ABSTRACT
The early-life gut microbiota is associated with potential development of diseases in adulthood. The sterile womb paradigm has been challenged by recent reports that revealed the presence of the meconium, amniotic fluid, and placenta microbiome. This study aimed to explore the maternal origin of the microbiota of neonate meconium by using the PacBio single-molecule real-time circular consensus sequencing technology. Such technology could produce high fidelity reads of full-length 16S rRNA genes, improving the sensitivity and specificity of taxonomic profiling. It also reduced the risk of false positives. This study analyzed the full-length 16S rRNA-based microbiota of maternal samples (amniotic fluid, feces, vaginal fluid, saliva) and first-pass meconium of 39 maternal-neonate pairs. Alpha- and beta-diversity analyses revealed sample type-specific microbiota features. Most sample types were dominated by sequences representing different genera (Lactobacillus and Curvibacter in the amniotic fluid and vaginal fluid microbiota; Bacillus and Escherichia/Shigella in the meconium microbiota; Bacteroides and Faecalibacterium in the maternal fecal microbiota; Streptococcus and Prevotella in the maternal saliva microbiota). Moreover, specific operational taxonomic units (OTUs) were identified in all sample types. Dyad analysis revealed common OTUs between the meconium microbiota and microbiota of multiple maternal samples. The meconium microbiota shared more features with the amniotic fluid microbiota than the maternal fecal and vaginal microbiota. Our results strongly suggested that the meconium microbiota was seeded from multiple maternal body sites, and the amniotic fluid microbiota contributed most to the seeding of the meconium microbiota among the investigated maternal body sites.
Introduction
The gut microbiota is closely connected with human health. Dysbiotic shifts in the gut microbiota are associated with various diseases, including obesity, inflammatory bowel disorder, autoimmune diseases, and gastrointestinal cancer.Citation1 Stewart et al. reported that the gut microbiome development progressed through three distinct phases: a developmental phase, a transitional phase, and a stable phase; and breast milk feeding was the most significant factor influencing the microbiome structure and development.Citation2 The gut microbiota in early life changed through interacting with the developing intestinal immune system.Citation3 Defects in the development of invariant natural killer T cells and B cells in germ-free mice could be partly corrected by early post-natal colonization of commensal bacteria,Citation4,Citation5 suggesting that age-sensitive exposure to the normal microbial flora provided critical developmental cues for the immune system. Moreover, colonizing pregnant mice reprogrammed early postnatal innate immune development by increasing the expression of genes encoding epithelial antibacterial peptides and metabolic pathways relevant to microbial molecules.Citation6 Thus, an in-depth understanding of the role of maternal bacteria in the seeding of the infant gut microbiota would help design early intervention strategies to improve human health, particularly in the aspect of immune system development. However, the origin of the neonatal gut microbiota remains controversial.Citation7
Recently, the sterile womb paradigm has been challenged. Jiménez et al. isolated bacteria from umbilical cord blood of healthy neonates and from murine amniotic fluid, suggesting that term fetuses were not completely sterile and that a prenatal mother-to-child efflux of commensal bacteria might exist.Citation8 The highly similar nucleotide variation patterns in some gut microbes of maternal-neonate pairs suggested potential vertical transmission of certain bacterial strains from mother to child.Citation9 Gestational diabetes mellitus was associated with concordant alterations of maternal and neonatal microbiota.Citation10 A strain-resolved metagenomic analysis revealed that the infant microbiome was largely acquired and transmitted maternally.Citation11 The in utero colonization hypothesis was further supported by the detection of microbes and/or microbiomes in samples of meconium,Citation12–Citation14 amniotic fluid,Citation15,Citation16 placenta,Citation17–Citation22 and uterusCitation23,Citation24 by metagenomic sequencing, conventional microbial cultivation and/or microscopic methods. We thus hypothesized the existence of a maternally derived neonatal microbiome.
Although a large body of studies has reported the existence of microbiomes of the placenta, amniotic fluid, the chorionic and amniotic membranes in normal, uncomplicated pregnancies, several studies presented contrary findings. Rehbinder et al. analyzed the amniotic fluid microbiota of 24 mothers and concluded that amniotic fluid was sterile in uncomplicated term pregnancy until uterine contractions and/or rupture of membranes.Citation25 Lim et al. found no obvious difference between the microbiota of amniotic fluid obtained from uncomplicated term pregnancies and buffer negative controls,Citation26 and de Goffau et al. found no evidence to support the existence of a placental microbiome in both complicated and uncomplicated pregnancies.Citation27 These studies supported the sterile womb paradigm and disproved the in utero colonization hypothesis. They further argued that the presence of a meconium microbiota in previous studies was merely the results of DNA contamination originated from laboratory reagents or acquired during delivery.
The accuracy of profiling microbial communities in low microbial biomass samples (e.g., placenta, amniotic fluid, meconium) has been hindered by our ability to distinguish the authentic signals beyond the level of background contamination. The placental microbiome was firstly characterized metagenomically by Aagaard et al. (2014) using whole-genome shotgun sequencing and 454 pyrosequencing technologies.Citation17 The 454 pyrosequencing technology produced sequences of medium read length (~450 bp).Citation28 By analyzing samples collected from multiple human body sites of pregnant and nonpregnant subjects, Aagaard et al. found that the placental microbiome comprised nonpathogenic commensals most akin to the oral microbiome.Citation17 In contrast, de Goffau et al. (2019) failed to identify distinguishable signals between the placental samples and contaminant controls by sequencing relatively short reads covering the V1-V2 hypervariable regions of the 16S rRNA (~260 bp).Citation27 The conflicting inferences could be resulted from the chosen technologies that relied on different sub-regions and read lengths of 16S rRNA genes, resulting in different power of taxonomic resolution. Moreover, the long-read sequencing technology was potentially advantageous in reducing the contamination risk for metagenomic profiling of low microbial biomass samples, and employing thoughtful filtering settings and vigorous contaminant controls might further ‘decontaminate’ the putative contaminant amplicon sequence variants.Citation22
Stinson et al. applied the PacBio single-molecule real-time (SMRT) sequencing technology together with workflows aiming to reduce contamination, showing the existence of the meconium and amniotic fluid microbiome beyond the level of background contamination.Citation16 The PacBio SMRT sequencing technology has the major advantage of producing long reads. It has been used to profile the microbial communities of various samples based on sequencing the full-length 16S rRNA gene to provide more precise species and strain-level data.Citation29,Citation30 Such fine-scale taxonomic resolution was unachievable by other currently available sequencing platforms. The high random error rate of PacBio sequencing could be rectified by the circular consensus sequencing (CCS) strategy, which created high fidelity reads by multiple passes of template molecules, achieving a low error rate of 0.007%.Citation31 Thus, combining these methods would enable more accurate taxonomic and phylogenetic resolution of microbial communities. Moreover, different sequences assigned under the same operational taxonomic unit (OTU) were closely related phylotypes, allowing OTU tracking analysis.
This study analyzed the high taxonomic resolution microbiota profiles of samples of 39 maternal-neonate pairs (Table S1) to investigate the contribution of the microbiota of the maternal feces (MF), vaginal fluid (MV), amniotic fluid (AF), and saliva (MS) to the seeding of the meconium microbiota (IF) by using the PacBio SMRT sequencing in combination with the CCS strategy.
Results
Dataset features and alpha-diversity
A total of 1,118,229 of 16S rRNA raw reads were generated from the 192 samples (mean = 5,824; range = 1,257–22,214; SD = 2,891). A total of 858,981 reads were delimited through PyNAST alignment and 100% sequence identity clustering. A total of 241,042 different OTUs (1,255 different OTUs/sample) were identified at 98.65% sequence similarity.
The Shannon diversity curves leveled off, suggesting that the sequencing depth was enough to capture a representative microbial diversity (Fig. S1a). The values of Shannon diversity indexes varied greatly between groups (meconium, 6.92 ± 0.75; amniotic fluid, 4.68 ± 1.94; maternal feces, 7.77 ± 0.91; vaginal fluid; 3.80 ± 1.75; maternal saliva, 8.11 ± 0.54). Pairwise comparison by Mann–Whitney test on Shannon diversity indexes revealed significant differences between most sample groups (P < .001 in most cases, except between amniotic fluid and vaginal fluid, P < .05; between maternal feces and saliva, P = .09; Fig. S1b). The coverage depth ranking was shown by the rank–abundance curves; a longer OTU ‘tail’ represented higher microbial diversity and richness. The maternal feces samples had apparently longer ‘tail’, whereas the vaginal fluid samples had the shortest ‘tail’ (Fig. S1 c).
Microbiota composition of five sample groups
The microbiota composition of all samples was presented at different taxonomic levels (Fig. S2).
Beta-diversity of the microbiota of the meconium and maternal samples
The difference in the microbiota structure between the meconium and other maternal samples was evaluated by principal coordinate analysis (PCoA) and Bray–Curtis dissimilarity (). Symbols representing maternal saliva clustered distinctly on the weighted and unweighted UniFrac PCoA score plots ()), suggesting that the microbiota structure of the maternal saliva samples was obviously different from that of the other sample types. On the weighted score plot, symbols representing the amniotic fluid, vaginal fluid, and meconium samples located closely, while symbols representing the maternal feces samples formed a distinct cluster (). The results of the PCoA performed based on the unweighted UniFrac distance were slightly different for the meconium and maternal feces samples. Symbols representing the amniotic fluid, vaginal fluid, and maternal feces samples clustered at the lower left quadrant, while symbols representing the meconium samples were distributed distinctly at the upper left quadrant (). Moreover, the meconium samples showed a low intragroup Bray–Curtis dissimilarity (0.6840; contrasting to 0.8214–0.9283 for the maternal samples; ). The intergroup distance between the amniotic fluid and vaginal fluid samples was the smallest (0.8510 versus 0.9743–0.9989 between other groups; )).
Figure 1. Dissimilarity-based multivariate analyses of microbiota communities of different sample types. Score plots of principal coordinates analysis (PCoA) of gut microbiota communities based on (a) weighted and (b) unweighted UniFrac distances. (c) Bray-Curtis dissimilarity matrix calculated based on microbial abundance patterns of operational taxonomic units (OTUs) of different sample types. A smaller value of the dissimilarity index indicates a higher similarity (i.e., not dissimilar) between samples. IF: meconium; AF: amniotic fluid; MF: maternal feces; MS: maternal saliva; MV: vaginal fluid.
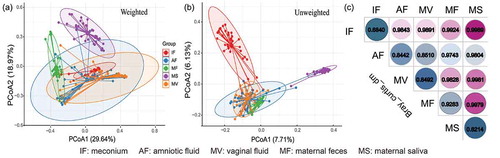
Sample type-specific OTUs
Sample type-specific OTUs (detected exclusively in one sample type) were identified in all sample groups (; Table S2), supporting the existence of a sample type-specific microbiota subset in each sample group. To ensure the sample type specificity, subsequent analyses only included OTUs that were detected in more than two samples within the same sample group. The maternal saliva samples had the most types of sample-specific OTUs (147 types; 146 identified species), followed by the samples of amniotic fluid (98 types; 88 identified species), meconium (82 types; 81 identified species), maternal feces (62 types; 61 identified species), and vaginal fluid (54 types; 53 identified species) (); Table S2). At the species level, the amniotic fluid-maternal feces, amniotic fluid-vaginal fluid, and amniotic fluid-meconium sample pairs shared eleven, three, and two species, respectively ()).
Figure 2. Distribution of sample type-specific operational taxonomic units (OTUs). (a) Types of OTUs that were found exclusively in one sample group. (b) Venn diagram showing common species between sample types, despite they were indeed assigned to different OTUs. The amniotic fluid-maternal feces, amniotic fluid-vaginal fluid, and amniotic fluid-meconium sample pairs shared eleven, three, and two common species, as listed (Table S2 for complete data). This analysis only included OTUs that were detected in more than two samples of the same sample group. IF: meconium; AF: amniotic fluid; MF: maternal feces; MS: maternal saliva; MV: vaginal fluid.
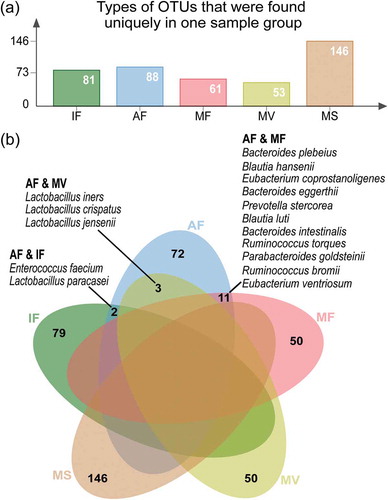
Prediction of the maternal origin of the meconium microbiota by SourceTracker
The SourceTracker software predicted the source of microbial communities in the input sample set.Citation32 In this study, SourceTracker was used to predict the likely origin of the meconium microbiota using the maternal microbiota as potential sources.Citation1 The SourceTracker analysis revealed that 8.03 ± 2.73% of the meconium OTUs matched with the maternal samples (). The 39 maternal-neonate pairs could be classified into 10 groups based on their OTU matching patterns. The meconium and amniotic fluid samples shared the highest level of common OTUs (4.12 ± 1.57%), followed by meconium-vaginal fluid sample pair (2.01 ± 1.14%) and meconium-maternal feces sample pair (1.81 ± 1.04%). The meconium and maternal saliva samples shared significantly fewer common OTUs compared with other sample pairs (0.10 ± 0.05%, P < .01 in all cases).
Table 1. Grouping of maternal-neonate pairs based on patterns of operational taxonomic units (OTUs) sharing between meconium and maternal microbiota.
Prediction of the maternal origin of the meconium microbiota by dyad analysis
To further track the maternal source of the meconium OTUs, dyad analysis was performed by matching the OTU dataset of the meconium microbiota with that of each type of maternal sample (). Consistent with the results generated by the SourceTracker software, the amniotic fluid microbiota shared the highest number of OTU types with the meconium microbiota (18.59 ± 3.22), followed by the microbiota of maternal feces (15.20 ± 3.90), vaginal fluid (7.72 ± 1.45), and maternal saliva (2.03 ± 0.89) ()). Eight meconium genera (Bacillus, Bacteroides, Curvibacter, Escherichia, Faecalibacterium, Lactobacillus, Lactococcus, Streptococcus) were commonly shared across all four types of maternal samples, while 19 meconium genera were in common with two or three types of maternal samples, respectively ()). Eight (shared by meconium and amniotic fluid), 13 (shared by meconium and maternal feces), and one (shared by meconium and maternal saliva) common genera were identified between the microbiota of meconium and only one maternal sample type (); Table S3). The meconium microbiota shared sequences representing 48, 45, 28, and 9 bacterial species with the microbiota of amniotic fluid, maternal feces, vaginal fluid, and maternal saliva, respectively (Table S4).
Figure 3. Prediction of the maternal origin of the meconium microbiota by dyad analysis. (a) Number of shared operational taxonomic units (OTUs) between the meconium microbiota and microbiota of different types of maternal samples. (b) Genus-level phylotypes of meconium OTUs that shared with two to four types of maternal samples. The upper half circle illustrates the distribution of sample sharing of these OTUs, while the lower half circle represents their genus-level phylotypes. The phylotype of each OTU shown in the upper half circle is illustrated by a line connecting to the lower half circle. Each assigned genus is illustrated by a different color, and the number written next to the lower circle represents the number of OTUs of the respective genus. The eight meconium genera listed on the right side were common to all four maternal sample types, while the 19 meconium genera listed on the left side were common to two or three types of maternal samples. (c) Meconium genera that were common to only one type of maternal sample. IF: meconium; AF: amniotic fluid; MF: maternal feces; MS: maternal saliva; MV: vaginal fluid.
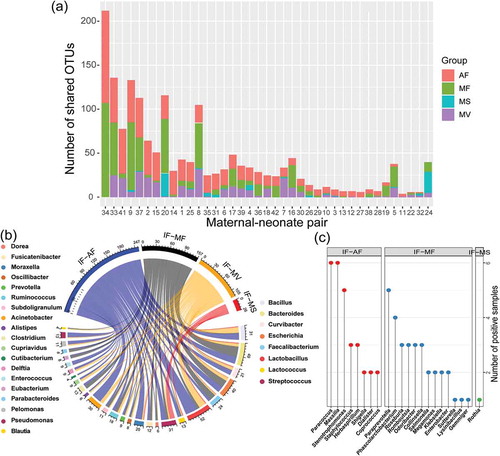
The meconium microbiota of neonates delivered via cesarean section and vaginal birth
The difference in the meconium microbiota structure between neonates delivered by cesarean section and vaginal birth was assessed by PCoA and Bray–Curtis dissimilarity distance. Symbols representing the meconium microbiota of the neonates born by cesarean section and vaginal delivery did not form distinct clustering pattern on the weighted and unweighted UniFrac distance PCoA score plots (Fig. S3a; S3b), suggesting no obvious difference in the meconium microbiota structure between the two groups of neonates. Consistently, the Bray–Curtis dissimilarity of the meconium microbiota of neonates delivered by cesarean section and vaginal birth exhibited no significant difference (P > .05; Fig. S3c). However, the meconium samples of six vaginally delivered neonates (IF8, IF9, IF19, IF20, IF30, and IF34; PCoA1 > 0.2 and <-0.2 on the weighted and unweighted score plots, respectively) showed obvious deviation from other samples. The meconium microbiota of these six samples had significantly more Escherichia fergusonii (62.01% versus 3.22% in other samples; Fig. S3d) and significantly less Bacillus cereus, Bacillus flexus, Bacillus safensis, Lactococcus piscium, Pseudomonas fragi, Oceanobacillus profundus, and Bacillus pumilus (0.01%-0.39% versus 1.2%-27.21% in other samples; Fig. S3d).
The amniotic fluid microbiota of neonates delivered via cesarean section and vaginal birth
Nonmetric multidimensional scaling (NMDS) analysis and Adonis test were performed to evaluate the difference in the amniotic fluid microbiota between neonates delivered by cesarean section and vaginal birth. Symbols representing the amniotic fluid microbiota of the neonates born by cesarean section and vaginal delivery did not form distinct clustering pattern on the NMDS score plot, suggesting no obvious difference in the structure of the amniotic fluid microbiota between the two groups of neonates. The Adonis test also found non-significant difference between the two groups (p = .06; Fig. S4).
Discussion
To explore the seeding of the meconium microbiota of neonates, full-length 16S rRNA-based microbiota profiles of the meconium and maternal samples of 39 maternal-neonate pairs were analyzed. Five types of samples, including maternal feces, vaginal fluid, maternal saliva, and two low microbial biomass samples (meconium and amniotic fluid), were analyzed.Citation16,Citation33 There was no doubt that the former three sample types contained specific microbiota.Citation34 The existence of microbiota in meconium and amniotic fluid was disputable due to detection methodologies and underlying contamination issues. Most previous studies were limited by employing methods that produced short amplicons. The PacBio SMRT platform could generate high-fidelity long reads when used together with the CCS strategy.Citation31 Thus, this study took advantage of the PacBio SMRT platform to improve the taxonomic sensitivity and specificity of microbiota profiling as well as to decrease the risk of false positives.Citation16,Citation35
To minimize the chance of contaminating the low microbial biomass samples, both the amniotic fluid and meconium samples used in this work were collected aseptically within the first couple of hours of birth at the labor ward. The amniotic fluid samples were carefully collected from the mouths of the neonates as soon as they were born. To ensure that the amniotic fluid samples obtained from the vaginally delivered neonates were not contaminated by secondary vaginal microbial contamination during passage through the birth canal, the amniotic fluid samples were aspirated from the back of throat of the neonates. The fact that no significant structural difference was found in the amniotic fluid microbiota between neonates delivered by cesarean section and vaginal birth supported that there was no obvious secondary microbial vaginal contamination. Differing from some previous studies that collected neonates’ fecal samples after several days of birth, this work analyzed the first-pass meconium samples, which represented the fetal gut contents at birth without environmental influences like diet.Citation36 Some reports found that the bacterial signatures of the microbiota of amniotic fluid and placenta were indistinguishable from the negative controls;Citation25,Citation26,Citation37 however, such conclusions were often drawn from analyzing a relatively small sample size. The small sample size might reduce the chance of amplifying scarce DNA in low microbial biomass samples, thus lowering the detection sensitivity and resolution.
Our work detected microbiota in all five sample types; and the microbiota of each group exhibited distinct features, as evidenced by significant differences in both alpha- and beta-diversity. Moreover, sample group-specific OTUs occurring exclusively in one sample type were identified in all sample groups. Indeed many sample group-specific OTUs were detected in multiple samples within the same group. For example, the most frequently detected OTUs in the whole dataset were denovo61553, denovo210693, denovo206472, denovo229606, denovo23827, and denovo190200 of the meconium microbiota, with each of them detected in more than 30 samples. The top four maternal-saliva-specific OTUs (each detected in >20 samples) were denovo227715, denovo105413, denovo84664, and denovo178858. Despite the high detection rates of these OTUs, they were found exclusively in the meconium and maternal saliva microbiota, respectively, suggesting high sample specificity. The species-level taxonomic assignment revealed some common species between the sample pairs of amniotic fluid-maternal feces, amniotic fluid-vaginal fluid, and amniotic fluid-meconium. The fact that they were assigned to different OTUs (cutoff at 98.65% dissimilarity) reflected their different origins, albeit grouped under the same species. Although some detected species were associated with multiple human body sites, many of them were indeed highly body site-specific. For example, 47 of the 146 saliva-specific species in the current dataset were reported as representative oral microbes,Citation38 while many other detected species (e.g., Prevotella melaninogenica, Prevotella dentali, and Actinomyces oris) were described as typical oral commensals.Citation39,Citation40 These data supported that each investigated sample type had unique microbiota signatures and a subset of body site-specific microbiota.
Furthermore, our data showed that apart from the amniotic fluid and vaginal fluid microbiota, which shared the same dominant genera (i.e., Lactobacillus and Curvibacter), other sample groups comprised specific dominant taxa in their microbiota (Bacillus and Escherichia/Shigella in meconium; Bacteroides and Faecalibacterium in maternal feces; Streptococcus and Prevotella in saliva). In consistent with some previous studies,Citation15,Citation41,Citation42 most aforementioned genera were typical members of their respective sample sites except that the genus Curvibacter has never been reported in the amniotic fluid and vaginal fluid microbiota. Members of the Curvibacter genus were previously identified in atherosclerotic plaques and were associated with the oral microflora.Citation43 It was shown that oral inoculation of pregnant mice with bacteria could lead to bacterial translocation into the amniotic fluid, placenta, and fetus, supporting a hematogenous bacterial transmission route from the oral cavity and/or gut to the intrauterine environment.Citation8,Citation44 Moreover, oral, gut, and vaginal bacteria might translocate to the intrauterine environment under maternal gestational stress.Citation44
The meconium microbiota was characterized by a high overall relative abundance of Bacillus sequences (59.76%; detected in 38/39 samples), contrasting to 3.53% detected by HITChip microarray in a previous study.Citation41 The dominance of Bacillus sequences could be an indication of contamination from external environments like the linens in the labor ward and hospital disinfectants,Citation45,Citation46 even though aseptic techniques were strictly observed throughout the sampling process. On the other hand, both the meconium and amniotic fluid samples were collected on the same day under the same environmental conditions, and only few Bacillus sequences were detected in the amniotic fluid microbiota (0.06%; even less for the other three types of maternal samples, representing 0.00%-0.04% of sequences). While the microbiota of most meconium samples comprised numerous Bacillus sequences, six samples were dominated with sequences representing the species Escherichia fergusonii (average of 62.01%), a species previously isolated from meconium samples.Citation41 These observations and the unique microbiota makeup of each sample type argued against the viewpoint of cross contamination due to experimental workflows and/or laboratory reagents. The dominance of sequences representing certain taxa could also be a result of PCR bias when the technique was applied to low microbial biomass samples, leading to preferential amplification of certain sequences in the samples and serious overestimation of these taxa. Thus, further dyad analysis was mainly performed based on identifying common OTU types shared between different samples of the same maternal-neonate pair rather than the absolute quantity or relative abundance of OTUs.
Despite the fact that the maternal saliva samples (8.11 ± 0.54) had a significantly higher Shannon diversity compared with other maternal sample types (3.80 ± 1.75 to 7.77 ± 0.91; P < .05), they had both a lower proportion (saliva: 0.10 ± 0.05%; other three types of maternal samples: 1.81 ± 1.04% to 4.12 ± 1.57%; ) and a lower number (saliva: 2.03 ± 0.89; other three types of maternal samples: 7.72 ± 1.45 to 18.59 ± 3.22) of OTU match with the meconium microbiota compared with the other three types of maternal samples (P < .05). These results suggested that the patterns of OTU match were not purely attributed to chance coincidence. The significantly lower level of matching was likely due to the fact that the buccal cavity was not a body site that related directly with the pregnancy and childbirth processes. Additionally, based on the patterns of OTU match, the 39 maternal-neonate pairs could be classified into 10 groups. Nineteen maternal-neonate pairs showed OTU matches between the microbiota of meconium and three maternal samples (namely amniotic fluid, maternal feces, and vaginal fluid) and no match was found in seven maternal-neonate pairs, suggesting that the patterns of OTU match varied largely between individuals.
Many OTUs in our dataset were commonly shared between the meconium microbiota and microbiota of multiple maternal samples, suggesting that the meconium microbiota was likely originated from multiple maternal sites, which was consistent with the findings of Ferretti et al.Citation11 Nevertheless, some genera were only shared between the meconium microbiota and the microbiota of one maternal sample type. For example, eight genera were exclusively shared between the meconium and amniotic fluid microbiota, while 13 genera were shared only between the meconium and maternal fecal microbiota. Most of these genera were previously detected in the meconium microbiota, including Massilia, Stenotrophomonas, Staphylococcus, Shigella, Dialister, Coprococcus, Phascolarctobacterium, Roseburia, Collinessa, Salmonella, Klebsella, and Enterobacter.Citation34 Moreover, the genera Massilia, Staphylococcus, and Shigella were previously reported in both meconium and amniotic fluid microbiota, while Roseburia, Collinsella, Salmonella, and Enterobacter were reported in both meconium and maternal fecal microbiota.Citation34 No OTU was shared exclusively between the microbiota detected in the meconium and vaginal fluid because all these common taxa overlapped with those shared between the meconium and amniotic fluid microbiota. These included members of seven genera (Acinetobacter, Cupriavidus, Cutibacterium, Delftia, Pelomonas, Lactobacillus, and Pseudomonas), all of which except Cutibacterium were previously detected in the microbiota of meconium and vaginal fluid/amniotic fluid.Citation34 The vaginal fluid samples were collected from the subjects soon before their admission to the delivery ward and rupture of membranes, and the amniotic fluid samples were aspirated from the back of the throat of the neonates. Therefore, the amniotic fluid and vaginal fluid samples had low chance of cross-contamination during sample collection. The close resemblance of the OTU sharing patterns between the amniotic fluid-meconium and vaginal fluid-meconium sample pairs was more likely resulted from the ascending translocation of vaginal bacteria to the amniotic cavity during pregnancy.Citation34,Citation47 Moreover, the fetus swallowed large quantities of amniotic fluid during the later stages of pregnancy, which could lead to a higher similarity between the amniotic fluid and meconium microbiota.Citation48
Hitherto, Stinson et al. have been the only published study that applied PacBio sequencing to describe the microbiota of meconium and amniotic fluid samples of maternal-neonate pairs.Citation16 Large differences were observed in the microbiota composition of the meconium and amniotic fluid samples between this study and Stinson et al. The discrepant results could be due to both an additional PCR decontamination step (using a double-strand specific DNase) in Stinson et al. and natural inter-ethnic/-individual variations in the microbiota composition. The incorporation of such decontamination step could minimize the risk of contamination from reagent kits, but meanwhile increased the chance of accidental removal of template DNA, compromising the microbiota diversity. Nevertheless, Stinson et al. and the current study identified 15 common meconium-associated species and 25 common amniotic fluid-associated species (Table S5), suggesting commonalities in the microbiota composition of samples collected from independent sources. Stinson et al. found that most meconium microbiota contained abundant Pelomonas puraquae sequences, and there was an intense negative correlation between the number of Pelomonas puraquae reads and the propionate level in the meconium samples, strongly suggesting that this species had true biological significance.Citation16 In the current dataset, Pelomonas puraquae sequences were detected in only five amniotic fluid samples, but Pelomonas saccharophila sequences were found in 13 meconium, 28 amniotic fluid, 18 vaginal fluid, three maternal feces, and one saliva samples (Table S6). Ten of the 13 Pelomonas saccharophila sequence-positive meconium samples also had Pelomonas saccharophila sequences in their amniotic fluid and/or vaginal fluid counterparts. Conversely, even Pelomonas saccharophila sequences were detected in three maternal feces and one saliva samples, their meconium counterparts were Pelomonas saccharophila sequence-negative. Our data supported that Pelomonas was a part of the meconium microbiota, and this species might be seeded from the amniotic fluid and/or vaginal tract microbiota.
This work suffered from some limitations. There might be some technical issues like batch effect or PCR bias, particularly when analyzing low microbial biomass samples. For example, the observation of non-significant difference in the structure of the meconium microbiota between neonates born by cesarean section and vaginal birth (except six vaginal cases) could have been a result of batch effect or other underlying technical issues. Another significant limitation was that contaminant and extraction blank controls were not included; thus, this work could not completely rule out the chance of contamination acquired during the experimental workflow.
In conclusion, the facts that some meconium OTUs were also detected in multiple maternal samples and that the meconium microbiota shared more features with the amniotic fluid microbiota than with microbiota of other maternal samples, suggesting that the meconium microbiota was likely seeded from multiple maternal sites and that the amniotic sac contributed more significantly than other investigated maternal body sites in this process.
Materials and methods
Human subjects
Thirty-nine healthy pregnant women (mean age = 28.64 ± 4.13; median age = 28; age range = 22–40), who visited the Department of Gynecology and Obstetrics of the Affiliated Hospital of Inner Mongolia Medical University, were recruited. One hundred ninety-two samples were collected from 39 maternal-neonate pairs, including first-pass meconium (39), maternal feces (39), amniotic fluid (39), vaginal fluid (39), and maternal saliva (36). All neonates were born at full-term (eight delivered by elective cesarean section and 31 delivered vaginally). No subject received any antibiotics during pregnancy. This work was approved by the Ethical Committee of the Affiliated Hospital of Inner Mongolia Medical University, Inner Mongolia, China. Written informed consent was obtained from all participated mothers prior to the study.
Sample collection
All samples were collected in sterile containers having an equal volume of sterile cryoprotectant. First-pass meconium samples (~15 g) were collected on sterilized diapers by the delivery doctors within the first few hours of birth at the labor ward. Samples of maternal feces (~15 g) and saliva (~4 mL) were collected by the mothers within 24 hours before giving birth. Maternal saliva samples were collected with a straw one-hour after meal, and the collected saliva was transferred to a sterile cryotube containing the cryoprotectant. Vaginal fluid samples (~4 mL) were collected from the participants with sterilized cotton swabs by the responsible medical doctors prior to admission to the delivery ward and rupture of membranes. Amniotic fluid samples (~2 mL) were the aspirates collected from the back of the throat of the neonates to minimize the chance of introducing secondary vaginal microbial contamination to the amniotic fluid samples of vaginally delivered neonates. The amniotic fluid samples were aspirated using sterile plastic pipettes by the delivery doctors immediately after birth prior to cord clamping. All samples were stored temporarily in an ice box and were transported promptly to the laboratory at 4°C. Thereafter, the samples were stored at −80°C until further processing.
Metagenomic DNA extraction
All molecular biology works were performed meticulously in a strictly controlled, separate, and sterile workplace. The metagenomic DNA of samples was extracted by the QIAamp Fast DNA Stool Mini Kit (Qiagen GmbH, Hilden, Germany) following the instructions of the manufacturer. Agarose gel electrophoresis and a Nanodrop spectrophotometer were used to ensure the quality of the extracted DNA. Extracted DNA was stored at −20°C until use.
16S rRNA amplification and SMRT sequencing
Full-length 16S rRNA was amplified by PCR with universal primers (27 F, AGAGTTTGATCMTGGCTCAG; 1492 R, ACCTTGTTACGACTT).Citation49 The 5ʹ primer ends were tagged with paired 16-nt symmetric barcodes. The thermocycling program was: 1 cycle of 95°C for 5 min, followed by 28 cycles of 95°C for 1 min, annealing at 58°C for 1 min, and 72°C for 2 min, followed by 1 cycle of 72°C for 10 min. The Agilent DNA 1000 Kit and an Agilent 2100 Bioanalyser (Agilent Technologies) were used for amplicon quantification. Amplicons (2 μg/sample) were used for constructing DNA libraries with the Pacific Biosciences Template Prep Kit 2.0. Sequencing was performed in the CCS mode on a PacBio RS II instrument with the P6/C4 chemistry.Citation29,Citation31
Sequence analyses
Raw data were processed by the protocol RS_ReadsOfinsert.1 (available under the SMRT Portal, version 2.7). The restrictive filter criteria were: (i) minimum full passes of up to 5; (ii) minimum predicted accuracy of 99; (iii) minimum insert read length of 1400; and (iv) maximum insert read length of 1800. Firstly, all reads were classified based on sample-specific primer barcodes. Barcode sequences were removed before extracting high-quality sequences by the Quantitative Insights Into Microbial Ecology package (QIIME; version 1.7). Then, the most abundant sequence of each cluster was selected as a representative to be aligned by PyNAST (100% clustering of sequence identity)Citation50 and UCLUST.Citation51 Afterward, the unique sequences were assigned to OTUs by UCLUST (cutoff at 98.65%).Citation52 Chimeric OTU sequences were screened and removed by ChimeraSlayer.Citation53 The remaining OTUs were taxonomically assigned by the Ribosomal Database Project (RDP) II and Greengenes (version 13_8) databases (minimum bootstrap threshold of 80%).Citation54,Citation55
Alpha- and beta-diversity were calculated from the de novo taxonomic tree constructed by the representative chimera-checked OTU dataset using FastTree.Citation56 To assess the sequencing depth and biodiversity richness, rarefaction curves and rank–abundance curves of all samples were constructed. The Shannon diversity index was calculated. The potential maternal origin of the meconium microbiota was predicted by SourceTracker (version 0.9.5).Citation32 Then, the OTU sharing patterns between the microbiota of the meconium and maternal counterpart samples of each maternal-neonate pair were analyzed. The Venn diagram was created by jvenn.Citation57
Statistical analyses
Statistical analyses were performed mainly with R packages (http://www.r-project.org/). Data were expressed in mean±SD unless otherwise stated. Principal coordinate analysis was performed based on the weighted and unweighted UniFrac distances to evaluate the structural difference in the microbiota between different sample groups.Citation58 The Bray–Curtis dissimilarity matrix, NMDS, and Adonis test were performed by the Vegan package in R. Significant differences between groups were evaluated by Mann-Whitney test or Kruskal–Wallis test at a confidence level of 0.05.
Data availability
Raw sequence data were deposited to the MG-RAST metagenomics database (Project number mgp79730).
Supplemental Material
Download MS Excel (136.5 KB)Supplemental Material
Download MS Word (3.9 MB)Supplementary material
Supplemental data for this article can be accessed here.
Additional information
Funding
References
- Chu DM, Ma J, Prince AL, Antony KM, Seferovic MD, Aagaard KM. Maturation of the infant microbiome community structure and function across multiple body sites and in relation to mode of delivery. Nat Med. 2017;23(3):314–14. doi:10.1038/nm.4272.
- Stewart CJ, Ajami NJ, O’Brien JL, Hutchinson DS, Smith DP, Wong MC, Ross MC, Lloyd RE, Doddapaneni H, Metcalf GA, et al. Temporal development of the gut microbiome in early childhood from the TEDDY study. Nature. 2018;562(7728):583–588. doi:10.1038/s41586-018-0617-x.
- Tanaka M, Nakayama J. Development of the gut microbiota in infancy and its impact on health in later life. Allergol Int. 2017;66:515–522. doi:10.1016/j.alit.2017.07.010.
- Wesemann DR, Portuguese AJ, Meyers RM, Gallagher MP, Cluff-Jones K, Magee JM, Panchakshari RA, Rodig SJ, Kepler TB, Alt FW. Microbial colonization influences early B-lineage development in the gut lamina propria. Nature. 2013;501(7465):112–115. doi:10.1038/nature12496.
- Olszak T, An D, Zeissig S, Vera MP, Richter J, Franke A, Glickman JN, Siebert R, Baron RM, Kasper DL, et al. Microbial exposure during early life has persistent effects on natural killer T cell function. Science. 2012;336(6080):489–493. doi:10.1126/science.1219328.
- Gomez de Aguero M, Ganal-Vonarburg SC, Fuhrer T, Rupp S, Uchimura Y, Li H, Steinert A, Heikenwalder M, Hapfelmeier S, Sauer U, et al. The maternal microbiota drives early postnatal innate immune development. Science. 2016;351(6279):1296–1302. doi:10.1126/science.aad2571.
- Huttenhower C, Gevers D, Knight R, Abubucker S, Badger JH, Chinwalla AT, Creasy HH, Earl AM, FitzGerald MG, Fulton RS, et al. Structure, function and diversity of the healthy human microbiome. Nature. 2012;486:207–214.
- Jiménez E, Fernández L, Marín ML, Martín R, Odriozola JM, Nueno-Palop C, Narbad A, Olivares M, Xaus J, Rodríguez JM. Isolation of commensal bacteria from umbilical cord blood of healthy neonates born by cesarean section. Curr Microbiol. 2005;51(4):270–274. doi:10.1007/s00284-005-0020-3.
- Yassour M, Jason E, Hogstrom LJ, Arthur TD, Tripathi S, Siljander H, Selvenius J, Oikarinen S, Hyöty H, Virtanen SM, et al. Strain-level analysis of mother-to-child bacterial transmission during the first few months of life. Cell Host Microbe. 2018;24(146–154.e4). doi:10.1016/j.chom.2018.06.007.
- Wang J, Zheng J, Shi W, Du N, Xu X, Zhang Y, Ji P, Zhang F, Jia Z, Wang Y, et al. Dysbiosis of maternal and neonatal microbiota associated with gestational diabetes mellitus. Gut. 2018;67(9):1614–1625. doi:10.1136/gutjnl-2018-315988.
- Ferretti P, Pasolli E, Tett A, Asnicar F, Gorfer V, Fedi S, Armanini F, Truong DT, Manara S, Zolfo M, et al. Mother-to-infant microbial transmission from different body sites shapes the developing infant gut microbiome. Cell Host Microbe. 2018;24(133–145.e5). doi:10.1016/j.chom.2018.06.005.
- Ardissone AN, de la Cruz DM, Davis-Richardson AG, Rechcigl KT, Li N, Drew JC, Murgas-Torrazza R, Sharma R, Hudak ML, Triplett EW, et al. Meconium microbiome analysis identifies bacteria correlated with premature birth. PLoS ONE. 2014;9(3):e90784. doi:10.1371/journal.pone.0090784.
- Chu DM, Antony KM, Ma J, Prince AL, Showalter L, Moller M, Aagaard KM. The early infant gut microbiome varies in association with a maternal high-fat diet. Genome Med Internet] 2016;8. [accessed 2017 Dec 14]. http://genomemedicine.biomedcentral.com/articles/10.1186/s13073-016-0330-z.
- Jiménez E, Marín ML, Martín R, Odriozola JM, Olivares M, Xaus J, Fernández L, Rodríguez JM. Is meconium from healthy newborns actually sterile? Res Microbiol. 2008;159(3):187–193. doi:10.1016/j.resmic.2007.12.007.
- Collado MC, Rautava S, Aakko J, Isolauri E, Salminen S. Human gut colonisation may be initiated in utero by distinct microbial communities in the placenta and amniotic fluid. Sci Rep [Internet] 2016;6(1). [accessed 2017 Dec 14http://www.nature.com/articles/srep23129.
- Stinson LF, Boyce MC, Payne MS, Keelan JA. The not-so-sterile womb: evidence that the human fetus is exposed to bacteria prior to birth. Front Microbiol. 2019;10:1124. doi:10.3389/fmicb.2019.01124.
- Aagaard K, Ma J, Antony KM, Ganu R, Petrosino J, Versalovic J. The placenta harbors a unique microbiome. Sci Transl Med. 2014;6(237):237ra65–237ra65. doi:10.1126/scitranslmed.3008599.
- Stout MJ, Conlon B, Landeau M, Lee I, Bower C, Zhao Q, Roehl KA, Nelson DM, Macones GA, Mysorekar IU. Identification of intracellular bacteria in the basal plate of the human placenta in term and preterm gestations. Am J Obstet Gynecol. 2013;208(3):226.e1-226.e7. doi:10.1016/j.ajog.2013.01.018.
- Onderdonk AB, Delaney ML, DuBois AM, Allred EN, Leviton A. Detection of bacteria in placental tissues obtained from extremely low gestational age neonates. Am J Obstet Gynecol. 2008;198(1):110.e1-110.e7. doi:10.1016/j.ajog.2007.05.044.
- Prince AL, Ma J, Kannan PS, Alvarez M, Gisslen T, Harris RA, Sweeney EL, Knox CL, Lambers DS, Jobe AH, et al. The placental membrane microbiome is altered among subjects with spontaneous preterm birth with and without chorioamnionitis. Am J Obstet Gynecol. 2016;214(5):627.e1-627.e16. doi:10.1016/j.ajog.2016.01.193.
- Stout MJ, Conlon B, Landeau M, Lee I, Bower C, Zhao Q, Roehl KA, Nelson DM, Macones GA, Mysorekar IU. Identification of intracellular bacteria in the basal plate of the human placenta in term and preterm gestations. Am J Obstet Gynecol. 2013;208(3):226.e1-7.
- Seferovic MD, Pace RM, Carroll M, Belfort B, Major AM, Chu DM, Racusin DA, Castro ECC, Muldrew KL, Versalovic J, et al. Visualization of microbes by 16S in situ hybridization in term and preterm placentas without intraamniotic infection. Am J Obstet Gynecol. 2019;221(2):146.e1-146.e23. doi:10.1016/j.ajog.2019.04.036.
- Chen C, Song X, Wei W, Zhong H, Dai J, Lan Z, Li F, Yu X, Feng Q, Wang Z, et al. The microbiota continuum along the female reproductive tract and its relation to uterine-related diseases. Nat Commun. 2017;8(1):875. doi:10.1038/s41467-017-00901-0.
- Walther-António MRS, Chen J, Multinu F, Hokenstad A, Distad TJ, Cheek EH, Keeney GL, Creedon DJ, Nelson H, Mariani A, et al. Potential contribution of the uterine microbiome in the development of endometrial cancer. Genome Med. 2016;8(1):122. doi:10.1186/s13073-016-0368-y.
- Rehbinder EM, Lødrup Carlsen KC, Staff AC, Angell IL, Landrø L, Hilde K, Gaustad P, Rudi K. Is amniotic fluid of women with uncomplicated term pregnancies free of bacteria? Am J Obstet Gynecol. 2018;219(3):289.e1-289.e12.
- Lim ES, Rodriguez C, Holtz LR. Amniotic fluid from healthy term pregnancies does not harbor a detectable microbial community. Microbiome. 2018;6(1):87. doi:10.1186/s40168-018-0475-7.
- de Goffau MC, Lager S, Sovio U, Gaccioli F, Cook E, Peacock SJ, Parkhill J, Charnock-Jones DS, Smith GCS. Human placenta has no microbiome but can contain potential pathogens. Nature. 2019;572:329–334.
- Luo C, Tsementzi D, Kyrpides N, Read T, Konstantinidis KT. Direct comparisons of illumina vs. Roche 454 sequencing technologies on the same microbial community DNA sample. PLoS ONE. 2012;7:e30087. doi:10.1371/journal.pone.0030087.
- Mosher JJ, Bernberg EL, Shevchenko O, Kan J, Kaplan LA. Efficacy of a 3rd generation high-throughput sequencing platform for analyses of 16S rRNA genes from environmental samples. J Microbiol Methods. 2013;95:175–181. doi:10.1016/j.mimet.2013.08.009.
- Johnson JS, Spakowicz DJ, Hong B-Y, Petersen LM, Demkowicz P, Chen L, Leopold SR, Hanson BM, Agresta HO, Gerstein M, et al. Evaluation of 16S rRNA gene sequencing for species and strain-level microbiome analysis. Nat Commun. 2019;10:5029. doi:10.1038/s41467-019-13036-1.
- Wagner J, Coupland P, Browne HP, Lawley TD, Francis SC, Parkhill J Evaluation of PacBio sequencing for full-length bacterial 16S rRNA gene classification. BMC Microbiol [Internet] 2016;16. [accessed 2017 Dec 13]. http://bmcmicrobiol.biomedcentral.com/articles/10.1186/s12866-016-0891-4
- Knights D, Kuczynski J, Charlson ES, Zaneveld J, Mozer MC, Collman RG, Bushman FD, Knight R, Kelley ST. Bayesian community-wide culture-independent microbial source tracking. Nat Methods. 2011;8:761–763. doi:10.1080/1040841X.2016.1211088.
- O’Callaghan J, Turner R, Dekker Nitert M, Barrett H, Clifton V, Pelzer E. Re‐assessing microbiomes in the low‐biomass reproductive niche. BJOG Int J Obstet Gynaecol. 2020;127:147–158. doi:10.1111/1471-0528.15974.
- Stinson LF, Payne MS, Keelan JA. Planting the seed: origins, composition, and postnatal health significance of the fetal gastrointestinal microbiota. Crit Rev Microbiol. 2017;43(3):352–369.
- Martínez-Porchas M, Villalpando-Canchola E, Vargas-Albores F. Significant loss of sensitivity and specificity in the taxonomic classification occurs when short 16S rRNA gene sequences are used. Heliyon. 2016;2(9):e00170. doi:10.1016/j.heliyon.2016.e00170.
- Perez-Muñoz ME, Arrieta M-C, Ramer-Tait AE, Walter J. A critical assessment of the “sterile womb” and “in utero colonization” hypotheses: implications for research on the pioneer infant microbiome. Microbiome. 2017;5(1):48. doi:10.1186/s40168-017-0268-4.
- Lauder AP, Roche AM, Sherrill-Mix S, Bailey A, Laughlin AL, Bittinger K, Leite R, Elovitz MA, Parry S, Bushman FD. Comparison of placenta samples with contamination controls does not provide evidence for a distinct placenta microbiota. Microbiome. 2016;4(1):29. doi:10.1186/s40168-016-0172-3.
- Siqueira JF, Rôças IN. The oral microbiota in health and disease: an overview of molecular findings. Methods Mol Biol Clifton NJ. 2017;1537:127–138.
- Ibrahim M, Subramanian A, Anishetty S. Comparative pan genome analysis of oral prevotella species implicated in periodontitis. Funct Integr Genomics. 2017;17(5):513–536. doi:10.1007/s10142-017-0550-3.
- Cavalcanti IMG, Del Bel Cury AA, Jenkinson HF, Nobbs AH. Interactions between Streptococcus oralis, Actinomyces oris, and Candida albicans in the development of multispecies oral microbial biofilms on salivary pellicle. Mol Oral Microbiol. 2017;32(1):60–73. doi:10.1111/omi.12154.
- Moles L, Gómez M, Heilig H, Bustos G, Fuentes S, de Vos W, Fernández L, Rodríguez JM, Jiménez E. Bacterial diversity in meconium of preterm neonates and evolution of their fecal microbiota during the first month of life. PLoS ONE. 2013;8:e66986. doi:10.1371/journal.pone.0066986.
- Grassl N, Kulak NA, Pichler G, Geyer PE, Jung J, Schubert S, Sinitcyn P, Cox J, Mann M. Ultra-deep and quantitative saliva proteome reveals dynamics of the oral microbiome. Genome Med. 2016;8(1):44. doi:10.1186/s13073-016-0293-0.
- Ziganshina EE, Sharifullina DM, Lozhkin AP, Khayrullin RN, Ignatyev IM, Ziganshin AM. Bacterial communities associated with atherosclerotic plaques from Russian individuals with atherosclerosis. Plos One. 2016;11:e0164836. doi:10.1371/journal.pone.0164836.
- Chen HJ, Gur TL. Intrauterine microbiota: missing, or the missing link? Trends Neurosci. 2019;42(6):402–413. doi:10.1016/j.tins.2019.03.008.
- Hsueh PR, Teng LJ, Yang PC, Pan HL, Ho SW, Luh KT. Nosocomial pseudoepidemic caused by bacillus cereus traced to contaminated ethyl alcohol from a liquor factory. J Clin Microbiol. 1999;37:2280–2284. doi:10.1128/JCM.37.7.2280-2284.1999.
- Hosein IK, Hoffman PN, Ellam S, Asseez T-M, Fakokunde A, Silles J, Devereux E, Kaur D, Bosanquet J. Summertime bacillus cereus colonization of hospital newborns traced to contaminated, laundered linen. J Hosp Infect. 2013;85(2):149–154. doi:10.1016/j.jhin.2013.06.001.
- Kim MJ, Romero R, Gervasi MT, Kim J-S, Yoo W, Lee D-C, Mittal P, Erez O, Kusanovic JP, Hassan SS, et al. Widespread microbial invasion of the chorioamniotic membranes is a consequence and not a cause of intra-amniotic infection. Lab Investig J Tech Methods Pathol. 2009;89:924–936. doi:10.1038/labinvest.2009.49.
- Ross MG, Nijland MJ. Fetal swallowing: relation to amniotic fluid regulation. Clin Obstet Gynecol. 1997;40(2):352–365. doi:10.1097/00003081-199706000-00011.
- Lane DJ. 16S/23S rRNA sequencing. In: Stackebrandt E, Goodfellow M, editors. Nucleic acid techniques in bacterial systematics. New York City (NY): John Wiley and Sons; 1991. p. 115–175.
- Caporaso JG, Bittinger K, Bushman FD, DeSantis TZ, Andersen GL, Knight R. PyNAST: a flexible tool for aligning sequences to a template alignment. Bioinform. 2010;26(2):266–267. doi:10.1093/bioinformatics/btp636.
- Edgar RC. Search and clustering orders of magnitude faster than BLAST. Bioinform. 2010;26(19):2460–2461. doi:10.1093/bioinformatics/btq461.
- Kim M, Oh H-S, Park S-C, Chun J. Towards a taxonomic coherence between average nucleotide identity and 16S rRNA gene sequence similarity for species demarcation of prokaryotes. Int J Syst Evol Microbiol. 2014;64(Pt_2):346–351. doi:10.1099/ijs.0.059774-0.
- Haas BJ, Gevers D, Earl AM, Feldgarden M, Ward DV, Giannoukos G, Ciulla D, Tabbaa D, Highlander SK, Sodergren E, et al. Chimeric 16S rRNA sequence formation and detection in sanger and 454-pyrosequenced PCR amplicons. Genome Res. 2011;21(3):494–504. doi:10.1101/gr.112730.110.
- Cole JR, Chai B, Farris RJ, Wang Q, Kulam-Syed-Mohideen AS, McGarrell DM, Bandela AM, Cardenas E, Garrity GM, Tiedje JM. The ribosomal database project (RDP-II): introducing myRDP space and quality controlled public data. Nucleic Acids Res. 2007;35:D169–72. doi:10.1093/nar/gkl889.
- DeSantis TZ, Hugenholtz P, Larsen N, Rojas M, Brodie EL, Keller K, Huber T, Dalevi D, Hu P, Andersen GL. Greengenes, a chimera-checked 16S rRNA gene database and workbench compatible with ARB. Appl Environ Microbiol. 2006;72(7):5069–5072. doi:10.1128/AEM.03006-05.
- Price MN, Dehal PS, Arkin AP. FastTree: computing large minimum evolution trees with profiles instead of a distance matrix. Mol Biol Evol. 2009;26(7):1641–1650. doi:10.1093/molbev/msp077.
- Bardou P, Mariette J, Escudié F, Djemiel C, Klopp C. jvenn: an interactive Venn diagram viewer. BMC Bioinform. 2014;15(1):293. doi:10.1186/1471-2105-15-293.
- Lozupone C, Knight R. UniFrac: a new phylogenetic method for comparing microbial communities. Appl Environ Microbiol. 2005;71(12):8228–8235. doi:10.1128/AEM.71.12.8228-8235.2005.