ABSTRACT
This study aimed to analyze metabolite abundances and proteome differences between buffalo milk from Binglangjiang (BBM) and Dehong (DBM). Untargeted UPLC-MS/MS, label-free proteomics approach, and bioinformatics analyses including GO, KEGG, and PPI were performed. The results revealed that catechol, L-proline, γ-aminobutyric acid, hippuric acid, creatinine, and betaine were detected in BBM and DBM as the major metabolites. One hundred thirteen proteins, mainly within the molecular weight range of 20–80 kDa, were identified, and the differential protein numbers of BBM were higher than those of DBM. These proteins were primarily involved in cholesterol metabolism, Staphylococcus aureus and Salmonella infections, the peroxisome proliferator-activated receptor signaling pathway, and coagulation cascades. Relative abundances of α-s1-casein, α-s2-casein, and β-casein were plentiful in BBM, while κ-casein, albumin, and α-lactalbumin were in DBM. PPI network analysis indicated that the identified proteins were highly interconnected. These findings promote a better understanding of quality biomarkers in dairy product improvements.
1. Introduction
Milk and its by-products are one of the most important protein sources produced by the mammary glands of mammals, providing complete dietary nutrition, bioavailable amino acid sources, and essential micronutrients, as well as readily digestible food for the human diet (Arslan et al., Citation2021; Davoodi et al., Citation2016; Leischner et al., Citation2021; Smith et al., Citation2022; Vargas-Ramella et al., Citation2021). At present, the global production of unprocessed milk is dominated by cattle (81%), followed by buffalo (15%), and a combination of goats, sheep, and camels (4%), totaling 902 million tons of solid milk and predicted to grow at 2.2% per annum by 2030 (OECD/FAO, Citation2021; van Heerden, Citation2021). Nonetheless, animal-based milk sources produced by minor dairy animals like buffalo, goat, sheep, camel, yak, horse, and donkey are also nutritious and economically impactful in some countries (Alichanidis et al., Citation2016; Faccia et al., Citation2020). In Asia continent, buffalo milk accounts for 35.30% (124,958,493 tons) of the total unprocessed milk production, where the majority is supplied by the five most prominent producers, including India, Pakistan, China, Egypt, and Nepal (Minervino et al., Citation2020). Across Yunnan province in the Southwestern region of China, recognized as the second biggest population center of buffaloes after Guangxi province, Binglangjiang (river type) and Dehong (swamp type) buffaloes are the most famous native dual-purpose breeds to produce meat and milk (Fan et al., Citation2019; Qiu et al., Citation2021; Yang et al., Citation2007; Zhou et al., Citation2020).
Previous studies reported that buffalo milk has been widely investigated for a long time in association with the particular use of the food industry because of its technological suitability, the differences in functional properties, and higher energy per unit volume (Arora et al., Citation2022; Jiang et al., Citation2022; Mejares et al., Citation2022; Tsuji et al., Citation2022). Buffalo milk contains high nutritive values, rich flavor profiles, and is more appropriate for processing to make various by-products, such as condensed milk, cheese, cream, yogurt, butter, and probiotics, as compared to cow milk (Jiang et al., Citation2022; Stobiecka et al., Citation2022; Vargas-Ramella et al., Citation2021). Buffalo milk poses a noticeable difference in physicochemical features relative to other domesticated ruminant species, such as cows, sheep, and goats (Bittante et al., Citation2022; Verduci et al., Citation2019; Yuan et al., Citation2022). It has specific nutrition properties with a higher level of proteins (e.g. α-s1-casein and κ-casein), fatty acids, lactose, vitamin A, calcium, a lower percentage of water, dry matter, vitamin E, riboflavin, larger casein micelles (ranging between 110 and 170 nm, with 2.68–3.72 mL g−1 of voluminosity and 2.60–2.90 g water g−1 of solvation), an absence of carotene, and reduced cholesterol in comparison with other ruminant milk (Bertoni et al., Citation2021; D’Ambrosio et al., Citation2008; El-Salam & El-Shibiny, Citation2011; Figliola et al., Citation2021; Garau et al., Citation2021; Guo & Hendricks, Citation2010). The gross chemical composition and particular biological characteristics of buffalo milk may be strongly affected by the differences in metabolic mechanisms due to geographical location, rearing system, feeding regime, and breed (Garau et al., Citation2021; Han et al., Citation2007; Sun et al., Citation2014; Yuan et al., Citation2022). Therefore, owing to the high-nutritive values and metabolites in buffalo milk being beneficial as potential ingredients in various food industry applications, there is a desire to study these biological differences among breeds in the light of new available emerging technologies.
Recent metabolomics and proteomics approaches are recognized as robust methods for the comprehensive investigation of milk and its by-products. Nuclear magnetic resonance (NMR) spectroscopy, gas chromatography-mass spectrometry (GC-MS), and liquid chromatography-mass spectrometry (LC-MS) are considered the most extensively used analytical methods to determine the metabolomes and proteomes in milk. Among those methods, LC-MS has quickly become the preferred analytical method due to its high reproducibility and sensitivity for analyzing an extensive range of chemical compounds in a single detection (Jiang et al., Citation2022; Lv et al., Citation2020; Shi et al., Citation2021; Trobbiani et al., Citation2017). In this study, we used an untargeted ultraperformance liquid chromatography – tandem mass spectrometry (UPLC-MS/MS) and quantitative label-free high-performance liquid chromatography – tandem mass spectrometry (HPLC-MS/MS)-based proteomics approach and performed gene ontology (GO), Kyoto Encyclopedia of Genes and Genomes (KEGG), and protein-protein interaction (PPI) analyses to identify the differentially expressed proteins in BBM and DBM in order to expand the molecular understanding of their metabolomics and proteomics profiles. To the best of our knowledge, this is the first study using label-free mass spectrometry with high mass accuracy in MS/MS scans for buffalo milk from Yunnan native purebreds.
2. Materials and methods
2.1. Milk sample collection
Sixty-five buffalo raw-milk samples were obtained from two native Yunnan breeds located on two different dairy farms in Dehong prefecture (Dehong buffalo breed, n = 35) and Tengchong county (Binglangjiang buffalo breed, n = 30), Yunnan province, China. The selection criteria for the buffalos were based on the clinical records, where the body temperature range, the absence of systemic signs, and mastitis status were normal. Fresh milk samples were transferred to 50 mL sterile centrifuge tubes, kept on ice, and immediately transported to the laboratory. All milk samples were separated into three parts and stored at − 80°C for further analysis. The use of all animals in this research was approved according to the Institutional Animal Care and Use Committee of Yunnan Agricultural University guidelines. On the first part of milk samples, routine tests were performed, including chemical composition analysis (fat, protein, lactose, and total solids) using a MilkoScan FT2 analyzer (FOSS Electric A/S Analytics, Hillerd, Denmark); protein quantification using a bicinchoninic acid (BCA) assay kit (Beijing Solarbio Life Science Co., Ltd., China); and A2 β-casein protein isoform concentration measurement using a sandwich enzyme-linked immunosorbent assay (ELISA) kit (Biosensis Pty., Ltd., Australia), following the manufacturer’s instructions. Previously, the buffalo DNA samples were genotyped using a sequencing approach, which revealed that all samples carried the A2A2 β-casein genotype and that the milk samples were A2 milk (Prabakusuma et al., Citation2022). The Mann-Whitney U test was performed in SPSS software v26.0 (IBM Corp., Armonk, NY, U.S.A.) for statistical analysis. The second and third parts of milk samples were prepared for untargeted metabolomics and label-free proteomics approaches, respectively.
2.2. Milk sample preparation for untargeted UPLC-MS/MS analysis
Before conducting untargeted UPLC-MS/MS analysis, the skim milk samples obtained from each breed of buffalo had been thawed at a temperature of 4°C. These samples from each breed of buffalo were then mixed to make a single sample of BBM and DBM. Those mixed samples were prepared with three biological replications for further analysis. An aliquot of 150 µl of each sample was transferred into 2 mL centrifuge tubes. A total of 200 µl methanol and 200 µl methyl tertiary-butyl ether were added into each centrifuge tube and thoroughly vortexed for 60 s. All samples were centrifuged at 4°C for 10 min at 12,000 rpm to separate large biomolecules. Before being subjected to autosampler vials, the supernatant was filtered through a 0.22-μm hydrophobic membrane to obtain the samples for LC-MS analysis. The metabolite from buffalo milk samples was extracted in accordance with several previous studies, with slight modifications (Shi et al., Citation2021; Tian et al., Citation2016; Villaseñor et al., Citation2014). The obtained filtrate in the centrifuge tube was kept at − 80°C until further analysis. To check the quality of the milk metabolite extract, the same amount of BBM and DBM samples was taken.
2.3. Untargeted UPLC-MS/MS analysis
An Acquity UPLC separation module with a column heater system (Waters Corp., Milford, MA, U.S.A.) was occupied. Chromatographic separation of milk metabolites was accomplished on a Thermo Ultimate 3000 system equipped with a UPLC® HSS T3 (150 × 2.1 mm, 1.8 µm) coupled with a Thermo Q Exactive HF-X mass spectrometer, which was installed with an electrospray ionization source (ESI) with a spray voltage of 3.5 kV (ESI+) and − 2.5 kV (ESI−). Ten µL of each milk sample was injected into the column at a flow rate of 0.45 mL/min. The data from mass spectrometry were obtained in both positive and negative ion modes. The analyzer scanned over a range of mass-to-charge ratios of 81–1,000 m/z for a full-scan at a mass resolution of R = 60,000. Data-dependent acquisition (DDA) MS/MS was performed with a high collision dissociation scan, and the normalized MS collision energy was 30 eV for precursor ions.
2.4. Database searching and metabolite identification
Raw MS/MS spectra were converted into mzXML format using Proteowizard software v3.0.8789. The XCMS package of R v3.1.3 was used to perform peak identification, filtration, and alignment processing to generate a data matrix, including mass-to-charge ratio, retention time, peak area, and precursor molecules in both positive and negative ion modes, which was exported to MS Excel for subsequent analysis (Smith et al., Citation2006). The metabolite identification was confirmed according to their molecular weight (MW), with an MW error of ≤ 30 ppm. The Human Metabolome Database (HMDB) (http://www.hmdb.ca), METLIN (http://metlin.scripps.edu), BioDeepDB (http://www.biodeep.cn/), MoNA (https://massbank.us/), MetDNA (http://metdna.zhulab.cn/), LipidMaps (http://www.lipidmaps.org), and mzCloud (https://www.mzcloud.org) were used to confirm the annotations according to the MS/MS fragmentation mode.
2.5. Milk sample preparation for quantitative label-free proteomics analysis
All milk samples were thawed at 4°C, 100 μL of each sample was taken, and 400 μL of 2X sodium deoxycholate (DOC) lysis buffer was added. Sample solutions were further sonicated on ice for 8 min with a 15% amplitude. Protein enzymatic hydrolysis was then performed according to the quantitative results of electrophoresis. Twenty μL of protein lysate for each sample was prepared for digestion. Thirty μL of 50 mM ammonium bicarbonate (ABC) pH 8.0 and 0.5 μL of 1 M dithiothreitol were added to each tube, mixed with a pipette, heated at 100°C for 5 minutes, centrifuged, and cooled to room temperature for denaturation. The protein lysate was transferred into a 10-kDa filter, centrifuged at 14,000 rpm for 10 min at 4°C, and 100 μL of 50 mM ABC was added and centrifuged again to obtain the filtrate. Fifty μL of 50 mM ABC and 0.5 μg of pancreatin enzyme were added to the filtrate in a tube and initially digested at 37°C for 4 h. The digested filtrate was digested again overnight at 37°C with the addition of 1 μg of pancreatin enzyme. The digested peptides were collected the next day, washed twice with 50 mM ABC, mixed with 20 μL of 10% formic acid (FA), precipitated with DOC, and centrifuged at 14,000 rpm for 10 min, and the peptide supernatant was taken for desalting.
2.6. HPLC-MS/MS analysis
HPLC-MS/MS analysis was performed with an Easy-nLC 1200 system coupled to an Orbitrap Fusion Lumos mass spectrometer (Thermo Fisher Scientific, Waltham, MA, U.S.A.) to generate peak lists and detect peptide signals from MS data acquisition. In this mass spectrometric analysis, a nano-electrospray ionization source (nano-ESI) with a spray voltage of 2,200 V and an ion transmission capillary temperature of 320°C was used. The prepared sample, as outlined in section 2.5, was dissolved in 100 μL of mobile phase A, consisting of 100% deionized water with a 0.1% FA aqueous solution for liquid chromatography separation. Data from mass spectrometry experiments used DDA in the positive ion mode method for acquisition. The total ion current chromatogram was obtained after scanning the mass spectrum signal (Figure S1). After first-round peptide-spectrum matching was completed, quantitative analysis was performed using the Sequest tool in the Proteome Discoverer software v2.4 (Thermo Fisher Scientific, Waltham, MA, U.S.A.). A ≤ 1% false discovery rate (FDR) was used at equal peptide and protein levels to increase the confidence and remove false-positive identification. The second round of peptide-spectrum filtering eliminated proteins supported by fewer than two distinct peptide identifications, and only identical proteins were conceded and grouped. The Proteome Discoverer software filtered the credible peptide spectrum matches (PSMs) from the search results, which contained at least one PSM with a credibility ≥ 99%. The UniProt database (Taxon ID: 89462) with protein records of 46,754 was used to annotate further matched spectra.
2.7. Bioinformatics analysis
The enrichment analysis was conducted using the Bubalus bubalis organism database in UniProtKB. The obtained protein list was exported to Microsoft Excel and subjected to the BioDeep bioinformatics resource to find GO annotations. The GO categories were processed and analyzed using the DAVID bioinformatics tool (https://david.ncifcrf.gov/), a free online resource that provides functional-related genes’ interpretation of enriched biological themes derived from genomic studies, and only the genes with a p-adjusted value of FDR of less than 0.05 were involved, and subsequent GO terms were plotted (Huang et al., Citation2009). GO enrichments were classified using Uniprot based on functional annotation involvement, including biological processes, molecular functions, and cellular components (Götz et al., Citation2008). The metabolic pathway analysis involved in differentially expressed genes was annotated using the KEGG database (https://www.genome.jp/kegg/) (Kanehisa et al., Citation2017). The clustering heat map was generated using the pheatmap package v2.0.3 (https://cran.r-project.org/web/packages/pheatmap/index.htmL). The in silico PPI analysis was performed using the STRING database platform v10.5 (https://string-db.org/), which allows for the critical integration and evaluation of the interactions among proteins, including physical direct and functional indirect associations (Szklarczyk et al., Citation2021). The results of the PPI analysis were then downloaded in XGMML format and input into Cytoscape56 software v3.9.0 (http://www.cytoscape.org/) to make a network diagram of the functional PPIs (Otasek et al., Citation2019).
3. Results and discussion
3.1. Routine analysis of buffalo milk composition
Before subjecting BBM and DBM to an untargeted metabolic analysis and a label-free proteomics approach, we analyzed the difference between the two milk types in the main nutritional composition, and the results are shown in . The concentrations of milk fat, lactose, total solids, and A2 β-casein protein isoform in BBM and DBM were not significantly different. However, only the protein content of BBM (4.25% ± 0.06) was significantly higher than that of DBM (4.18% ± 0.04) (p < .05) and slightly lower than that of the Murrah and Nili-Ravi buffalo breeds studied in Guangxi province (4.92% and 4.54%, respectively) (Zhou et al., Citation2018). Using a genomic sequencing approach for single nucleotide polymorphism (SNP) genotyping, all buffalo DNA samples that corresponded with the milk samples observed in this study were determined to carry the A2A2 β-casein genotype only and all milk samples were categorized as A2 β-casein milk variant. It indicated that the mutation Pro67/His67 in the amino acid sequence located at exon 7 of chromosome 6 in the CNS2 gene encoded β-casein milk phenotype was absent in the buffaloes. This secondary result was also consistent with the findings reported by Pineda et al. (Citation2019) and de Oliveira et al. (Citation2021), who genotyped several buffalo breeds of water and swamp types in the Philippines and Brazil, respectively. Our present study has demonstrated the reliability of quantifying A2 β-casein protein isoform concentration in fresh buffalo milk samples through the use of the sandwich ELISA. This method used a chicken anti-bovine A2 β-casein detection antibody, a pre-coated rabbit anti-bovine A2 β-casein polyclonal capture antibody, and a horseradish peroxidase-conjugated secondary donkey anti-chicken IgY antibody for signal amplification. The results revealed that the average concentration of A2 β-casein protein isoform in DBM was higher than that in BBM (). The optical density values of the two milk types detected by a sandwich ELISA assay were associated with the positive interaction between the A2 β-casein protein isoform and the enzyme-labelled antibodies.
Table 1. Milk composition comparison of BBM and DBM.
3.2. Buffalo milk metabolite profiles
This present study is the first investigation to simultaneously elucidate differential metabolites and proteomes of water and swamp buffalo milk from two famous native breeds in Yunnan province. To investigate the difference in metabolite profiles of BBM and DBM, an Acquity UPLC-MS/MS coupled to a Thermo Q Exactive HF-X high-resolution mass spectrometry was used, and 500 and 319 metabolites were identified in ESI+ and ESI – modes, respectively (Table S1). The information on the determination of major differential milk metabolite compounds showed an apparent propensity for separation between the two buffaloes (p ≤ .05), and the information on the determination of those milk metabolite compounds is presented in . A continuous scan of average m/z was controlled between 80 and 790 at different retention times within 1.38–13.07, indicating that the most differential metabolite compounds were detected within 1–4 min. The UPLC-MS/MS analysis results indicated that pipecolic acid, fomepizole, 5-hydroxyindoleacetic acid, 1,2,3-trihydroxybenzene, and maltol metabolite compounds detected in [M+H]+ were more relatively abundant with a higher peak area than other metabolites in BBM, which were 22.18 ppm, 16.09 ppm, 16.04 ppm, 15.21 ppm, and 13.05 ppm, respectively ().
Table 2. Determination of major differential metabolite compounds of BBM by UPLC-MS/MS.
Table 3. Determination of major differential metabolite compounds of DBM by UPLC-MS/MS.
In bovine animals, particularly in buffalo, the greater concentrations of L-pipecolic acid in milk may be linked to and involved in the lysine degradation pathway (bbub00310), especially to form L-2-aminoadipate 6-semialdehyde (Jorge-Smeding et al., Citation2021). In addition, fomepizole compounds play an essential role in blocking zinc-containing alcohol dehydrogenase (ADH) to promote acute alcoholic liver damage in buffalo (bbub04936) and other mammals (Tvrdý et al., Citation2021). 5-hydroxyindoleacetic acid (5HIAA), a biogenic amine related to the C3 carbon atom of an indole that is a primary metabolite of serotonin, metanephrines, or catecholamines (bbub_M00042), is catalyzed by the mitochondrial enzyme aldehyde dehydrogenase and commonly used as a neuroendocrine tumor secretory biomarker (Corcuff et al., Citation2017). Related to bovine milk processing, Kokkinidou and Peterson (Citation2014) reported that the addition of phenolic compound 1,2,3-trihydroxybenzene prior to ultrahigh temperature pasteurization significantly decreased the key transient of Maillard reaction (MR) intermediates and linked off-flavor compounds. Jo et al. found that during pasteurization, changes in the amino groups and lactose milk proteins in MR were caused by the heat treatment process. These changes caused the amino groups and lactose milk proteins to unfold, which made sweet aromatic volatile compounds like maltol (Jo et al., Citation2017).
In DBM, the most relatively abundant metabolites were cellobiose (29.68 ppm), 2-deoxystreptamine (20.86 ppm), phloroglucinol (13.13 ppm), 1,2,3-trihydroxybenzene (12.22 ppm), and creatine (10.34 ppm) (). Cellobiose was identified in [M – H]– and is involved in several pathways, including starch and sucrose metabolism (ko00500), metabolic pathways (ko01100), ATP-binding cassette transporters (ko02010), and the phosphotransferase system (ko02060). The pyranosyl groups in cellobiose and lactose are connected to the C-4 atom of d-glucose by a β-(1,4’) glycosidic linkage (Ouellette & Rawn, Citation2019). The high concentration of 2-deoxystreptamine found in DBM, identified in ESI+ [M+H]+, was strongly associated with biosynthesis of secondary metabolites (ko01110) and had an essential function in antimicrobial activity against protozoal and bacterial infections (ko00524). Through balancing metabolic hydrogen in the bovine rumen, the phenolic compound phloroglucinol contributes to stimulating ruminant methane reduction (Martinez-Fernandez et al., Citation2017; Sarwono et al., Citation2019). In DBM, the 1,2,3-trihydroxybenzene differential metabolite compound was also found in a higher concentration, although lower than in BBM. Additionally, creatine plays a vital role in arginine and proline metabolism (bbub00330) and any metabolic pathway (bbub01100) in buffalo. Creatine, along with important metabolic sources like glycine, glutathione, and serine plays an important role in a number of biological processes, such as the activity of lactating mammary epithelial cells, the balance of energy in bovine animals, and their response to heat stress (López et al., Citation2021; Sun et al., Citation2017).
Catechol, L-proline, γ-aminobutyric acid (GABA), hippuric acid, creatinine, betaine, D-fructose, taurine, cytosine, L-carnitine, and butyryl-L-carnitine were also detected in both buffalo milk groups as the major metabolite compounds with a high concentration. The mass-to-charge in mzXML format of those metabolites converted from MS/MS spectra is shown in Figure S2. Previous studies have reported that the differential metabolite and proteome profiles of buffalo milk vary by breed, location, and condition of the rearing region, and it was estimated that technological properties in making dairy by-products also change (de Nicola et al., Citation2020; Garau et al., Citation2021; Li et al.,Citation2018, Citation2021; Pu et al., Citation2021; Salzano et al., Citation2020; Shi et al., Citation2021). These results were also in line with our current research, which found that the metabolomes and proteomes of buffalo milk were different in light of breed, especially between the BBM and DBM.
3.3. Buffalo milk proteome profiles
One hundred thirteen proteins were identified and quantified through analyzing the BBM and DBM datasets resulting from the label-free HPLC-MS/MS-based proteomics approach. The specific number of identified proteins in BBM and DBM is demonstrated in . Of these identified proteins, there were 96 quantitative proteins identified in BBM, which is higher than those in DBM, with 64 proteins detected, and 47 proteins were found in both buffalo milk samples (Table S4). For quality control of the distributions of all the identified protein quantitative values in the corresponding samples, analyses of the relative standard deviation (RSD) and correlation coefficients between two samples were performed and are showcased in . The measurements showed that the coefficient of variation (CV), which is the ratio of the standard deviation to the mean value, was greatly reduced after data correction. This led to a lower CV, which showed good repeatability and reproducibility in identifying the same analyte under different conditions and with certain adjustments.
Figure 2. (a) RSD coefficient distribution before and after data correction of all the protein quantitative values in the corresponding sample. A lower CV coefficient indicated better repeatability of all samples. (b) Pearson correlation coefficient of all quantitative protein expressions between the two corresponding samples.
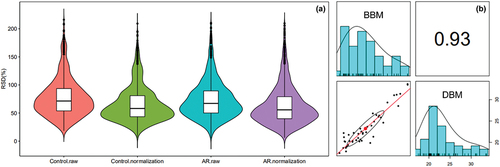
Furthermore, the analysis of the physical and chemical properties of the identified proteins showed that the iso-electric point (pI) value ranged between 4.5 and 5.5, the MW was mainly observed within 20–80 kDa (Figure S3a), the mass error was centered at 0 and concentrated in the range below 10 ppm (Figure S3b), and most of the peptide lengths were distributed between 7 and 23 amino acid residues (Figure S4). The results indicated that the pI, MW, mass error, and peptide length met the requirements and conformed to the rule of trypsin digestion of peptides. The cumulative distribution of quantitative protein value and the degree of dispersion of the data distribution in the corresponding samples were also evaluated and shown in Figure S5, where it was concluded that the protein quantitative value distribution between two buffalo milk samples was not completely the same. Both BBM and DBM suggested containing the same common proteomes in large numbers, which also included four types of phosphoprotein casein family; these were α-s1-casein (CSN1S1), α-s2-casein (CSN1S2), β-casein (CSN2), κ-casein (CSN3), albumin (ALB), α-lactalbumin (LALBA), lipoprotein lipase (LPL), lactotransferrin (LTF), fatty acid-binding protein (FABP3), and progestogen associated endometrial protein (PAEP) (Table S4). Three out of four caseins were detected in higher quantities in BBM than those in DBM; those were α-s1-casein, α-s2-casein, β-casein (Table S4). Among the casein family, α-s2-casein is the most hydrophilic, with more stable calcium-phosphate micelles, non-thermostable, non-acidic peptides, and has a lower efficiency in translation than other caseins (Cosenza et al., Citation2011; Rehman et al., Citation2021). Previous studies reported that α-s1-casein, α-s2-casein, β-casein, and β-lactoglobulin were found to be among the most abundant proteins in bovine milk based on qualitative similarity profiling analysis and were utilized further as the key protein ingredients in infant formula production as well (Raikos & Dassios, Citation2014; Smolenski et al., Citation2014; Xu et al., Citation2022).
The α-s1-casein protein (Uniprot ID O62823) is highly related to compositional aspects in buffalo milk, where a higher content of α-s1-casein exerts higher protein and total solids, increasing appropriateness in cheese-making properties (Kashwa, Citation2016). In humans, α-s1-casein contributes to the immune system’s development, delivers caseins from the endoplasmic reticulum to the Golgi apparatus, and provides a source of amino acids for newborns (Stocco et al., Citation2018; Zhang et al., Citation2021a, Citation2021b). The α-s2-casein protein-coding gene (O62825) plays an essential role in the capable transportation of calcium phosphate (Cosenza et al., Citation2021; Rehman et al., Citation2021). Lactotransferrin (O77698), also known as lactoferrin, is a major iron-binding transport protein that regulates antimicrobial activity in terms of the prevention of microbiological infection diseases, which is related to its ability to sequester free iron and to release lipopolysaccharides from the bacterial outer membrane (Giacinti et al., Citation2013). In relation to human health, lactoferrin is responsible for various critical functional actions in preventing necrotizing enterocolitis and sepsis and reducing infection risks associated with respiratory and gastrointestinal pathogens in neonates, infants, and young children (Björmsjö et al., Citation2022; He et al., Citation2018; Manzoni, Citation2016; Manzoni et al., Citation2018). Moreover, albumin (P02769) has a ligand-binding capacity in the transportation of a diverse range of molecules, nutrients, metals, and metabolites and controls the growth of enterobacteriaceae, such as Escherichia coli and Salmonella enterica, through its ability to bind the siderophore enterobactin and inhibit enterobactin-mediated iron uptake of bacteria (Behnsen et al., Citation2021; Majorek et al., Citation2012).
Bovine milk casein contributes to multifunctional functions, including stimulating cellular immune functions, providing amino acids and calcium, and stabilizing chemotactic responses (Kawasaki et al., Citation2011; Vordenbaumen et al., Citation2013, Citation2016; Wada & Lonnerdal, Citation2014; Yang et al., Citation2016). In dairy by-product making, such as cheese manufacturing, casein structure and composition are technically influential. The understanding of buffalo milk protein expressions expands the insights about the behavior of curd formation and the aggregating mechanisms of Ca2+ sensitive proteins via calcium-phosphate bridges in casein micelle, in particular strongly related to κ-casein content and reversible protein post-translational modifications (PTMs) (D’Ambrosio et al., Citation2008; Fan et al., Citation2019; Gai et al., Citation2021; Li et al., Citation2016; Nguyen et al., Citation2017). However, the low relative abundance of proteins presented in BBM and DBM also plays an essential function in several signaling pathways in buffalo milk production, including membrane/protein trafficking (Ras-related protein Rap-1b, Ras-related protein Rab-18, ADP-ribosylation factor 2), cell signaling (CIDE-N domain-containing protein), defense/immunity (HHIP like 2), and fat transport/metabolism (diazepam binding inhibitor, acyl-CoA binding protein).
3.4. GO enrichments of identified proteins
All identified proteins in BBM and DBM were subjected to GO enrichment analysis to obtain insights into three distinctive functional classifications and characteristics, particularly in exploring the possible molecular mechanisms influencing buffalo milk production. GO analysis demonstrated that 73 identified proteins were enriched in a broad range of complex biological processes, molecular functions, and cellular components, which were classified into 22, 6, and 16 functional categories, respectively (p < .05) (). The GO enrichments for mapping upregulated differentially expressed proteins in BBM and DBM are listed in Table S2 accordingly. Among those proteins, the biological processes are mainly associated with cellular processes (11%), biological regulation (9%), regulation of biological processes (9%), metabolic processes (8%), response to stimulus (8%), and multicellular organismal processes (6%) (). Expressed proteins related to cell, biological regulation, and metabolic processes, as well as homeostasis, are well known in relation to buffalo milk production and composition (Du et al., Citation2019, Citation2020).
The cellular process terms (GO:0009987) with the largest number of expressed proteins (38) in the biological processes and networks play an important role in physiological changes in the buffalo mammary gland and ovarian follicle to produce milk, which is mainly related to cell differentiation, proliferation, apoptosis, and intracellular transportation (Buaban et al., Citation2022; Ye et al., Citation2022). In terms of the molecular functions, those expressed proteins included binding (53%), catalytic activity (20%), molecular function regulator (14%), structural molecule activity (8%), and antioxidant activity (3%) (). The binding terms (GO:0005488) with the highest number of expressed proteins (34) in molecular function describe the non-covalent, selective, often stoichiometric, interaction of a molecule with one or more molecules or certain sites. While the primary cellular component terms involved in cells, cell parts, organelles, extracellular regions, and membranes were 17%, 16%, 14%, 10%, and 8%, respectively. The cell terms (GO:0005623) with the most expressed proteins (38) in cellular components are in charge of basic structural and functional units in buffalo ().
3.5. KEGG pathways of differential expressed proteins
The KEGG annotations provided a critical reference for investigating the functions and biological systems in buffalo milk. In this study, 52 enriched KEGG maps were obtained using the identified proteins, which were primarily related to the top 20 pathways, and many proteins in those pathways were associated with cholesterol metabolism (bbub04979), Staphylococcus aureus (S. aureus) infection (bbub05150), peroxisome proliferator-activated receptor (PPAR) signaling pathway (bbub03320), coagulation cascades (bbub04610), and Salmonella infection (bbub05132) ( and Table S3). Forty-five genes were detected in differential protein expression linked to those functional pathways. KEGG enrichment analysis revealed the highest number of involved genes in the KEGG pathway was exhibited in S. aureus infection with a rich factor of 0.06 (p < .05), including complement C3 (C3), complement component 4A (C4A), keratins (KRT15, KRT18, KRT28), and complement factor B (CFB) genes. While NPC intracellular cholesterol transporter 2 (NPC2), lipoprotein lipase (LPL), CD36 molecule (CD36), apolipoprotein A1 (APOA1), and angiopoietin-like 4 (ANGPTL4) genes were identified in cholesterol metabolism; LPL, CD36, APOA1, ANGPTL4, and fatty acid-binding protein 3 (FABP3) were in the PPAR signaling pathway; C3, C4A, serpin family A member 1 (SERPINA1), clusterin (CLU), and complement factor B (CFB) were in coagulation cascades; and β-actin (ACTB), myeloid cell-specific leucine-rich glycoprotein CD14 (CD14), ras homolog GTPase family member A and B (RhoA, RhoB), and leucocyte-specific cell division cycle 42 homolog precursor (CDC42) genes were in Salmonella infection.
We also found eight significantly upregulated differentially expressed protein-coding genes in BBM and DBM in the top 20 enriched pathways, including family keratin of epithelial cell markers (KRT15, KRT18, KRT28); ACTB, determining cell motility in relaying chemical signals; C3, regulating the innate immune system; RhoA, RhoB, transducing extracellular signals to the actin cytoskeleton for modifying axon growth and outgrowth cone motility; and CDC42, involved in epithelial cell polarization processes (Table S3). Together with lymphocytes, neutrophils, and macrophages, exfoliated epithelial cells comprise milk somatic cells (Lu et al., Citation2016; Robenek et al., Citation2006). Mammary epithelial cells can develop apocrine secretion to produce milk fat, referred to as milk fat globule proteins, after being formed in the endoplasmic reticulum membranes and delivered to the apical plasma membrane (Walter et al., Citation2020). Milk fat globule proteins are linked to a number of biological functions and are a key indicator of the nutritional value of bovine milk (Zhang et al., Citation2021a).
3.6. PPI networks
Protein expression data were converted to co-expression networks in this experiment for a network-level comparison of the BBM and DBM proteomes, visualizing protein-protein interactions in both breeds. The interaction score is set to 0.4, and the density of observed high interactions was significant according to the statistical calculation available in STRING (p < 10−6). One hundred fifteen experimentally determined interactions and 359 nodes, with approximately 90% of the proteins having at least two or more connections, were observed. The combined score ranged from 0.401 to 0.999, with the highest score of the co-expression protein network being 0.925 (Table S5). The preponderance of multitudinous interactions among proteins in the PPI network demonstrated a strongly interconnected network where most proteins influenced the expression of other proteins related to buffalo milk synthesis (). The PPI network for BBM and DBM proteomes also exhibited a dense core with highly interconnected proteins.
Figure 5. Ppis of the identified proteins in BBM and DBM. STRING database was used to establish the protein-protein interactions of quantified proteins identified by HPLC-MS/MS. Each node in the PPI network represents an identified protein, and the type of node represents the degree of connection of the protein.
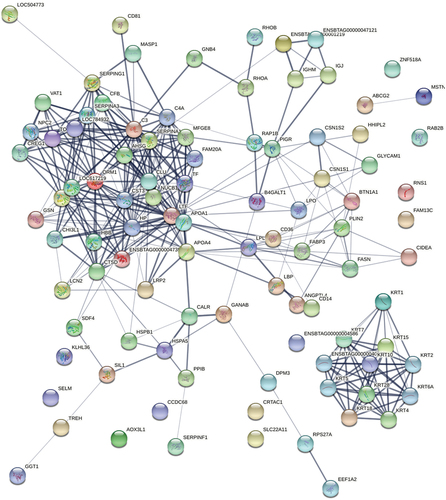
4. Conclusion
This present study provides detailed investigation results for differential metabolites and proteomes of BBM and DBM through an untargeted metabolomics and quantitative label-free proteomics approach. Main polypeptide fractions, including α-s1-casein, β-s2-casein, β-casein, κ-casein, and α-lactalbumin, were identified in higher protein abundances. Differentially expressed proteins were primarily involved in regulating cholesterol metabolism, the infection of S. aureus and Salmonella, the peroxisome proliferator-activated receptor signaling pathway, and coagulation cascades. By elucidating some of the primary differential metabolites and proteomes influencing cheese-making properties and organoleptic qualities, together with total protein/fat content, Ca2+ concentration, and pH level, this study demonstrates a fundamental basis for the coagulation mechanisms applied in dairy production. Additionally, the number of minor protein fractions detected in this study also emphasizes the multiple functions of buffalo milk, particularly in providing nutrition for the newborn through its significant metabolites and protein components, supporting neonate development, and promoting the protection of the gastrointestinal tract from pathogens. Our findings provided a more in-depth reference for both human health and dairy food manufacturers based on buffalo milk processing.
Supplemental Material
Download MS Word (783.7 KB)Acknowledgments
We would like to extend our heartfelt gratitude to the anonymous reviewers and colleagues for their constructive comments and advice on this manuscript. We also thank Professor Huaming Mao in College of Animal Science and Technology, Yunnan Agricultural University, and Suzhou Panomic Biomedical Technology Co., Ltd., China, for experimental support.
Disclosure statement
No potential conflict of interest was reported by the author(s).
Supplementary material
Supplemental data for this article can be accessed online at https://doi.org/10.1080/19476337.2023.2264953.
Additional information
Funding
References
- Alichanidis, E., Moatsou, G., & Polychroniadou, A. (2016). Chapter 5 – Composition and properties of non-cow milk and products. In E. Tsakalidou & K. Papadimitriou (Eds.), Non-bovine milk and milk products (pp. 81–116). Academic Press. Retrieved December 22, 2021, from https://doi.org/10.1016/C2014-0-04073-7
- Arora, S., Sindhu, J. S., & Khetra, Y. (2022). Buffalo milk. In P. L. H. McSweeney & J. P. McNamara (Eds.), Encyclopedia of dairy sciences (3rd ed., pp. 784–796). Academic Press. Retrieved May 10, 2022, from https://doi.org/10.1016/B978-0-12-818766-1.00125-2
- Arslan, A., Kaplan, M., Duman, H., Bayraktar, A., Ertürk, M., Henrick, B. M., Frese, S. A., & Karav, S. (2021). Bovine colostrum and its potential for human health and nutrition. Frontiers in Nutrition, 8, 651721. https://doi.org/10.3389/fnut.2021.651721
- Behnsen, J., Zhi, H., Aron, A. T., Subramanian, V., Santus, W., Lee, M. H., Gerner, R. R., Petras, D., Liu, J. Z., Green, K. D., Price, S. L., Camacho, J., Hillman, H., Tjokrosurjo, J., Montaldo, N. P., Hoover, E. M., Treacy-Abarca, S., Gilston, B. A. Dorrestein, P. C. (2021). Siderophore-mediated zinc acquisition enhances enterobacterial colonization of the inflamed gut. Nature Communications, 12(1), 7016. https://doi.org/10.1038/s41467-021-27297-2
- Bertoni, A., Álvarezmacías, A., Mota-Rojas, D., Dávalos, J. L., & Minervino, A. H. H. (2021). Dual-purpose water buffalo production systems in tropical Latin America: Bases for a sustainable model. Animals, 11(10), 2910. https://doi.org/10.3390/ani11102910
- Bittante, G., Amalfitano, N., Bergamaschi, M., Patel, N., Haddi, M. L., Benabid, H., Pazzola, M., Vacca, G. M., Tagliapietra, F., & Schiavon, S. (2022). Composition and aptitude for cheese-making of milk from cows, buffaloes, goats, sheep, dromedary camels, and donkeys. Journal of Dairy Science, 105(3), 2132–2152. https://doi.org/10.3168/jds.2021-20961
- Björmsjö, M., Hernell, O., Lönnerdal, B., & Berglund, S. K. (2022). Immunological effects of adding bovine lactoferrin and reducing iron in infant formula: A randomized controlled trial. Journal of Pediatric Gastroenterology and Nutrition, 74(3), e65–e72. https://doi.org/10.1097/MPG.0000000000003367
- Buaban, S., Lengnudum, K., Boonkum, W., & Phakdeedindan, P. (2022). Genome-wide association study on milk production and somatic cell score for Thai dairy cattle using weighted single-step approach with random regression test-day model. Journal of Dairy Science, 105(1), 468–494. https://doi.org/10.3168/jds.2020-19826
- Corcuff, J. B., Chardon, L., El Hajji Ridah, I., & Brossaud, J. (2017). Urinary sampling for 5HIAA and metanephrines determination: Revisiting the recommendations. Endocrine Connections, 6(6), R87–R98. https://doi.org/10.1530/EC-17-0071
- Cosenza, G., Gallo, D., Auzino, B., Gaspa, G., & Pauciullo, A. (2021). Complete CSN1S2 characterization, novel allele identification and association with milk fatty acid composition in River buffalo. Frontiers in Genetics, 11, 622494. https://doi.org/10.3389/fgene.2020.622494
- Cosenza, G., Pauciullo, A., Coletta, A., DiFrancia, A., Feligini, M., Gallo, D., DiBerardino, D., & Ramunno, L. (2011). Short communication: Translational efficiency of casein transcripts in mediterranean river buffalo. Journal of Dairy Science, 94(11), 5691–5694. https://doi.org/10.3168/jds.2010-4086
- D’Ambrosio, C., Arena, S., Salzano, A. M., Renzone, G., Ledda, L., & Scaloni, A. (2008). A proteomics characterization of water buffalo milk fractions describing PTM of major species and the identification of minor components involved in nutrient delivery and defense against pathogens. Proteomics, 8(17), 3657–3666. https://doi.org/10.1002/pmic.200701148
- Davoodi, S. H., Shahbazi, R., Esmaeili, S., Sohrabvandi, S., Mortazavian, A., Jazayeri, S., & Taslimi, A. (2016). Health-related aspects of milk proteins. Iranian Journal of Pharmaceutical Sciences, 15(3), 573–591. Retrieved December 22, 2021, from https://www.ncbi.nlm.nih.gov/pmc/articles/PMC5149046/pdf/ijpr-15-573.pdf
- de Nicola, D., Vinale, F., Salzano, A., d’Errico, G., Vassetti, A., D’Onofrio, N., Balestrieri, M. L., & Neglia, G. (2020). Milk metabolomics reveals potential biomarkers for early prediction of pregnancy in buffaloes having undergone artificial insemination. Animals, 10(5), 758. https://doi.org/10.3390/ani10050758
- de Oliveira, L. S. M., Alves, J. S., Bastos, M. S., da Cruz, V. A. R., Pinto, L. F. B., Tonhati, H., Costa, R. B., & de Camargo, G. M. F. (2021). Water buffaloes (Bubalus bubalis) only have A2A2 genotype for beta-casein. Tropical Animal Health and Production, 53(1), 145. https://doi.org/10.1007/s11250-021-02591-9
- Du, C., Deng, T. X., Zhou, Y., Ghanem, N., & Hua, G. H. (2020). Bioinformatics analysis of candidate genes for milk production traits in water buffalo (Bubalus bubalis). Tropical Animal Health and Production, 52(1), 63–69. https://doi.org/10.1007/s11250-019-01984-1
- Du, C., Deng, T., Zhou, Y., Ye, T., Zhou, Z., Zhang, S., Shao, B., Wei, P., Sun, H., Khan, F. A., Yang, L., & Hua, G. (2019). Systematic analyses for candidate genes of milk production traits in water buffalo (Bubalus bubalis). Animal Genetic, 50(3), 207–216. https://doi.org/10.1111/age.12739
- El-Salam, M. H. A., & El-Shibiny, S. (2011). A comprehensive review on the composition and properties of buffalo milk. Dairy Science and Technology, 91(6), 663–699. https://doi.org/10.1007/s13594-011-0029-2
- Faccia, M., D’Alessandro, A. G., Summer, A., & Hailu, Y. (2020). Milk products from minor dairy species: A review. Animals, 10(8), 1260. https://doi.org/10.3390/ani10081260
- Fan, X., Zhang, Z., Qiu, L., Zhang, Y., & Miao, Y. (2019). Polymorphisms of the kappa casein (CSN3) gene and inference of its variants in water buffalo (Bubalus bubalis). Archives Animal Breeding, 62(2), 585–596. https://doi.org/10.5194/aab-62-585-2019
- Figliola, L., Santillo, A., Ciliberti, M. G., Caroprese, M., & Albenzio, M. (2021). Chapter 7 – Nonbovine milk products as probiotic and prebiotic food. In A. G. da Cruz, C. S. Ranadheera, F. Nazzaro & A. Mortazavian (Eds.), Probiotics and prebiotics in foods (pp. 115–133). Academic Press. Retrieved December 30, 2021, from https://doi.org/10.1016/B978-0-12-819662-5.00007-0
- Gai, N., Uniacke-Lowe, T., O’Regan, J., Faulkner, H., & Kelly, A. L. (2021). Effect of protein genotypes on physicochemical properties and protein functionality of bovine milk: A review. Foods, 10, 2409. https://doi.org/10.3390/foods10102409
- Garau, V., Manis, C., Scano, P., & Caboni, P. (2021). Compositional characteristics of mediterranean buffalo milk and whey. Dairy, 2(3), 469–488. https://doi.org/10.3390/foods10102409
- Giacinti, G., Basiricò, L., Ronchi, B., & Bernabucci, U. (2013). Lactoferrin concentration in buffalo milk. Italian Journal of Animal Science, 12(1), 1. https://doi.org/10.4081/ijas.2013.e23
- Götz, S., García-Gómez, J. M., Terol, J., Williams, T. D., Nagaraj, S. H., Nueda, M. J., Robles, M., Talón, M., Dopazo, J., & Conesa, A. (2008). High-throughput functional annotation and data mining with the Blast2GO suite. Nucleic Acids Research, 36(10), 3420–3435. https://doi.org/10.1093/nar/gkn176
- Guo, M., & Hendricks, G. 2010. Chapter 14 – Improving buffalo milk. In M. W. Griffiths Ed. Improving the safety and quality of milk: Improving quality in milk products (pp. 402–416). Woodhead Publishing. https://doi.org/10.1533/9781845699437.3.402
- Han, B. Z., Meng, Y., Li, M., Yang, Y. X., Ren, F. Z., Zeng, Q. K., & Robert, N. M. (2007). A survey on the microbiological and chemical composition of buffalo milk in China. Food Control, 18(6), 742–746. https://doi.org/10.1016/j.foodcont.2006.03.011
- He, Y., Cao, L., & Yu, J. (2018). Prophylactic lactoferrin for preventing late-onset sepsis and necrotizing enterocolitis in preterm infants: A PRISMA-compliant systematic review and meta-analysis. Medicine (Baltimore), 97(35), e11976. https://doi.org/10.1097/MD.0000000000011976
- Huang, W., Sherman, B. T., & Lempicki, R. A. (2009). Systematic and integrative analysis of large gene lists using DAVID bioinformatics resources. Nature Protocols, 4(1), 44–57. https://doi.org/10.1038/nprot.2008.211
- Jiang, M., Meng, Z., Cheng, Z., Zhan, K., Ma, X., Yang, T., Huang, Y., Yan, Q., Gong, X., & Zhao, G. (2022). Effects of buffalo milk and cow milk on lipid metabolism in obese mice induced by high fat. Frontiers in Nutrition, 9, 841800. https://doi.org/10.3389/fnut.2022.841800
- Jo, Y., Benoist, D. M., Barbano, D. M., & Drake, M. A. (2017). Flavor and flavor chemistry differences among milks processed by high-temperature, short-time pasteurization or ultra-pasteurization. Journal of Dairy Science, 101(5), 3812–3828. https://doi.org/10.3168/jds.2017-14071
- Jorge-Smeding, E., Carriquiry, M., Cantalapiedra-Hijar, G., Mendoza, A., & Astessiano, A. L. (2021). Plasma concentrations of branched-chain amino acids differ with holstein genetic strain in pasture-based dairy systems. Science Report, 11(1), 22414. https://doi.org/10.1038/s41598-021-01564-0
- Kanehisa, M., Furumichi, M., Tanabe, M., Sato, Y., & Morishima, K. (2017). KEGG: New perspectives on genomes, pathways, diseases and drugs. Nucleic Acids Research, 45(D1), D353–D361. https://doi.org/10.1093/nar/gkw1092
- Kashwa, M. (2016). Composition of water buffalo milk during the first period of lactation - relation to mozzarella cheese properties [ Master Thesis]. Department of Animal Nutrition and Management, Swedish University of Agricultural Sciences. Retrieved January 13, 2022, from https://stud.epsilon.slu.se/8724/7/kashwa_m_160108.pdf
- Kawasaki, K., Lafont, A. G., & Sire, J. Y. (2011). The evolution of milk casein genes from tooth genes before the origin of mammals. Molecular Biology and Evolution, 28(7), 2053–2061. https://doi.org/10.1093/molbev/msr020
- Kokkinidou, S., & Peterson, D. G. (2014). Control of maillard-type off-flavor development in ultrahigh-temperature-processed bovine milk by phenolic chemistry. Journal of Agricultural and Food Chemistry, 62(32), 8023–8033. https://doi.org/10.1021/jf501919y
- Leischner, C., Egert, S., Burkard, M., & Venturelli, S. (2021). Potential protective protein components of cow’s milk against certain tumor entities. Nutrients, 13(6), 1974. https://doi.org/10.3390/nu13061974
- Li, S., Li, L., Zeng, Q., Liu, J., & Ren, D. (2016). Separation and quantification of milk casein from different buffalo breeds. Journal of Dairy Research, 83(3), 317–325. https://doi.org/10.1017/S0022029916000455
- Li, S., Li, L., Zeng, Q., Liu, J., Yang, Y., & Ren, D. (2018). Quantitative differences in whey proteins among murrah, Nili-Ravi and mediterranean buffaloes using a TMT proteomics approach. Food Chemistry, 15(269), 228–235. https://doi.org/10.1016/j.foodchem.2018.06.122
- Li, S., Yang, Y., Chen, C., Li, L., Valencak, T. G., & Ren, D. (2021). Differences in milk fat globule membrane proteins among Murrah, Nili-Ravi and mediterranean buffaloes revealed by a TMT proteomics approach. Food Research International, 139, 109847. https://doi.org/10.1016/j.foodres.2020.109847
- López, R. A., Adrien, M. D. L., Ruprechter, G., de Torres, E., Meikle, A., & Moyna, G. (2021). Monitoring the transition period in dairy cows through 1H NMR-based untargeted metabolomics. Dairy, 2(3), 356–366. https://doi.org/10.3390/dairy2030028
- Lu, J., Argov-Argaman, N., Anggrek, J., Boeren, S., van Hooijdonk, T., Vervoort, J., & Hettinga, K. A. (2016). The protein and lipid composition of the membrane of milk fat globules depends on their size. Journal of Dairy Science, 99(6), 4726–4738. https://doi.org/10.3168/jds.2015-10375
- Lv, Z., Liu, H., Yang, Y., Bu, D., Zang, C., Yang, K., Yu, X., & Wang, J. (2020). Changes in metabolites from bovine milk with β-casein variants revealed by metabolomics. Animals, 10(6), 954. https://doi.org/10.3390/ani10060954
- Majorek, K. A., Porebski, P. J., Dayal, A., Zimmerman, M. D., Jablonska, K., Stewart, A. J., Chruszcz, M., & Minor, W. (2012). Structural and immunologic characterization of bovine, horse, and rabbit serum albumins. Molecular Immunology, 52(3–4), 174–182. https://doi.org/10.1016/j.molimm.2012.05.011
- Manzoni, P. (2016). Clinical benefits of lactoferrin for infants and children. The Journal of Pediatrics, 173(Suppl), S43–52. https://doi.org/10.1016/j.jpeds.2016.02.075
- Manzoni, P., Dall’agnola, A., Tomé, D., Kaufman, D. A., Tavella, E., Pieretto, M., Messina, A., De Luca, D., Bellaiche, M., Mosca, A., Piloquet, H., Simeoni, U., Picaud, J. C., & Del Vecchio, A. (2018). Role of lactoferrin in neonates and infants: An update. American Journal of Perinatology, 35(6), 561–565. https://doi.org/10.1055/s-0038-1639359
- Martinez-Fernandez, G., Denman, S. E., Cheung, J., & McSweeney, C. S. (2017). Phloroglucinol degradation in the rumen promotes the capture of excess hydrogen generated from methanogenesis inhibition. Frontiers in Microbiology, 8, 1871. https://doi.org/10.3389/fmicb.2017.01871
- Mejares, C. T., Huppertz, T., & Chandrapala, J. (2022). Thermal processing of buffalo milk – A review. International Dairy Journal, 129, 105311. https://doi.org/10.1016/j.idairyj.2021.105311
- Minervino, A. H. H., Zava, M., Vecchio, D., & Borghese, A. (2020). Bubalus bubalis: A short story. Frontiers in Veterinary Science, 7, 570413. https://doi.org/10.3389/fvets.2020.570413
- Nguyen, H. T. H., Ong, L., Lopez, C., Kentish, S. E., & Gras, S. L. (2017). Microstructure and physicochemical properties reveal differences between high moisture buffalo and bovine mozzarella cheeses. Food Research International, 102, 458–467. https://doi.org/10.1016/j.foodres.2017.09.032
- OECD/FAO. (2021). OECD-FAO agricultural outlook 2021-2030. OECD Publishing. Retrieved December 17, 2021, from https://doi.org/10.1787/19428846-en
- Otasek, D., Morris, J. H., Bouças, J., Pico, A. R., & Demchak, B. (2019). Cytoscape automation: Empowering workflow-based network analysis. Genome Biology, 20(1), 185. https://doi.org/10.1186/s13059-019-1758-4
- Ouellette, R. J., & Rawn, J. D. (2019). Chapter 28 – Carbohydrates. In R. J. Ouellette & J. D. Rawn (Eds.),Organic chemistry: Structure, mechanism, synthesis. (2nd ed.). Academic Press. Retrieved January 11, 2022, from https://doi.org/10.1016/C2016-0-04004-4
- Pineda, P. S., Santos, J. D. D., & Flores, E. B. (2019). Genetic polymorphism of β-casein exon 7 in buffaloes. Philippine Journal of Veterinary and Animal Sciences, 45(3), 197–202. Retrieved January 11, 2022, from https://ovcre.uplb.edu.ph/journals-uplb/index.php/PJVAS/article/view/293.
- Prabakusuma, A. S., Aleryani, H., Kong, X., Shi, X., & Huang, A. (2022). Genotyping, physicochemical characterization, and protein isoform quantification of β-casein A2 milk in chinese simmental and Angus cattle. Emirates Journal of Food and Agriculture, 34(8), 675–687. https://doi.org/10.9755/ejfa.2022.v34.i8.2882
- Pu, J., Vinitchaikul, P., Gu, Z., Mao, H., & Zhang, F. (2021). The use of metabolomics to reveal differences in functional substances of milk whey of dairy buffaloes raised at different altitudes. Food & Function, 12(12), 5440. https://doi.org/10.1039/D0FO03231J
- Qiu, L., Fan, X., Teng, X., Wang, P., & Miao, Y. (2021). Molecular cloning, functional characterization, tissue expression and polymorphism analysis of buffalo PRDX6 gene. Czech Journal of Animal Science, 66(11), 450–458. https://doi.org/10.17221/42/2021-CJAS
- Raikos, V., & Dassios, T. (2014). Health-promoting properties of bioactive peptides derived from milk proteins in infant food: A review. Dairy Science and Technology, 94(2), 91–101. https://doi.org/10.1007/s13594-013-0152-3
- Rehman, S., Feng, T., Wu, S., Luo, X., Lei, A., Luobu, B., Hassan, F., & Liu, Q. (2021). Comparative genomics, evolutionary and gene regulatory regions analysis of casein gene family in Bubalus bubalis. Frontiers in Genetics, 12, 662609. https://doi.org/10.3389/fgene.2021.662609
- Robenek, H., Hofnagel, O., Buers, I., Lorkowski, S., Schnoor, M., Robenek, M. J., Heid, H., Troyer, D., & Severs, N. J. (2006). Butyrophilin controls milk fat globule secretion. Proceedings of the National Academy of Sciences, 103(27), 10385–10390. https://doi.org/10.1073/pnas.0600795103
- Salzano, A., Manganiello, G., Neglia, G., Vinale, F., De Nicola, D., D’Occhio, M., & Campanile, G. (2020). A preliminary study on metabolome profiles of buffalo milk and corresponding mozzarella cheese: Safeguarding the authenticity and traceability of protected status buffalo dairy products. Molecules, 25(2), 304. https://doi.org/10.3390/molecules25020304
- Sarwono, K. A., Kondo, M., Ban-Tokuda, T., Jayanegara, A., & Matsui, H. (2019). Effects of phloroglucinol on in vitro methanogenesis, rumen fermentation, and microbial population density. Tropical Animal Science Journal, 42(2), 121–127. https://doi.org/10.5398/tasj.2019.42.2.121
- Shi, W., Yuan, X., Cui, K., Li, H., Fu, P., Rehman, S. U., Shi, D., Liu, Q., & Li, Z. (2021). LC-MS/MS based metabolomics reveal candidate biomarkers and metabolic changes in different buffalo species. Animals, 11(2), 560. https://doi.org/10.3390/ani11020560
- Smith, N. W., Fletcher, A. J., Hill, J. P., & McNabb, W. C. (2022). Modeling the contribution of milk to global nutrition. Frontiers in Nutrition, 8, 716100. https://doi.org/10.3389/fnut.2021.716100
- Smith, C. A., Want, E. J., O’Maille, G., Abagyan, R., & Siuzdak, G. (2006). XCMS: Processing mass spectrometry data for metabolite profiling using nonlinear peak alignment, matching, and identification. Analytical Chemistry, 78(3), 779–787. https://doi.org/10.1021/ac051437y
- Smolenski, G. A., Broadhurst, M. K., Stelwagen, K., Haigh, B. J., & Wheeler, T. T. (2014). Host defence related responses in bovine milk during an experimentally induced Streptococcus uberis infection. Proteome Science, 12(1), 19. https://doi.org/10.1186/1477-5956-12-19
- Stobiecka, M., Król, J., & Brodziak, A. (2022). Antioxidant activity of milk and dairy products. Animals, 12(3), 245. https://doi.org/10.3390/ani12030245
- Stocco, G., Pazzola, M., Dettori, M. L., Paschino, P., Bittante, G., & Vacca, G. M. (2018). Effect of composition on coagulation, curd firming, and syneresis of goat milk. Journal of Dairy Science, 101(11), 9693–9702. https://doi.org/10.3168/jds.2018-15027
- Sun, Q., Lv, J. P., Liu, L., Zhang, S. W., Liang, X., & Lu, J. (2014). Comparison of milk samples collected from some buffalo breeds and crossbreeds in China. Dairy Science and Technology, 94(4), 387–395. https://doi.org/10.1007/s13594-013-0159-9
- Sun, H. Z., Shi, K., Wu, X. H., Xue, M. Y., Wei, Z. H., Liu, J. X., & Liu, H. Y. (2017). Lactation-related metabolic mechanism investigated based on mammary gland metabolomics and 4 biofluids’ metabolomics relationships in dairy cows. BMC Genomics, 18(1), 936. https://doi.org/10.1186/s12864-017-4314-1
- Szklarczyk, D., Gable, A. L., Nastou, K. C., Lyon, D., Kirsch, R., Pyysalo, S., Doncheva, N. T., Legeay, M., Fang, T., Bork, P., Jensen, L. J., & von Mering, C. (2021). The STRING database in 2021: Customizable protein-protein networks, and functional characterization of user-uploaded gene/measurement sets. Nucleic Acids Research, 49(18), 10800. https://doi.org/10.1093/nar/gkab835
- Tian, H., Zheng, N., Wang, W., Cheng, J., Li, S., Zhang, Y., & Wang, J. (2016). Integrated metabolomics study of the milk of heat-stressed lactating dairy cows. Science Report, 6(1), 24208. https://doi.org/10.1038/srep24208
- Trobbiani, S., Stockham, P., & Scott, T. (2017). Increasing the linear dynamic range in LC-MS: Is it valid to use a less abundant isotopologue? Drug Testing and Analysis, 9(10), 1630–1636. https://doi.org/10.1002/dta.2175
- Tsuji, T., Febriany, D. S., Widiastuti, I., & Yazid, M. (2022). Uses of domestic water buffalo milk in South Sumatra, Indonesia. IOP Conference Series: Earth and Environmental Science, 995, 012018. https://doi.org/10.1088/1755-1315/995/1/012018
- Tvrdý, V., Hrubša, M., Jirkovský, E., Biedermann, D., Kutý, M., Valentová, K., Kren, V., & Mladenka, P. (2021). Silymarin dehydroflavonolignans chelate zinc and partially inhibit alcohol dehydrogenase. Nutrients, 13(12), 4238. https://doi.org/10.3390/nu13124238
- van Heerden, B. (2021). Lacto data: A milk SA publication compiled by the milk producers’ organization. Retrieved December 22, 2021, from https://www.mpo.co.za/wp-content/uploads/2021/05/LACTO-DATA-MAY-21.pdf
- Vargas-Ramella, M., Pateiro, M., Maggiolino, A., Faccia, M., Franco, D., De Palo, P., & Lorenzo, J. M. (2021). Buffalo milk as a source of probiotic functional products. Microorganisms [Internet], 9(11), 2303. https://doi.org/10.3390/microorganisms9112303
- Verduci, E., D’Elios, S., Cerrato, L., Comberiati, P., Calvani, M., Palazzo, S., Martelli, A., Landi, M., Trikamjee, T., & Peroni, D. G. (2019). Cow’s milk substitutes for children: Nutritional aspects of milk from different mammalian species, special formula and plant-based beverages. Nutrients, 11(8), 1739. https://doi.org/10.3390/nu11081739
- Villaseñor, A., Garcia-Perez, I., Garcia, A., Posma, J. M., Fernández-López, M., Nicholas, A. J., Modi, N., Holmes, E., & Barbas, C. (2014). Breast milk metabolome characterization in a single-phase extraction, multiplatform analytical approach. Analytical Chemistry, 86(16), 8245–8252. https://doi.org/10.1021/ac501853d
- Vordenbaumen, S., Braukmann, A., Altendorfer, I., Bleck, E., Jose, J., & Schneider, M. (2013). Human casein alpha s1 (CSN1S1) skews in vitro differentiation of monocytes towards macrophages. BMC Immunology, 14(1), 46. https://doi.org/10.1186/1471-2172-14-46
- Vordenbaumen, S., Saenger, T., Braukmann, A., Tahan, T., Bleck, E., Jose, J., & Schneider, M. (2016). Human casein alpha s1 induces proinflammatory cytokine expression in monocytic cells by TLR4 signaling. Molecular Nutrition & Food Research, 60(5), 1079–1089. https://doi.org/10.1002/mnfr.201500792
- Wada, Y., & Lonnerdal, B. (2014). Bioactive peptides derived from human milk proteins — Mechanisms of action. Journal of Nutritional Biochemistry, 25(5), 503–514. https://doi.org/10.1016/j.jnutbio.2013.10.012
- Walter, L., Narayana, V. K., Fry, R., Logan, A., Tull, D., & Leury, B. (2020). Milk fat globule size development in the mammary epithelial cell: A potential role for ether phosphatidylethanolamine. Science Report, 10(1), 12299. https://doi.org/10.1038/s41598-020-69036-5
- Xu, T., Chen, J., Yang, K., Qiao, W., Zhao, J., & Chen, L. (2022). Quantitative determination of whey protein to casein ratio in infant formula milk powder. Frontiers in Chemistry, 10, 872251. https://doi.org/10.3389/fchem.2022.872251
- Yang, M., Cong, M., Peng, X., Wu, J., Wu, R., Liu, B., Ye, W., & Yue, X. (2016). Quantitative proteomics analysis of milk fat globule membrane (MFGM) proteins in human and bovine colostrum and mature milk samples through iTRAQ labeling. Food & Function, 7(5), 2438–2450. https://doi.org/10.1039/C6FO00083E
- Yang, X. L., Zeng, Q., Qin, J., & Yang, C. (2007). Dairy buffalo breeding in countryside of China. Italian Journal of Animal Science, 6(sup2), 25–29. https://doi.org/10.4081/ijas.2007.s2.25
- Ye, T., Shaukat, A., Yang, L., Chen, C., Zhou, Y., & Yang, L. (2022). Evolutionary and association analysis of buffalo FABP family genes reveal their potential role in milk performance. Genes, 13(4), 600. https://doi.org/10.3390/genes13040600
- Yuan, X., Shi, W., Jiang, J., Li, Z., Fu, P., Yang, C., Rehman, S. U., Pauciullo, A., Liu, Q., & Shi, D. (2022). Comparative metabolomics analysis of milk components between Italian mediterranean buffaloes and Chinese holstein cows based on LC-MS/MS technology. PLoS ONE, 17(1), e0262878. https://doi.org/10.1371/journal.pone.0262878
- Zhang, Y., Fan, X., Zhou, F., Li, W., Ouyang, Y., & Miao, Y. (2021b). Polymorphisms of the CSN1S1 gene and its protein variants in river and swamp buffalo (Bubalus bubalis). Pakistan Journal of Zoology, 53(4), 1233–1242. https://doi.org/10.17582/journal.pjz/20191202121250
- Zhang, X., Jiang, B., Ji, C., Li, H., Yang, L., Jiang, G., Wang, Y., Liu, G., Liu, G., Min, L., & Zhao, F. (2021a). Quantitative label-free proteomics analysis of milk fat globule membrane in donkey and human milk. Frontiers in Nutrition, 8, 670099. https://doi.org/10.3389/fnut.2021.670099
- Zhou, L., Tang, Q., Iqbal, M. W., Xia, Z., Huang, F., Li, L., Liang, M., Lin, B., Qin, G., & Zou, C. (2018). A comparison of milk protein, fat, lactose, total solids and amino acid profiles of three different buffalo breeds in Guangxi, China. Italian Journal of Animal Science, 17(4), 873–878. https://doi.org/10.1080/1828051X.2018.1443288
- Zhou, F., Zhang, Y., Teng, X., & Miao, Y. (2020). Identification, molecular characteristics, and tissue differential expression of DGAT2 full-CDS cDNA sequence in Binglangjiang buffalo (Bubalus bubalis). Archives Animal Breeding, 63(1), 81–90. https://doi.org/10.5194/aab-63-81-2020