ABSTRACT
Phyllanthus emblica L. (P. emblica L.) is a widely consumed healthy fruit known for its taste and benefits. This study used association rule mining to demonstrate its anticancer properties, analyzing its 65 chemical components, 1767 related targets, and 4282 efficacy items. Myricetin was identified as a key component targeting CA2, which is involved in cancer pathways. The research suggested P. emblica L.’s potential against non-small cell lung cancer and esophageal cancer, which was supported by experiments showing significant growth inhibition in related cancer cells. This approach offers a more targeted and convincing understanding of P. emblica L.’s effects, improving upon traditional food research methods.
1. Introduction
Phyllanthus emblica L. (P. emblica L.), also known as Yuganzi in Chinese and Indian Gooseberry or Amla in English, is a fruit widely consumed in subtropical areas belonging to the family Euphorbiaceae and order Geraniales (Gantait et al., Citation2021; Huang et al., Citation2021). It not only possesses high edible value but also provides impressive medicinal value, and is a Chinese medicinal homologous food. Modern phytochemical and bioefficacy studies have revealed that P. emblica L. possesses antioxidant activity, anti-Alzheimer’s disease activity (Gan et al., Citation2022), prevention of skin aging (Chaikul et al., Citation2021), relief of functional dyspepsia (Li et al., Citation2022), protection of the nervous system (Sarmah et al., Citation2022), hepatoprotective effects on nonalcoholic steatohepatitis (Tung et al., Citation2018) and control of diabetic complications (Huang et al., Citation2021). Due to its multiple benefits, refreshing taste and delightful aroma, P. emblica L. is considered one of the most popular natural and nutritional health foods. Unfortunately, research on the efficacy of P. emblica L. is rather blind and limited, and some useful values of efficacy are ignored, so we started with big data to explore more of its potential in a deep and multidimensional way.
Association rule mining is an important topic in data mining and has been widely studied in industry in recent years. It is a rule-based machine learning algorithm that discovers the relationship between items in a data set by exploring the items that precede it and the items that follow it (Giulia et al., Citation2022). The aim is to use some indexes to distinguish the strong rules existing in the database and the association links hidden in the big data set. The association rule was initially applied mainly to retailing in economics, where a market basket analysis sales strategy was proposed (Fagerlind et al., Citation2022). Association rule mining has also been widely used in education (Shi et al., Citation2022), hydroelectric engineering (Chen et al., Citation2022), finance (Hsieh et al., Citation2014), transportation (Xu et al., Citation2018), medicine (Vougas et al., Citation2019), banking (Birjandi & Khasteh, Citation2021) and corporate governance (Wang, Citation2022) to broaden their application prospects and drive innovation, but there are few applications and studies in the field of food. In this work, we innovatively introduce association rule mining into the analysis and confirmation of the efficacy of P. emblica L. Starting from the composition, the target is to act as an intermediate transition to theoretically reveal the possible efficacy of P. emblica L.After the identification of the compositions of P. emblica L., the targets and efficacy corresponding to the compositions were obtained by mining databases and platforms. The compositions, targets and efficacy were analyzed for their respective internal interactions as well as their associations, and their contributions were determined by the centrality values of each parameter. The data set for each item was scanned multidimensionally during the analysis, and the candidate set was used to generate frequent sets to push out the dominant items as a way to identify better possibilities for the effect of P. emblica L.
Based on the theoretical basis of association rule mining, this study explores the efficacy possibilities of P. emblica L. in a multidimensional manner by developing vertically from composition to target to efficacy, as well as exploring the internal association of each item horizontally. Finally, its anticancer activity was experimentally verified. This not only provides new clues for the development of anticancer foods but also opens up new ideas for the research of functional foods.
2. Materials and methods
2.1. Retrieval and identification of P. emblica L. Composition
The compositions of P. emblica L. were obtained statistically from our pre-experiments (Zheng et al., Citation2013), the literature, the Traditional Chinese Medicine Systems Pharmacology database (TCMSP, http://sm.nwsuaf.edu.cn/lsp/tcmsp.php) (Ru et al., Citation2014), Bioinformatics Analysis Tool for the Molecular Mechanism of Traditional Chinese Medicine (BATMAN, http://bionet.ncpsb.org/batman-tcm) (Liu et al., Citation2016) and the Traditional Chinese Medicine Integrated Database (TCMID, http://www.megabionet.org/tcmid/) (Xue et al., Citation2013). We searched as much as possible to list more compositions of P. emblica L. and verify their presence.
2.2. Retrieval and analysis of P. emblica L. related targets
The Simplified Molecular Input Line Entry System (SMILES) format of the P. emblica L. composition was obtained from PubChem (https://pubchem.ncbi.nlm.nih.gov/) (Kim et al., Citation2021) and then imported into the SwissTargetPrediction database (http://www.swisstargetprediction. ch/) (Gfeller et al., Citation2014), selecting “probability” > 0 to obtain the potentially valid targets of the compositions.
The Search Tool for the Retrieval of Interacting Genes (STRING, https://string-db.org/) is an online tool for evaluating potential interactions between targets (Von Mering et al., Citation2003). Based on the String database, target‒target interaction analysis was carried out for the species range “Homo sapiens”, and the minimum needed interaction score was set to the highest confidence (0.900). Remove the free node, and the rest of the settings can be left at default settings.
2.3. Retrieval and analysis of P. emblica L. related efficacy
P. emblica L. deduplication targets were searched in the Comparative Toxicogenomics Database (CTD, http://ctdbase.org/) (Mattingly et al., Citation2006), GeneCards (https://www.genecards.org) (Safran et al., Citation2010), Online Mendelian Inheritance in Man (OMIM, http://omim.org) (Amberger et al., Citation2015) and DisGeNET databases (http://www.disgenet.org) (Piñero et al., Citation2015) to predict their relevant efficacy. Targets were analyzed in the Metascape (http://metascape.org/) database for Gene Ontology (GO) and Kyoto Encyclopedia of Genes and Genomes (KEGG) enrichment (Zhou et al., Citation2019). The cBioPortal online platform (http://www.cbioportal.org) was used to select cancer cell lines to detect all target alterations (Gao et al., Citation2013).
2.4. Validation of the anticancer efficacy of P. emblica L.
Preparation of P. emblica L. extract: Fresh P. emblica L. was washed and dried to a moisture content of less than 3%, then crushed and sieved through a 60-mesh sieve to obtain P. emblica L. pulp powder. Then, the powder was mixed with 60% ethanol or water at a ratio of 1:8 (w/v), and the mixture was continuously sonicated for 1 h. The supernatant was collected after centrifugation at 5000 rpm for 15 min, concentrated and adjusted to a concentration of 1:10 (v/v) and stored for use.
Cell Lines and Cell Culture: A549 non-small lung cancer cells and ECA-109 esophageal cancer cells were purchased from American Type Culture Collection (ATCC, Manassas, VA, U.S.A.). They were cultured in RPMI-1640 medium (Gibco, Carlsbad, CA, U.S.A.) supplemented with 10% fetal bovine serum and 1% antibiotics (50 units/ml penicillin and 50 units/ml streptomycin) at 37°C in a humidified atmosphere of 5% CO2.
MTT assay for the inhibition rate of cell proliferation: An MTT assay was performed to assess the viability of A549 and ECA-109 cells following treatment with P. emblica L. ethanol and water extracts. A single cell suspension (1 × 105 cells/mL) was inoculated on 96-well plates (100 μL per well), and the culture medium was used as a blank control and incubated for 24 h at 37°C with 5% CO2. Cells were treated with P. emblica L. ethanol and water extracts at final concentrations of 16 μL/mL, 12 μL/mL, 8 μL/mL, 4 μL/mL and 2 μL/mL. Then, 20 μL of MTT (5 mg/mL in PBS) reagent was added to each well and incubated for 4 h in the dark, and the formazan crystals were dissolved in 200 mL of dimethyl sulfoxide (DMSO). The optical density (OD) value of each well was measured at 492 nm (OD492) to monitor the effects on cell proliferation. The tests were repeated in triplicate. The inhibitory rate of cell growth was calculated by the following formula: inhibition rate (%) = (ODcontrol − ODtreated)/ODcontrol × 100%. The 50% growth inhibitory concentrations of extracts (IC50) values were calculated by nonlinear regression analysis (Dai et al., Citation2020).
2.5. Data analysis
Conventional statistical analysis: Data location, counting, duplicate checking and distribution were statistically analyzed with Microsoft Excel (Microsoft Corporation, Redmond, WA, U.S.A.) and Statistical Package for Social Sciences version 17 (SPSS; IBM Inc., Armonk, New York).
Association mining analysis: A composition-target, target-efficiency interaction network was established by Cytoscape 3.9.1 (https://cytoscape.org/) (Shannon et al., Citation2003). The frequent itemset was generated by the cytoHubba plugin and Degree algorithms for filtering. The node size and color shade can reflect the contribution of each item in the network (Wu et al., Citation2022).
3. Results
3.1. Composition analysis of P. emblica L.
Combining our previous research results, databases and literature, as many compositions of P. emblica L. as possible were collected and further identified. The statistical results identified a total of 65 compounds, including 20 acids, 17 flavonoids, 11 esters, 6 sugars, 5 phenols, 4 alcohols and 2 aldehydes ().
3.2. Retrieval and analysis of P. emblica L. composition-related targets
A search for potentially relevant targets of P. emblica L. compositions in various platforms and databases revealed a total of 49 compositions with corresponding targets (Supplemental Table S1). The three compositions of myricetin, kaempferol and scutellarein corresponded to the largest number of targets, all of which were 100. Myricetin corresponded to MAPT, KDM4E, GPR35, etc., kaempferol corresponded to NOX4, AKR1B1, XDH, etc., and scutellarein corresponded to PTPRS, AMY1A and GRK6, etc. The following compositions with a high number of target associations were naringenin and aristolochic acid with 97 (CYP19A1, CA7, ABCC1, etc.) and 81 (CDK2, EGFR, SORT1, etc.), respectively. Different compositions also share the same target, so there is a common crossover between the targets. CA2 occurs up to 24 times, with common crossover compositions such as gallic acid, ellagic acid and methyl gallate. The next most highly expressed targets were CA12 and CA4, with 23 species of protocatechuic acid, kaempferol and eriodictyol and 22 species of naringenin, trans-cinnamic acid and hydroquinone as intersecting compositions ().
Figure 2. Diagram of the target analysis related to the composition of P. emblica L. (a) correlation plot of compositions and targets. Compositions with higher contributions are indicated in red, and the bar chart below corresponds to their degree values. Targets with higher contributions are shown in blue, and the right bars correspond to their degree values. (b) interaction diagram between targets. Targets with strong interactions are marked.
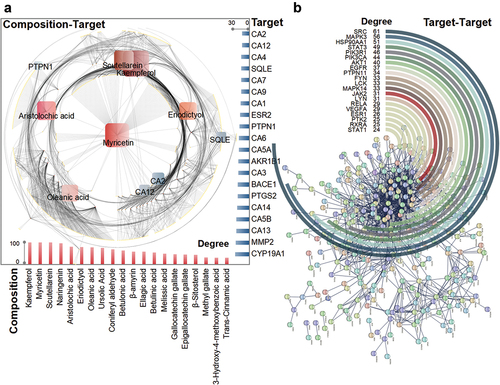
In addition to the number of target occurrences, the interactions between targets are also noteworthy. The targets of all compositions were pooled to obtain 1767 targets, and the number of targets was 431 after removing the overlap. Then, the interactions were analyzed without duplicate targets, the number of interacting targets was 249, and the number of edges was 1208. Among the interacting targets, SRC performed the best, interacting with 61 targets, including STAT3, STAT1, and SYK. This was followed by MAPK3 and HSP90AA1, which interacted with 56 (NFKBIA, NR3C1, MKNK2, etc.) and 51 (MMP2, IL2, NR3C1, etc.) targets, respectively ().
3.3. Retrieval and analysis of P. emblica L. target-related efficacy
The deduplicated targets of P. emblica L. were used as antecedents to further search for efficacy associations. A total of 374 targets were found to be associated with 4282 efficacies (Supplemental Table S2). The highest contributing target was TNF, with 190 efficacies searched for breast neoplasms, anemia, hyperalgesia, etc. The next two targets with higher contributions were PTGS2 and VEGFA, with 108 (stroke, stomach ulcer, papilloma, etc.) and 74 (hemorrhage, neoplasms, psoriasis, etc.) associated efficacy searches, respectively. The most frequent possible efficacy was prostatic neoplasms, with 76 associated targets, such as FGF2, CYP19A1 and HSD17B1. This was followed by liver cirrhosis and breast neoplasms, with 73 (TGFBR1, REN, NFE2L2, etc.) and 69 (CYP1A1, STAT3, TUBB3, etc.) relevant targets, respectively ().
Figure 3. Diagram of the efficacy analysis related to the target of P. emblica L. (a) correlation plots of targets and efficacy. Targets with higher contributions are indicated in red, and the bar chart below corresponds to their degree values. Efficacy with higher contribution is shown in blue, and the right bars correspond to their degree values. (b) KEGG pathway enrichment plot of the target. (b) GO enrichment plot of the target. The three parts from left to right are shown as CC, BP and MF. Both GO and KEGG were enriched in the top 20.
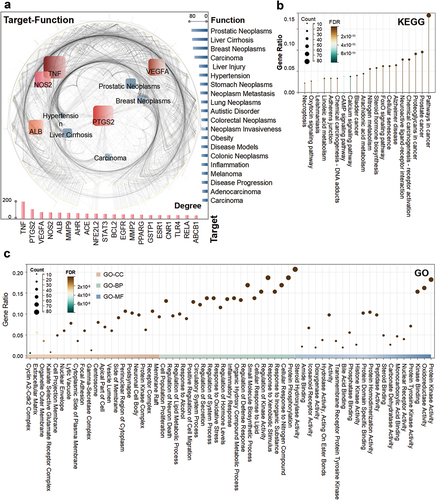
Meanwhile, GO and KEGG functional enrichment analyses were performed with deduplicated targets. GO includes three parts: cellular component (CC), biological process (BP) and molecular function (MF). In order of gene ratio, GO-CC enriched items are membrane raft, receptor complex, protein kinase complex, etc. The GO-BP enrichment items were protein phosphorylation, cellular response to nitrogen compound, response to inorganic substance, etc., and the GO-MF enrichment items were protein kinase activity, oxidoreductase activity and kinase substance, etc. (). The pathways with superior KEGG enrichment were pathways in cancer, prostate cancer, proteoglycans in cancer, etc. ().
3.4. Multidimensional mining of the relationship between the efficacy of P. emblica L.
Since a large number of efficacy items were retrieved from the targets, they were classified and analyzed, and categories including more than 20 items were selected for presentation. The efficacy associated with P. emblica L. focuses mainly on cancer, encapsulating items with 114 types of prostatic neoplasms, breast neoplasms, carcinoma, etc. The second is cardiovascular disease, including 62 items (thrombosis, hyperemia, stroke, etc.). This was followed by 53 items related to digestive system disease (). A single efficacy item was clustered in different categories, such as esophageal cancer, which was grouped into both cancer and digestive system disease. Among them, the intersection of cancer and digestive system disease displayed the most, with 25 efficacies, such as colonic neoplasms, stomach neoplasms and liver neoplasms ().
Figure 4. Multidimensional mining analysis diagram of P. emblica L. efficacy. (a) category clustering of efficacy. Left: number of efficacies encapsulated in different categories. Right: visual network of different efficacy-focused categories. (b) multiple venn diagram of cancer and other disease categories. The results are shown separately for clarity. (c) the alteration frequency of targets in different cancer cell lines.
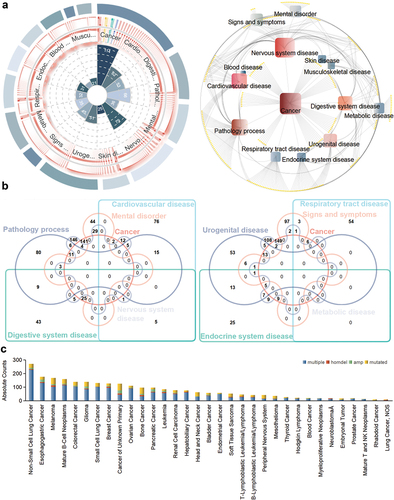
Analysis of specific genetic alterations in cancer cell lines through cBioPortal in three databases: Cancer Cell Line Encyclopedia (CCLE, Broad 2019, 1739 samples), Cancer Cell Line Encyclopedia (CCLE, Novartis/Broad, Nature 2012, 1020 samples) and National Cancer Institute (NCI-60, Cancer Res 2012, 67 samples) for P. emblica L. targets. The results showed that the highest values of P. emblica L.-related target alterations were found in non-small cell lung cancer cell lines and esophageal cancer cell lines. P. emblica L.-related targets were substantially altered in 276 cases of non-small cell lung cancer cell lines and in 178 cases of esophageal cancer cell lines ().
3.5. Validation of the anticancer efficacy of P. emblica L.
Non-small lung cancer cells A549 and esophageal cancer cells ECA-109 were selected to validate the efficacy of P. emblica L. After ethanol and water extract treatments, the proliferation inhibition rate of both cells was positively correlated with the concentration of P. emblica L. extract, i.e. cell growth was significantly inhibited by varying concentrations of P. emblica L. In the presence of ethanol extract, with increasing concentration, the cell morphology of A549 was gradually crinkled and deformed from the normal tightly distributed stereotaxic shuttle shape, accompanied by an increase in the cell gap, and finally the cells were completely vacuolated and necrotic. The water extract also caused A549 cells to change from a tight stereoscopic shuttle shape to an obvious rounding, increased gap, blurred cell membrane boundary, and finally vacuolation with increasing concentration. The morphological variation of ECA-109 cells was similar to that of A549 cells by the action of P. emblica L. extract, and it is noteworthy that the cell membrane ruptured with 16 μL/mL ethanol extract. The IC50 values for A549 cells were 13.13 (ethanol extract) and 7.66 (water extract), and those for ECA-109 cells were 5.56 (ethanol extract) and 4.13 (water extract) ().
4. Discussion
With the increasing pursuit of health, consuming healthy food has gradually become a new way of life. P. emblica L. is an important dual-use resource with wide applications in medicine and health care and possesses promising development prospects. Traditionally, it is believed that P. emblica L. exhibits potent antioxidant and anti-inflammatory activities (Li et al., Citation2020). However, preliminary studies on the efficacy of P. emblica L. are limited and blinded, and its efficacy and scope of application are unclear. Big data platforms can systematically and comprehensively guide and support more possibilities. This study starts from the principle of association rule mining, from composition to target to efficacy, and layer-by-layer progressive mining purposefully elucidates the efficacy of P. emblica L. Association rule mining is an important technique in the field of data mining, which is currently a hot research topic in various fields. This study breaks through the barriers of traditional thinking in efficacy research and introduces this theory in food efficacy to achieve interdisciplinary integration.
The material basis of the efficacy study is the composition, so the idea of this study is to start from the composition of P. emblica L. The composition has been extensively detected and analyzed by different methods in the previous period, and we performed systematic statistics and identification. Sixty-five compounds were found in P. emblica L. The target is the bridge between the composition and the efficacy, and the composition acts on the target to show its corresponding efficacy. Target mining is a very important part of the whole analysis process, and 49 compositions of P. emblica L. were revealed to carry corresponding targets. The compositions with the most retrieved targets were myricetin, kaempferol and scutellarein, and the target with the highest number of occurrences was CA2. Target interaction analysis showed that the targets with excellent interaction performance were SRC, MAPK3 and HSP90AA1. Further multidimensional exploration of the efficacy of P. emblica L. through targets, functional enrichment and category clustering, all of which point to cancer. Target expression showed that it was actively expressed in non-small lung cancer cells and esophageal cancer cells. The anticancer effects of P. emblica L. have been reported in skin cancer (Kunchana et al., Citation2021), lung cancer (Wang et al., Citation2017), and colon cancer (Guo et al., Citation2013), but little research has been conducted in non-small lung cancer cells and esophageal cancer cells. Myricetin is the main composition of P. emblica L. (Wu et al., Citation2022), and its targets are the most numerous. Recent studies have identified myricetin and its derivatives as new anticancer weapons against non-small lung cancer cells (Li et al., Citation2022; Zhou et al., Citation2023), as well as its apoptosis-promoting effect on esophageal cancer cells (Zang et al., Citation2014). Therefore, this composition deserves to be studied further in the near future. It should be noted here that the compositions, targets and efficacy are continuously being updated, shared and integrated, and the whole analysis process needs to be further explored accordingly. Hopefully, continuous updates will provide more possibilities for P. emblica L. efficacy.
Cancer mortality is a major public health problem worldwide, and its prevention and treatment cannot be ignored. The unsatisfactory therapeutic effects of cancer, beyond the treatments developed to date, have not been addressed by expanding the toolbox (Liu et al., Citation2023). In view of the increasing incidence of cancer and people’s pursuit of a healthy lifestyle, the development of anticancer foods has become a hot spot of research today. For example, Cheonggukjang (fermented soybean) possesses anti-colon cancer properties (Lim et al., Citation2023). Matcha can affect the proliferation and viability of breast cancer cells (Sokary et al., Citation2023), and (-)-oleocanthal preferentially induces the death of tumor hematopoietic cells (Pastorio et al., Citation2022). Foods reported in the dietary treatment of non-small lung cancer include Camellia nitidissima Chi (Wang et al., Citation2022), cranberry (Yu et al., Citation2022) and Korean ginseng (Ginseng Rh2+) (Lev-Ari et al., Citation2021). Foods that fight esophageal cancer include black raspberries and strawberries (Shi & Chen, Citation2022). Compared with other cancers, there are few anticancer food products for non-small cell lung cancer and esophageal cancer, which shows that the future research and application prospects of P. emblica L. are very promising.
In this work, based on association rule mining and multidimensional analysis, the efficacy of P. emblica L. was localized after target mining through the statistics and identification of the compositions, and its inhibitory effect on non-small lung cancer cells and esophageal cancer cells was finally demonstrated. Association rule mining on big data is rarely applied in the food field, and we use this principle to overcome the traditional limitations and blindness, which lays the foundation for establishing a systematic approach to scientific research.
5. Conclusion
Association rule mining and multidimensional analysis is an approach that combines data analysis and machine learning to study the relationship between the material basis and biological effects. Compositions, targets and efficacy related to P. emblica L. were obtained by mining in a big data environment. The preferred compositions, targets and efficacy of P. emblica L. were obtained by multidimensional analysis of the associations within and among the above three units, and this information helped us to develop new research ideas. By further focusing on functional enrichment and cell line target expression, it was discovered that P. emblica L. may be associated with non-small cell lung cancer and esophageal cancer. Two cell lines, the non-small lung cancer cell A549 and esophageal cancer cell ECA-109, were selected to confirm this speculation. This not only indicates that association rule mining combined with multidimensional analysis can provide theoretical support for the derivation of P. emblica L. efficacy but also points out that P. emblica L., as a natural resource, deserves attention in the prevention and adjuvant treatment of cancer.
Acronyms
P. emblica L. | = | Phyllanthus emblica L. |
TCMSP | = | Traditional Chinese Medicine Systems Pharmacology database |
BATMAN | = | Bioinformatics Analysis Tool for the Molecular Mechanism of Traditional Chinese Medicine |
TCMID | = | Traditional Chinese Medicine Integrated Database |
SMILES | = | Simplified Molecular Input Line Entry System |
STRING | = | Search Tool for the Retrieval of Interacting Genes |
CTD | = | Comparative Toxicogenomics Database |
OMIM | = | Online Mendelian Inheritance in Man |
GO | = | Gene Ontology |
KEGG | = | Kyoto Encyclopedia of Genes and Genomes |
ATCC | = | American Type Culture Collection |
DMSO | = | Dimethyl Sulfoxide |
OD | = | Optical Density |
IC50 | = | The 50% Growth Inhibitory Concentrations |
SPSS | = | Statistical Package for Social Sciences |
CC | = | Cellular Component |
BP | = | Biological Process |
MF | = | Molecular Function |
CCLE | = | Cancer Cell Line Encyclopedia |
NCI | = | National Cancer Institute |
Author contributions
Conceptualization, Yaqun Liu and Yuzhong Zheng; methodology, Jiaoyun Jiang and Zhenxia Zhang; software, Yaqun Liu and Qionglu Huang; validation, Yaqun Liu, Qionglu Huang, Mouquan Liu and Zhenxia Zhang; formal analysis, Yaqun Liu and Yuzhong Zheng; investigation, Yongping Huang, Xianghui Zou, Ying Nie and Lianghui Chen; data curation, Yaqun Liu, Jiaoyun Jiang and Qionglu Huang; writing – original draft preparation, Yaqun Liu; writing – review and editing, Yuzhong Zheng; visualization, Yaqun Liu and Qionglu Huang; supervision, Yuzhong Zheng. All authors have read and agreed to the published version of the manuscript.
Supplemental Material
Download MS Word (518 KB)Supplemental Material
Download MS Word (21 KB)Acknowledgments
We are very grateful for the support of the Science and Technology Specialists Program of Guangdong Province: Technical popularization of valuable fruits and ecological upgrading of aquaculture.
Disclosure statement
No potential conflict of interest was reported by the author(s).
Data availability statement
All data generated or analysed during this study are included in this published article. The datasets used and analyzed in this study can be found in Supplementary Table.
Supplementary material
Supplemental data for this article can be accessed online at https://doi.org/10.1080/19476337.2023.2293920.
Additional information
Funding
References
- Amberger, J. S., Bocchini, C. A., Schiettecatte, F., Scott, A. F., & Hamosh, A. (2015). Omim.Org: Online mendelian inheritance in man (OMIM®), an online catalog of human genes and genetic disorders. Nucleic Acids Research, 43(Database issue), D789–D798. https://doi.org/10.1093/nar/gku1205
- Birjandi, S. M., & Khasteh, S. H. (2021). A survey on data mining techniques used in medicine. Journal of Diabetes & Metabolic Disorders, 20(2), 2055–9. https://doi.org/10.1007/s40200-021-00884-2
- Chaikul, P., Kanlayavattanakul, M., Somkumnerd, J., & Lourith, N. (2021). Phyllanthus emblica L. (amla) branch: A safe and effective ingredient against skin aging. Journal of Traditional and Complementary Medicine, 11(5), 390–399. https://doi.org/10.1016/j.jtcme.2021.02.004
- Chen, S., Xi, J., Chen, Y., & Zhao, J. (2022). Association mining of near misses in hydropower engineering construction based on convolutional neural network text classification. Computational Intelligence and Neuroscience, 4851615. https://doi.org/10.1155/2022/4851615
- Dai, L. P., Li, X. F., Feng, Q. M., Zhang, L.-X., Liu, Q.-Y., Xu, E.-P., Wu, H., & Wang, Z.-M. (2020). Isolation and identification of two pairs of cytotoxic diterpene tautomers and their tautomerization mechanisms. Scientific Reports, 10(1), 1442. https://doi.org/10.1038/s41598-020-58260-8
- Fagerlind, H., Harvey, L., Humburg, P., Davidsson, J., & Brown, J. (2022). Identifying individual-based injury patterns in multi-trauma road users by using an association rule mining method. Accident Analysis & Prevention, 164, 106479. https://doi.org/10.1016/j.aap.2021.106479
- Gan, J., Zhang, X., Ma, C., Sun, L., Feng, Y., He, Z., & Zhang, H. (2022). Purification of polyphenols from Phyllanthus emblica L. pomace using macroporous resins: Antioxidant activity and potential anti-Alzheimer’s effects. Journal of Food Science, 87(3), 1244–1256. https://doi.org/10.1111/1750-3841.16028
- Gantait, S., Mahanta, M., Bera, S., Verma, S. K. (2021). Advances in biotechnology of emblica officinalis gaertn. syn. Phyllanthus emblica L: A nutraceuticals-rich fruit tree with multifaceted ethnomedicinal uses. Biotechnology, 11(2), 62. https://doi.org/10.1007/s13205-020-02615-5
- Gao, J., Aksoy, B. A., Dogrusoz, U., Dresdner, G., Gross, B., Sumer, S. O., Sun, Y., Jacobsen, A., Sinha, R., Larsson, E., Cerami, E., Sander, C., & Schultz, N. (2013). Integrative analysis of complex cancer genomics and clinical profiles using the cbioportal. Science Signaling, 6(269), 11. https://doi.org/10.1126/scisignal.2004088
- Gfeller, D., Grosdidier, A., Wirth, M., Daina, A., Michielin, O., & Zoete, V. (2014). Swiss target prediction: A web server for target prediction of bioactive small molecules. Nucleic Acids Research, 42(Web Server issue), W32–W38. https://doi.org/10.1093/nar/gku293
- Giulia, A., Anna, S., Antonia, B., Dario, P., & Maurizio, C. (2022). Extending association rule mining to microbiome pattern analysis: Tools and guidelines to support real applications. Frontiers in Bioinformatics, 1, 794547. https://doi.org/10.3389/fbinf.2021.794547
- Guo, X., Ni, J., Liu, X., Xue, J., & Wang, X. (2013). Phyllanthus emblica L. fruit extract induces chromosomal instability and suppresses necrosis in human colon cancer cells. International Journal for Vitamin and Nutrition Research, 83(5), 271–280. https://doi.org/10.1024/0300-9831/a000169
- Hsieh, Y. L., Yang, D. L., & Wu, J. (2014). Effective application of improved profit-mining algorithm for the interday trading model. Scientific World Journal, 2014, 874825. https://doi.org/10.1155/2014/874825
- Huang, H. Z., Qiu, M., Lin, J. Z., Li, M.-Q., Ma, X.-T., Ran, F., Luo, C.-H., Wei, X.-C., Xu, R.-C., Tan, P., Fan, S.-H., Yang, M., Han, L., & Zhang, D.-K. (2021). Potential effect of tropical fruits Phyllanthus emblica L. for the prevention and management of type 2 diabetic complications: A systematic review of recent advances. European Journal of Nutrition, 60(7), 3525–3542. https://doi.org/10.1007/s00394-020-02471-2
- Kim, S., Chen, J., Cheng, T., Gindulyte, A., He, J., He, S., Li, Q., Shoemaker, B. A., Thiessen, P. A., Yu, B., Zaslavsky, L., Zhang, J., & Bolton, E. E. (2021). Pubchem in 2021: New data content and improved web interfaces. Nucleic Acids Research, 49(D1), D1388–D1395. https://doi.org/10.1093/nar/gkaa971
- Kunchana, K., Jarisarapurin, W., Chularojmontri, L., & Wattanapitayakul, S. K. (2021). Potential use of amla (Phyllanthus emblica L.) fruit extract to protect skin keratinocytes from inflammation and apoptosis after UVB irradiation. Antioxidants (Basel), 10(5), 703. https://doi.org/10.3390/antiox10050703
- Lev-Ari, S., Starr, A. N., Vexler, A., Kalich-Philosoph, L., Yoo, H.-S., Kwon, K.-R., Yadgar, M., Bondar, E., Bar-Shai, A., Volovitz, I., & Schwarz, Y. (2021). Rh2-enriched Korean ginseng (Ginseng Rh2+) inhibits tumor growth and development of metastasis of non-small cell lung cancer. Food & Function, 12(17), 8068–8077. https://doi.org/10.1039/d1fo00643f
- Li, M., Zha, G., Chen, R., Chen, X., Sun, Q., & Jiang, H. (2022). Anticancer effects of myricetin derivatives in non-small cell lung cancer in vitro and in vivo. Pharmacology Research & Perspectives, 10(1), e00905. https://doi.org/10.1002/prp2.905
- Li, W., Zhang, X., Chen, R., Li, Y., Miao, J., Liu, G., Lan, Y., Chen, Y., & Cao, Y. (2020). HPLC fingerprint analysis of Phyllanthus emblica ethanol extract and their antioxidant and anti-inflammatory properties. Journal of Ethnopharmacology, 254, 112740. https://doi.org/10.1016/j.jep.2020.112740
- Li, X., Lin, Y., Jiang, Y., Wu, B., & Yu, Y. (2022). Aqueous extract of Phyllanthus emblica L. alleviates functional dyspepsia through regulating gastrointestinal hormones and gut microbiome in vivo. Foods, 11(10), 1491. https://doi.org/10.3390/foods11101491
- Lim, H. J., Park, I. S., Jeong, S. J., Ha, G.-S., Yang, H.-J., Jeong, D.-Y., Kim, S.-Y., & Jung, C.-H. (2023). Effects of cheonggukjang (fermented soybean) on the development of colitis-associated colorectal cancer in mice. Foods, 12(2), 383. https://doi.org/10.3390/foods12020383
- Liu, Z., Guo, F., Wang, Y., Li, C., Zhang, X., Li, H., Diao, L., Gu, J., Wang, W., Li, D., & He, F. (2016). BATMAN-TCM: A bioinformatics analysis tool for molecular mechAnism of traditional chinese medicine. Scientific Reports, 6, 21146. https://doi.org/10.1038/srep21146
- Liu, Z., Shi, M., Ren, Y., Xu, H., Weng, S., Ning, W., Ge, X., Liu, L., Guo, C., Duo, M., Li, L., Li, J., & Han, X. (2023). Recent advances and applications of CRISPR-Cas9 in cancer immunotherapy. Molecular Cancer, 22(1), 35. https://doi.org/10.1186/s12943-023-01738-6
- Mattingly, C. J., Rosenstein, M. C., Colby, G. T., Forrest J. N., Jr., & Boyer, J. L. (2006). The Comparative toxicogenomics database (CTD): A resource for comparative toxicological studies. Journal of Experimental Zoology Part A: Comparative Experimental Biology, 305(9), 689–692. https://doi.org/10.1002/jez.a.307
- Pastorio, C., Torres-Rusillo, S., Ortega-Vidal, J., Jiménez-López, M. C., Iañez, I., Salido, S., Santamaría, M., Altarejos, J., & Molina, I. J. (2022). (−)-Oleocanthal induces death preferentially in tumor hematopoietic cells through caspase dependent and independent mechanisms. Food & Function, 13(21), 11334–11341. https://doi.org/10.1039/d2fo01222g
- Piñero, J., Bravo, À., Queralt-Rosinach, N., Gutiérrez-Sacristán, A., Deu-Pons, J., Centeno, E., García-García, J., Sanz, F., & Furlong, L. I. (2015). Disgenet: A comprehensive platform integrating information on human disease-associated genes and variants. Nucleic Acids Research, 45(D1), D833–D839. https://doi.org/10.1093/nar/gkw943
- Ru, J., Li, P., Wang, J., Zhou, W., Li, B., Huang, C., Li, P., Guo, Z., Tao, W., Yang, Y., Xu, X., Li, Y., Wang, Y., & Yang, L. (2014). TCMSP: A database of systems pharmacology for drug discovery from herbal medicines. Journal of Cheminformatics, 6(1), 13. https://doi.org/10.1186/1758-2946-6-13
- Safran, M., Dalah, I., Alexander, J., Rosen, N., Iny Stein, T., Shmoish, M., Nativ, N., Bahir, I., Doniger, T., Krug, H., Sirota-Madi, A., Olender, T., Golan, Y., Stelzer, G., Harel, A., & Lancet, D. (2010). Genecards version 3: The human gene integrator. Database-Oxford, 2010, baq020. https://doi.org/10.1093/database/baq020
- Sarmah, D., Verma, G., Datta, A., Vadak, N., Chaudhary, A., Kalia, K., & Bhattacharya, P. (2022). Phyllanthus emblica L. regulates BDNF/PI3K pathway to modulate glutathione for mitoprotection and neuroprotection in a rodent model of ischemic stroke. Central Nervous System Agents in Medicinal Chemistry, 22(3), 175–187. https://doi.org/10.2174/1871524922666220607093400
- Shannon, P., Markiel, A., Ozier, O., Baliga N. S., Wang J. T., Ramage, D., Amin, N., Schwikowski, B., & Ideker, T. (2003). Cytoscape: A software environment for integrated models of biomolecular interaction networks. Genome Research, 13(11), 2498–2504. https://doi.org/10.1101/gr.1239303
- Shi, L., Zhu, Q., & Khan, R. (2022). Association rule analysis of influencing factors of literature curriculum interest based on data mining. Computational Intelligence and Neuroscience, 2022, 1–8. https://doi.org/10.1155/2022/6866134
- Shi, N., & Chen, T. (2022). Chemopreventive properties of black raspberries and strawberries in esophageal cancer review. Antioxidants-Basel, 11(9), 1815. https://doi.org/10.3390/antiox11091815
- Sokary, S., Al-Asmakh, M., Zakaria, Z., & Bawadi, H. (2023). The therapeutic potential of matcha tea: A critical review on human and animal studies. Current Research in Food Science, 6, 100396. https://doi.org/10.1016/j.crfs.2022.11.015
- Tung, Y. T., Huang, C. Z., Lin, J. H., Yen, G. C. (2018). Effect of Phyllanthus emblica L. fruit on methionine and choline-deficiency diet-induced nonalcoholic steatohepatitis. Journal of Food & Drug Analysis, 26(4), 1245–1252. https://doi.org/10.1016/j.jfda.2017.12.005
- Von Mering, C., Huynen, M., Jaeggi, D., Schmidt, S., Bork, P., & Snel, B. (2003). String: A database of predicted functional associations between proteins. Nucleic Acids Research, 31(1), 258–261. https://doi.org/10.1093/nar/gkg034
- Vougas, K., Sakellaropoulos, T., Kotsinas, A., Foukas, G. R. P., Ntargaras, A., Koinis, F., Polyzos, A., Myrianthopoulos, V., Zhou, H., Narang, S., Georgoulias, V., Alexopoulos, L., Aifantis, I., Townsend, P. A., Sfikakis, P., Fitzgerald, R., Thanos, D., Bartek, J., Petty, R., … Tsirigos, A. (2019). Machine learning and data mining frameworks for predicting drug response in cancer: An overview and a novel in silico screening process based on association rule mining. Pharmacology & Therapeutics, 203, 107395. https://doi.org/10.1016/j.pharmthera.2019.107395
- Wang, C. C., Yuan, J. R., Wang, C. F., Yang, N., Chen, J., Liu, D., Song, J., Feng, L., Tan, X.-B., & Jia, X.-B. (2017). Anti-inflammatory effects of Phyllanthus emblica L on benzopyrene-induced precancerous lung lesion by regulating the IL-1β/miR-101/Lin28B signaling pathway. Integrative Cancer Therapies, 16(4), 505–515. https://doi.org/10.1177/1534735416659358
- Wang, M. (2022). Comprehensive evaluation of government economic management performance based on multidimensional data mining in fuzzy comprehensive environment. Journal of Environmental and Public Health, 2022, 1–10. https://doi.org/10.1155/2022/4265125
- Wang, Z., Hou, X., Li, M., Ji, R., Li, Z., Wang, Y., Guo, Y., Liu, D., Huang, B., & Du, H. (2022). Active fractions of golden-flowered tea (camellia nitidissima chi) inhibit epidermal growth factor receptor mutated non-small cell lung cancer via multiple pathways and targets in vitro and in vivo. Frontiers in Nutrition, 9, 1014414. https://doi.org/10.3389/fnut.2022.1014414
- Wu, M., Cai, J., Fang, Z., Li, S., Huang, Z., Tang, Z., Luo, Q., & Chen, H. (2022). The composition and anti-aging activities of polyphenol extract from Phyllanthus emblica L. fruit. Nutrients, 14(4), 857. https://doi.org/10.3390/nu14040857
- Wu, Q., Ying, X., Yu, W., Li, H., Wei, W., Lin, X., & Zhang, X. (2022). Identification of ferroptosis-related genes in syncytiotrophoblast-derived extracellular vesicles of preeclampsia. Medicine, 101(44), e31583. https://doi.org/10.1097/MD.0000000000031583
- Xu, C., Bao, J., Wang, C., & Liu, P. (2018). Association rule analysis of factors contributing to extraordinarily severe traffic crashes in China. Journal of Safety Research, 67, 65–75. https://doi.org/10.1016/j.jsr.2018.09.013
- Xue, R., Fang, Z., Zhang, M., Yi, Z., Wen, C., & Shi, T. (2013). TCMID: Traditional Chinese Medicine integrative database for herb molecular mechanism analysis. Nucleic Acids Research, 41(Database issue), D1089–95. https://doi.org/10.1093/nar/gks1100
- Yu, C. P., Tsai, P. L., Li, P. Y., Hsu, P.-W., Lin, S.-P., Lee Chao, P.-D., & Hou, Y.-C. (2022). Cranberry ingestion modulated drug transporters and metabolizing enzymes: Gefitinib used as a probe substrate in rats. Molecules, 27(18), 5772. https://doi.org/10.3390/molecules27185772
- Zang, W., Wang, T., Wang, Y., Li, M., Xuan, X., Ma, Y., Du, Y., Liu, K., Dong, Z., & Zhao, G. (2014). Myricetin exerts anti-proliferative, anti-invasive, and pro-apoptotic effects on esophageal carcinoma EC9706 and KYSE30 cells via RSK2. Tumour Biology: The Journal of the International Society for Oncodevelopmental Biology and Medicine, 35(12), 12583–12592. https://doi.org/10.1007/s13277-014-2579-4
- Zheng, Y. Z., Zhang, Z. X., Dong, T. X., & Zhan, H. Q. (2013). Analysis of HPLC fingerprints and determination of gallic acid and ellagic acid of phyllanthi fructus from Phyllanthus emblica. Chinese Journal of Experimental Traditional Medical Formulae, 19(23), 94–99. https://doi.org/10.11653/syfj2013230094
- Zhou, H., Xu, L., Shi, Y., Gu, S., Wu, N., Liu, F., Huang, Y., Qian, Z., Xue, W., Wang, X., & Chen, F. (2023). A novel myricetin derivative with anti-cancer properties induces cell cycle arrest and apoptosis in A549 cells. Biological & Pharmaceutical Bulletin, 46(1), 42–51. https://doi.org/10.1248/bpb.b22-00483
- Zhou, Y., Zhou, B., Pache, L., Chang, M., Khodabakhshi, A. H., Tanaseichuk, O., Benner, C., & Chanda, S. K. (2019). Metascape provides a biologist-oriented resource for the analysis of systems-level dataset. Nature Communications, 10(1), 1523. https://doi.org/10.1038/s41467-019-09234-6