellent solubility and low viscosity at high concentrations.Citation25 In contrast, the viscosity at high concentrations will be less important for antibodies administered intravenously.Citation26 Unfortunately, the TPP is not always clear during the early stages of antibody discovery and development which complicates the definition of all important physicochemical features.
Despite the product-related differences, there are some general requirements that apply for most antibody drug candidates.Citation20,Citation22 For example, high binding affinity to the pharmacological target is a requirement for antagonist antibodies, while the absence of toxicity, low immunogenicity, and high binding specificity will be important for most antibody drug candidates.Citation20 Similarly, high production yields are desirable for all antibody drugs. Next, the candidates should exhibit good aqueous solubility and high colloidal stability manifested as low tendency for self-association and aggregation. For example, high self-interactions are correlated to formulation issues such as opalescence or elevated viscosity at high protein concentrationsCitation27 and aggregation during long-term storage.Citation28 Colloidal stability will also play a role during standard production steps such as tangential flow filtration and upconcentration.Citation29 In addition, it is important that the candidates have sufficient conformational stability that ensures that the unfolded state populations will be minimal at ambient conditions for biomanufacturing, storage, and postproduction handling, and also because unfolded molecules are non-functional and often immunogenic.Citation30 Unfolded states, by exposing to the solvent hydrophobic patches typically buried in the native structure, are particularly prone to aggregation and therefore even small populations of them can result in substantial formation of aggregates over time.Citation31 A drug-like antibody ideally exhibits low heterogeneity after secretion from mammalian cells, as well as sufficient resistance to aggregation and unfolding at low pH that is used to elute the bound antibodies during affinity chromatography and for subsequent viral inactivation.Citation32 In addition, interfacial stability related to adsorption and unfolding at different interfaces is not only important during manufacturing but also for the so-called “last mile” where the antibody is subjected to postproduction handling (dilution, mixing, in-hospital transport) that is particularly likely to cause interfacial stress.Citation33 Just as importantly, drug-like antibodies will exhibit high chemical stability displayed as lower tendency for chemical changes, such as fragmentation, oxidation, deamidation, and isomerization.Citation34
These basic examples of desired drug-like properties illustrate just some of the many aspects that must be considered during antibody development. Predicting all the desired biological and physicochemical properties from antibody sequences is considered a “Holy Grail” in antibody drug development. Unfortunately, only some known liabilities can be identified directly from the sequence; for example, these could be motifs related to accelerated chemical degradationCitation34 or polyreactivity.Citation12 Other liabilities such as polyspecificity, tendency for non-native aggregation, or poor conformational stability are generally harder to predict accurately, and therefore are often screened for in high-throughput biological and biophysical assays.Citation9,Citation35 Conversely, poor interfacial stability can become evident only much later in pre-clinical development when production processes are scaled up and the drug product is filled into the intended primary package.Citation36
The definitions and classification of drug-like properties of antibodies are still developing. For example, polyreactivity and polyspecificity were historically used as synonyms. However, Cunningham et al. proposed that polyreactivity describes the general non-specific stickiness of antibodies, while polyspecificity refers to off-target specific interactions with a discrete number of biomolecules different from the main target.Citation37 Although both phenomena are related to non-specific binding and could affect the pharmacokinetic properties of the mAbs, polyreactivity can be tested in vitro with general reagents (e.g., cell lysates, nucleic acids), while polyspecificity is more difficult to investigate since not every possible off-target in the human body can be included in in vitro screening assays.Citation37 Readers interested in the non-specific binding of antibodies can read a recent review focusing on the molecular origins of this phenomenon.Citation38
The discovery and selection of drug-like antibodies require orthogonal methods. A toolbox based on well-established biophysical and computational techniques is increasingly adopted by the pharmaceutical sector.Citation10,Citation18–20,Citation39,Citation40 Even if these approaches have brought great improvements to antibody development, there are still many unmet needs, including: (i) discovery approaches that yield more drug-like antibodies with desired specificities, (ii) high-throughput candidate optimization to find mutations that enhance multiple desired properties, and (iii) accurate experimental prediction of multiple liabilities from scare material amounts.
Discovery approaches yielding highly developable antibodies
The hybridoma technique introduced in the 1970s enabled researchers to isolate and produce large amounts of purified mAbs with desired specificities.Citation41 This method was used to discover many of the antibody drugs on the market today.Citation42 However, the approaches to find new antibodies have developed significantly in the last three decades.Citation43 For example, sequences of antigen-specific mAbs can be identified directly from B-cells derived from immunized (transgenic) animals or convalescent patients.Citation44,Citation45 Furthermore, antigen-specific mAbs can be obtained by screening large immune, naïve, or (semi-)synthetic antibody libraries via phage display, bacterial display, ribosome display, yeast surface display or mammalian surface display.Citation46 These different antibody discovery strategies can be biased towards yielding mAbs with different physicochemical properties.Citation9,Citation47 Therefore, the selection of antibodies with drug-like features starts already at the earliest discovery stages.
The use of antibody libraries and display technologies enables new approaches to identify mAbs that combine desired antigen specificity and favorable physicochemical properties. We will discuss in particular (i) libraries that are designed to contain antibody sequences with fewer liabilities, (ii) selection steps to deplete screened libraries of candidates that exhibit issues such as high aggregation propensity or polyreactivity, and (iii) mammalian cell display to select developable mAbs.
Rationally designed antibody libraries
Traditional antibody libraries contain an enormous number of antibody sequences. Not surprisingly, the candidates in these libraries can have unpaired cysteines, deamidation hotspots or motifs related to non-specific binding that are not desirable from a developability perspective.Citation48,Citation49 Therefore, it is very attractive to design antibody libraries that contain sequences with fewer liabilities.
The value of designed libraries for the selection of developable antibodies was recently demonstrated by Teixeira et al.Citation48 In this study, next-generation antibody libraries were prepared by the grafting of natural human complementarity-determining regions (CDRs) onto well-behaved scaffolds (). The HCDR3 sequences were obtained directly from B cells of healthy donors. The remaining CDRs were obtained from next-generation sequencing datasets by excluding CDRs that contain known liability amino acid motifs and selecting the sequences with good expression using yeast display of single-CDR libraries. Ultimately, the resulting libraries were used to isolate high-affinity binders against several antigens. Most importantly, the identified antibodies exhibited favorable behavior in several assays probing the specificity, thermal stability, self-interaction, or aggregation propensity.Citation48 Interestingly, similar designed library approaches can also be used for simultaneous affinity maturation and removal of sequence liabilities from lead antibody candidates.Citation50
Figure 1. Approaches to discover antibody candidates with fewer physicochemical liabilities. a Rationally designed antibody libraries can be created by selecting HCDR1-2 and LCDR1-3 sequences without known sequence-based liabilities, screening the selected sequences as single-CDR libraries for expression on yeast and combining the sequences with well-behaved human scFv scaffolds and diverse HCDR3 sequences obtained from human donors. b VHH phage libraries can be depleted of aggregation-prone sequences before biopanning to increase the probability of selecting VHH with resistance to aggregation. c Integration of antibody genes into a single locus allows transcriptional normalization and comparison of antibody variants based on their expression level on the surface of mammalian cells. High display level of the antibodies is correlated with favorable biophysical properties.
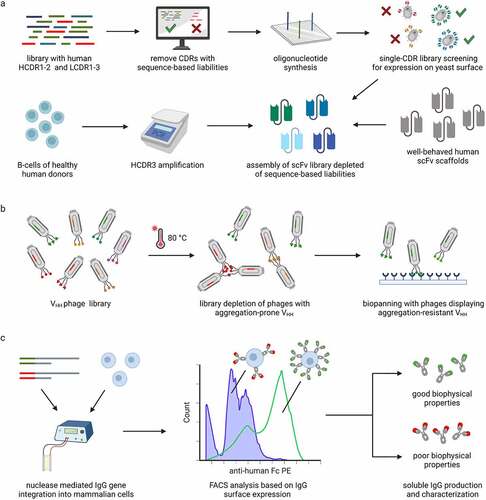
Another compelling study focused on the design of smart nanobody libraries.Citation51 The authors developed an autoregressive model that was trained on naïve nanobody repertoires from llamas. The model was used to predict functional constraints in the sequences and to design a virtual library of 107 fit nanobody sequences. The library was then purged of undesired sequences such as candidates that are likely to have a post-translational modification in the HCDR3. Finally, 185,836 nanobody sequences were selected and used to create a small but diverse synthetic library. The designed library was expressed well on the surface of yeast and yielded a nanobody specific for human serum albumin. Although the affinity of the binder was low (estimated Kd of 9.8 µM), this study demonstrates the feasibility of a promising approach to design smart nanobody libraries that contain fit and carefully selected sequences.Citation51
Removing candidates with liabilities by experimental selection
Selection rounds aimed at depleting libraries of candidates with undesired properties can facilitate the discovery of antibodies with fewer physicochemical liabilities.Citation52,Citation53 Importantly, each display strategy offers different selection opportunities that depend on the compatibility of the technique with the conditions needed to select for a certain property.
For example, Jespers et al. reported a strategy to identify aggregation-resistant antibody heavy chain variable domains (VHHs) by stressing phage-displayed VHHs via exposure to high temperatures of up to 80°C ().Citation54 Interestingly, they found that VHHs selected by this approach can exhibit remarkable resistance to aggregation coupled to fully reversible thermal unfolding.Citation54 Later, this methodology was used to define general strategies to engineer aggregation-resistant VH and VL domains by introducing negative charges at specific positions.Citation55 More importantly, the selected aggregation-resistant antibody domains also exhibited a set of other desirable biophysical properties such as heat-refoldability, shortened elution time in size-exclusion chromatography and high-expression yields from bacterial systems.Citation55 Noteworthily, the selection on phage can also be based on other types of stress. For example, the phage library can be incubated at low pH to select candidates that are stable during low-pH viral inactivation.Citation56
Yeast surface display can also be combined with selection rounds to obtain antibodies with desired physicochemical properties. In particular, the combination of yeast surface display and flow cytometry can be used to screen for non-specific binding of candidates at the library level.Citation55 For example, Kelly et al. used yeast surface display and fluorescence-activated cell sorting (FACS) to dissect a nonimmune human antibody library into a population that exhibits non-specific binding and a population that does not exhibit non-specific binding to four reagents.Citation57 Interestingly, the authors found that members of the VH6 family exhibit high level of non-specific binding suggesting that this family can be excluded from libraries aimed at isolating developable antibodies. In an analogical way, molecular counter selection during yeast surface display can be used to remove antibody candidates that bind to polyreactivity reagents while still allowing the discovery of high-affinity binders.Citation58
Mammalian cell surface display to select developable antibodies
The mammalian display technology has developed significantly over the last two decades.Citation46,Citation59 Importantly, the advances in gene editing techniques have allowed the integration of a single antibody gene at a defined locus while allowing the display of millions of antibody variants in a cell population.Citation60 By using targeted integration of the antibody genes, transcriptional normalization is achieved and the differences in the display level can be attributed to the properties of the antibodies. Key opportunities offered by contemporary mammalian cell display technologies were demonstrated in a recent study by Dyson et al.Citation61 The authors used nuclease-mediated integration of antibody genes into a single locus and elegantly showed that higher level of antibody display on the mammalian cell membrane was correlated to improved biophysical properties such as higher solubility and lower self-interaction propensity ().Citation61 In contrast, the display level on yeast could not be used to distinguish between the antibodies with different biophysical properties which was explained by specific differences in how the antibodies are displayed on the yeast cell wall and the mammalian cell membrane.Citation61 In addition, Dyson et al. showed that their mammalian cell display approach can be combined with molecular counterselection for non-specific binding to heparin and heat-shock proteins to identify polyreactive antibodies therefore offering the possibility to select antibodies with both favorable biophysical properties and high specificity directly from mammalian cell display.Citation61
Rational optimization of antibody candidates
Novel discovery approaches can yield antigen-specific mAbs with fewer physicochemical issues, but it is still possible that some of these candidates need further optimization. A major challenge is to select mutations that simultaneously improve multiple features in an antibody while maintaining or increasing the binding affinity to the target.Citation22,Citation62 Predicting the effects of combinations of several mutations can be particularly difficult. Therefore, it is highly desirable to use experimental methods that interrogate a broad mutational space to find which combinations of mutations lead to the desired enhancement of antibody features. A plethora of techniques for rational optimization of antibody binding, often together with enhanced soluble expression in bacteria or improved stability, have been reported.Citation63–66 Here we focus on the recent advances in phage-assisted continuous evolution (PACE),Citation67 tripartite β-lactamase enzyme assays (TPBLAs),Citation68 autonomous hypermutation yeast surface display (AHEAD) and machine learning (ML) for the optimization of antibodies.Citation43
Phage-assisted continuous evolution
In PACE, E.coli cell suspension constantly flows through a fixed-volume vessel that contains a population of phage DNA vectors called selection phage (SP) ().Citation67 The gene of a protein that will be optimized is incorporated in the SP by replacing the pIII gene encoding a protein important for phage infectivity. Instead, the pIII gene is included in an accessory plasmid in the host bacteria, and pIII production is made dependent on the properties (e.g., activity) of the protein that is being optimized. The mutation rate in the system is increased by including a mutagenesis plasmid in the bacterial cells. As a result, novel mutations in the evolving proteins occur and only phage vectors with optimized variants that induce sufficient pIII production will propagate quickly in the vessel and avoid being washed away due to the continuous dilution with fresh bacterial suspension.Citation67
Figure 2. Approaches to select mutations that optimize antibody properties. a Schematic overview of phage assisted continuous evolution. Host bacteria contain accessory plasmid with the pIII phage gene needed for infectivity and a mutagenesis plasmid. The host bacteria flow constantly through a vessel (“lagoon”) containing selection phages that contain the gene of interest (GOI). The production of the pIII is dependent on properties of the GOI. Only selection phages that encode functional variants of the GOI produce pIII and infectious progeny that can reinfect new host bacteria to remain in the “lagoon”. Phages with non-functional variants cannot infect new host bacteria before being washed away from the flow. b In the tripartite β-lactamase enzyme assay, scFv sequences are inserted between two domains of β-lactamase. If the scFv are resistant to aggregation in the bacterial periplasm, the split β-lactamase can fold into a functional form and the bacteria gain resistance to ampicillin. Selection of different variants can be made by assessing the growth of bacterial clones on agar plates with different ampicillin concentrations. c Machine learning model trained on a library with >107 emibetuzumab Fab variants sorted for binding affinity and specificity can predict mutations that co-optimize antibody affinity and specificity.

PACE has been developed further and applied to optimize antibody fragments. For example, Wang et al. developed a soluble expression PACE (SE-PACE) by introducing an AND gate based on split-intein pIII that allows two simultaneous positive selections.Citation69 The authors used SE-PACE to optimize disulfide-free antibody fragments and were able to preserve the target-binding affinity while improving thermodynamic stability and the soluble expression of a single-chain variable domain (scFv) in bacteria. However, a drawback of PACE and SE-PACE is that the selections are performed in cytoplasm, and therefore, the optimizations are efficient for antibody fragments lacking disulfide bonds.Citation69 To solve this limitation, Morrison et al. reported periplasmic PACE (pPACE) that is suitable for the optimization of disulfide-containing proteins.Citation70 The authors established pPACE by exploiting fusion strategies to the CadC sensor to link protein properties in the periplasm to transcriptional activation in the cytoplasm. They then used scFv Ω-graft antibody mutants with different affinity for a leucin zipper antigen to demonstrate that variants with higher binding affinity are enriched during pPACE.Citation70 Next, they developed a split-intein signal peptide pPACE to also control the export to the periplasm and apply simultaneous selection pressure for both soluble periplasmic expression and target binding. Using pPACE, the authors were able to discover mutations that optimize the binding affinity and soluble periplasmic expression of scFvs from trastuzumab and a Ω-graft antibody.Citation70 Due to the working principle of pPACE, the main applications of the method are expected to be in the optimization of antibodies against antigens that are compatible with periplasmic expression.
Tripartite β-lactamase enzyme assay
TPBLA links the aggregation of a protein of interest to bacterial antibiotic resistance.Citation71 The TPBLA was recently adapted by Ebo et al. to study the aggregation propensity of antibodies ().Citation68 In their assay, the scFv sequences are inserted between two domains of the periplasmic β-lactamase of E.coli. As a result, aggregation-prone scFv sequences result in non-functional β-lactamase fusions leading to lower ampicillin resistance. This allows the ranking of scFv variants by studying the growth behavior of E.coli clones in the presence of antibiotics. The authors first used model scFvs with different aggregation propensity to demonstrate that scFv aggregation correlates with bacterial survival. Next, they applied the TPBLA in a directed evolution experiment to identify mutations that reduce the aggregation propensity of scFvs. To this end, they used error-prone PCR to generate mutants of a model scFv. Bacterial cells were then transformed using the mutated library and selected on ampicillin agar. Remarkably, 185 variants bearing mutations were identified, 181 of which displayed enhanced bacterial growth compared to wildtype, and 12 were better than the best known rationally optimized triple scFv mutant. Most importantly, the authors showed that the ranking of the scFvs based on bacterial growth correlated with the aggregation and self-association propensity of the respective IgGs, while the binding to the target of 10 randomly selected optimized variants was preserved.Citation68 An interesting finding of the study was that TPBLA can be used to identify mutational hotspots in the antibody fragments that can be targeted in further optimization experiments.
Quite generally, while very powerful, these approaches that rely on random mutations to generate diversity for selection do not safeguard against the occurrence of immunogenic motifs or of chemical and post-translational liabilities.
Autonomous hypermutation yeast surface display
In addition to bacterial systems, there are notable, new yeast-based approaches for rational antibody optimization. For example, Wellner et al. reported AHEAD – a yeast-based method for in vitro affinity maturation of antibody fragments.Citation72 The technology uses an error-prone DNA polymerase that replicates a specific cytosolic plasmid (p1) which propagates stably in Saccharomyces cerevisiae. When antibody sequences are included in p1, the specific DNA polymerase diversifies the antibodies by continuous mutation, while the yeast surface display allows selection of candidates with improved binding affinity via FACS. The first version of AHEAD suffered from low levels of surface display of the inserted sequences. To solve this issue, Wellner et al. developed AHEAD 2.0 by changing the fusion position of the antibody sequences in the yeast Aga2p, using a new p1-specific promoter, introducing a stronger secretory leader sequence, and incorporating a polyadenosine tail.Citation72 These modifications improved the surface display of nanobodies by about 25-fold. Next, the authors used AHEAD 2.0 to improve the binding affinity of several nanobodies against SARS-CoV-2. The authors parallelized eight affinity maturation experiments. After three to eight cycles of yeast culturing and FACS selection, multiple high affinity binders were isolated. Virus neutralization assays revealed that the neutralization potencies were increased up to 925-fold for the AHEAD-optimized nanobodies.Citation72
Machine learning for rational antibody optimization
ML models can largely support the discovery and engineering of antibodies in essentially all steps, from rational and de-novo design to developability and formulation optimization.Citation39 ML possesses the ability to learn input-output relationships with low computational time and resource requirement, even when the underlying physical principles are not completely understood. These features can significantly accelerate the discovery and developability process.
One recent report demonstrated how an existing clinical-stage antibody (emibetuzumab) could be co-optimized for both high affinity and low non-specific binding using machine learning ().Citation73 This approach involved generating a multi-site mutant library of >107 emibetuzumab variants with mutations in the heavy chain CDRs. The resulting library was displayed on the surface of yeast in the Fab format and sorted for high and low levels of on-target (affinity) and off-target (non-specific) binding using FACS.
The enriched libraries for each property were deep sequenced and machine learning classification models were trained to predict the class (high or low) of each property (affinity and non-specific binding) for every mutant in the library.Citation73 Interestingly, the investigators found that relatively simple models, such as linear discriminant analysis models, could be used not only to predict the class of each property but also continuous variables that were strongly correlated with both affinity and non-specific binding. The ability to predict intraclass variability (e.g., high vs. very high affinity) is critical when attempting to solve challenging co-optimization problems that are common in antibody engineering. Indeed, the investigators used the continuous predictions from the affinity and non-specific binding models to identify rare antibody mutants with co-optimal combinations of high affinity and low non-specific binding located at the Pareto frontier. This type of approach is particularly exciting because it even enables the prediction of the impacts of novel mutations that were not sampled in the original library, which greatly expands the sequence space that can be explored to improve antibody properties ().
While thisCitation73 and related studiesCitation74,Citation75 focused on library sorting and machine learning methods for co-optimizing two specific antibody properties, namely affinity and non-specific binding, it should be straightforward to apply these and related approaches to other combinations of antibody properties. Indeed, it was recently shown that antibody affinity and self-association can be co-optimized at the library stage by enriching antibody libraries for high antigen binding and low binding to novel quantum dot-IgG conjugates.Citation76 The quantum dots (QDs) were conjugated to a high self-association IgG (lenzilumab), and low QD-lenzilumab binding to the yeast-displayed library served as a surrogate for low antibody self-association. The investigators used this approach to identify rare variants of a clinical-stage antibody (bococizumab) with high affinity and low self-association in physiological conditions (pH 7.4, PBS). Moreover, tradeoffs between antibody affinity and stability are common during affinity maturation,Citation77,Citation78 and FACS-based methods for selecting thermostabilized antibodies from yeast-displayed librariesCitation79,Citation80 can be used to select antibodies with co-optimized combinations of affinity and stability.Citation81 Related approaches have also been reported using mammalian (CHO)-based antibody display technologies.Citation82
In another example, deep neural networks were applied to predict antigen specificity from a diverse space of antibody variants of trastuzumab.Citation83 After training the model, the authors could identify a subset of variants which not only were specific to human epidermal growth factor receptor 2 (HER2) but could also be filtered for viscosity, clearance, solubility, and immunogenicity. Overall, from a computational library of approximately 108 trastuzumab variants, this method identified thousands of optimized lead candidates. These examples show the potential of deep learning in assisting antibody design, engineering, and optimization by analyzing large amounts of data.
Orthogonal approaches for the selection of drug-like antibodies
The discovery and optimization strategies described in the previous paragraphs typically lead to 10–1000 candidates with comparable binding affinity and specificity. The identification of the lead molecule among these candidates requires a further selection step based on the analysis of multiple drug-like properties, possibly improved via optimization of formulation. These properties, globally defined as “developability” of the drug, include several properties related to intermolecular interactions, solubility, conformational stability, interfacial stability and non-native aggregation. No single property can predict the success of a potential drug-like candidate in advancing clinical stages, which rather depends on the overall behavior of the molecule with respect to all these multiple properties.Citation9,Citation35 These physicochemical properties should be therefore globally evaluated and optimized. A non-comprehensive selection of key properties and recently developed assays to evaluate them is proposed in the following. Particular attention has been given to methods that require micrograms or even nanograms of crude antibody material and are therefore attractive during early-stage screening.
A different perspective on the non-specificity of antibody drugs
A particularly important property already addressed during the discovery stage is the non-specific binding of antibodies, which is related to poor solution behavior in vitro and faster clearance in vivo.Citation9,Citation37,Citation84 Therefore, it is essential to evaluate whether antibody candidates exhibit non-specific binding to molecules different from their target. In these assays, different polyreactivity reagents such as cell lysates, nucleic acids, heparin or purified unrelated proteins are used. Antibodies can exhibit non-specific binding not only to macromolecules but also to low molecular weight compounds (such as enzymatic cofactors), which are often overlooked in polyreactivity assays for antibody drugs.Citation85,Citation86
A recent study by Lecerf et al. revealed that some clinical-stage antibodies interact with heme.Citation87 Heme is an abundant prosthetic group with a porphyrin structure and a molecular mass of 616 Da. Strikingly, the binding to heme-induced polyreactivity in some of the antibodies illustrates that non-specificity can be potentiated by small molecules that are abundant in the human body.Citation87 In addition, the authors reported that binding to heme correlates with hydrophobicity, self-association tendency and intrinsic polyreactivity.Citation9,Citation87 Interestingly, several antibodies in the study by Lecerf et al. also interacted with another small molecule, folate, hinting that therapeutic mAbs might be predisposed to non-specific interactions with different heterocyclic compounds.
Traditionally, the non-specific binding is investigated for the purified, native antibody candidates. However, it has been known for some time that the polyreactivity of antibodies can change upon chemical changes such as oxidation.Citation88–90 Recently, Lecerf et al. demonstrated that exposure to pro-oxidative agents such as ferrous and hypochlorite ions can induce significant non-specificity in clinical-stage antibodies.Citation91 Since oxidation in therapeutic antibodies can occur during manufacturing, storage, postproduction handling or even in vivo post administration,Citation92 the oxidation-induced polyreactivity antibody drugs might be another aspect that should be understood better and considered during developability assessment.
Overall, the increase in non-specificity upon interaction with abundant small molecules or upon chemical changes demonstrates a potential unmet need for predictive assays to capture these aspects of antibody non-specificity.
Assessing antibody self-association with low antibody concentrations
It is desirable to prepare antibody therapeutics as concentrated liquid formulations for subcutaneous delivery, but different antibody variants can display highly variable and difficult-to-predict solution properties, including large differences in their viscosity, opalescence, and/or aggregation properties. Therefore, there is broad interest in early-stage assays that can be used for selecting antibody candidates with low risk for possessing undesirable solution properties. Attractive intermolecular interactions, characterized for instance by the self-association parameter (kD) and second virial coefficient (B22), have been shown to correlate to a good extent with viscosity and opalescent behavior at high antibody concentrations.Citation27 There is therefore interest in developing assays capable to measure intermolecular protein–protein interactions with high throughput and consuming a limited amount of sample.
One recently reported assay, namely charged-stabilized self-interaction nanoparticle spectroscopy (CS-SINS), evaluated the self-association of antibodies using immunogold conjugates.Citation93 The assay involves first immobilizing an anti-human Fc capture antibody on gold nanoparticles, along with polylysine to maximize colloidal stability (). Next, the conjugates are used to capture human mAbs and assay mAb self-association by evaluating the shift in the plasmon wavelength of the absorbance spectra. The CS-SINS measurements were conducted in a common antibody formulation condition (pH 6, 10 mM histidine), which is not possible using a related assay (AC-SINS)Citation94–96 due to the instability of the immunogold conjugates in this formulation condition. Importantly, the investigators found that the CS-SINS assay was able to identify antibodies with low risk for high viscosity and opalescence when concentrated to 150 mg/mL.Citation93 The ability of CS-SINS to evaluate antibody self-association at ultra-dilute antibody concentrations (0.01 mg/mL) makes it particularly attractive to employ in early-stage developability studies.
Figure 3. Methods to select antibody candidates with desired physicochemical properties. a Charge-stabilized self-interaction nanoparticle spectroscopy (CS-SINS) can assess the self-association of antibody candidates in common pharmaceutical buffers (10 mM histidine pH 6) at ultra-dilute antibody concentrations (0.01 mg/mL). The CS-SINS score is calculated from the plasmon shift due to self-association of the nanoparticle conjugates and can predict solution properties such as viscosity and opalescence at high antibody concentrations. b Modulated scanning fluorimetry (MSF) employs incremental heating and cooling cycles to simultaneously probe the thermal unfolding and unfolding reversibility of dozens of antibody candidates by consuming only 10 µL per condition. c The nanoparticle-surface mediated stress assay uses nanoparticles with different surface properties. The nanoparticles are mixed and incubated with antibody candidates. The large nanoparticle surface accelerates the surface-induced aggregation of antibodies and allows ranking of candidates based on their interfacial stability.

Another approach with similar advantages has been developed based on asymmetrical flow field-flow fractionation (AF4), a powerful separation technique, in which the samples are injected and focused onto a membrane in a separation channel.Citation97 The membrane is permeable for the buffer components but not for the analyzed biomolecules. Subsequently, the analytes are eluted from the channel under a cross-flow to enable separation based on diffusion coefficients. The focusing step during AF4 increases the concentration of the analytes close to the membrane, thereby, accentuating protein–protein and protein–membrane interactions that affect the elution profile of the analytes.Citation98
Wahlund and colleagues utilized AF4 to study the self-interactions of four immunoglobulins.Citation99 In brief, the mAbs were injected and focused on the semipermeable membrane to increase the concentration and propel the self-interactions of the antibodies. The antibody recovery and retention time in AF4 correlated well with the diffusion interaction parameter kD from dynamic light scattering which is a valuable molecular descriptor of colloidal properties of mAbs.Citation99 There are two valuable features of this AF4 approach – (i) the starting antibody concentration can be low since the concentration is increased during the measurement, and (ii) the buffer of the injected antibody is exchanged for the running buffer in the AF4, which removes the need for a separate buffer-exchange of the candidates.
Estimating non-native aggregation tendency from refoldability assays
In addition to viscosity, solubility and phase separation, another important property of antibodies is stability against aggregation during manufacturing, delivery, and storage. Often aggregation is triggered by a chemical or conformational change of the antibody structure and is therefore defined as non-native.Citation100
The non-native aggregation propensity of antibody candidates is difficult to predict. This is partly due to the complex nature of this degradation pathway that includes multiple elementary steps of nucleation and aggregate growth that are dependent on both structural (e.g., partial unfolding) and colloidal aspects.Citation100 The aggregation mechanisms substantially vary between antibodies and are strongly dependent on extrinsic factors such as temperature and pH.Citation31,Citation101,Citation102 As a consequence, accelerated stability studies at 40°C or higher temperatures may not always be representative of the stability of antibodies at lower storage temperatures.Citation103 Moreover, aggregation stability studies can provide high-quality mechanistic information but are in general time- and material-consuming, and therefore not compatible with high-throughput screening.
The tendency for non-native aggregation of proteins can be estimated from refoldability studies.Citation104 For example, antibody domains and nanobodies that exhibit reversible thermal unfolding are resistant to non-native aggregation.Citation54,Citation105 Traditionally, the thermal unfolding reversibility is studied by differential scanning calorimetry or spectroscopic techniques using a linear heating ramp (to track unfolding) and a subsequent cooling ramp (to track refolding).Citation54 Alternatively, two subsequent heating ramps can be applied to the same sample. However, unfolding reversibility after complete thermal denaturation of scFvs, Fabs and full-length IgGsCitation106 is rare which obstructs the application of this approach for candidate comparison.
Modulated scanning fluorimetry (MSF) extends the capabilities of traditional techniques to study thermal unfolding reversibility ().Citation107 MSF employs fast heating and cooling cycles applied to dozens of samples simultaneously. Every subsequent heating cycle reaches a slightly higher temperature (e.g., 1°C higher) than the previous one while the cooling cycle always ends at the same baseline temperature (e.g., 20°C). This allows the protein to recover from the structural perturbations caused at each heating step. From the fluorescence signal at the baseline, estimations can be made about the peak temperatures that cause irreversible structural changes in a protein. The MSF can be applied to crude samples and a wide range of proteins at concentrations as low as 0.1 mg/mL by consuming only 10 µL per condition.Citation107
The unfolding reversibility can also be determined isothermally by using chemical denaturants such as urea. The denaturants are added in high concentrations to cause substantial antibody unfolding and then the denaturant is removed quickly by dialysis or dilution in multiwell plates.Citation108,Citation109 Subsequently, the amount of monomeric protein is assessed via size-exclusion chromatography and compared to a reference sample to determine the degree of unfolding reversibility. These measurements can be performed at any temperature of interest and the data is complementary to the thermal denaturation data to estimate the non-native aggregation propensity of antibody candidates.Citation110
Interfacial stability
The previous paragraphs described assays to evaluate bulk properties of antibody solutions. Proteins and antibodies have a high propensity to interact and adsorb to different types of interfaces.Citation111 In case of antibodies, interactions with interfaces are detrimental since they can lead to protein loss (and therefore reduced activity) and, in many cases, to formation of protein particles. Air-water and silicon oil–water interfaces are only few examples of the large variety of surfaces that have been demonstrated to induce protein aggregation via promoting heterogeneous nucleation events. For a detailed discussion on the topic, the reader is referred to a recent review.Citation111
In contrast to the large number of assays to measure bulk properties, methods to evaluate interfacial stability are less developed and largely include classical shaking assays or are limited to the most common and well-known interfaces. However, during its life-cycle an antibody can be in contact with a wide range of surfaces, and some of them may be unpredictable or even unknown.
Recently, a nanoparticle-based approach has been developed to assess the stability of antibodies against model surfaces that encode the most common types of interactions, such as electrostatic and hydrophobic interactions ().Citation112,Citation113 The advantage of the approach is the high surface-to-volume ratio provided by the nanoparticles, which allows an accurate control of the chemistry and the amount of surface introduced in solution, minimizing interference from other interactions with the material of the test tube. Moreover, the approach is compatible with a conventional multi-well plate reader format and has therefore good throughput. The assay can identify antibodies with a particular high tendency to interact with different model surfaces, leading to the assessment of an overall interfacial stability score.
Selecting candidates with lower tendency to form subvisible and visible particles
As discussed in the previous sections, proteins can aggregate via complex pathways.Citation31 Several strategies exist to screen for candidates with low tendency to aggregate based on the analysis of protein-protein, protein-interface interactions or refoldability.Citation27,Citation28,Citation93,Citation110 However, it is currently close to impossible to predict the tendency of candidates to form larger aggregates, i.e., subvisible and visible protein particles, during production, storage, and post-production handling.
Consequently, the analytical characterization of subvisible protein particles during product development is essential and is ideally incorporated in early-stage candidate screenings. Not optimizing for candidates as early as possible, i.e., ideally during molecule design, with low tendency to form subvisible protein particles is a gap in product development. One challenge is the need for representative down-scale models of relevant stress conditions that occur during the drug product’s life-cycle, which require only µL of sample. Whereas incubation at elevated temperatures and freeze-thawing are rather straight forward to perform in microplates, the primary packaging has a strong impact on interfacial and mechanical stress on the protein. Progress has been achieved, e.g., by using nanoparticle-based assays for interfacial stress as discussed above. Johann et al. recently reported a shaking stress protocol in microplates that is in agreement with shaking stress performed in vials.Citation114 In addition, the fact that subvisible and visible particles are rarely analyzed during discovery and candidate selection is in many cases limited by the lack of high-throughput analytical techniques which can cope with the very limited sample volumes during early stages of development.Citation115,Citation116
Today, many different analytical techniques are available, which in general can cover the whole size range from nano- to millimeter including the subvisible particle size range from ca. 0.1–100 µm.116 In particular, for late-stage stability studies and batch release,Citation115 typically light obscuration according to USP<788>/Ph. Eur. 2.9.19 for particles >10 µm and >25 µm is performed, which consumes 25 mL of sample, and still ~5 mL when a “low-volume” method according to USP<787> is used. Liquid handler for both light obscuration and flow-imaging microscopy are on the market, but one measurement requires at least several hundred microliters, which is often not available during discovery. Therefore, microplate reader with dynamic light scattering (DLS) is often used,Citation115 which can very sensitively detect particles in the nanometer- and submicron-size range on a qualitative level. However, DLS cannot quantity particles, which impedes a reliable ranking of candidates. Similarly, the turbidity can be measured because the opalescence of solution increases in the presence of light-scattering protein particles. Several methods are available to determine the opalescence in a microplate, including a recently published method using a DLS/static light scattering (SLS) plate reader.Citation117 Therefore, opalescence measurements can be easily included in high-throughput screenings but cannot provide size or concentrations of protein particles.
In contrast, backgrounded membrane imaging (BMI) is available as a method for sizing and counting of subvisible protein particles larger than ~2 µm after filtration onto a membrane in 96-well plate formate.Citation118 Because only ~50 µL are required per well, the method can be integrated into high-throughput screenings. However, sizing and counting is less reliable as compared to flow-imaging microscopy because the boundaries of low-contrast particles might not be detected correctly.Citation118,Citation119 Moreover, low analyzed volumes result in low particle counts and high extrapolation factors, which is a statistical challenge for any particle analysis method.Citation120 Nevertheless, a relative comparison or ranking by using backgrounded membrane imaging or other low-volume methods to rule out bad candidates is often sufficient for early screenings.
Moreover, it was recently demonstrated that particle images obtained from BMI in the dried state after filtration contain valuable morphological information which can be analyzed by machine learning.Citation121 Compressing the morphological information from the particle images into a low-dimensional representation may enable an automated assessment, which would be very valuable in the high-throughput setup. Consequently, insights into not only particle size and concentration but also particle morphology would be available at early stage, leading into a substantial database over time, which could finally help to facilitate the development of future candidates with low tendency to form subvisible protein particles.
Emerging in silico tools to support discovery and selection
In recent years, the availability of increasingly powerful computers and computer algorithms, of rapidly growing amounts of quantitative measurements, and of high-quality sequencing and structural data are enabling the development of disruptive computational methods to support antibody development. These advances are making it possible to complement well-established in vivo (first generation) and in vitro (second generation) methods of antibody discovery with novel in silico (third generation) approaches.Citation122
It is foreseeable that, in the future, most of the antibody discovery and optimization work will happen entirely in silico. At present, however, computational methods of de novo antibody design are still in their infancy. The de novo design of antibody binding using approaches relying on molecular modelling has generally met low success rates and required recursive experimental screenings and large libraries,Citation122–127 which hampers competitiveness with established laboratory-based approaches. Conversely, rapid fragment-based combinatorial approaches showed higher success rates for unstructured epitopes,Citation128–132 and recently also for structured ones.Citation133 However, the resulting binding affinities are still orders of magnitude away from a therapeutically relevant range, the applicability of these approaches is somewhat restricted by the available fragments in their databases, and the resulting designed antibodies may harbor non-human, immunogenic motifs.Citation133
On the other hand, methods to predict and optimize important properties, including developability potential and humanization, are far more mature, and are already offering time- and cost-effective opportunities to streamline hit selection.Citation18,Citation134 Antibody discovery campaigns usually yield many initial hits (100s to 1000s), which are commonly prepared in minute amounts, low concentrations, and relatively low purity.Citation18 Therefore, conducting the full spectrum of developability in vitro assays combined with binding and functional studies remains a challenge. Thus, algorithms that can guide hit prioritization and help rule out poorly behaved antibodies are very useful.
One example is the Therapeutic Antibody Profiler (TAP) proposed by the Deane group.Citation135 The idea behind the approach consists in finding molecular descriptors that distinguish clinical-stage antibodies from human repertoire antibodies. The underlying hypothesis is that clinical-stage antibodies are enriched in favorable developability attributes that are not necessarily present in repertoire antibodies. TAP ultimately uses five developability attributes to classify antibodies according to whether their attributes fall within the distributions of those of clinical-stage therapeutics, akin to the Lipinski’s rule of five used for small molecules. Four of the profiling rules evaluate the CDRs by analyzing length, presence of hydrophobic patches, presence of positively charged patches, and presence of negatively charged patches. The final term considers charge symmetry across the variable domains. More recently, Labute and coworkers proposed a modified version of this method, which improves in particular the charge descriptors.Citation136 These approaches, rather than predicting individual biophysical properties, provide a holistic view of developability potential by assessing the similarity of a new candidate to clinical-stage antibodies.
As with the Lipinski’s rule of five, the thresholds themselves should not be interpreted as hard rules, and the distance of candidates from the boundaries of the distributions of clinical-stage antibodies should be considered. Besides, advances in process development and formulation may redefine these boundaries, which may also differ for new antibody drug candidates, depending for instance on the required dosage and formulation concentration. Another limitation of these approaches is that not all clinical-stage antibodies are devoid of biophysical or developability liabilities.Citation9,Citation137 For example, a recent work predicted that 27% of approved antibodies, and almost 40% of post-phase-ii not-yet-approved antibodies have at least one developability flag.Citation137
Another class of computational methods to assist the assessment of developability potential aims at predicting directly specific properties that are associated with developability. Examples include the detection of chemical liabilities or of sites prone to post-translational modification, and the prediction of properties like humanness or immunogenicity, stability, solubility, viscosity, and poly-specificity. Many of these methods, and in particular those focusing on the prediction of aggregation propensity, have recently been reviewed elsewhere and are listed in comprehensive tables.Citation18,Citation134
All methods discussed so far can also assist antibody engineering, that is the design of mutations that improve the molecular trait(s) of interest on an antibody, for example during the lead optimization phase. However, to use them for this purpose, one should generate in silico mutational variants of the wildtype antibody, and then score these using the relevant method(s) to identify mutations predicted to improve the property(ies) of interest. This process is far from straightforward, first because most of these methods do not provide direct insights into which sites should be mutated, and second because molecular traits are very often conflicting, as mutations that improve one tend to worsen the others.Citation122
The co-optimization of multiple molecular traits remains a largely open challenge. Even experimentally, methods of affinity maturation are routinely used to optimize binding affinity, but this often comes at the expenses of other properties that underpin developability potential, including stability, solubility and polyreactivity.Citation50,Citation73,Citation77,Citation78 Multi-trait co-optimization has been compared to solving a Rubik’s cube, where each face represents one biophysical property. Changing one face will affect other faces, often detrimentally, and solving a single face is much simpler than completing the puzzle.
Computational approaches offer a promising avenue to address this challenge, as they allow for highly controlled parallel screenings of multiple biophysical properties. Moreover, computer calculations are rapid, inexpensive, and have no material requirements, while their ability to pinpoint specific mutations reduces cost and environmental impact of downstream experiments by massively lowering the number of candidates for screening. Taken together, these advantages make the implementation of computational methods in antibody and protein development pipelines particularly attractive.
A recently proposed approach contributes to addressing this challenge by introducing a fully automated computational strategy for the simultaneous optimization of solubility and conformational stability, which have been reported to be conflicting in many cases,Citation122,Citation138–140 while not affecting binding. The method, called CamSol Combination,Citation141 works by removing surface-exposed aggregation hotspots leading to poor solubility, and by proposing mutations expected to increase conformational stability and solubility, thus decreasing the population of partially or fully unfolded states in solution. The approach leverages phylogenetic information to reduce false-positive predictions using an ad-hoc recipe for antibody variable domains, thus reducing the chances of introducing non-human potentially immunogenic mutations. Then, the pipeline relies on the CamSol methodCitation142 to carry out predictions of solubility changes upon mutation, and on the energy function Fold-XCitation143 for the predictions of the associated stability changes. The method was validated experimentally by expressing 42 designed variants of six different antibodies: three nanobodies and three scFvs, two of which are approved therapeutics.
Quite generally, hits with the best functionality obtained from screening campaigns are often not those with the best developability profile. Therefore, this type of algorithms that can guide antibody engineering by suggesting specific mutations that can improve developability without affecting functionality are potentially very useful, especially when used in synergy with increasingly accurate approaches of antibody structural modellingCitation144–147 and paratope prediction.Citation148–150
Outlook and outstanding challenges
Here, we summarized a selection of methods that can facilitate the discovery, optimization, and selection of drug-like antibodies. Despite the impressive progress in technologies applied to antibody drug development, there are still many outstanding challenges. Even state-of-the-art antibody discovery platforms can sometimes yield candidates with desired biological activity but poor physicochemical properties. Techniques that yield only antigen-specific antibodies with drug-like features will be invaluable to streamline future drug development. Currently, many important physicochemical properties and stability aspects of mAbs cannot be predicted from the primary sequence. The predictions of antibody properties from sequences and in different formulations are expected to improve significantly as new large experimental datasets and analysis algorithms emerge. It is expected that advances in microfluidic technology will contribute to expand the quality and quantity of the experimental datasets.Citation151,Citation152 With more accurate sequence-based developability assessments, it will be possible to significantly reduce the number of candidates to be expressed for experimental characterization.Citation134 Still, biophysical techniques will be needed to validate the drug-like features of lead candidates. The challenge is to develop a portfolio of methods that can predict all physicochemical and stability issues of antibodies. This analytical toolbox will ideally work with micrograms or even nanograms of crude antibody material.Citation20
In addition to candidate selection and optimization, it will be essential to improve formulation development approaches for antibody candidates. For example, another powerful application of computational approaches based on ML is the possibility to reduce the number of experiments required to optimize a target biophysical property of an antibody. For instance, Narayanan et al. developed a Bayesian algorithm to simultaneously optimize conformational stability and interfacial stability of single-chain variants by optimizing the formulation.Citation153 The algorithm could identify the minimal concentration of surfactant required to optimize both properties. The algorithm identifies the optimum target with a reduced number of experiments with respect to traditional design of experiments, allows to use prior knowledge and identified trade-off between multiple properties. Overall, it can accelerate design of formulation to optimize multiple biophysical properties of antibodies.
Another outstanding challenge is that antibody-based therapeutics are becoming more diverse. For example, antibody fragments, different antibody classes (e.g., IgG2, IgG4 and IgM), and multi-specific formats are becoming increasingly popular. However, switching the antibody format (e.g., from full-length IgG to scFv) or the antibody class can affect developability potential. Methods to predict the developability potential of antibodies upon format change or class switching will be very valuable in future. In addition, bi- and multi-specific antibodies enable novel therapeutic avenues but often possess poor physicochemical properties that hinder pharmaceutical development.Citation154 Therefore, it becomes necessary to establish approaches to discover drug-like multispecific antibodies.Citation155 In that regard, new methods to explore the combinatorial space for multi-specific antibody design will be important to assess not only the biological activity but also the physicochemical properties of the candidates.Citation156 In addition, antibody-drug conjugates (ADCs) are also often affected by physicochemical properties. For ADCs, it is necessary to determine early developability criteria not only for the antibody but also for the linker and the conjugated drug.Citation6 In that respect, it will be important to rationalize the selection of combinations between antibodies, linkers and payloads that result in ADCs with favorable physicochemical properties.
In summary, the outstanding challenges to deliver the next generation of antibody-based drugsCitation157 will have to be addressed by innovations at all discovery and development stages.
Abbreviations
AF4, asymmetrical flow field-flow fractionation; AHEAD: autonomous hypermutation yeast surface display; BMI – backgrounded membrane imaging; CDR - complementarity-determining region; CS-SINS - charged-stabilized self-interaction nanoparticle spectroscopy; GOI - gene of interest; DLS – dynamic light scattering; FACS - fluorescence-activated cell sorting; FDA - U.S. Food and Drug Administration; HCDR – complementarity-determining region in the heavy chain; HER2 - human epidermal growth factor receptor 2; IgG – immunoglobulin G; INN - international nonproprietary name; LCDR - complementarity-determining region in the light chain; ML – machine learning; MSF – modulated scanning fluorimetry; PACE - phage-assisted continuous evolution; pPACE – periplasmic phage-assisted continuous evolution; QD – quantum dot; scFv - single-chain variable domain; SE-PACE – soluble expression phage-assisted continuous evolution; SLS – static light scattering; SP – selection phage; TAP – therapeutic antibody profiler; TPBLA - tripartite β-lactamase enzyme assay; TPP – target product profile.
Acknowledgments
Images in the manuscript were created with BioRender.com.
Disclosure statement
No potential conflict of interest was reported by the authors.
Additional information
Funding
References
- Mullard A. FDA approves 100th monoclonal antibody product. Nat Rev Drug Discov. 2021;20(7):491–17. doi:10.1038/d41573-021-00079-7.
- Wilkinson I, Hale G. Systematic analysis of the varied designs of 819 therapeutic antibodies and Fc fusion proteins assigned international nonproprietary names. MAbs. 2022;14(1):2123299. doi:10.1080/19420862.2022.2123299.
- Brinkmann U, Kontermann RE. The making of bispecific antibodies. MAbs. 2017;9(2):182–212. doi:10.1080/19420862.2016.1268307.
- Jovčevska I, Muyldermans S. The therapeutic potential of nanobodies. BioDrugs. 2020;34(1):11–26. doi:10.1007/s40259-019-00392-z.
- Keyt BA, Baliga R, Sinclair AM, Carroll SF, Peterson MS. Structure, function, and therapeutic use of IgM antibodies. Antibodies. 2020;9(4):1–35. doi:10.3390/antib9040053.
- Beck A, Goetsch L, Dumontet C, Corvaïa N. Strategies and challenges for the next generation of antibody-drug conjugates. Nat Rev Drug Discov. 2017;16(5):315–37. doi:10.1038/nrd.2016.268.
- Kelley B. Developing therapeutic monoclonal antibodies at pandemic pace. Nat Biotechnol. 2020;38(5):540–45. doi:10.1038/s41587-020-0512-5.
- Kelley B, de Moor P, Douglas K, Renshaw T, Traviglia S. Monoclonal antibody therapies for COVID-19: lessons learned and implications for the development of future products. Curr Opin Biotechnol. 2022;78:102798. doi:10.1016/j.copbio.2022.102798.
- Jain T, Sun T, Durand S, Hall A, Houston NR, Nett JH, Sharkey B, Bobrowicz B, Caffry I, Yu Y. Biophysical properties of the clinical-stage antibody landscape. Proc Natl Acad Sci U S A. 2017;114(5):944–49. doi:10.1073/pnas.1616408114.
- Lu X, Nobrega RP, Lynaugh H, Jain T, Barlow K, Boland T, Sivasubramanian A, Vásquez M, Xu Y. Deamidation and isomerization liability analysis of 131 clinical-stage antibodies. MAbs. 2019;11(1):45–57. doi:10.1080/19420862.2018.1548233.
- Whitaker N, Xiong J, Pace SE, Kumar V, Middaugh CR, Joshi SB, Volkin DB. A formulation development approach to identify and select stable ultra–high-concentration monoclonal antibody formulations with reduced viscosities. J Pharm Sci. 2017;106(11):3230–41. doi:10.1016/j.xphs.2017.06.017.
- Rabia LA, Zhang Y, Ludwig SD, Julian MC, Tessier PM, Daggett V. Net charge of antibody complementarity-determining regions is a key predictor of specificity. Protein Engineering, Design and Selection. 2018;31(11):409–18. doi:10.1093/protein/gzz002.
- Ponsel D, Neugebauer J, Ladetzki-Baehs K, Tissot K. High affinity, developability and functional size: the holy grail of combinatorial antibody library generation. Molecules. 2011;16(5):3675–700. doi:10.3390/molecules16053675.
- Lauer TM, Agrawal NJ, Chennamsetty N, Egodage K, Helk B, Trout BL. Developability index: a rapid in silico tool for the screening of antibody aggregation propensity. J Pharm Sci. 2012;101(1):102–15. doi:10.1002/jps.22758.
- von Kreudenstein TS, Escobar-Carbrera E, Lario PI, D'Angelo I, Brault K, Kelly J, Durocher Y, Baardsnes J, Woods RJ, Xie MH. Improving biophysical properties of a bispecific antibody scaffold to aid developability: quality by molecular design. MAbs. 2013;5(5):646–54. doi:10.4161/mabs.25632.
- Starr CG, Tessier PM. Selecting and engineering monoclonal antibodies with drug-like specificity. Curr Opin Biotechnol. 2019;60:119–27. doi:10.1016/j.copbio.2019.01.008.
- Zhang Y, Wu L, Gupta P, Desai AA, Smith MD, Rabia LA, Ludwig SD, Tessier PM. Physicochemical rules for identifying monoclonal antibodies with drug-like specificity. Mol Pharm. 2020;17(7):2555–69. doi:10.1021/acs.molpharmaceut.0c00257.
- Wolf Pérez AM, Lorenzen N, Vendruscolo M, Sormanni P. Assessment of therapeutic antibody developability by combinations of in vitro and in silico methods. Therapeutic Antibodies Methods in Molecular Biology. New York, NY: Humana: 2022;57–113. doi:10.1007/978-1-0716-1450-1_4.
- Bailly M, Mieczkowski C, Juan V, Metwally E, Tomazela D, Baker J, Uchida M, Kofman E, Raoufi F, Motlagh S, et al. Predicting antibody developability profiles through early stage discovery screening. MAbs. 2020;12(1):1743053. doi:10.1080/19420862.2020.1743053.
- Makowski EK, Wu L, Gupta P, Tessier PM. Discovery-stage identification of drug-like antibodies using emerging experimental and computational methods. MAbs. 2021;13(1):1895540. doi:10.1080/19420862.2021.1895540.
- Kumar S, Roffi K, Tomar DS, Cirelli D, Luksha N, Meyer D, Mitchell J, Allen MJ, Li L , et al. Rational optimization of a monoclonal antibody for simultaneous improvements in its solution properties and biological activity. Protein Engineering, Design and Selection. 2018;31(7–8):313–25. doi:10.1093/protein/gzy020.
- Rabia LA, Desai AA, Jhajj HS, Tessier PM. Understanding and overcoming trade-offs between antibody affinity, specificity, stability and solubility. Biochem Eng J. 2018;137:365–74. doi:10.1016/j.bej.2018.06.003.
- Tiller KE, Li L, Kumar S, Julian MC, Garde S, Tessier PM. Arginine mutations in antibody complementarity-determining regions display context-dependent affinity/specificity trade-offs. Journal of Biological Chemistry. 2017;292(40):16638–52. doi:10.1074/jbc.M117.783837.
- Hoover JM, Prinslow EG, Teigler JE, Truppo MD, la Porte SL. Therapeutic antibody discovery. In: Remington: the science and practice of pharmacy. Cambridge, MA: Academic Press; 2020. p. 417–36.
- Jiskoot W, Hawe A, Menzen T, Volkin DB, Crommelin DJA. Ongoing challenges to develop high concentration monoclonal antibody-based formulations for subcutaneous administration: quo Vadis? J Pharm Sci. 2022;111(4):861–67. doi:10.1016/J.XPHS.2021.11.008.
- Silence K, Dreier T, Moshir M, Ulrichts P, Gabriels SM, Saunders M, Wajant H, Brouckaert P, Huyghe L, Van Hauwermeiren T, et al. ARGX-110, a highly potent antibody targeting CD70, eliminates tumors via both enhanced ADCC and immune checkpoint blockade. MAbs. 2014;6(2):523–32. doi:10.4161/mabs.27398.
- Kingsbury JS, Saini A, Auclair SM, Fu L, Lantz MM, Halloran KT, Calero-Rubio C, Schwenger W, Airiau CY, Zhang J, et al. A single molecular descriptor to predict solution behavior of therapeutic antibodies. Sci Adv. 2020;6(32):eabb0372. doi:10.1126/sciadv.abb0372.
- Svilenov HL, Winter G. Formulations that suppress aggregation during long-term storage of a bispecific antibody are characterized by high refoldability and colloidal stability. J Pharm Sci. 2020;109(6):2048–58. doi:10.1016/j.xphs.2020.03.011.
- Baek Y, Singh N, Arunkumar A, Borys M, Li ZJ, Zydney AL. Ultrafiltration behavior of monoclonal antibodies and Fc-fusion proteins: effects of physical properties. Biotechnol Bioeng. 2017;114(9):2057–65. doi:10.1002/bit.26326.
- Clarkson BR, Schön A, Freire E. Conformational stability and self-association equilibrium in biologics. Drug Discov Today. 2016;21(2):342–47. doi:10.1016/j.drudis.2015.11.007.
- Roberts CJ. Therapeutic protein aggregation: mechanisms, design, and control. Trends Biotechnol. 2014;32(7):372–80. doi:10.1016/j.tibtech.2014.05.005.
- Mazzer AR, Perraud X, Halley J, O’Hara J, Bracewell DG. Protein A chromatography increases monoclonal antibody aggregation rate during subsequent low pH virus inactivation hold. J Chromatogr A. 2015;1415:83–90. doi:10.1016/j.chroma.2015.08.068.
- Nejadnik MR, Randolph TW, Volkin DB, Schöneich C, Carpenter JF, Crommelin DJA, Jiskoot W. Postproduction handling and administration of protein pharmaceuticals and potential instability issues. J Pharm Sci. 2018;107(8):2013–19. doi:10.1016/j.xphs.2018.04.005.
- Manning MC, Chou DK, Murphy BM, Payne RW, Katayama DS. Stability of protein pharmaceuticals: an update. Pharm Res. 2010;27(4):544–75. doi:10.1007/s11095-009-0045-6.
- Gentiluomo L, Svilenov HL, Augustijn D, El Bialy I, Greco ML, Kulakova A, Indrakumar S, Mahapatra S, Morales MM, Pohl C. Advancing therapeutic protein discovery and development through comprehensive computational and biophysical characterization. Mol Pharm. 2020;17(2):426–40. doi:10.1021/acs.molpharmaceut.9b00852.
- Koepf E, Eisele S, Schroeder R, Brezesinski G, Friess W. Notorious but not understood: how liquid-air interfacial stress triggers protein aggregation. Int J Pharm. 2018;537(1–2):202–12. doi:10.1016/j.ijpharm.2017.12.043.
- Cunningham O, Scott M, Zhou ZS, Finlay WJJ. Polyreactivity and polyspecificity in therapeutic antibody development: risk factors for failure in preclinical and clinical development campaigns. MAbs. 2021;13(1):1999195. doi:10.1080/19420862.2021.1999195.
- Ausserwöger H, Schneider MM, Herling TW, Arosio P, Invernizzi G, Knowles TPJ, Lorenzen N. Non-specificity as the sticky problem in therapeutic antibody development. Nat Rev Chem. 2022;6(12):844–61. doi:10.1038/s41570-022-00438-x.
- Narayanan H, Dingfelder F, Butté A, Lorenzen N, Sokolov M, Arosio P. Machine learning for biologics: opportunities for protein engineering, developability, and formulation. Trends Pharmacol Sci. 2021;42(3):151–65. doi:10.1016/j.tips.2020.12.004.
- Brader ML, Estey T, Bai S, Alston RW, Lucas KK, Lantz S, Landsman P, Maloney KM. Examination of thermal unfolding and aggregation profiles of a series of developable therapeutic monoclonal antibodies. Mol Pharm. 2015;12(4):1005–17. doi:10.1021/mp400666b.
- Köhler G, Milstein C. Continuous cultures of fused cells secreting antibody of predefined specificity. Nature. 1975;256(5517):495–97. doi:10.1038/256495a0.
- Lu RM, Hwang YC, Liu IJ, Lee -C-C, Tsai H-Z, Li H-J, Wu H-C. Development of therapeutic antibodies for the treatment of diseases. J Biomed Sci. 2020;27(1):1–30. doi:10.1186/s12929-019-0592-z.
- Laustsen AH, Greiff V, Karatt-Vellatt A, Muyldermans S, Jenkins TP. Animal immunization, in vitro display technologies, and machine learning for antibody discovery. Trends Biotechnol. 2021;39(12):1263–73. doi:10.1016/j.tibtech.2021.03.003.
- Reddy ST, Ge X, Miklos AE, Hughes RA, Kang SH, Hoi KH, Chrysostomou C, Hunicke-Smith SP, Iverson BL, Tucker PW, et al. Monoclonal antibodies isolated without screening by analyzing the variable-gene repertoire of plasma cells. Nat Biotechnol. 2010;28(9):965–69. doi:10.1038/nbt.1673.
- Zost SJ, Gilchuk P, Case JB, Binshtein E, Chen RE, Nkolola JP, Schäfer A, Reidy JX, Trivette A, Nargi RS, et al. Potently neutralizing and protective human antibodies against SARS-CoV-2. Nature. 2020;584(7821):443–49. doi:10.1038/s41586-020-2548-6.
- Valldorf B, Hinz SC, Russo G, Pekar L, Mohr L, Klemm J, Doerner A, Krah S, Hust M, Zielonka S, et al. Antibody display technologies: selecting the cream of the crop. Biol Chem. 2022;403(5):455–77. doi:10.1515/hsz-2020-0377.
- Kaleli NE, Karadag M, Kalyoncu S. Phage display derived therapeutic antibodies have enriched aliphatic content: insights for developability issues. Proteins: Structure, Function and Bioinformatics. 2019;87(7):607–18. doi:10.1002/prot.25685.
- Reis Teixeira A A, Erasmus MF, D’Angelo S, Naranjo L, Ferrara F, Leal-Lopes C, Durrant O, Galmiche C, Morelli A, Scott-Tucker A, et al. Drug-like antibodies with high affinity, diversity and developability directly from next-generation antibody libraries. MAbs. 2021;13(1):1980942. doi:10.1080/19420862.2021.1980942.
- Kelly RL, Le D, Zhao J, Wittrup KD. Reduction of nonspecificity motifs in synthetic antibody libraries. J Mol Biol. 2018;430(1):119–30. doi:10.1016/j.jmb.2017.11.008.
- Teixeira AAR, D’Angelo S, Erasmus MF, Leal-Lopes C, Ferrara F, Spector LP, Naranjo L, Molina E, Max T, DeAguero A, et al. Simultaneous affinity maturation and developability enhancement using natural liability-free CDRs. MAbs. 2022;14(1):2115200. doi:10.1080/19420862.2022.2115200.
- Shin JE, Riesselman AJ, Kollasch AW, McMahon C, Simon E, Sander C, Manglik A, Kruse AC, Marks DS. Protein design and variant prediction using autoregressive generative models. Nat Commun. 2021;12(1):1–11. doi:10.1038/s41467-021-22732-w.
- Jung S, Honegger A, Plückthun A. Selection for improved protein stability by phage display. J Mol Biol. 1999;294(1):163–80. doi:10.1006/jmbi.1999.3196.
- Shusta EV, Holler PD, Kieke MC, Kranz DM, Wittrup KD. Directed evolution of a stable scaffold for T-cell receptor engineering. Nat Biotechnol. 2000;18(7):754–59. doi:10.1038/77325.
- Jespers L, Schon O, Famm K, Winter G. Aggregation-resistant domain antibodies selected on phage by heat denaturation. Nat Biotechnol. 2004;22(9):1161–65. doi:10.1038/nbt1000.
- Dudgeon K, Rouet R, Kokmeijer I, Schofield P, Stolp J, Langley D, Stock D, Christ D. General strategy for the generation of human antibody variable domains with increased aggregation resistance. Proc Natl Acad Sci U S A. 2012;109(27):10879–84. doi:10.1073/pnas.1202866109.
- Famm K, Hansen L, Christ D, Winter G. Thermodynamically stable aggregation-resistant antibody domains through directed evolution. J Mol Biol. 2008;376(4):926–31. doi:10.1016/j.jmb.2007.10.075.
- Kelly RL, Zhao J, Le D, Wittrup KD. Nonspecificity in a nonimmune human scFv repertoire. MAbs. 2017;9(7):1029–35. doi:10.1080/19420862.2017.1356528.
- Xu Y, Roach W, Sun T, Jain T, Prinz B, Yu TY, Torrey J, Thomas J, Bobrowicz P, Vásquez M. Addressing polyspecificity of antibodies selected from an in vitro yeast presentation system: A FACS-based, high-throughput selection and analytical tool. Protein Engineering, Design and Selection. 2013;26(10):663–70. doi:10.1093/protein/gzt047.
- Mason DM, Weber CR, Parola C, Meng SM, Greiff V, Kelton WJ, Reddy ST. High-throughput antibody engineering in mammalian cells by CRISPR/Cas9-mediated homology-directed mutagenesis. Nucleic Acids Res. 2018;46(14):7436–49. doi:10.1093/nar/gky550.
- Parthiban K, Perera RL, Sattar M, Huang Y, Mayle S, Masters E, Griffiths D, Surade S, Leah R, Dyson MR, et al. A comprehensive search of functional sequence space using large mammalian display libraries created by gene editing. MAbs. 2019;11(5):884–98. doi:10.1080/19420862.2019.1618673.
- Dyson MR, Masters E, Pazeraitis D, Perera RL, Syrjanen JL, Surade S, Thorsteinson N, Parthiban K, Jones PC, Sattar M, et al. Beyond affinity: selection of antibody variants with optimal biophysical properties and reduced immunogenicity from mammalian display libraries. MAbs. 2020;12(1):1829335. doi:10.1080/19420862.2020.1829335.
- Tiller KE, Chowdhury R, Li T, Ludwig SD, Sen S, Maranas CD, Tessier PM. Facile affinity maturation of antibody variable domains using natural diversity mutagenesis. Front Immunol. 2017;8:986. doi:10.3389/fimmu.2017.00986.
- Ki JJ, Min JS, Iverson BL, Georgiou G. APEx 2-hybrid, a quantitative protein-protein interaction assay for antibody discovery and engineering. Proc Natl Acad Sci U S A. 2007;104(20):8247–52. doi:10.1073/pnas.0702650104.
- Waraho-Zhmayev D, Meksiriporn B, Portnoff AD, DeLisa MP, Bradbury A. Optimizing recombinant antibodies for intracellular function using hitchhiker-mediated survival selection. Protein Engineering, Design and Selection. 2014;27(10):351–58. doi:10.1093/protein/gzu038.
- Lombana TN, Dillon M, Bevers J, Spiess C. Optimizing antibody expression by using the naturally occurring framework diversity in a live bacterial antibody display system. Sci Rep. 2015;5(1):1–14. doi:10.1038/srep17488.
- Moghaddam-Taaheri P, Ikonomova SP, Gong Z, Wisniewski JQ, Karlsson AJ. Bacterial inner-membrane display for screening a library of antibody fragments. Journal of Visualized Experiments. 2016;116:e54583. doi:10.3791/54583.
- Esvelt KM, Carlson JC, Liu DR. A system for the continuous directed evolution of biomolecules. Nature. 2011;472(7344):499–503. doi:10.1038/nature09929.
- Ebo JS, Saunders JC, Devine PWA, Gordon AM, Warwick AS, Schiffrin B, Chin SE, England E, Button JD, Lloyd C. An in vivo platform to select and evolve aggregation-resistant proteins. Nat Commun. 2020;11(1):1–12. doi:10.1038/s41467-020-15667-1.
- Wang T, Badran AH, Huang TP, Liu DR. Continuous directed evolution of proteins with improved soluble expression. Nat Chem Biol. 2018;14(10):972–80. doi:10.1038/s41589-018-0121-5.
- Morrison MS, Wang T, Raguram A, Hemez C, Liu DR. Disulfide-compatible phage-assisted continuous evolution in the periplasmic space. Nat Commun. 2021;12(1):1–14. doi:10.1038/s41467-021-26279-8.
- Saunders JC, Young LM, Mahood RA, Jackson MP, Revill CH, Foster RJ, Smith DA, Ashcroft AE, Brockwell DJ, Radford SE, et al. An in vivo platform for identifying inhibitors of protein aggregation. Nat Chem Biol. 2016;12(2):94–101. doi:10.1038/nchembio.1988.
- Wellner A, McMahon C, Gilman MSA, Clements JR, Clark S, Nguyen KM, Ho MH, Hu VJ, Shin J-E, Feldman J, et al. Rapid generation of potent antibodies by autonomous hypermutation in yeast. Nat Chem Biol. 2021;17(10):1057–64. doi:10.1038/s41589-021-00832-4.
- Makowski EK, Kinnunen PC, Huang J, Wu L, Smith MD, Wang T, Desai AA, Streu CN, Zhang Y, Zupancic JM, et al. Co-optimization of therapeutic antibody affinity and specificity using machine learning models that generalize to novel mutational space. Nat Commun. 2022;13(1):1–14. doi:10.1038/s41467-022-31457-3.
- Saksena SD, Liu G, Banholzer C, Horny G, Ewert S, Gifford DK. Computational counterselection identifies nonspecific therapeutic biologic candidates. Cell Reports Methods. 2022;2(7):100254. doi:10.1016/j.crmeth.2022.100254.
- Liu G, Zeng H, Mueller J, Carter B, Wang Z, Schilz J, Horny G, Birnbaum ME, Ewert S, Gifford DK, et al. Antibody complementarity determining region design using high-capacity machine learning. Bioinformatics. 2020;36(7):2126–33. doi:10.1093/bioinformatics/btz895.
- Makowski EK, Chen H, Lambert M, Bennett EM, Eschmann N, Zhang Y, Zupancic JM, Desai AA, Smith MD, Lou W. Reduction of therapeutic antibody self-association using yeast-display selections and machine learning. MAbs. 2022;14(1). doi:10.1080/19420862.2022.2146629
- Julian MC, Li L, Garde S, Wilen R, Tessier PM. Efficient affinity maturation of antibody variable domains requires co-selection of compensatory mutations to maintain thermodynamic stability. Sci Rep. 2017;7(1):1–13. doi:10.1038/srep45259.
- Shehata L, Maurer DP, Wec AZ, Lilov A, Champney E, Sun T, Archambault K, Burnina I, Lynaugh H, Zhi X, et al. Affinity maturation enhances antibody specificity but compromises conformational stability. Cell Rep. 2019;28(13):3300–08. doi:10.1016/j.celrep.2019.08.056.
- Traxlmayr MW, Faissner M, Stadlmayr G, Hasenhindl C, Antes B, Rüker F, Obinger C. Directed evolution of stabilized IgG1-Fc scaffolds by application of strong heat shock to libraries displayed on yeast. Biochim Biophys Acta Proteins Proteom. 2012;1824(4):542–49. doi:10.1016/j.bbapap.2012.01.006.
- Traxlmayr MW, Obinger C. Directed evolution of proteins for increased stability and expression using yeast display. Arch Biochem Biophys. 2012;526(2):174–80. doi:10.1016/j.abb.2012.04.022.
- Xu L, Kohli N, Rennard R, Jiao Y, Razlog M, Zhang K, Baum J, Johnson B, Tang J, Schoeberl B, et al. Rapid optimization and prototyping for therapeutic antibody-like molecules. MAbs. 2013;5(2):237–54. doi:10.4161/mabs.23363.
- Luo R, Qu B, An L, Zhao Y, Cao Y, Ren P, Hang H. Simultaneous maturation of single chain antibody stability and affinity by CHO cell display. Bioengineering. 2022;9(8):360. doi:10.3390/bioengineering9080360.
- Mason DM, Friedensohn S, Weber CR, Jordi C, Wagner B, Meng SM, Ehling RA, Bonati L, Dahinden J, Gainza P, et al. Optimization of therapeutic antibodies by predicting antigen specificity from antibody sequence via deep learning. Nat Biomed Eng. 2021;5(6):600–12. doi:10.1038/s41551-021-00699-9.
- Kelly RL, Sun T, Jain T, Caffry I, Yu Y, Cao Y, Lynaugh H, Brown M, Vásquez M, Wittrup KD. High throughput cross-interaction measures for human IgG1 antibodies correlate with clearance rates in mice. MAbs. 2015;7(4):770–77. doi:10.1080/19420862.2015.1043503/SUPPL_FILE/KMAB_A_1043503_SM9491.ZIP.
- Watson CD, Ford HC. High-affinity binding of riboflavin and FAD by immunoglobulins from normal human serum. Biochem Int. 1988; 16(6):1067–74. [Accessed October 10, 2022]. https://europepmc.org/article/med/3178858.
- Bowen RAR, Drake SK, Vanjani R, Huey ED, Grafman J, Horne MK. Markedly increased vitamin B12 concentrations attributable to IgG-IgM-vitamin B12 immune complexes. Clin Chem. 2006;52(11):2107–14. doi:10.1373/clinchem.2006.073882.
- Lecerf M, Kanyavuz A, Rossini S, Dimitrov JD. Interaction of clinical-stage antibodies with heme predicts their physiochemical and binding qualities. Commun Biol. 2021;4(1):1–13. doi:10.1038/s42003-021-01931-7.
- Dimitrov JD, Ivanovska ND, Lacroix-Desmazes S, Doltchinkova VR, Kaveri SV, Vassilev TL. Ferrous ions and reactive oxygen species increase antigen-binding and anti-inflammatory activities of immunoglobulin G. Journal of Biological Chemistry. 2006;281(1):439–46. doi:10.1074/jbc.M509190200.
- McIntyre JA. The appearance and disappearance of antiphospholipid autoantibodies subsequent to oxidation-reduction reactions. Thromb Res. 2004;114(5–6):579–87. doi:10.1016/j.thromres.2004.08.008.
- McIntyre JA, Wagenknecht DR, Faulk WP. Autoantibodies unmasked by redox reactions. J Autoimmun. 2005;24(4):311–17. doi:10.1016/j.jaut.2005.03.005.
- Lecerf M, Lacombe R, Kanyavuz A, Dimitrov JD. Functional changes of therapeutic antibodies upon exposure to pro-oxidative agents. Antibodies. 2022;11(1):11. doi:10.3390/antib11010011.
- Gupta S, Jiskoot W, Schöneich C, Rathore AS. Oxidation and deamidation of monoclonal antibody products: potential impact on stability, biological activity, and efficacy. J Pharm Sci. 2022;111(4):903–18. doi:10.1016/j.xphs.2021.11.024.
- Starr CG, Makowski EK, Wu L, Berg B, Kingsbury JS, Gokarn YR, Tessier PM. Ultradilute measurements of self-association for the identification of antibodies with favorable high-concentration solution properties. Mol Pharm. 2021;18(7):2744–53. doi:10.1021/acs.molpharmaceut.1c00280.
- Wu J, Schultz JS, Weldon CL, Sule SV, Chai Q, Geng SB, Dickinson CD, Tessier PM. Discovery of highly soluble antibodies prior to purification using affinity-capture self-interaction nanoparticle spectroscopy. Protein Engineering, Design and Selection. 2015;28(10):403–14. doi:10.1093/protein/gzv045.
- Sule SV, Dickinson CD, Lu J, Chow CK, Tessier PM. Rapid analysis of antibody self-association in complex mixtures using immunogold conjugates. Mol Pharm. 2013;10(4):1322–31. doi:10.1021/mp300524x.
- Liu Y, Caffry I, Wu J, Geng SB, Jain T, Sun T, Reid F, Cao Y, Estep P, Yu Y, et al. High-throughput screening for developability during early-stage antibody discovery using self-interaction nanoparticle spectroscopy. MAbs. 2014;6(2):483–92. doi:10.4161/mabs.27431.
- Fraunhofer W, Winter G. The use of asymmetrical flow field-flow fractionation in pharmaceutics and biopharmaceutics. European Journal of Pharmaceutics and Biopharmaceutics. 2004;58(2):369–83. doi:10.1016/j.ejpb.2004.03.034.
- Marioli M, Kok WT. Recovery, overloading, and protein interactions in asymmetrical flow field-flow fractionation. Anal Bioanal Chem. 2019;411(11):2327–38. doi:10.1007/s00216-019-01673-w.
- Wahlund PO, Lorenzen N, Rischel C. Screening for protein–protein interactions with asymmetrical flow field-flow fractionation. J Pharm Sci. 2021;110(6):2336–39. doi:10.1016/J.XPHS.2021.02.026.
- Das TK, Chou DK, Jiskoot W, Arosio P. Nucleation in protein aggregation in biotherapeutic development: a look into the heart of the event. J Pharm Sci. 2022;111(4):951–59. doi:10.1016/j.xphs.2022.01.017.
- Wang W, Roberts CJ. Non-Arrhenius protein aggregation. AAPS Journal. 2013;15(3):840–51. doi:10.1208/s12248-013-9485-3.
- Arosio P, Rima S, Morbidelli M. Aggregation mechanism of an IgG2 and two IgG1 monoclonal antibodies at low pH: from oligomers to larger aggregates. Pharm Res. 2013;30(3):641–54. doi:10.1007/s11095-012-0885-3.
- Wälchli R, Vermeire PJ, Massant J, Arosio P. Accelerated aggregation studies of monoclonal antibodies: considerations for storage stability. J Pharm Sci. 2020;109(1):595–602. doi:10.1016/j.xphs.2019.10.048.
- Jgs H, Middelberg APJ. Estimating the potential refolding yield of recombinant proteins expressed as inclusion bodies. Biotechnol Bioeng. 2004;87(5):584–92. doi:10.1002/bit.20148.
- Kunz P, Zinner K, Mücke N, Bartoschik T, Muyldermans S, Hoheisel JD. The structural basis of nanobody unfolding reversibility and thermoresistance. Sci Rep. 2018;8(1):1–10. doi:10.1038/s41598-018-26338-z.
- Schön A, Freire E. Reversibility and irreversibility in the temperature denaturation of monoclonal antibodies. Anal Biochem. 2021;626:114240. doi:10.1016/j.ab.2021.114240.
- Svilenov HL, Menzen T, Richter K, Winter G. Modulated scanning fluorimetry can quickly assess thermal protein unfolding reversibility in microvolume samples. Mol Pharm. 2020;17(7):2638–47. doi:10.1021/acs.molpharmaceut.0c00330.
- Svilenov H, Winter G. The ReFOLD assay for protein formulation studies and prediction of protein aggregation during long-term storage. European Journal of Pharmaceutics and Biopharmaceutics. 2019;137:131–39. doi:10.1016/j.ejpb.2019.02.018.
- Svilenov H, Gentiluomo L, Friess W, Roessner D, Winter G. A new approach to study the physical stability of monoclonal antibody formulations—dilution from a denaturant. J Pharm Sci. 2018;107(12):3007–13. doi:10.1016/j.xphs.2018.08.004.
- Berner C, Menzen T, Winter G, Svilenov HL. Combining unfolding reversibility studies and molecular dynamics simulations to select aggregation-resistant antibodies. Mol Pharm. 2021;18(6):2242–53. doi:10.1021/acs.molpharmaceut.1c00017.
- Kopp MRG, Grigolato F, Zürcher D, Das TK, Chou D, Wuchner K, Arosio P. Surface-induced protein aggregation and particle formation in biologics: current understanding of mechanisms, detection and mitigation strategies.J Pharm Sci. - October 9 2022; Accepted manuscript, In press 10.1016/j.xphs.2022.10.009
- Kopp MRG, Capasso Palmiero U, Arosio P. A nanoparticle-based assay to evaluate surface-induced antibody instability. Mol Pharm. 2020;17(3):909–18. doi:10.1021/acs.molpharmaceut.9b01168.
- Kopp MRG, Wolf Pérez AM, Zucca MV, Capasso Palmiero U, Friedrichsen B, Lorenzen N, Arosio P. An accelerated surface-mediated stress assay of antibody instability for developability studies. MAbs. 2020;12(1):1815995. doi:10.1080/19420862.2020.1815995.
- Johann F, Wöll S, Winzer M, Snell J, Valldorf B, Gieseler H. Miniaturized forced degradation of therapeutic proteins and ADCs by agitation-induced aggregation using orbital shaking of microplates. J Pharm Sci. 2022;111(5):1401–13. doi:10.1016/j.xphs.2021.09.027.
- Mathaes R, Narhi L, Hawe A, Matter A, Bechtold-Peters K, Kenrick S, Kar S, Laskina O, Carpenter J, Cavicchi R. Phase-appropriate application of analytical methods to monitor subvisible particles across the biotherapeutic drug product life cycle. AAPS Journal. 2020;22(1):1–7. doi:10.1208/s12248-019-0384-0.
- Roesch A, Zölls S, Stadler D, Helbig C, Wuchner K, Kersten G, Hawe A, Jiskoot W, Menzen T. Particles in biopharmaceutical formulations, Part 2: an update on analytical techniques and applications for therapeutic proteins, viruses, vaccines and cells. J Pharm Sci. 2022;111(4):933–50. doi:10.1016/j.xphs.2021.12.011.
- Kunz P, Stuckenberger E, Hausmann K, Gentiluomo L, Neustrup M, Michalakis S, Rieser R, Romeijn S, Wichmann C, Windisch R, et al. Understanding opalescence measurements of biologics – a comparison study of methods, standards, and molecules. Int J Pharm. 2022;628:122321. doi:10.1016/j.ijpharm.2022.122321.
- Helbig C, Ammann G, Menzen T, Friess W, Wuchner K, Hawe A. Backgrounded membrane imaging (BMI) for high-throughput characterization of subvisible particles during biopharmaceutical drug product development. J Pharm Sci. 2020;109(1):264–76. doi:10.1016/j.xphs.2019.03.024.
- Murphy MI, Bruque M, Hanford A, Trayton I, Handali M, Leissa JA, Hasige S, Day K, Patel SM. Qualitative High-throughput analysis of subvisible particles in biological formulations using backgrounded membrane imaging. J Pharm Sci. 2022;111(6):1605–13. doi:10.1016/j.xphs.2022.03.010.
- Gühlke M, Hecht J, Böhrer A, Hawe A, Nikels F, Garidel P, Menzen T. Taking subvisible particle quantitation to the limit: uncertainties and statistical challenges with ophthalmic products for intravitreal injection. J Pharm Sci. 2020;109(1):505–14. doi:10.1016/j.xphs.2019.10.061.
- Calderon CP, Levačić A K, Helbig C, Wuchner K, Menzen T. Combining machine learning and backgrounded membrane imaging: a case study in comparing and classifying different types of biopharmaceutically relevant particles. J Pharm Sci. 2022;111(9):2422–34. doi:10.1016/j.xphs.2022.05.022.
- Sormanni P, Aprile FA, Vendruscolo M. Third generation antibody discovery methods: in silico rational design. Chem Soc Rev. 2018;47(24):9137–57. doi:10.1039/c8cs00523k.
- Fischman S, Ofran Y. Computational design of antibodies. Curr Opin Struct Biol. 2018;51:156–62. doi:10.1016/j.sbi.2018.04.007.
- Baran D, Pszolla MG, Lapidoth GD, Norn C, Dym O, Unger T, Albeck S, Tyka MD, Fleishman SJ. Principles for computational design of binding antibodies. Proc Natl Acad Sci U S A. 2017;114(41):10900–05. doi:10.1073/pnas.1707171114.
- Nimrod G, Fischman S, Austin M, Herman A, Keyes F, Leiderman O, Hargreaves D, Strajbl M, Breed J, Klompus K. Computational design of epitope-specific functional antibodies. Cell Rep. 2018;25(8):2121–31. doi:10.1016/j.celrep.2018.10.081.
- Entzminger KC, Hyun JM, Pantazes RJ, Patterson-Orazem AC, Qerqez AN, Frye ZP, Hughes RA, Ellington AD, Lieberman RL, Maranas CD. De novo design of antibody complementarity determining regions binding a FLAG tetra-peptide. Sci Rep. 2017;7(1):1–11. doi:10.1038/s41598-017-10737-9.
- Poosarla VG, Li T, Goh BC, Schulten K, Wood TK, Maranas CD. Computational de novo design of antibodies binding to a peptide with high affinity. Biotechnol Bioeng. 2017;114(6):1331–42. doi:10.1002/bit.26244.
- Pras A The Molecular Role of SERF in Amyloid Formation: A Fine Line between Toxicity and Function. PhD Thesis. 2021. doi:10.33612/diss.177554757
- Aprile FA, Sormanni P, Podpolny M, Chhangur S, Needham L-M, Ruggeri FS, Perni M, Limbocker R, Heller GT, Sneideris T, et al. Rational design of a conformation-specific antibody for the quantification of Aβ oligomers. Proc Natl Acad Sci U S A. 2020;117(24):13509–18. doi:10.1073/pnas.1919464117.
- Lin J, Figazzolo C, Metrick MA, Sormanni P, Vendruscolo M. Computational maturation of a single-domain antibody against Aβ42 aggregation. Chem Sci. 2021;12(41):13940–48. doi:10.1039/d1sc03898b.
- Kulenkampff K, Emin D, Staats R, Zhang YP, Sakhnini L, Kouli A, Rimon O, Lobanova E, Williams-Gray CH, Aprile FA, et al. An antibody scanning method for the detection of α-synuclein oligomers in the serum of Parkinson’s disease patients. Chem Sci. 2022;13(46):13815–28. doi:10.1039/d2sc00066k.
- Sormanni P, Aprile FA, Vendruscolo M. Rational design of antibodies targeting specific epitopes within intrinsically disordered proteins. Proc Natl Acad Sci U S A. 2015;112(32):9902–07. doi:10.1073/pnas.1422401112.
- Aguilar Rangel M, Bedwell A, Costanzi E, Taylor RJ, Russo R, Bernardes GJL, Ricagno S, Frydman J, Vendruscolo M, Sormanni P, et al. Fragment-based computational design of antibodies targeting structured epitopes. Sci Adv. 2022;8(45):9540. doi:10.1126/SCIADV.ABP9540/SUPPL_FILE/SCIADV.ABP9540_TABLE_S3.ZIP.
- Khetan R, Curtis R, Deane CM, Hadsund JT, Kar U, Krawczyk K, Kuroda D, Robinson SA, Sormanni P, Tsumoto K, et al. Current advances in biopharmaceutical informatics: guidelines, impact and challenges in the computational developability assessment of antibody therapeutics. MAbs. 2022;14(1):2020082. doi:10.1080/19420862.2021.2020082.
- Raybould MIJ, Marks C, Krawczyk K, Taddese B, Nowak J, Lewis AP, Bujotzek A, Shi J, Deane CM. Five computational developability guidelines for therapeutic antibody profiling. Proc Natl Acad Sci U S A. 2019;116(10):4025–30. doi:10.1073/pnas.1810576116.
- Thorsteinson N, Gunn JR, Kelly K, Long W, Labute P. Structure-based charge calculations for predicting isoelectric point, viscosity, clearance, and profiling antibody therapeutics. MAbs. 2021;13(1):1981805. doi:10.1080/19420862.2021.1981805.
- Ahmed L, Gupta P, Martin KP, Scheer JM, Nixon AE, Kumar S. Intrinsic physicochemical profile of marketed antibody-based biotherapeutics. Proc Natl Acad Sci U S A. 2021;118(37):e2020577118. doi:10.1073/pnas.2020577118.
- Broom A, Jacobi Z, Trainor K, Meiering EM. Computational tools help improve protein stability but with a solubility tradeoff. Journal of Biological Chemistry. 2017;292(35):14349–61. doi:10.1074/jbc.M117.784165.
- Gil-Garcia M, Banó-Polo M, Varejao N, Jamroz M, Kuriata A, Díaz-Caballero M, Lascorz J, Morel B, Navarro S, Reverter D, et al. Combining structural aggregation propensity and stability predictions to redesign protein solubility. Mol Pharm. 2018;15(9):3846–59. doi:10.1021/acs.molpharmaceut.8b00341.
- van der Kant R, Karow-Zwick AR, van Durme J, Blech M, Gallardo R, Seeliger D, Aßfalg K, Baatsen P, Compernolle G, Gils A, et al. Prediction and reduction of the aggregation of monoclonal antibodies. J Mol Biol. 2017;429(8):1244–61. doi:10.1016/j.jmb.2017.03.014.
- Rosace A, Bennett A, Oeller M, Mortensen MM, Sakhnini LI, Sormanni P. Automated optimisation of solubility and conformational stability of antibodies and proteins. bioRxiv. Published online October 18, 2022. doi:10.1101/2022.05.20.492798.
- Sormanni P, Aprile FA, Vendruscolo M. The CamSol method of rational design of protein mutants with enhanced solubility. J Mol Biol. 2015;427(2):478–90. doi:10.1016/j.jmb.2014.09.026.
- Schymkowitz J, Borg J, Stricher F, Nys R, Rousseau F, Serrano L. The FoldX web server: an online force field. Nucleic Acids Res. 2005;33(SUPPL. 2):W382–W388. doi:10.1093/nar/gki387.
- Abanades B, Georges G, Bujotzek A, Deane CM, Xu J. ABlooper: fast accurate antibody CDR loop structure prediction with accuracy estimation. Bioinformatics. 2022;38(7):1877–80. doi:10.1093/bioinformatics/btac016.
- Ruffolo JA, Sulam J, Gray JJ. Antibody structure prediction using interpretable deep learning. Patterns. 2022;3(2):100406. doi:10.1016/j.patter.2021.100406.
- Cohen T, Halfon M, Schneidman-Duhovny D. NanoNet: rapid and accurate end-to-end nanobody modeling by deep learning. Front Immunol. 2022;13:4319. doi:10.3389/fimmu.2022.958584.
- Schneider C, Raybould MIJ, Deane CM. SAbDab in the age of biotherapeutics: updates including SAbDab-nano, the nanobody structure tracker. Nucleic Acids Res. 2022;50(D1):D1368–D1372. doi:10.1093/nar/gkab1050.
- Liberis E, Velickovic P, Sormanni P, Vendruscolo M, Lio P, Hancock J. Parapred: antibody paratope prediction using convolutional and recurrent neural networks. Bioinformatics. 2018;34(17):2944–50. doi:10.1093/bioinformatics/bty305.
- Deac A, Veličković P, Sormanni P. Attentive Cross-Modal Paratope Prediction. Journal of Computational Biology. 2019;26(6):536–45. doi:10.1089/cmb.2018.0175.
- Leem J, Mitchell LS, Farmery JHR, Barton J, Galson JD. Deciphering the language of antibodies using self-supervised learning. Patterns. 2022;3(7):100513. doi:10.1016/j.patter.2022.100513.
- Kopp MRG, Arosio P. Microfluidic Approaches for the Characterization of Therapeutic Proteins. J Pharm Sci. 2018;107(5):1228–36. doi:10.1016/j.xphs.2018.01.001.
- Erkamp NA, Oeller M, Sneideris T, Ausserwӧger H, Levin A, Welsh T, Qi R, Qian D, Zhu H, Sormanni P. Multi-dimensional protein solubility optimization with an ultra-high-throughput microfluidic platform. bioRxiv. Published online October 22 2022. 10.1101/2022.10.21.513267. 2022.10.21.513267.
- Narayanan H, Dingfelder F, Condado Morales I, Patel B, Heding KE, Bjelke JR, Egebjerg T, Butté A, Sokolov M, Lorenzen N, et al. Design of biopharmaceutical formulations accelerated by machine learning. Mol Pharm. 2021;18(10):3843–53. doi:10.1021/acs.molpharmaceut.1c00469.
- Brinkmann U, Kontermann RE. Bispecific antibodies. Science. 2021;372(6545):916–17. doi:10.1126/science.abg1209.
- Sawant MS, Streu CN, Wu L, Tessier PM. Toward drug-like multispecific antibodies by design. Int J Mol Sci. 2020;21(20):1–41. doi:10.3390/ijms21207496.
- Dengl S, Mayer K, Bormann F, Duerr H, Hoffmann E, Nussbaum B, Tischler M, Wagner M, Kuglstatter A, Leibrock L, et al. Format chain exchange (FORCE) for high-throughput generation of bispecific antibodies in combinatorial binder-format matrices. Nat Commun. 2020;11(1):1–11. doi:10.1038/s41467-020-18477-7.
- Carter PJ, Rajpal A. Designing antibodies as therapeutics. Cell. 2022;185(15):2789–805. doi:10.1016/j.cell.2022.05.029.