ABSTRACT
Bile acid metabolism by the gut microbiome exerts both beneficial and harmful effects on host health. Microbial bile salt hydrolases (BSHs), which initiate bile acid metabolism, exhibit both positive and negative effects on host physiology. In this study, 5,790 BSH homologs were collected and classified into seven clusters based on a sequence similarity network. Next, the abundance and distribution of BSH in 380 metagenomes from healthy participants were analyzed. It was observed that different clusters occupied diverse ecological niches in the human microbiome and that the clusters with signal peptides were relatively abundant in the gut. Then, the association between BSH clusters and 12 human diseases was analyzed by comparing the abundances of BSH genes in patients (n = 1,605) and healthy controls (n = 1,540). The analysis identified a significant association between BSH gene abundance and 10 human diseases, including gastrointestinal diseases, obesity, type 2 diabetes, liver diseases, cardiovascular diseases, and neurological diseases. The associations were further validated by separate cohorts with inflammatory bowel diseases and colorectal cancer. These large-scale studies of enzyme sequences combined with metagenomic data provide a reproducible assessment of the association between gut BSHs and human diseases. This information can contribute to future diagnostic and therapeutic applications of BSH-active bacteria for improving human health.
Introduction
Human microbiome studies have shown that microbes play a crucial role in maintaining health. In particular, gut bacteria benefit humans in a number of ways: they metabolize inaccessible components of food, synthesize molecules used in human metabolism, offer protection from pathogens, and regulate the immune system.Citation1 Alterations in the gut microbiome are linked with many human diseases, such as pathophysiological obesity, inflammatory diseases, metabolic syndromes, cancer, and neurodegenerative diseases.Citation2 The causal relationship between dysbiosis and obesity has been established from animal studies.Citation3 Induction and promotion of liver cancer through the regulation of the immune system by dysbiosis has also been reported.Citation4 However, the biochemical mechanisms by which gut microbes influence host physiology remain unknown. Providing a mechanistic understanding of microbial functions and their metabolic capabilities would illustrate the impact of these organisms on human health. This knowledge can be used in the treatment of relevant diseases.
The investigation of the biochemical functions of microbial species and their metabolites represents an exciting opportunity to bridge the knowledge gap between gut bacteria and their effect on host metabolism.Citation1,Citation5 Microbial symbionts derive different metabolites from different sources. Some metabolites are derived from carbohydrate and protein fermentation, including short-chain fatty acids, branched-chain fatty acids, and indoles.Citation6 Dimethylamine and trimethylamine are products of the metabolism of dietary choline by intestinal bacteria.Citation7 Secondary bile acids (BAs) are produced by the bacterial metabolism of primary BAs, which are produced in the liver.Citation8 These molecules are strongly involved in the gut microbiome–host metabolic axis. Among them, secondary BAs have been reported to be associated with obesity, diabetes, colon cancer, polycystic ovary syndrome, liver cancer, and other liver diseases.Citation9–Citation11 The transformation from primary BAs to secondary BAs primarily includes two steps: the hydrolysis of conjugated BAs to free primary BAs and the 7α/β-dehydroxylation of cholic acid and chenodeoxycholic acid, yielding deoxycholic acid and lithocholic acid, respectively. Bacterial bile salt hydrolases (BSHs) are responsible for the hydrolysis of conjugated BAs in the gut.Citation12 BSHs display both positive and negative effects on host health: BSH activity reduces serum cholesterol levels and confers bacterial resistance to BAs; however, it also leads to lipid metabolism disorders.Citation13 As key mediators and gatekeepers of BA transformation, these enzymes have been considered a promising target in the manipulation of gut microbiota to benefit human health.Citation12,Citation14
Whole metagenome shotgun sequencing technologies have provided important information regarding the connection between the gut microbiome and its host. Considering the gatekeeper role of BSHs in secondary BA metabolism and their important relationship with human health, we first collected the experimentally characterized BSH sequences and performed protein sequence similarity network (SSN) analysis to cluster the BSH homologs. Then, we analyzed the abundance and distribution of BSH genes in 380 metagenomes from healthy participants. Finally, we mapped the BSH gene sequences to the gut metagenomic data of 1,605 participants with disease symptoms from publicly available datasets, which were geographically and technically diverse. The large-scale studies indicate that the abundances of different BSH clusters are significantly associated with gastrointestinal diseases, obesity, diabetes, liver diseases, and other remote organ diseases.
Results
Enzyme sequence similarity network classified BSHs into seven clusters
We reviewed the experimentally characterized BSHs in previously published papers.Citation15–Citation17 A total of 44 enzymes that had been previously characterized were selected (Supplementary Table S1). These proteins were used as query sequences to search the UniProt protein database. A total of 5,790 BSH homologs were obtained (Supplementary Dataset S1). We further used the Enzyme Function Initiative-Enzyme Similarity Tool (EFI-EST) to build SSNs with these sequences and mapped the experimentally characterized BSHs on the SSN. The SSN was built with an e-value threshold of 10−40 initially, at which >30% sequence identity was the cutoff for an edge between proteins. The results showed that the enzymes can be grouped into four clusters; however, the enzymes belonging to cluster 1 can further be separated into four sub-clusters (Supplementary Figure S1). When we increased the e-value threshold of SSN to 10−60 (>40% sequence identity), the four sub-clusters were separated and the 5,790 BSH homologs were resolved into seven distinct clusters. In the SSN with an e-value of 10−60, each cluster contained at least one enzyme that had been biochemically characterized (). Further increasing the e-value to 10−75 (>50% sequence identity) led to the overclassification of the proteins into >20 clusters since many clusters did not contain any experimentally characterized BSH (Supplementary Figure S1). We chose the SSN with an e-value of 10−60 for further analysis, which is consistent with previous studies that have shown that homologous proteins with >40% identity are more likely to share biochemical or functional similarity, as judged by their Enzyme Commission numbers.Citation18 We chose one protein sequence from each cluster, and the alignment of these sequences showed that the catalytic cysteine at the N-terminus was highly conserved (Supplementary Figure S2). To illustrate the phylogenetic relationship among the clusters, phylogenetic analysis was performed. The proteins from the same cluster always clustered together with a high level of bootstrap confidence (>85%) in the phylogenetic tree, which further supported the classification of BSHs (Supplementary Figure S3). Moreover, the low bootstrap values (<40%) between the clusters indicated that the evolutionary relationship of the enzymes from different clusters was not particularly significant.
The SSN was analyzed by taxonomic classification at the phylum level to explore the origin of these sequences. Cluster 1 contained 2,342 BSH homologs, which were dominantly from Proteobacteria (50.52%) and Bacteroidetes (18.46%). The archaeal BSHs present in Cluster 1 accounted for only 2.44% of total BSHs in the cluster. In Cluster 2 (1,961 protein sequences), the proteins were primarily from Firmicutes (73.12%) and Actinobacteria (18.46%). The BSHs in Cluster 3 (813 protein sequences) were also predominantly from Proteobacteria (53.67%) and Bacteroidetes (24.32%), similar to the case for those from Cluster 1. The BSHs in Cluster 4 (175 protein sequences) were mainly from Firmicutes (79.52%) and Spirochetes (9.64%). In other clusters (Cluster 5–7), most BSHs were from Firmicutes. We further characterized whether the proteins in each cluster contained signal peptides, which are the N-terminal sorting sequences for targeting proteins into or across membranes (Supplementary Figure S4). The results showed that 75% of proteins in Cluster 1 and 89% of proteins in Cluster 3 contained signal peptides, while the proteins in other clusters did not contain signal peptides. Based on the phylogenetic and signal peptide analysis, the BSH classification indicated a strong likelihood that the enzymes in each cluster shared an immediate common ancestor, as well as similar physiological functions.
Use of SSN and ShortBRED to estimate the distribution and abundance of BSHs in healthy human microbiomes
ShortBRED was used to profile the abundances of the 7 BSH clusters in 380 high-quality metagenomes sequenced from healthy participants during the human microbiome project (HMP) (Supplementary Dataset S2). The metagenomic data were taken from six different sites in the human body: the stool, buccal mucosa, supragingival plaque, tongue dorsum, anterior nares (skin on the face), and vaginal fornix. The microbiome of the stool was used to represent the microbiome of the lower gastrointestinal tract. The data from the buccal mucosa, supragingival plaque, and tongue dorsum were reflective of the oral microbiome. The body sites covered aerobic (face skin), microaerobic (oral and vagina), and anaerobic (gastrointestinal tract) environments. The unique protein sequence markers (85% amino acid identity) were identified for BSHs from each cluster using ShortBRED-Identify. The abundance of each marker in the metagenomic reads was then measured using ShortBRED-Quantify. The abundance of each BSH cluster within each metagenome was quantified by cataloging the sequence markers in the SSN. Finally, the differential abundance values were normalized using previously estimated average microbial genome sizes.Citation19
ShortBRED, together with the SSN, revealed the distribution and abundance of each BSH cluster in human microbiomes from healthy participants (). BSH gene sequences from all seven clusters in the SSN were detected in the human microbiome; however, the abundances and distributions of the clusters were quite different. The enzymes from Cluster 1 were the most abundant in stool samples (median value = 0.25) (). Cluster 3 enzymes were the second most abundant enzymes in the stool samples (median value = 0.16). The median value of Cluster 2 was 0.10; however, the enzymes belonging to this cluster were most abundant in the posterior vagina (median value = 0.09). Cluster 4 enzymes showed relatively low abundances in samples from all body sites but showed higher abundances in samples from both the face skin and oral cavity. The enzymes from other clusters (Clusters 5–7) showed quite low abundances and narrow distributions in human microbiomes (Supplementary Figure S5). The varying abundances of BSHs shed light on the ecological contexts of the enzymes from different clusters and also suggested that enzymes from each cluster may have a different association with the host physiology and health.
Comparative analysis of metagenomic datasets identifies the relationship between the gut BSHs and human diseases
The high abundance and broad distribution of the BSHs form Cluster 1–4 in healthy human gut metagenomes suggested that these clusters might be associated with certain disease states. We performed a comparison analysis of these genes across multiple shotgun metagenomic case-control studies to explore any association between microbiome and diseases. We reviewed the metagenomic whole-genome sequencing datasets of the human gut microbiomes from NCBI Sequence Read Archive (SRA) section (recorded until June 2019). These microbiomes were sequenced on the Illumina platforms. Totally, 20 publicly available and geographically diverse metagenomic studies were retrieved, which covered 12 diseases, including gastrointestinal diseases [ulcerative colitis (UC), Crohn’s disease (CD), colorectal adenomas (CA), and colorectal cancer (CRC)], obesity, type 2 diabetes (T2D), liver diseases [mild nonalcoholic fatty liver disease (NAFLD), advanced NAFLD, and liver cirrhosis], cardiovascular diseases (CVDs), neurological diseases (Parkinson’s disease and epilepsy), and breast cancer (BR) (Supplementary Table S2). In total, 1,605 samples from patients with the above diseases and 1,540 healthy control (CTRLs) samples were analyzed in our study. The DNA sequences of the genes from Clusters 1–4 were retrieved from the UniProt database. The abundances of the genes in Clusters 1–4 were quantified by mapping the genes to the gut metagenomic datasets and the significant value was calculated using the Wilcoxon rank sum test if not specifically indicated ( and Supplementary Figure S6). The abundance of BSHs from Clusters 5–7 was low in healthy individuals (). Further mapping of the genes to the metagenomic cohorts associated with the diseases indicated that the genes from these clusters were present at a low abundance in the human gut (Supplementary Figure S7). Therefore, these clusters were not considered for further analysis.
Figure 3. Bubble plot of the association between the abundance of bile salt hydrolases (BSHs) and host diseases.
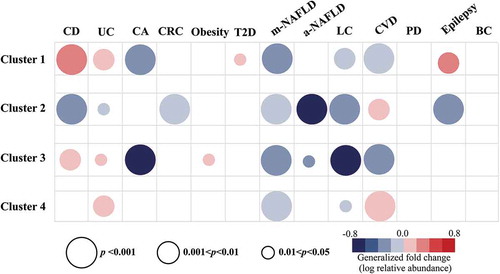
The fact that BAs affect the gastrointestinal tract motivated us to examine the abundance of BSHs in inflammatory diseases (UC and CD), CA, and CRC (). The results showed that the BSH abundance was significantly associated with inflammatory bowel diseases (IBDs) (). Based on the analysis of the two cohorts from Europe (Denmark and Spain) and USA, the abundance of enzymes with signal peptides (Cluster 1 and Cluster 3) increased significantly in the gut of patients with CD (n = 190, n = 174 CTRLs, p = 2.2e-16 and 0.003 for Clusters 1 and 3, respectively) and UC (n = 148, n = 174 CTRLs, p = 0.004 and 0.034 for Clusters 1 and 3, respectively). In contrast, enzymes from Cluster 2 (without signal peptides) displayed a significantly reduced abundance in the gut of patients with CD (p = 2.2e-16). The data for CA (n = 117, n = 152 CTRLs) were collected from three independent studies from five countries, namely Austria, Canada, France, Germany, and the USA. The abundance of the genes in the Clusters 1 and 3 showed a negatively significant association with the disease (p = 3.6e-10 and 2.5e-8, respectively). A similar relationship was observed with regard to the abundance of Cluster 2 BSHs between CRC patients and CTRLs (n = 254, n = 285 CTRLs, p = 2.7e-05), where the data for CRC were retrieved from six independent cohorts from Austria, China, Canada, Italy, Germany, France, and the USA. Based on these analyses, we propose that BSH gene abundance is significantly associated with various gastrointestinal tract diseases including CD, UC, CA, and CRC; however, the positive or negative relationship varied between the BSH clusters.
A “Western” diet that is high in fats and low in fiber contributes to the increased occurrence of metabolic diseases such as obesity and diabetes. Obesity is a major risk factor for T2D and accounts for 90–95% of all diabetes cases.Citation20 The concentration of BAs increased in the patients with obesity or T2D; modulation of BA levels and signaling has been recognized as a potent therapeutic approach to treat these diseases.Citation21 We compared the abundance of BSHs in the gut between patients and controls. The results showed that Cluster 3 was significantly increased in the obesity cohort from Denmark (n = 169, n = 123 CTRLs, p = 0.021). Similarly, the abundance of enzymes from Cluster 1 was increased in the gut of patients with T2D, compared to that in case of the cohort from China (n = 187, n = 183 CTRLs, p = 0.028). Interestingly, both the clusters contained enzymes with the N-terminal signal peptides. Furthermore, the increased level of enzymes from these two clusters is consistent with the increased level of total BAs in the patients.
BAs are synthesized and conjugated in the liver. Furthermore, the secondary BAs produced in the gut; they can be transported to the liver via the hepatic portal vein and can affect liver health.Citation4 We further measured the BSH gene abundance in the gut microbiome of patients with liver diseases using the NAFLD cohort from USA and the LC cohort from China (). In case of mild NAFLD (n = 72, n = 308 CTRLs), the abundances of enzymes from Clusters 1–4 showed a significant relation with the disease (p = 1.4e-06, 5.0e-08, 1.0e-10, and 0.0004, respectively). The abundances of BSHs from Clusters 2 and 3 were also related with advanced NAFLD (n = 14, n = 308 CTRLs, p = 1.3e-07 and 0.043). A negative relation was also observed between LC patients and CTRLs in case of the BSH genes from Clusters 1, 2, 3, and 4 (n = 123, n = 114 CTRLs, p = 0.008, 1.2e-06, 1.0e-16, and 0.048, respectively). In these significant relations, the abundance of BSH genes was always reduced. Overall, the abundance of the majority of BSH gene clusters in the gut was negatively related with NAFLD and LC.
Gut microbiome alterations have also been reported to be associated with additional conditions in remote organs, such CVD, neurological disorders, and BR.Citation22 We were able to quantify the abundance of different BSH clusters in the gut microbiomes of subjects with related conditions (). All four BSH clusters were related with CVD (n = 171, n = 214 CTRLs, p = 7.4e-04, 0.0011, 8.8e-07, and 6.9-e04, respectively) based on the analysis of a metagenomic dataset from China. The abundances of BSHs from Cluster 2 and Cluster 4, which do not harbor signal peptides, were significantly increased; the abundances of BSHs from Cluster 1 and Cluster 3, which contain signal peptides, were significantly reduced in the patients with CVD. We also measured the abundance of BSH genes in a Parkinson’s disease metagenomic cohort from Germany (n = 31, n = 28 CTRLs). The results showed that the abundance of BSH genes was not associated with the disease (p > 0.05, Wilcoxon test or t-test). However, the abundances of the BSH genes of Cluster 1 and Cluster 2 were significantly associated with epilepsy, based on the analysis of a dataset from Germany (n = 24, n = 22 CTRLs, p = 0.002 and 2.3e-15). An altered gut metagenome has been associated with BR;Citation23 however, we found that BSH gene abundance was not significantly different between healthy participants and breast cancer patients by analyzing the metagenomic cohort from China (n = 62, n = 71 CTRLs, p > 0.05, Wilcoxon test or t-test). These analyses demonstrate that the abundance of BSHs in gut microbiomes is also related with the diseases in remote organs.
Validation of associations between BSH abundance and diseases in independent study populations
To validate the associations between BSH gene abundance in the gut and human diseases, we performed independent cohort validation using two additional independent metagenomic cohorts with CD (n = 13, n = 236 CTRLs), UC (n = 69, n = 236 CTRLs), and CRC (n = 91, n = 61 CTRLs) (). The cohort with UC and CD were retrieved from one independent study in Denmark and Spain, while the cohort with CRC was retrieved from another independent study in France and Germany. The calculation and statistical analysis methods used in validation studies were identical to those used in testing cohorts. Validation of the CD cohort showed that BSHs from Clusters 1, 2, and 3 were significantly associated with the disease status (p = 0.0042, 0.024, and 0.046, respectively). Validation of the UC cohort demonstrated that all clusters from 1 to 4 were associated with the disease conditions (p = 0.031, 0.0074, 0.035, and 0.0001, respectively). Finally, only Cluster 2 BSHs were significantly associated with CRC in cohorts from France and Germany (p = 3.8e-07, respectively). These results indicated that the quantification of BSHs in the validation cohorts was highly consistent with the changes in BSH in testing cohorts with respect to significance. Altogether, these results establish a relation between BSHs and human diseases.
Discussion
Deciphering the function, classification, and evolution of a large protein family with diverse contemporary functions is a challenging task. Phylogenetic trees, together with dendrograms, have long been used to describe the sequence relationships between protein families; however, this method is computationally intensive and requires an accurate sequence alignment that is difficult to achieve on a large scale.Citation24 In contrast, protein SSN, which is based on a much larger number of sequences, can establish a global view to interpret sequences as well as structural and functional relationships that are not easily accessible from smaller scale approaches. Although the SSN methods are based on sequence identities from BLAST bit-scores, which is different from sequence alignments in phylogenetic analyses, previous studies have shown that SSNs serve as a visually useful tool to reveal sequence–structure–function associations and evolutionary relationships between protein families.Citation25,Citation26 In case of BSHs, previous studies have classified BSHs into three, four, or eight clusters based on phylogenetic analysis; however, these analyses were based on <400 protein sequences.Citation15–Citation17 Song et al. grouped BSHs into eight clusters using phylogenetic analysis, but the classification separated several BSHs with high sequence identity into different clusters,Citation17 which led to overclassification. In this study, we collected the experimentally characterized enzymes from previous studies and further extended the amount to 5,790 protein sequences. The SSN classified the enzymes into 7 clusters, as shown in the phylogenetic tree (). The classification system imparted some unique characteristics to each cluster. First, the sequence identities among the proteins from different clusters were <40%. Furthermore, the phylogenetic tree showed that there was no clear evolutionary relationship among the 7 clusters. This analysis suggested that the 7 clusters may have evolved from different hydrolase precursors and may have arisen multiple times throughout the evolutionary history, which is consistent with the previous study on BSH evolution.Citation5 Second, the classification system separated the enzymes with and without signal peptides: most of the enzymes in Clusters 1 and 3 have N-terminal signal peptides, while those in other clusters lack these peptides. Third, the classification system separated the enzymes from different phyla (). The enzymes in Clusters 1 and 3 were mainly from Proteobacteria and Bacteroidetes, while those in other Clusters were mainly from Firmicutes. Fourth, the classification linked the different ecological niches of the bacteria with BSHs. The bacteria encoding the BSHs in Clusters 1, 2, and 3 were abundant in the human gut, while the bacteria encoding the BSHs in Cluster 4 were abundant in oral cavity. Furthermore, the bacteria encoding the BSHs in Cluster 2 were abundant in the vagina. Together, these analyses showed that the classification of BSHs based on a large-scale SSN provides new insights into the evolution, function, and ecology of the BSH enzyme family.
Previous SSN studies of glycyl radical enzymes and transporters have suggested that proteins in each cluster of an SSN share a similar biochemical activity;Citation26,Citation27 however, this conclusion does not seem to apply to BSH clusters. The enzymes in Cluster 2 have been well-studied. Two enzymes in this cluster (UniProt ID: R6GBZ3 and A0A380KNN4) have shown 8-fold differences in the specific activity compared to that of GCA even though they share only 60% identity.Citation17 On the other hand, enzymes from Cluster 2 (R6GBZ3) and Cluster 7 (E2YQS1) have shown specific activity similar to that of GCA even though they share only 20% identity.Citation17 The experimentally characterized enzymes from the 7 clusters can hydrolyze both tauro- and glyco-conjugated bile salts. BSH is closely related to penicillin V amidase, and the substrate specificities of the two enzymes cannot be distinguished on the basis of protein sequence or phylogenetic tree analysis.Citation16 Additional studies on the biochemistry and structure of BSHs will be needed to clarify the promiscuity and substrate specificity of the enzymes.
The SSN, together with ShortBRED showed the abundance and distribution of BSHs in human microbiomes, which provided new insights into the ecological niches of bacteria harboring different clusters of BSHs (). The BSHs in Cluster 2 were predominant in the vagina, suggesting that the bacteria harboring the BSHs in Cluster 2 can colonize the vagina. Similarly, the BSHs in Cluster 4 were highly abundant in the supragingival plaque, implicating that these BSHs may be related to dental health. These data provide important clues to precisely manipulate BSH-active bacteria in order to improve human health. For example, the bacteria harboring the BSHs in Cluster 2, which show good colonization in the vagina, may serve as promising probiotics for improving vaginal health. These data also helped us rank the priority of BSHs for further study. Of the 44 experimentally characterized enzymes, 34 enzymes belonged to Cluster 2, and only 5 enzymes (two enzymes in Cluster 1 and three enzymes in Cluster 3) harbored signal peptides (Supplementary Table S1). However, the ShortBRED results showed that the enzymes belonging to Cluster 1 and Cluster 3 were more abundant in the gut than the enzymes in Cluster 2, suggesting that the focus of BSH research should switch from Cluster 2 to Clusters 1 and 3.
BA metabolism, which primarily involves hydrolysis and 7α/β-dehydroxylation reactions, is associated with many human diseases.Citation22 Our results showed that BSH gene abundance is associated with IBDs, CA, and CRC (). In the present study, the decreased abundance of Cluster 2 BSH genes observed in CD conditions is consistent with a previous study.Citation28 We further showed that the abundance of Cluster 1 and Cluster 3 BSH genes is enhanced in the IBDs. These results are in accordance with the two well-established IBD-associated taxonomic signatures: (i) phylum-level decrease in Firmicutes, and (ii) phylum-level increase in Proteobacteria,Citation29 since the BSHs in Cluster 2 are predominantly from Firmicutes, while the BSHs in Clusters 1 and 3 are predominantly from Proteobacteria. Furthermore, our analysis from six independent studies in seven countries indicated that the abundance of Cluster 2 BSH genes, predominantly of Firmicutes, is associated with CRC, which was consistent with the previous studies that showed that Firmicutes were significantly depleted in the gut microbiome of CRC patients.Citation30,Citation31 Taken together, these analyses suggest that the abundance of BSHs is highly related with gastrointestinal diseases.
High-fat diets increase the levels of BAs in the gut. The BA concentration can reach 1 mM in the middle gut after the intake of a high-fat meal.Citation32,Citation33 The abundance of Firmicutes is shown to increase and the abundance of Bacteroidetes is shown to decrease in the guts of both a mouse model with high-fat diet-induced obesity or humans with obesity, compared to the respective lean control subjects.Citation34 High-level expression of recombinant BSH in conventionally raised mice leads to a significant reduction in conjugated BAs, plasma cholesterol, liver triglycerides, and host weight gain,Citation35 suggesting that BSH is an important target to regulate host lipid metabolism and weight gain. Our analysis showed that the abundance of BSHs from Firmicutes (Cluster 2 and 4) was not associated with obesity and T2D. In contrast, the BSHs from Cluster 1 and Cluster 3, which contain N-terminal signal peptides and are predominantly from Proteobacteria and Bacteroidetes, increased significantly in the patients with T2D and obesity, respectively, suggesting that BSHs from Proteobacteria or Bacteroidetes may contribute immensely to host health. This result is in accordance with the previous study which showed that the BSH with signal peptide from Bacteroides thetaiotaomicron can alter the in vivo BA pool and exert significant effects on the host metabolic status.Citation5 Considering the significance of probiotics with BSH activity as a possible approach to prevent and treat obesity, we propose that the bacteria with BSH activity belonging to Bacteroidetes but not Firmicutes can be potential probiotics to ameliorate obesity.
Fatty liver diseases are strongly associated with obesity and T2D, and may develop to nonalcoholic steatohepatitis, cirrhosis, and eventually, liver cancer.Citation36 In patients with NAFLD, total fecal BA concentrations are elevated;Citation37 in contrast, cirrhosis is associated with a decrease in total fecal BA concentration.Citation38,Citation39 Our analysis showed that the majority of BSH clusters were less abundant in patients with liver diseases than in control subjects. The decrease of bacteria with BSH activity was also observed in piglet model of short bowel syndrome-associated liver disease, which was regulated by altered farnesoid X receptor (FXR) signaling.Citation40 Zhang et al. reported that FXR plays important roles in shaping the gut microbiota of mice and treatment with FXR antagonist can decrease both the abundance of bacteria encoding BSHs and BSH activity.Citation41 Interestingly, the expression of FXR is downregulated during the development of liver diseases.Citation42 On the basis of this background, we proposed that the decrease of BSH abundance in patients with liver diseases is associated with the host FXR expression level; however, further experiments need to be performed to elucidate the related underlying mechanisms.
BSH gene abundance is also associated with diseases in the remote organs, such as CVD and epilepsy (). The BSH-active probiotic bacteria have been shown to possess cholesterol-lowering efficacy, and high cholesterol has been closely associated with CVD, implying that these bacteria may have great potential for improving cardiovascular function.Citation43 In addition, the BAs produced by BSH activity can regulate cardiovascular function through G-protein-coupled receptors (TGR5 and muscarinic receptors) and nuclear receptors (FXR and pregnane X receptor), which are expressed in cardiovascular tissues.Citation44 In particular, the clusters of enzymes with and without signal peptides displayed inverse relations with CVD. These analyses suggest that BSHs in different clusters may have varying associations with CVD. Similarly, BAs also play important roles in brain signaling, where they bind membrane-bound or nuclear receptors. Therefore, the effects of BAs can also be seen in neurological diseases.Citation45 However, the mechanisms underlying the regulation of neurological diseases by BSHs remain poorly understood.
There is high variability between individuals with respect to the composition of the gut microbiome, which can be explained by the diet, early microbial exposure, genetic background, and other factors; unfortunately, these factors can cause false associations.Citation46 In this study, we performed a large-scale analysis of BSHs to overcome these confounding factors. We collected a large number of BSH homologs for classification. Then, we quantified the abundance and distribution of the enzymes in a large number of healthy participants. Finally, we identified the associations between the enzyme clusters and human diseases using large-scale cohort studies. Taken together, we suggested that the pipeline used in the present study from enzyme sequences to metagenomic data can also be applied to study the relationship between other microbial enzymes and host health. The pipeline can further be improved by augmenting the two databases used in the study. First, the number of BSH sequences can be increased. We collected a large number of BSH sequences including the experimentally characterized BSHs and their homologs that should ideally cover the majority BSHs in the gut; however, the “dark matter” in gut microbiomes, which includes sequences that are not annotated precisely, is yet to be explored.Citation47 Hundreds of novel enzymes can be discovered from metagenomes every year.Citation48 It is very likely that novel BSHs can be discovered and characterized experimentally from the human gut in the near future, which can further increase our wealth of knowledge about the relationship between BSHs and host health. Second, the extension of metagenomic datasets can improve the accuracy of relationships between the diseases and the gene abundance. We retrieved 20 metagenomic datasets and all of them were sequenced on the Illumina platforms ranging from Illumina Genome Analyzer II to Illumina HiSeq 4000. However, each methodological step including sample collection, storage, DNA extraction, and sequencing may affect the overall end result.Citation49,Citation50 This issue can be properly addressed by analyzing multiple metagenomic case-control studies, which is an effective way to increase the reproducibility and predictive accuracy.Citation51,Citation52 In the present study, we used more than one dataset to quantify the relationship between BSHs and gastrointestinal tract diseases, including CD, UC, CA, and CRC, since the microbiomes in these diseases have been well-characterized and investigated in multiple research studies. We only used one dataset to examine the relationship between the microbiome and other diseases; however, validation studies using independent cohorts with UC, CD, and CRC suggested that the relationships identified by the pipeline were highly reproducible. Finally, we propose that the pipeline used in the study is highly efficient and reliable. In future, more metagenomic datasets should be included with increased knowledge of whole metagenomic sequencing, which can provide more accurate and explicit details regarding the relationships between the microbial gene abundance and human diseases.
Conclusions
Taken together, we performed a large-scale study using enzyme sequences and metagenomic data to provide an assessment of the association between BSHs and human diseases. First, a new classification system was developed to separate the enzymes into seven clusters. Second, the abundance and distribution analysis of the BSHs in healthy participants enabled the identification of the primary clusters in the human microbiome. Third, the associations between BSH clusters and several human diseases were discovered. Our work elucidates the association between a given BSH cluster and a specific disease, even if it is challenging to clarify the associate, contributing, or causal relationship between BSH and the diseases. However, our analysis provides the basis for future diagnostic applications of BSHs as a valuable noninvasive biomarker of several diseases. Furthermore, our data could contribute to future researches that aim to precisely manipulate BSH-active bacteria for use as probiotics to improve human health.
Methods
Enzyme discovery and in silico characterization of BSHs
The complete amino acid sequences of experimentally characterized enzymes with a representative function in humans were collected from previously published literature (publication cutoff: January 2019). Summaries of their accession numbers, origins, signal peptides, and references are listed in Supplementary Table S1. These proteins were combined as seed sequences and putative BSHs were searched in the UniProt database (Version: 2019_02) using BLASTP with a cutoff e–value of 10−5. The obtained BSH homologs are listed in Dataset S1 (Supporting Information). The EFI-EST tool was used to construct the protein SSNs,Citation24 which were visualized by Cytoscape 3.3.Citation53 Networks were generated at score values of e = 10−40,10−60, or 10−75. The corresponding protein sequence identity values were 30%, 40%, and 50%, respectively. To reduce the file size of the networks, the networks were filtered as 90% representative node networks. Each node in the SSN contained sequences with >90% amino acid identity and each edge indicated that the two nodes connected by that edge shared an e-value less than the selected cutoff. Multiple alignments were constructed using the Clustal Omega server.Citation54 The phylogenetic trees were generated with MEGA X based on Clustal Omega alignments using maximum likelihood (ML) methods and bootstrapping with 1000 iterations.Citation55 The signal peptides of the proteins were predicted by SignalP 5.0.Citation56
Determination of BSH abundance using healthy human metagenomic data
ShortBRED was used to determine the abundance of BSHs in human metagenomes as previously reported.Citation57 It was run on the EFI-chemically guided functional profiling (EFI-CGFP) platform.Citation47 The BSHs in the clusters were filtered at an 85% amino acid similarity threshold to identify non-redundant representative sequences. UniRef90 (Version: 2019_02) was used as a comprehensive, non-redundant protein reference catalog. These representative BSH sequences were compared with UniRef90 using ShortBRED-Identify, which was run with the default parameters to identify representative peptide markers for the BSH clusters. After obtaining distinguishing peptide markers, ShortBRED-Quantify was carried out with the default parameters to quantify the marker abundances against 380 metagenomic datasets from the HMP and then map these to the SSN clusters. The relative abundance based on reads per kilobase million (RPKM) values calculated by ShortBRED-Quantify was converted into “copies per microbial genome” as previously reported.Citation27
Abundance analysis of BSH genes in human gut metagenomes under disease conditions
The metagenomic whole-genome sequencing datasets of the human gut microbiomes that were generated on Illumina platforms from 2012 to June 2019 were downloaded from the SRA of the NCBI (Supplementary Table S2). The datasets containing the sample record for ambiguous attributes were excluded. On the basis of disease, data were classified into the individual patient samples and corresponding healthy controls to examine the association of BSH genes with each disease. To homogenize diverse datasets, low-quality reads were trimmed at a quality threshold of 30 bp and a minimum length of 50 bp using Sickle software (version 1.33, https://github.com/najoshi/sickle). The high-quality sequencing reads were matched to the nucleotide sequences of each BSH cluster using the Burrows-Wheeler Alignment (BWA) MEM algorithm (version 0.7.17-r1194-dirty) with default settings.Citation58 The SAMtools program (version 1.9) was used to filter aligned reads by only retaining reads that showed mapping quality above 1.Citation59 The reads matched to each BSH cluster were counted using BEDtools (version 2.27.1-dirty, https://bedtools.readthedocs.io/). The read counts of BSH genes in the gut metagenome samples were normalized to read counts per million metagenome reads and visualized by constructing boxplots using the ggplot2 package (version 3.1.0) in the R programming language.
Statistical analysis
All statistical analyses were performed using R (version 3.5.3). Normality of a given abundance of BSH genes was assessed by the Shapiro–Wilk test. If the data were normally distributed, two-sample Student’s t-test was performed. If the data were not normally distributed, two-sample Wilcoxon rank sum test (Mann-Whitney test) was employed to calculate the significance of relative abundance between healthy and diseased subjects. Logarithmic general fold change was also calculated for diseased subjects and healthy controls using quantiles ranging from 0.1 to 0.9, with equal increments of 0.1.Citation52
Disclosure of Potential Conflicts of interest
The authors report no conflicts of interest.
Supplemental Material
Download PDF (1.7 MB)Supplementary material
Supplemental data for this article can be accessed on the publisher’s website.
Additional information
Funding
References
- Koppel N, Balskus EP. Exploring and understanding the biochemical diversity of the human microbiota. Cell Chem Biol. 2016;23(1):18–30. doi:https://doi.org/10.1016/j.chembiol.2015.12.008.
- Gilbert JA, Blaser MJ, Caporaso JG, Jansson JK, Lynch SV, Knight R. Current understanding of the human microbiome. Nat Med. 2018;24(4):392–400. doi:https://doi.org/10.1038/nm.4517.
- Ridaura VK, Faith JJ, Rey FE, Cheng J, Duncan AE, Kau AL, Griffin NW, Lombard V, Henrissat B, Bain JR, et al. Gut microbiota from twins discordant for obesity modulate metabolism in mice. Science. 2013;341(6150):1241214. doi:https://doi.org/10.1126/science.1241214.
- Jia B, Jeon CO. Promotion and induction of liver cancer by gut microbiome-mediated modulation of bile acids. PLoS Pathog. 2019;15(9):e1007954. doi:https://doi.org/10.1371/journal.ppat.1007954.
- Yao L, Seaton SC, Ndousse-Fetter S, Adhikari AA, DiBenedetto N, Mina AI, Banks AS, Bry L, Devlin AS. A selective gut bacterial bile salt hydrolase alters host metabolism. Elife. 2018;7. doi:https://doi.org/10.7554/eLife.37182.
- Canfora EE, Meex RCR, Venema K, Blaak EE. Gut microbial metabolites in obesity, NAFLD and T2DM. Nat Rev Endocrinol. 2019;15(5):261–273. doi:https://doi.org/10.1038/s41574-019-0156-z.
- Cho CE, Taesuwan S, Malysheva OV, Bender E, Tulchinsky NF, Yan J, Sutter JL, Caudill MA. Trimethylamine-N-oxide (TMAO) response to animal source foods varies among healthy young men and is influenced by their gut microbiota composition: A randomized controlled trial. Mol Nutr Food Res. 2017;61. doi:https://doi.org/10.1002/mnfr.201600324.
- Winston JA, Theriot CM. Diversification of host bile acids by members of the gut microbiota. Gut Microbes. 2019:1–14. doi:https://doi.org/10.1080/19490976.2019.1674124.
- Tilg H, Cani PD, Mayer EA. Gut microbiome and liver diseases. Gut. 2016;65(12):2035–2044. doi:https://doi.org/10.1136/gutjnl-2016-312729.
- Yu LX, Schwabe RF. The gut microbiome and liver cancer: mechanisms and clinical translation. Nat Rev Gastroenterol Hepatol. 2017;14(9):527–539. doi:https://doi.org/10.1038/nrgastro.2017.72.
- Shapiro H, Kolodziejczyk AA, Halstuch D, Elinav E. Bile acids in glucose metabolism in health and disease. J Exp Med. 2018;215(2):383–396. doi:https://doi.org/10.1084/jem.20171965.
- Foley MH, O’Flaherty S, Barrangou R, Theriot CM. Bile salt hydrolases: gatekeepers of bile acid metabolism and host-microbiome crosstalk in the gastrointestinal tract. PLoS Pathog. 2019;15(3):e1007581. doi:https://doi.org/10.1371/journal.ppat.1007581.
- Jones ML, Tomaro-Duchesneau C, Martoni CJ, Prakash S. Cholesterol lowering with bile salt hydrolase-active probiotic bacteria, mechanism of action, clinical evidence, and future direction for heart health applications. Expert Opin Biol Ther. 2013;13(5):631–642. doi:https://doi.org/10.1517/14712598.2013.758706.
- Joyce SA, Shanahan F, Hill C, Gahan CGM. Bacterial bile salt hydrolase in host metabolism: potential for influencing gastrointestinal microbe-host crosstalk. Gut Microbes. 2014;5(5):669–674. doi:https://doi.org/10.4161/19490976.2014.969986.
- Dong Z, Lee BH. Bile salt hydrolases: structure and function, substrate preference, and inhibitor development. Protein Sci. 2018;27(10):1742–1754. doi:https://doi.org/10.1002/pro.3484.
- Jones BV, Begley M, Hill C, Gahan CG, Marchesi JR. Functional and comparative metagenomic analysis of bile salt hydrolase activity in the human gut microbiome. Proc Natl Acad Sci USA. 2008;105(36):13580–13585. doi:https://doi.org/10.1073/pnas.0804437105.
- Song Z, Cai Y, Lao X, Wang X, Lin X, Cui Y, Kalavagunta PK, Liao J, Jin L, Shang J, et al. Taxonomic profiling and populational patterns of bacterial bile salt hydrolase (BSH) genes based on worldwide human gut microbiome. Microbiome. 2019;7(1):9. doi:https://doi.org/10.1186/s40168-019-0628-3.
- Pearson WR An introduction to sequence similarity (“homology”) searching. Current protocols in bioinformatics/editoral board, Andreas D Baxevanis [et al] 2013; Chapter 3: Unit31. doi:https://doi.org/10.1002/0471250953.bi0301s42.
- Nayfach S, Pollard KS. Average genome size estimation improves comparative metagenomics and sheds light on the functional ecology of the human microbiome. Genome Biol. 2015;16(1):51. doi:https://doi.org/10.1186/s13059-015-0611-7.
- Vazquez G, Duval S, Jacobs DR Jr., Silventoinen K. Comparison of body mass index, waist circumference, and waist/hip ratio in predicting incident diabetes: a meta-analysis. Epidemiol Rev. 2007;29(1):115–128. doi:https://doi.org/10.1093/epirev/mxm008.
- Tomkin GH, Owens D. Obesity diabetes and the role of bile acids in metabolism. J Transl Int Med. 2016;4(2):73–80. doi:https://doi.org/10.1515/jtim-2016-0018.
- Durack J, Lynch SV. The gut microbiome: relationships with disease and opportunities for therapy. J Exp Med. 2019;216(1):20–40. doi:https://doi.org/10.1084/jem.20180448.
- Zhu J, Liao M, Yao Z, Liang W, Li Q, Liu J, Yang H, Ji Y, Wei W, Tan A, et al. Breast cancer in postmenopausal women is associated with an altered gut metagenome. Microbiome. 2018;6(1):136. doi:https://doi.org/10.1186/s40168-018-0515-3.
- Gerlt JA, Bouvier JT, Davidson DB, Imker HJ, Sadkhin B, Slater DR, Whalen KL. Enzyme function initiative-enzyme similarity tool (EFI-EST): A web tool for generating protein sequence similarity networks. Biochim Biophys Acta. 2015;1854(8):1019–1037. doi:https://doi.org/10.1016/j.bbapap.2015.04.015.
- Akiva E, Copp JN, Tokuriki N, Babbitt PC. Evolutionary and molecular foundations of multiple contemporary functions of the nitroreductase superfamily. Proc Natl Acad Sci USA. 2017;114(45):E9549–E58. doi:https://doi.org/10.1073/pnas.1706849114.
- Jia B, Yuan DP, Lan WJ, Xuan YH, Jeon CO. New insight into the classification and evolution of glucose transporters in the Metazoa. Faseb J. 2019;33(6):7519–7528. doi:https://doi.org/10.1096/fj.201802617R.
- Levin BJ, Huang YY, Peck SC, Wei Y, Martíez-del Campo A, Marks JA, Franzosa EA, Huttenhower C, Balskus EP. A prominent glycyl radical enzyme in human gut microbiomes metabolizes trans-4-hydroxy-prolinetrans −4-hydroxy-l-proline. Science. 2017;355(6325):eaai8386. doi:https://doi.org/10.1126/science.aai8386.
- Ogilvie LA, Jones BV. Dysbiosis modulates capacity for bile acid modification in the gut microbiomes of patients with inflammatory bowel disease: a mechanism and marker of disease? Gut. 2012;61(11):1642–1643. doi:https://doi.org/10.1136/gutjnl-2012-302137.
- Huttenhower C, Kostic AD, Xavier RJ. Inflammatory bowel disease as a model for translating the microbiome. Immunity. 2014;40(6):843–854. doi:https://doi.org/10.1016/j.immuni.2014.05.013.
- Ahn J, Sinha R, Pei Z, Dominianni C, Wu J, Shi J, Goedert JJ, Hayes RB, Yang L. Human gut microbiome and risk for colorectal cancer. J Natl Cancer Inst. 2013;105(24):1907–1911. doi:https://doi.org/10.1093/jnci/djt300.
- Kostic AD, Gevers D, Pedamallu CS, Michaud M, Duke F, Earl AM, Ojesina AI, Jung J, Bass AJ, Tabernero J. Genomic analysis identifies association of Fusobacterium with colorectal carcinoma. Genome Res. 2012;22(2):292–298. doi:https://doi.org/10.1101/gr.126573.111.
- Ridlon JM, Kang DJ, Hylemon PB, Bajaj JS. Bile acids and the gut microbiome. Curr Opin Gastroenterol. 2014;30(3):332–338. doi:https://doi.org/10.1097/MOG.0000000000000057.
- Bernstein C, Bernstein H, Garewal H, Dinning P, Jabi R, Sampliner RE, McCuskey MK, Panda M, Roe DJ, L’Heureux L, et al. A bile acid-induced apoptosis assay for colon cancer risk and associated quality control studies.. Cancer Res. 1999;59(10):2353–2357.
- Musso G, Gambino R, Cassader M. Obesity, diabetes, and gut microbiota: the hygiene hypothesis expanded? Diabetes Care. 2010;33(10):2277–2284. doi:https://doi.org/10.2337/dc10-0556.
- Joyce SA, MacSharry J, Casey PG, Kinsella M, Murphy EF, Shanahan F, Hill C, Gahan CGM. Regulation of host weight gain and lipid metabolism by bacterial bile acid modification in the gut. Proc Natl Acad Sci USA. 2014;111(20):7421–7426. doi:https://doi.org/10.1073/pnas.1323599111.
- Ratziu V, Marchesini G. When the journey from obesity to cirrhosis takes an early start. J Hepatol. 2016;65(2):249–251. doi:https://doi.org/10.1016/j.jhep.2016.05.021.
- Mouzaki M, Wang AY, Bandsma R, Comelli EM, Arendt BM, Zhang L, Fung S, Fischer SE, McGilvray IG, Allard JP. Bile acids and dysbiosis in non-alcoholic fatty liver disease. PLoS One. 2016;11(5):e0151829–e. doi:https://doi.org/10.1371/journal.pone.0151829.
- Kakiyama G, Pandak WM, Gillevet PM, Hylemon PB, Heuman DM, Daita K, Takei H, Muto A, Nittono H, Ridlon JM. Modulation of the fecal bile acid profile by gut microbiota in cirrhosis. J Hepatol. 2013;58(5):949–955. doi:https://doi.org/10.1016/j.jhep.2013.01.003.
- Ridlon JM, Alves JM, Hylemon PB, Bajaj JS. Cirrhosis, bile acids and gut microbiota. Gut Microbes. 2013;4(5):382–387. doi:https://doi.org/10.4161/gmic.25723.
- Pereira-Fantini PM, Lapthorne S, Joyce SA, Dellios NL, Wilson G, Fouhy F, Thomas SL, Scurr M, Hill C, Gahan CGM. Altered FXR signalling is associated with bile acid dysmetabolism in short bowel syndrome-associated liver disease. J Hepatol. 2014;61(5):1115–1125. doi:https://doi.org/10.1016/j.jhep.2014.06.025.
- Zhang L, Xie C, Nichols RG, Chan SHJ, Jiang C, Hao R, Smith PB, Cai J, Simons MN, Hatzakis E. Farnesoid X receptor signaling shapes the gut microbiota and controls hepatic lipid metabolism. mSystems. 2016;1(5):e00070–16. doi:https://doi.org/10.1128/mSystems.00070-16.
- Armstrong LE, Guo GL. Role of FXR in liver inflammation during nonalcoholic steatohepatitis. Curr Pharmacol Rep. 2017;3(2):92–100. doi:https://doi.org/10.1007/s40495-017-0085-2.
- Jones ML, Tomaro-Duchesneau C, Martoni CJ, Prakash S. Cholesterol lowering with bile salt hydrolase-active probiotic bacteria, mechanism of action, clinical evidence, and future direction for heart health applications. Expert Opin Biol Ther. 2013;13(5):631–642. doi:https://doi.org/10.1517/14712598.2013.758706.
- Khurana S, Raufman JP, Pallone TL. Bile acids regulate cardiovascular function. Clin Transl Res. 2011;4:210–218. doi:https://doi.org/10.1111/j.1752-8062.2011.00272.x.
- McMillin M, DeMorrow S. Effects of bile acids on neurological function and disease. Faseb J. 2016;30(11):3658–3668. doi:https://doi.org/10.1096/fj.201600275R.
- Schmidt TSB, Raes J, Bork P. The human gut microbiome: from association to modulation. Cell. 2018;172(6):1198–1215. doi:https://doi.org/10.1016/j.cell.2018.02.044.
- Zallot R, Oberg N, Gerlt JA. The EFI web resource for genomic enzymology tools: leveraging protein, genome, and metagenome databases to discover novel enzymes and metabolic pathways. Biochemistry. 2019;58(41):4169–4182. doi:https://doi.org/10.1021/acs.biochem.9b00735.
- Berini F, Casciello C, Marcone GL, Marinelli F. Metagenomics: novel enzymes from non-culturable microbes. FEMS Microbiol Lett. 2017:364. doi:https://doi.org/10.1093/femsle/fnx211.
- Panek M, Cipcic Paljetak H, Baresic A, Peric M, Matijasic M, Lojkic I, Vranešić Bender D, Krznarić Ž, Verbanac D. Methodology challenges in studying human gut microbiota - effects of collection, storage, DNA extraction and next generation sequencing technologies. Sci Rep. 2018;8(1):5143. doi:https://doi.org/10.1038/s41598-018-23296-4.
- Knudsen BE, Bergmark L, Munk P, Lukjancenko O, Prieme A, Aarestrup FM, Pamp SJ. Impact of sample type and DNA isolation procedure on genomic inference of microbiome composition. mSystems. 2016;1. doi:https://doi.org/10.1128/mSystems.00095-16.
- Thomas AM, Manghi P, Asnicar F, Pasolli E, Armanini F, Zolfo M, Beghini F, Manara S, Karcher N, Pozzi C, et al. Metagenomic analysis of colorectal cancer datasets identifies cross-cohort microbial diagnostic signatures and a link with choline degradation. Nat Med. 2019;25:667–678. doi:https://doi.org/10.1038/s41591-019-0405-7.
- Wirbel J, Pyl PT, Kartal E, Zych K, Kashani A, Milanese A, Fleck JS, Voigt AY, Palleja A, Ponnudurai R, et al. Meta-analysis of fecal metagenomes reveals global microbial signatures that are specific for colorectal cancer. Nat Med. 2019;25:679–689. doi:https://doi.org/10.1038/s41591-019-0406-6.
- Shannon P, Markiel A, Ozier O, Baliga NS, Wang JT, Ramage D, Amin N, Schwikowski B, Ideker T. Cytoscape: a software environment for integrated models of biomolecular interaction networks. Genome Res. 2003;13(11):2498–2504. doi:https://doi.org/10.1101/gr.1239303.
- Madeira F, Park YM, Lee J, Buso N, Gur T, Madhusoodanan N, Basutkar P, Tivey ARN, Potter SC, Finn RD, et al. The EMBL-EBI search and sequence analysis tools APIs in 2019. Nucleic Acids Res. 2019. doi:https://doi.org/10.1093/nar/gkz268.
- Kumar S, Stecher G, Li M, Knyaz C, Tamura K. MEGA X: molecular evolutionary genetics analysis across computing platforms. Mol Biol Evol. 2018;35(6):1547–1549. doi:https://doi.org/10.1093/molbev/msy096.
- Almagro Armenteros JJ, Tsirigos KD, Søderby CK, Petersen TN, Winther O, Brunak S, von Heijne G, Nielsen H. SignalP 5.0 improves signal peptide predictions using deep neural networks. Nat Biotech. 2019;37:420–423. doi:https://doi.org/10.1038/s41587-019-0036-z.
- Kaminski J, Gibson MK, Franzosa EA, Segata N, Dantas G, Huttenhower C. High-specificity targeted functional profiling in microbial communities with ShortBRED. PLoS Comput Biol. 2015;11(12):e1004557. doi:https://doi.org/10.1371/journal.pcbi.1004557.
- Li H, Durbin R. Fast and accurate short read alignment with Burrows-Wheeler transform. Bioinformatics. 2009;25(14):1754–1760. doi:https://doi.org/10.1093/bioinformatics/btp324.
- Li H, Handsaker B, Wysoker A, Fennell T, Ruan J, Homer N, Marth G, Abecasis G, Durbin R. The sequence alignment/map format and SAMtools. Bioinformatics. 2009;25(16):2078–2079. doi:https://doi.org/10.1093/bioinformatics/btp352.