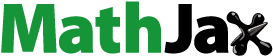
ABSTRACT
Mounting evidence has shown that gut microbiome is associated with various cancers, including gastrointestinal (GI) tract and non-GI tract cancers. But microbiome data have unique characteristics and pose major challenges when using standard statistical methods causing results to be invalid or misleading. Thus, to analyze microbiome data, it not only needs appropriate statistical methods, but also requires microbiome data to be normalized prior to statistical analysis. Here, we first describe the unique characteristics of microbiome data and the challenges in analyzing them (Section 2). Then, we provide an overall review on the available normalization methods of 16S rRNA and shotgun metagenomic data along with examples of their applications in microbiome cancer research (Section 3). In Section 4, we comprehensively investigate how the normalization methods of 16S rRNA and shotgun metagenomic data are evaluated. Finally, we summarize and conclude with remarks on statistical normalization methods (Section 5). Altogether, this review aims to provide a broad and comprehensive view and remarks on the promises and challenges of the statistical normalization methods in microbiome data with microbiome cancer research examples.
Introduction
The advancement of high-throughput sequencing and next generation sequencing (NGS) techniques, including 16S ribosomal RNA (rRNA) and whole shotgun metagenomic sequencing (WSMS), has promoted microbiome study in cancer research.Citation1 It has been shown that the microbiome is associated with various cancers, which not only include gastrointestinal (GI) tract cancers,Citation2,Citation3 such as esophageal cancer,Citation4 gastric cancer,Citation5 colorectal cancers (CRC),Citation6–8 pancreatic cancer,Citation9–11 liver cancer,Citation12–14 but also include non-GI tract cancers such as lung cancer,Citation15 breast cancer,Citation16 prostate cancer,Citation17 melanoma,Citation18,Citation19 and epithelial tumors.Citation20
However, microbiome data analysis is very challenging because microbiome data have some unique characteristics, which often make the results of standard statistical tests/methods to be invalid or misleading. Thus, microbiome data not only need appropriate statistical methods for analysis, but also require normalization prior to analysis. In this review article, we describe some unique characteristics of microbiome data and the challenges in analysis, provide an overall review on the available normalization methods of 16S rRNA and shotgun metagenomic data with examples in microbiome cancer research. We also comprehensively investigate how the normalization methods of 16S rRNA and shotgun metagenomic data along with their accompanying statistical methods are evaluated.
Unique characteristics and challenges for statistical analysis of 16S rRNA and shotgun metagenomic data
Microbiome raw sequence data generally are generated via amplicon sequencing and shotgun metagenomic sequencing techniques. The amplicon sequencing approach only amplifies and sequences one particular gene, often 16S ribosome RNA (rRNA) gene, while shotgun metagenomic sequencing approach assays the collective genome from all the microbial species in a given sample. The 16S rRNA sequencing approach has been described with more details else where.Citation21,Citation22 Here, we introduce a little bit more on the history of shotgun sequencing method before presenting the unique characteristics of microbiome data.
The shotgun sequencing technique was developed to complement traditional phylotyping approaches such as 16S rRNA sequencing approach and genome sequencing of culturable ecosystem members by combining an environment (the “metagenome” of a community).Citation23,Citation24 In 2004, large-scale environmental shotgun sequencing projects were first published.Citation25–27 Shotgun metagenomics studies microorganisms or microbial communities by sequencing DNA fragments directly from samples without any prior cultivation of individual isolates.Citation28 With advancements in shotgun sequencing technologies, this approach very largely facilitates our efforts to investigate the genetic basis of environmental diversity, promising not only to uncover the identity but also the functionality of the most “unculturable” microbial species on earth, and provide insights into our understanding of ecosystem functioning.Citation24 Through using high-throughput sequencing, almost an entire microbial community can be profiled, which enables us to study the unculturable microorganisms in their natural state.Citation29,Citation30
Metagenomic analyses have three basic tasks via taxonomic analysis, functional analysis, and comparative analysis to answer three basic questions: 1) who are they 2) what can they do, and 3) how to compare them? Gene-centric metagenomic analysis quantifies the biological functions (“genes”) present in a metagenome. The generated metagenomic data can be organized in a gene expression matrix or a table of gene counts (observed DNA fragments) with N rows and P columns. The N rows are combinedly referred to as genes, corresponding to bins, typically representing genes, including gene families, functional groups, or single genes. The P columns represent metagenomes from different conditions of samples. Typically, the gene count table is generated through several steps.Citation31,Citation32 Among them, three steps are critical.Citation33: The first step is to assess the quality of the generated sequence DNA fragments (reads). The second step is to align reads to an annotated reference database. The third step is to estimate or bin the abundance of each gene to match each generated sequence read against an annotated reference database.
Microbiome research has expanded rapidly across diverse fields over the past two decades. However, this extremely fast-growing discipline faces a variety of challenges.Citation34–37 In our books and reviewes, we have described some unique characteristics of microbiome data and the challenges in statistical analysis of microbiome data.Citation22,Citation33–35,Citation38,Citation39 Microbiome data, including 16S rRNA and metagenomic shotgun sequencing, have six unique characteristics. They are:
classified into hierarchical taxonomic ranks and encoded as a phylogenetic tree;
multivariate or high dimensional;
compositional;
over-dispersed;
sparse and often have excess zeros (zero-inflated); and
heterogeneous.
Shotgun metagenomics and 16S rRNA studies may have slightly different data characteristics.Citation35,Citation36 Compared to 16S rRNA study, 1) it has even smaller number of samples; 2) it is more plagued by high levels of biological and technical variability; 3) its zero-inflation is more due to under-sampling; 4) its data characteristics is closer to RNA-seq data; and 5) it has more over-dispersion compared to zero-inflation. However, both shotgun metagenomics and 16S rRNA studies face similar challenges.
The unique structure and characteristics of microbiome data pose major challenges on data integration and statistical analysis. At least four challenges in the statistical analysis of microbiome data can be summarized: 1) high-dimensionality causes the large P and small N problem; 2) tree structure and compositionality cause the dependency problem; 3) sparsity with excess zeros causes the over-dispersion and zero-inflation problems; and 4) heterogeneity challenges data integration, modeling, and meta-analysis.
In summary, 1) the large P and small N, and sparsity problems not only require additional assumptions for accurate inference, but also severely reduce the power for inferencing taxon-taxon or gene-gene association analyses; 2) the compositionality may introduce false positive taxon-taxon or taxa-covariate associations, precluding traditional correlation analysis for the detection of taxon-taxon or gene-gene relationships; and 3) the heterogeneity not only challenges integrating the data, and conducting comparisons of studies and meta-analysis, but also makes interpretability difficult.
Normalization methods for 16S rRNA and shotgun metagenomic data
It is now commonly accepted that preprocessing a normalization step can significantly improve the quality of the downstream analysis, in particular, for the differential gene expression analysis of RNA-seq data and the differential abundance analysis of microbiome data. Various systemic biases exist in microbiome data that impact detecting biological variations between samples, such as variations in sample collection, library preparation, and sequencing process, i.e., uneven sampling depth and sparsity. Normalization is expected to mitigate some of these artifactual biases in the original measurements to enable downstream analysis to accurately compare biological differences. The choice of different normalization methods often leads to a very different result in terms of differential gene expression or microbial abundance analysis. Nevertheless, which normalization method is more appropriate to the fitting data is still arguable.
In early microbiome literature, most normalization and associated statistical methods were adopted from other relevant research fields such as RNA-sequencing and ecology. Around twelve years ago, the term “differential abundance” was coined as a direct analogy to differential expression from RNA-Seq and has been adopted for use in microbiome literature.Citation40–42 Currently, several normalization methods have been developed for 16S rRNA microbiome data, while specifically designed normalization methods for shotgun metagenomics data are rare. Most normalization methods used in shotgun metagenomics data are adopted from other fields of high-dimensional count data, such as RNA-seq data. As a foundation for quantitative analysis, Beszteri et al.Citation43 and Frank and SørensenCitation44 have evaluated the normalization method, “average genome size”, for metagenomics data. Similar to RNA-seq data, after initial quality control steps to account for errors in the sequencing process, microbial community sequencing data is typically organized into a so-called “OTU-table (matrix)” or “ASV-table (matrix)” or feature-table, in which each row presents the OTU (observed counts of clustered sequences: taxon or bacteria types), each column presents the sample (or library), and each cell presents the number of OTU (or taxon) read counts mapped to the OTU (taxon) in the sample. Data normalization and differential abundant analysis typically start with the “OTU-table (matrix)”. Similar to RNA-seq data and 16S rRNA-seq data, normalization in shotgun metagenomic data is the processing of the count matrix of gene abundance data, with each row presenting samples and each column presenting genes. The read counts describe the number of DNA fragments sampled for each gene from a microbial community.
Most normalization methods that are currently used in 16S rRNA-seq and shotgun metagenomic data are adopted from RNA-seq data, which can be summarized into four categories, based on technical or statistical approach as well as the kinds of data that these methods originally target to normalize (): 1) Ecology data-based normalization methods. 2) Traditional normalization methods. 3) RNA-seq data-based normalization methods. 4) Microbiome data-based normalization methods, including a) mitigating over-dispersion normalization; b) mitigating zero-inflation normalization; c) compositionally aware normalization; and d) hybrid-based normalization methods. We remark on the normalization methods for shotgun metagenomic data separately when they are different from the use in 16S rRNA data.
Table 1. Normalization methods in 16S rRNA and shotgun metagenomic data.
Ecology data-based normalization methods
Rarefying as normalization
Rarefying plays the normalization role to adjust the sequencing depth in high-throughput sequencing research. The term rarefying originates in rarefaction.Citation49 Rarefaction in physics refers to the reduction of an item’s density, as the opposite of compression, such as a form of wave decompression. Rarefaction method is a nonparametric resampling technique. In ecology, it was first proposed in 1968 by Howard Sanders to reduce the effect of sample size in diversity measurements.Citation45
Rarefaction allows each sample to generate a line, so-called rarefaction curves, to calculate species richness for a given number of individual samples. These curves plot the number of species as a function of the number of samples. In general, rarefaction curves grow rapidly at first because the most common species are found and then plateau, as only the rarest species remain to be sampled. Thus, the rarefaction method has an advantage: it is dependent on the shape of the species abundance curve rather than the absolute number of specimens per sample.Citation45 However, the individual-based taxon resampling curves have been evaluated as unstable performance:Citation40 Depending on the settings, they could be justified for coverage analysis or species richness estimation,Citation74 or could have worse performance than parametric methods.Citation75
The idea of sampling, without replacement, in ecology’s rarefaction has been adopted by some microbiome researchers. This method has originally been commonly used to repeatedly assess alpha diversity (i.e., species richness) from the sampling effortCitation74,Citation76 and later has been used for the analysis of beta-diversity measures.Citation77,Citation78 Now, most major packages for the analysis of ecology and microbiome data, including mothur,Citation79 QIIME,Citation80 vegan,Citation81 phyloseq,Citation48 and microbiome,Citation82 provide options of rarefying samples for normalization. However, to differentiate from the rarefaction in ecology, and to avoid confusion with rarefaction in physics and ecology, these researchers have intentionally used an alternative name, “rarefy” or “rarefying” or “rarefied counts”, when referring to the normalization procedure/results, instead of rarefaction. For instance, the term “rrarefy” has been used in QIIME,Citation80 the term “rarefy” has been used in the phyloseq package,Citation48 and the term “rarefy” in the microbiome package.Citation82 Microbiome studies adopted the rarefaction method from ecology, but the rarefaction procedure is used in the sense that it randomly subsamples each sample to a common depth. In other words, it is used as an ad hoc procedure to normalize microbiome read counts that have resulted from libraries with widely-differing sizes, such as in QIIME.Citation80
As a normalization step in microbiome studies, rarefaction method has been used not only for alpha diversity analysis, but also prior to beta diversity analysis.Citation77,Citation83,Citation84 The rationale behind the subsampling depth choice is believed to be to strike a compromise between information loss and dataset balance. The researchers who use rarefaction method hope that decreasing the subsampling depth can improve a dataset’s balance, while it could also lead to the suboptimal use of the information contained in the dataset.Citation85 ln microbiome literature, different thresholds of subsampling depth have been used: either the lowest number of sequences produced from any sampleCitation77 or even less,Citation84 or arbitrary depth.Citation83 This approach will remove any taxa or OTUs that are no longer present in any sample after random subsampling. The trade-off between number of samples and the depth of coverage has been evaluated; approximately 100 sequences per sample are generally sufficient to reveal the underlying gradient/beta diversity to obtain good qualities of clustering analysis, compared to the clustering observed when analyzing the complete dataset.Citation86 These results demonstrate that increasing the number of sequences per sample does not necessarily improve the ability to detect ecological patterns.Citation86–88
Aguirre de Cárcer et al.Citation85 conducted a systematic evaluation of different subsampling depths and proposed a strategy for recoding singletons as zeros on the beta diversity measures. It showed that subsampling to the minimum as a normalization strategy did not perform particularly well, with data sets presenting some degree of coverage heterogeneity, while subsampling to the median did have benefit: it either improved the analysis or still retained a larger proportion of the initial sequences, when it had no effect. It also showed that multiple rarefaction with a recoding strategy, which randomly subsamples each sample to the median 100 times and then uses the average, and recodes values lower than 1.01 as zero, substantially improved the resolution of the analyses compared to both the initial and subsampling to the minimum strategies.Citation85 However, McMurdie and Holmes (2014).Citation40 showed that rarefied counts could result in an unacceptably high rate of false positive OTUs and failed to account for over-dispersion, resulting in a systematic bias that increases the Type-I error rate even after correcting for multiple-hypotheses. Thus, “rarefying microbiome data is inadmissible.”
Like in 16S rRNA-seq data, rarefying is also commonly used in shotgun metagenomics.Citation89,Citation90 For a review article, the reader is referred to.Citation91 Rarefying can be implemented in phyloseq package.Citation48 To correct for the different sequencing depth, the use of rarefying was reported in microbiome human breast cancer study,Citation92 CRC study.Citation93–95 and gut microbiome cancer and anti – PD-1 immunotherapy (Gopalakrishnan et al. 2018).Citation20 We will discuss this topic further in Section 4.2.
Traditional normalization methods
In the early development, like adopting rarefying for microbiome research, microbiome researchers adopted and most widely used scaling or size factor normalization methods to normalize both 16S rRNA and shotgun metagenomic microbiome data, including TSSCitation42,Citation66 or proportion,Citation40,Citation46 where normalization is based on scaling, first to estimate a sample-specific factor (size factor), and then using this sample-specific factor to correct the OTU(ASV/gene) abundances.
Total sum scaling (TSS) or proportion
In RNA-seq data, the proportion method was proposed in Bergemann and WilsonCitation96 and evaluated by simulated data from exponential, Poisson, binomial, and normal models as well as real microarray and RNA-seq data. The proportion method averages the sum of the amount of mRNA in the test sample over the total amount of the expressed mRNA, represented by the sum of the test and reference samples.
For RNA-seq literature, TSS normalization has been evaluated to incorrectly bias differential abundance estimatesCitation50,Citation51 because a few genes could be sampled preferentially as the sequencing yield increases in RNA-seq data derived through high-throughput technologies.
For 16S rRNA-seq data, TSS or proportion also has been reviewed to be having limitations, including 1) is not robust to outliers,Citation66 is inefficient to address heteroscedasticity,Citation46 is unable to address over-dispersion, and resulting in a high rate of false positives in differential abundance testing for species.Citation40,Citation46,Citation50,Citation97 This systematic bias increases the Type-I error rate even after correcting for multiple-testing.Citation40 2) TSS relies on the constant-sum constraint; hence it cannot remove compositionality and instead, is prone to create compositional effects, making nondifferential taxa or OTUs appear to be differential.Citation66,Citation98–100 Thus, due to strong compositional effects, TSS has a poor performance in terms of both false discovery rate (FDR) control and statistical power at the same false positive rate, compared to other scaling or size factor-based methods such as GMPR and RLE.Citation66 3) Especially, due to the nature of compositionality, proportions are criticized resulting in spurious correlations when comparing the abundance of specific OTUs relative to other OTUs.Citation101
Actually, like rarefying, TSS or proportion is built on the assumption that the individual gene or taxon counts in each sample was randomly sampled from the reads in multiple samples. Thus, counts can be divided by the total library size to convert to proportion, and gene expression analysis or taxon abundance analysis can be fit via a Poisson distribution. Since in both RNA-seq data and 16S rRNA-seq data, the assumption cannot meet, the sample proportion approach is inappropriate for the detection of differentially abundant species,Citation40 and should not be used for most statistical analyses.Citation46 However, McKnight et al. (2019)Citation54 demonstrated that although proportions are not suitable for differential abundance testing,Citation40,Citation46,Citation50,Citation97, proportion method is the most suitable method for community-level comparisons using dissimilarity and distance measures because the proportion method can produce the most accurate Bray-Curtis dissimilarities and the subsequent PCoA and PERMANOVA. Thus, they suggested that proportions (preferably) of rarefied data should be generally used for community-level comparisons.Citation54
For shotgun metagenomic data, TSS or total count (TC) method has been evaluated having overall similar or higher performance than median and upper quartile,Citation91 which is inconsistent with the evaluation results in RNA-seq data. Although theoretically, TC can be heavily dominated by the most common genes than the alternatives, median and upper quartile, because the alternatives replace the sum with the 50th or the 75th percentile of the gene count distribution as a scaling factor are more robust. However, Pereira et al.Citation91 did not observe any tendencies that the median or the upper quartile had an overall higher performance than total count.
Total Sum Scaling (TSS) was reported from these microbiome CRC studies,Citation102 and microbiome cancer diagnostic study.Citation103 In another CRC studyCitation104 consensus reads were normalized by converting OTUs for each sample to a percentage of the reads for a given sample. The relative abundance (total-sum-scaled) data were also reported from the gut microbiome and cancer immunotherapy studies.Citation18,Citation105
Log-transformation as normalization
Generally, transformations, including log and power transformations, and variance stabilization normalization (VSN)Citation106,Citation107 have three overlapping goalsCitation108: 1) correct for heteroscedasticity,Citation109 2) convert multiplicative relations into additive relations, and 3) make skewed distributions (more) symmetric. With the common belief that the log transformation can make data conform more closely to the normal distribution,Citation110,Citation111 it is expected to reduce the skewness of the data and the variability due to outliers. Thus, in practice, log transformation is used as a common remedy to deal with skewed data. It is used under the assumption that the log-transformed data have a distribution equal or close to the normal distribution.Citation111
Log transformation has been reviewed having both benefits and drawbacks in metabolomics.Citation112 For example, it is able to convert right-skewed data to be symmetric, to adjust heteroscedasticity, and to transform the relationship of metabolites from multiplication to addition,Citation108,Citation113 and particularly, it can perfectly remove heteroscedasticity for a relative constant standard deviation.Citation109
However, log transformation: 1) cannot handle the zero value because log zero is undefined; 2) it has limited effect on values with a large relative standard deviation, which unfortunately is the usual case in microbiome data; and 3) it tends to inflate the variance of values near zero,Citation114 although it can reduce the large variance of large values.
Log transformation has the general form of log (x, base), with the addition of the shift parameter to compute logarithms with any desired base. In microbiome study, the log2 transformation with adding a small pseudo value is commonly used. Log transformation with the addition of the shift parameter not only cannot help in reducing the variability, but also can be quite problematic to test the equality of means of two samples using log transformation when there are values close to 0 in the samples.
In microbiome literature, it was shownCitation54 that log transformation suppresses large differences in common OTUs while amplifying slight differences in rare OTUs. In other words, log transformation tends to exaggerate the importance of differences among rare OTUs, while suppressing the importance of differences among common OTUs. Therefore, log transformation is generally not recommended for community-level comparisons because it distorts communities and alters species evenness. The log2-normalization method was used to normalize bacterium counts in a microbiome bladder cancer studyCitation115 and the log10-normalized counts were used in this microbiome CRC study.Citation8
RNA-Seq data-based normalization methods
Scaling or size factor normalization methods, most adopted directly from RNA-seq studies, first calculate the fixed values or proportions, called scale factors; then multiply the matrix counts by the scale factors, which is referred to as scaling the counts. The specific effects of the scaling methods are determined by the chosen scaling factors and how they are applied. RNA-seq data-based normalization methods commonly assume that the property of the majority of genes (in microbiome case, OTUs/ASVs/taxa) are not differentially abundant.
Quantile (Q)
Q method matches each lane’s distribution of gene read counts to a reference distribution, defined in terms of parameters quantiles such as simply scale counts within lanes by their median. In other words, similar to rarefying, Q normalization does not use scaling, instead it makes the gene abundance distributions in different samples identical by adjusting their quantiles based on a reference distribution derived by averaging over all the samples.Citation50,Citation56,Citation116 The Q method was evaluated by RNA-seq data in.Citation50,Citation97,Citation117,Citation118 Previously, quantiles has been used to normalize single channel or A-value microarray intensities between arrays.Citation56,Citation57 In package limma, the quantiles method is used to normalize the columns of a matrix to have the same quantiles.Citation119 Q normalization can be implemented in R package by adapting from the algorithm described in Bolstad et al.Citation56 In practice, the median over the quantiles is typically calculated to preserve the discrete structure of the data, and one of the two middle values is randomly selected when the number of samples are even.Citation91 Examples of Q normalization can be found in these CRC studies.Citation120
Median (med)
In RNA-seq study, the median (Med) normalization method was proposed as a more robust alternative to the total counts (TC) methodCitation50 to normalize data from RNA-seq experiments. Med calculates the scaling (normalization) factors in a way similar in principle to TC: the total counts are replaced by the median (50th percentile) of genes counts that are nonzero in at least one sample. Thus, the normalized data by Med is expected to be less influenced by most highly abundant genes. Median normalization can be performed via the edgeR package.Citation121 Med has been evaluated by RNA-seq data inCitation50,Citation51,Citation61 and application and evaluation in metagenomic data have been seen inCitation91,Citation122 Median normalization method was reported in microbiome CRC and immunotherapy studies.Citation123,Citation124
Log upper quartile (LUQ)
In RNA-seq studies, LUQ normalization method is typically called as upper quartile (UQ), which was first proposed and evaluated by Bullard et al.Citation50 and also evaluated by Dillies et al.Citation51 Overall, UQ, Med, DESeq-RLE and edgeR-TMM perform similarly on the varied data sets, both in terms of the qualitative characteristics of the normalized data and the results of the differential expression analysis. In microbiome literature, it is either referred to as the upper-quartile log-fold change (UQ-logFC)Citation40 or log upper quartile (LUQ)Citation46 to emphasize its application to log transformation. UQ normalization method estimates the scaling factors based on the 75th percentile of the OTU(ASV/gene) count distribution. When the library composition across samples are different, such as the high-count OTUs(ASVs/genes) or a large number of zero counts present, then differences between the Med and UQ methods are expected.Citation51: Compared to the median method, the UQ normalization is expected to further increase the effects of robustness. In shotgun metagenomic data, UQ normalization method has been evaluated in.Citation91,Citation122 UQ (LUQ) normalization is implemented in the edgeR package.Citation121 Examples of using the UQ normalization method are available from the microbiome lung cancer development studyCitation125 and the breast cancer tumor growth and metastatic progression study.Citation126
Conditional quantile normalization (CQN)
CQNCitation58 was proposed to remove the technical variability in RNA-seq data. The normalization algorithm is presented by a conditional Poisson statistical model to account for both the correction for systematic biases and adjusting for distributional distortions. It assumes that the log gene expression level for gene at a given sample is a random variable and the marginal distribution of the most log gene expression levels are independent and identically distributed across samples. In the conditional Poisson model, the mean parameter of the log gene expression level for the sample gene is modeled with three components: 1) A nondecreasing function to account for the nonlinear fact of count distributions across the different samples; 2) A nondecreasing function to account for sample-dependent systematic biases. These nondecreasing functions could be smooth functions that can be modeled as (parametric) natural cubic splines; and 3) a log parameter to adjust for the sequencing depth in millions. The use of CQN has two advantages: First, compared to scaling-based normalization methods.Citation50,Citation61,Citation127 such as edgeR-TMM that only can provide more robust estimates of the shift in location, CQN has the advantage of resulting in sample distributions with comparable scales and shapes.Citation58 Since RNA-seq data exist with several sources of unwanted technical variability, such as the differences in cDNA amplification efficiency and other technical artifacts as well as the differences in distribution shapes and scales that persist after accounting for library size, a normalization based on robust estimates of both scales and shapes is important. Second, compared to RPKM normalization,Citation128 its performance shows a strong dependence on fold change and guanine-cytosine content (GC-content). CQN has shown substantial improvement and eliminated the dependence on fold change and GC content. The reason is that RPKM normalization takes gene length into account to normalize genes by dividing by the gene length, and assumes that the effect is considered static and constant for all samples. However, CQN has the disadvantage: it needs a large amount of data for each sample and a parsimonious model to define a stable algorithm. The CQN method can be implemented via the R Bioconductor package cqn. CQN was reported from breast cancer microbiota and host gene expression study to normalize the host gene expression counts.Citation129
Smooth quantile normalization(qsmooth)
qsmoothCitation59 was proposed based on the assumption that each sample should have the same statistical distribution (i.e., have the same shape of distribution) within biological groups (or conditions), but statistical distributions of the samples between the groups may differ. qsmooth takes the approach of normalization in terms of global transformations, generalizing quantile normalization. However, different from other global normalization methods, which assume that the observed variabilities in global properties (e.g., differences in the total, upper quartile or median gene expression, and proportion of differentially expressed genes) are caused by technical reasons and are unrelated to the biology of interest. qsmooth aims to remove both systematic bias and unwanted technical variation in high-throughput data.
qsmooth is a generalized quantile normalization, in which a weight is computed for each quantile by comparing the variability between groups, relative to the total variability between and within groups. The weight is based on the variability between groups. The less variability between groups, the larger the weight toward one, and the quantile is shrunk toward the overall reference quantile. Thus, depending on the biological variability, the weight may vary between 0 and 1 across the quantiles. In one extreme case, when there are no global differences in distributions, i.e., global differences in distributions correspond to differences in biological groups, qsmooth is similar to standard quantile normalization within each biological group, then the weight is zero.
It was demonstratedCitation59 that qsmooth has advantages including
1) preserving global differences in distributions, corresponding to different biological groups, while scaling normalization methods, e.g., UQ, edgeR-TMM, DESeq-RLE (see below) could not well control the variability between distributions within tissues; 2) reducing the root mean squared error (RMSE) of the overall variability across distributions, and particularly, compared to Q normalization, qsmooth normalization preserves the tissue-specificity, whereas Q normalization removes the biologically known tissue-specific expression; and 3) maintaining a better bias-variance tradeoff with an increasing small bias but a tradeoff with a reduction in variance.
However, qsmooth adds pseudo count of 1 to all counts and then performs log 2 transformation of the counts. This transformation approach has a limitation. qsmooth can be implemented via the R package qsmooth. qsmooth was used by combining with the batch effect correction method to remove known batch effects using a bladder cancer gene expression data.Citation59 Another example of using qsmooth in cancer study is available from the colon cancer drug metabolism study.Citation130
Trimmed mean of M-values (edgeR-TMM)
TMM method was proposed based on the hypothesis that most genes are not differential expressed(DE).Citation121 Applying TMM to 16S rRNA-seq and shotgun metagenomic data, the TMM factor is calculated for each sample, with one sample set as a reference sample and the others as test samples to compare the OTU (ASV/taxon/gene) abundances in the samples. For each test sample, the TMM scaling factor is calculated as the weighted trimmed mean of log ratios between each pair of this test and the reference, after excluding the highest OTUs (ASVs/taxa/genes) and the OTUs (ASVs/taxa/genes) with the largest log ratios or log-fold change. By default, 30% of the log fold change and 5% of the mean abundance are trimmed. This minimizes the log fold change between the samples for most OTUs (ASVs/taxa/genes).Citation61,Citation121 Due to the assumption that the majority of OTUs (ASVs/taxa/genes) are not differentially abundant, like the scaling factors in DESeq, the TMM scaling factors are usually around 1. However, different from DESeq, its scaling factors applies to read counts; TMM applies scaling factors to library sizes. The performance of edgeR-TMM has been evaluated by RNA-seq data in,Citation131 by 16S rRNA-seq data in,Citation40,Citation46,Citation54,Citation66 and by shotgun metagenomic data.Citation91 The edgeR-TMM normalization is implemented in the edgeR package,Citation121 which by default, trims 30% of the log fold change and 5% of the mean abundance. The examples of using edgeR-TMM normalization method are from the CRC studyCitation8 and the lung cancer study.Citation132
Relative log expression (DESeq-RLE)
DESeq normalization method is included in the DESeq package, which was proposed based on the hypothesis that most genes are not differential expressed (DE). DESeq calculates the scaling factor for a given sample as the median of the ratio for each gene, its read count over its geometric mean across all samples.Citation127 The calcNormFactors() function in the edgeR packageCitation121 can calculate RLE normalization factors when the setting method = “RLE” and the TMM normalization factors when the setting method = “TMM”. To avoid confusion, these two normalization methods are sometimes referred to as the edgeR-RLE normalization,Citation40 and edgeR-TMM normalization,Citation46 respectively.
The default normalization methods used in edgeR and DESeq are similar. Ander and HuberCitation127 thought that their DESeq normalization method is similar to the relative log expression normalization method (“RLE”), implemented in edgeR and proposed by Robinson and Oshlack.Citation61 To avoid confusion, we call DESeq normalization method as DESeq-RLE. The edgeR and DESeq normalization methods have similar purposes:Citation133 1) both use normalization to adjust for sequencing depths because different depths are sequenced by different libraries, and 2) both use the normalizations as internal procedure, that is, are built in the statistical model as offsets to often ensure that parameters are comparable, while without actually altering the raw read counts.Citation61 However, the default normalization methods in the packages DESeq and edgeR, are different because “edgeR uses the trimmed mean of M values, whereas DESeq uses a relative log expression approach by creating a virtual library that every sample is compared against”, although in practice, “the normalization factors are often similar”.Citation134 Thus, we do not confuse the DESeq-RLE with edgeR-RLE (relative log expression normalization). Actually, the results obtained from the functions calcNormFactors() with method = “RLE” in the edgeR package (edgeR-RLE) and estimateSizeFactorsForMatrix () in the DESeq(2) package are different. Because the function calcNormFactors() works with pre-normalized counts rather than with raw counts,Citation135 RLE in the edgeR package needs to be further normalized to be identical to the DESeq method and to present as DESeq-RLE.Citation136 The following quote from the authors of DESeq can best describe the differences and similarities between these two normalization methods.Citation134:
By default, edgeR uses the number of mapped reads (i.e., count table column sums) and estimates an additional normalization factor to account for sample-specific effects (e.g., diversity);Citation61 these two factors are combined and used as an offset in the NB model. Analogously, DESeq defines a virtual reference sample by taking the median of each gene’s values across samples and then computes size factors as the median of ratios of each sample to the reference sample. Generally, the ratios of the size factors should roughly match the ratios of the library sizes. Dividing each column of the count table by the corresponding size factor yields normalized count values, which can be scaled to give a counts per million interpretation. (see also edgeR’s cpm function)
To estimate these size factors, the second version of DESeq package (DESeq2) offers the same normalization method that is already used in DESeq, called “the median-of-ratios method”.Citation62 But DESeq2 has the advantage to calculate gene-specific normalization factors to account for further sources of technical biases, such as differing dependence on GC content, gene length or the like.Citation62 Thus, in this review article, we name the “Relative Log Expression” normalization that is implemented by default in the DESeq and DESeq2 packages as DESeq-RLE, which is considered equivalent to the DESeq-median-of-ratios method (label as the “median-of-ratios method”). We name the relative log expression normalization (RLE) that implemented in edgeRCitation121,Citation127 as edgeR-RLE to differentiate it from DESeq-RLE.
Like edgeR-TMM, the effective application of DESeq-RLE to 16S rRNA-seq data need to assume most taxa or OTUs are not differentially abundant. Then scaling factors can be calculated by comparing the samples to a reference. However, different from TMM setting one sample in the study as a reference, DESeq-RLE instead uses a pseudo-reference which is calculated for each taxon or OTU by taking the geometric mean of its abundances across all samples. The normalization factors are then calculated as the median of all the ratios between taxon or OTU counts between samples and the reference. Choosing the median is to prevent the large count values of taxa or OTUs from having undue influence on the values of other taxa or OTUs. Then, using the scaled counts for all the taxa or OTUs and assuming a Negative Binomial (NB) distribution, a mean-variance relation is fit. DESeq-RLE through using a log-like transformation in the NB generalized linear model (GLM) to adjust the matrix counts, with expect to that the variance in the taxon or OTU’s counts across samples is approximately independent of its mean.Citation127
Although edgeR-TMM and DESeq-RLE normalization methods are slightly different, one common characteristic of them is that both are used in NB model along with offset to stabilize variance. Thus, DESeq normalization method has been referred to as relevant variance stabilization transformation (DESeqVS).Citation40,Citation46 In summary, the development of normalization methods that were adopted directly from RNA-seq study have two characteristics: 1) Using a stable and robust value such as median or 75% percentile of count as reference; and 2) Using NB model to address over-dispersion.
In RNA-seq data, it was evaluatedCitation51,Citation131 only DESeq-RLE and edgeR-TMM are able to control the false-positive rate while also maintaining the power to detect differentially expressed genes, especially in the presence of high-count genes. In 16S rRNA-seq data, different evaluation conclusions were achieved: on one hand, it was shownCitation40 that DESeq-RLE and edgeR-TMM had better performance than rarefying and proportion methods based on differential abundance analysis. On the other hand, it was shownCitation46,Citation54 that DESeq-RLE and edgeR-TMM had worse performance in community-level comparisons compared to rarefying and proportion methods. The edgeR-TMM and DESeq-RLE methods were also evaluated having better performance by shotgun metagenomic data,Citation91 which is consistent with previous evaluations by RNA-seq dataCitation51 and 16S rRNA-seq count data.Citation40 DESeq-RLE normalization can be performed by calling the estimateSizeFactors() and sizeFactors() functions in the packages DESeqCitation127 and DESeq2.Citation62 DESeq-RLE was reported from the microbiome prostate cancer study,Citation17 and microbiome GI tract cancer studyCitation2, and a microbiome breast cancer study.Citation137
Other library size/subsampling-based normalization methods in RNA-seq data
Other RNA-seq data normalization methods were also adopted into microbiome cancer research.
To remove the data’s heteroskedasticity and to not perform imputation of missing sequences, sometime unaltered reads per kilobase of transcript per million mapped reads (RPKM) or fragments per kilobase of transcript per million mapped reads (FPKM) or counts per million (CPM) or log-count per million (log-CPM) data normalization method via the Voom algorithmCitation138 is used. For example, the count abundance of oral microbiota across samples was normalized to one million counts in an oral microbiome cancer study.Citation139 The gene abundance table was normalized by the FPKM strategy, in which the gene abundance is normalized by the gene size and the number of total mapped reads reported in frequency.Citation20 In RNA-seq and micorbiome literatures, the “reads per kilobase per million” (RPKM)Citation128 normalization was often used.Citation18,Citation105,Citation140
Microbiome Data-Based Normalization Methods
We categorize the microbiome data-based normalization methods into four groups: 1) toward mitigating over-dispersion, 2) toward mitigating zero-inflation, 3) toward mitigating compositionality, and 4) hybrid-based normalization methods.
Normalization methods toward mitigating over-dispersion
Cumulative sum scaling (metagenomeSeq-CSS)
CSS normalizationCitation42 is a normalization method, specifically designed for microbiome data and is implemented via metagenomeSeq package. CSS aims to correct the bias in the assessment of differential abundance, introduced by total-sum normalization. CSS method is to divide raw counts into the cumulative sum of counts, up to a percentile, determined using a data-driven approach so as to capture the relatively invariant count distribution for a data set. In other words, the choices of percentiles are driven by empirical rather than theoretical considerations.
CSS method is an adaptive extension of the quantile normalization method.Citation50 CSS is a bridge of normalization methods of RNA-seq and 16s rRNA-seq data. It adopts both strategies used in RNA-seq study; that is, combining a stable and robust value (by default, 50th percentile is set as the threshold) and a NB model to mitigate over-dispersion of microbiome data. MetagenomeSeq uses a log transformation (log2(yij+1)) followed by correction for zero-inflation, based on a Gaussian mixture model,Citation42 and performs a statistical inference after transformation, using a normal inverse gamma empirical Bayes model to moderate the gene-specific variance estimates.Citation141 Thus MetagenomeSeq functions to address over-dispersion and zero-inflation. Although the CSS method is coupled with a zero-inflated model in metagenomes package, aiming to handle the high number of zero observations encountered in the metagenomic data, the CSS method itself is a normalization method for mitigating over-dispersion of the microbiome data.
In summary, CSS normalization was developed to address the influence of larger microbiome count values. In 16S rRNA data, this method has been evaluated to having several advantages, including: 1) CSS is similar to LUQ, but more flexible than LUQ because it allows a dependent threshold to determine each sample’s quantile divisor.Citation46 Particularly, it showed that 2) CSS, along with GMPR, is overall more robust to sample-specific outlier OTUs than TSS and RNA-seq normalization methods;Citation66 3) CSS has a higher performance compared to similar algorithms on sparse data.Citation67,Citation142; and 4) CSS may have superior performance for weighted metrics, although it is arguable.Citation42,Citation46,Citation143,Citation144 In shotgun metagenomic data, Pereira et al.Citation91 also showed that CSS, along with edgeR-TMM and DESeq-RLE, has a high overall performance for larger group sizes and particularly has a good performance at controlling the FDR, even for highly unbalanced effect. The evaluation results are in line with the overall finding in 16S rRNA-seq that metagenomeSeq CSS performs well when there is an adequate number of biological replicates.Citation40 The high overall performance for larger group sizes may be because CSS optimizes, including genes, to calculate the scaling factor, and hence minimizes the variability. However, CSS has the challenge to determine the percentiles for microbiome data sets with high variable countsCitation66 and has been particularly evaluated to yield a large number of false positives even though metagenomeSeq has a high power with small effect size,Citation71 having among the worst performance for the low group size.Citation91 Thus, Pereira et al.Citation91 strongly suggested that CSS normalization method should only be applied to data sets with sufficiently many samples. CSS normalization is implemented in the metagenomeSeq package.Citation145 Please note that in the most recent version (version 1.34.0), metagenomeSeq prefers to use the wrench normalization (WN) method over CSS. CSS algorithm was used in the CRC study to normalize both gene frequency data and taxonomic data.Citation146,Citation147
Reversed cumulative sum scaling (RCSS)
RCSS.Citation63 was developed as a variant of CSS in shotgun metagenomic data in which the normalization factor is calculated as the sum of all genes with an abundance larger than the median. Like TC, UQ and Q normalization methods, RCSS was evaluated producing highly skewed P-value distribution and hence resulting in biased FDRs when the effect of differentially abundant genes becomes unbalanced between the groups.Citation91 RCSS can be implemented in R using the function colQuantiles() from the matrixStats package and the function sum() over a logical vector. RCSS is included in MetaAnalystCitation148 as one of five normalization methods (i.e., total counts, median, upper quartile, reversed RCSS, and z-score) of data preprocessing for subsequent analysis.
Normalization methods toward mitigating zero-inflation
Ratio approach for identifying differential abundance (RAIDA)
RAIDACitation64 was developed using the ratio between features (OTUs/taxa) in a modified zero-inflated lognormal model to identify differentially abundant features (DA-DAFs) or differentially abundant OTUs (DA-OTUs). As a normalization method, the development of RAIDA was motivated by the undesirable results produced when applying the total sum, mean, or median normalization methods to normalize the large amount of differences in total abundances of DA-DAFs across different conditions. Like edgeR and DESeq, RAIDA assumes that the majority of features (or OTUs) are not differentially abundant, and uses the ratio between the counts of features (or OTUs) in each sample to eliminate possible problems associated with counts on different scales, within and between conditions. Because microbiome data have many zeros and the ratio of zeros is undefined, RAIDA assumes that most zero values are due to undersamplingCitation149 of the microbial community or of insufficient sequencing depth. Thus, RAIDA uses a modified zero-inflated log-normal model to calculate size factors by adding a small number to the observed count of each feature (or OTU) for each sample before computing the ratios. A lognormal distribution is often assumed for non-zero ratios in the compositional data analysis.Citation69 By adding a small number to the observed count, the original ratios of zeros can be fit with a modified lognormal model.
Sohn et al.Citation64 showed that the performance of RAIDA 1) is not affected by the total different abundances of DA-DAFs (DA-OTUs) across different conditions and hence, RAIDA can remove possible problems associated with counts on different scales, within and between conditions; and 2) compared to edgeR-TMM,Citation121 Metastats,Citation41 and metagenomeSeqCitation42 normalization methods, RAIDA performs consistently, powerful for both the balanced and unbalanced conditions, and hence greatly outperforms these existing methods. For example, metagenomeSeq and RAIDA can provide better power to detect the ratio of true positives to positives, but metagenomeSeq could significantly increase FDR in both the balanced and unbalanced conditions. The edgeR-TMM performs best for the balanced conditions in terms of controlling FDR, However, for the unbalanced conditions, as the percent of DAFs increases, RAIDA surpasses edgeR. Another simulation studyCitation66 demonstrated that DESeq2 and RAIDA are overall more robust and powerful than edgeR and metagenomeSeq, and are able to control the FDR, close to the nominal level. In summary, RAIDA is effective at controlling FDR close to the nominal level of 0.05, regardless of the outlier, which confirms that the method is robust. Like DESeq(2) is accompanied with a NB model, RAIDA is accompanied with a moderated t-statistics, which was evaluated as having very low power in detecting differential abundance for small or medium effect sizes and its overall performance is highly sensitive to the library size.Citation71 For example, when effect sizes and library sizes are small, RAIDA underperforms compared to RioNorm2.Citation71 RAIDA was reported to having been used to identify the differentially abundant gut microbes between CRC and healthy samples.Citation150
Geometric mean of pairwise ratios (GMPR)
GMPRCitation66 was specifically proposed for zero-inflated sequencing data such as microbiome sequencing data. As we described above, compared to RNA-Seq data, microbiome sequencing data are more severely over-dispersed and zero-inflated. However, the existing normalization methods, including the traditional and the RNA-seq data-based normalization methods as well as CSS normalization, which was specifically designed for microbiome data, cannot effectively handle over-dispersed and zero-inflated microbiome data. Motivated by the inability of these available normalization methods to normalize microbiome sequencing data, GMPR was particularly developed to normalize zero-inflated count data, with application to microbiome sequencing data and to other sequencing data with excess zeros, such as, single-cell RNA-Seq data.Citation66
GMPR normalization method extends the idea of RLE normalization for RNA-seq data and relies on the same assumption that the large part of count data is invariant in the 16S OTU-count table. The reason that GMPR reverses the order of the first two steps of RLE method is that RLE fails for OTUs with zero values because geometric mean is not well defined for zero. It was demonstratedCitation66 that GMPR outperforms RNA-Seq and CSS normalization methods mainly because it 1) is robust to differential and outlier OTUs, 2) improves the performance of differential abundance analysis and the reproducibility of normalized abundance, and 3) reduces the inter sample variability of normalized abundances. However, GMPR method also has some limitations.Citation66:
Its appropriateness relies on the assumption that most taxa or OTUs in the count data are invariant.Citation66 However, as in RNA-seq data, actually in practice, the assumption may not meet in some 16S rRNA-seq data and the assumption would even be extremely difficult to check,and have been rarely checked.Citation51,Citation151
It is mainly applied to taxon-level analysis of differential abundance and its reproducibility could be improved to identify the “truly” differential taxa under the compositional context.
It is computationally complicated and inefficient for large samples sizes.
GMPR is accompanied by an omnibus test using a zero-inflated negative binomial (ZINB) distribution, which was evaluated as having very low power in detecting differential abundance for small or medium effect sizes, and its overall performance is highly sensitive to the library size.Citation71
It was shownCitation71 that if the study is interested in the absolute abundance counts instead of the relative abundance (i.e., proportion) and when the effect size increases, then it is inappropriate to use the omnibus test because, like DESeq, DESeq2, and metagenomeSeq, it tends to detect more false positives. GMPR is implemented with the GMPR R package. GMPR were reported in an association study of cancer and microbiomeCitation152 and in a study comparing the fecal microbiome of CRC patients and healthy controls as a function of age.Citation153
Wrench normalization (WN)
Wrench normalizationCitation67 was proposed to analyze and correct compositional bias in sparse sequencing count data. Here, compositional bias refers to the bias that occur inherently due to the sequencing process, which produce the taxa abundances to be relative (compositional) rather than absolute. Without the correction of the compositional bias, inference of absolute abundances will be confounded. The WN method belongs to the count-based data normalization approach, which is different from the approach that uses log-ratio family to address the compositionality. We will review the log-ratio-based approach in Section 3.4.3. The WN method was developed based on two assumptions.Citation67: 1) most taxa do not change across conditions/groups; and 2) zero observations in samples that are mainly caused by sequencing technology are correlated with compositional changes. The WN method is used along with a count-based hurdle log-normal distribution model, in which the probabilities of zero values are determined by sample covariates, including the total sequencing depth, and the positive count value is modeled as a log-Gaussian random variable. The mean of log-Gaussian random variable is determined by 1) the chosen log-reference value, 2) log-sample-depth, 3) the log-net fold change relative to reference (the log of sum of group-wise effect, two-way group-sample interaction, a three-way group-sample-taxon interaction random effect), and 4) a noise term.
WN was developed with these backgrounds: On one hand, in library size/subsampling-based approaches (e.g., TC, RPKM, FPKM, CPM), the normalization factors are adjusted by sample depths, but usually the taxon high intra- and inter-group taxon diversity is not adjusted in their general experimental settings via the framework of generalized linear models. On the other hand, the reference-based normalization and robust fold-change estimation approaches such as edgeR-TMM and DESeq methods overcome compositional bias at high sample depths, significantly take account for the taxon high intra- and inter-group taxon diversity, and hence outperform library size-based approaches. However, such techniques were originally developed for bulk RNA-seq data and face major difficulties with sparse 16S rRNA count data.Citation67
Different from existing normalization approaches, WN derives the estimate of compositional correction factor for every sample, based on the ratio of its proportions to that of the reference. The compositional correction factors are estimated to 1) adjust for the taxon-wise values within and across samples, 2) smooth the taxon-wise estimates across samples before deriving the sample-wise factors using an empirical Bayes strategy, which makes the computed factors robust to low sequencing depths and low abundant taxa.
In summary, WN is a reference-based compositional correction method and can be broadly viewed as a generalization of edgeR-TMMCitation61 for zero-inflated data.
However, unlike existing commonly used count-based data normalization techniques, in that, either TMM/DESeq/CSS normalization methods results in loss of information due to ignoring zero values or library size scaling (e.g., unaltered RPKM/CPM), rarefaction/subsampling can only correct the technical bias that is correlated with library size but cannot correct for compositional bias due to sparsity in 16S rRNA-seq count data.Citation67 It was shownCitation67 that the WN method has a better normalization accuracy, leading to reduced false positive calls in differential abundance analysis and improved quality of positive associations to reconstruct precise group-wise estimates and provide rich annotation in discoveries. Especially, it can still offer robust protection against compositional bias for low sequencing depths and low abundant taxa at higher coverages. Thus, it is a better alternative for under-sampling microbiome data.
Overall, the WN method, paired with the zero-inflated log-normal regression, tends to be more accurate and robust to sparsity. Thus, this method has better performance in terms of controlling the FDR while maintaining the higher power. The advantage in this approach may lie in considering zeros, adjusting both the intra- taxon and inter-group taxon variabilities, and incorporating group information to provide more reliable estimates. The benefits of using WN method, paired with the zero-inflated log-normal regression, to analyze sparse 16S rRNA microbiome data have been evaluated in.Citation154,Citation155 However, since this method assumes that most taxa do not change across conditions/study groups, on average, and uses the average of the ratios of relative abundances across taxa as the estimated scaling factor, the WN method would still suffer in the analysis of taxa arising from arbitrary general conditions.Citation67; In other words, it faces challenges when the effect sizes of differentially abundant taxa are too large.Citation156 Additionally, the current version of Wrench approach is not flexible; it only supports two-group comparison and cannot adjust for continuous covariates (e.g., age, time) or cannot normalize data based on continuous covariates.Citation67 A Bioconductor R package Wrench is available for Wrench normalization for sparse count data.Citation157
Normalization methods toward mitigating compositionality
A data is defined as compositional if it contains multiple parts of nonnegative numbers, whose sum is 1Citation69 (p.25) or any constant-sum constraintCitation158 (p.10). That is, compositional data can be represented by a constant sum of real vectors with positive components, such as per unit (proportions), percent, parts per million, and parts per billion. Because compositional components are dependent, which violates the assumption of independence, when applied to the standard statistical methods, usually compositional data are transformed prior to statistical analysis. Compositionally aware transformation can be considered as normalization. Several such transformations have been proposed in literature, including the center log-ratio transform (clr),Citation68,Citation159 additive log-ratio transform (alr),Citation69,Citation159 isometric log-ratio transform (ilr),Citation160 the inter quartile log-ratio (iqlr),Citation161,Citation162 and sequential binary partitions or balance trees,Citation163,Citation164 which uses phylogenetic trees data to guide the ilr transformations. Among them, the transforms clr, alr, and iqlr are adopted into ALDEx2 (clr-and iqlr-based method),Citation162 and ANCOM(alr -based method),Citation98 the two most often used microbiome compositional software, to transform/normalize microbiome data to account for the compositional structure of microbiome data.
clr-transformation and ALDEx2
It was shownCitation162 that the clr-transformation algorithm could be used to analyze next-generation sequencing data, including RNA-seq and microbiome data, and it has been adopted into some software, including ALDEx2(ALDEx).Citation161,Citation165 ALDEx2 was developed to find the differential expression of genes, relative to the geometric mean abundance between two or more groups. There are two benefits of using clr-transformation: 1) It is able to remove the unit-sum constraint of compositional data, hence allowing ratios of components to be analyzed in the Euclidean space; and 2) taking the ratio with respect to the geometric mean of the whole composition leads data to fall into a P-1 hyperplane of P-dimensional Euclidean space, while after the transformation, the data remains in P-dimension. Furthermore, ALDEx2 has been demonstrated as having these advantages: 1) is robust.Citation161; 2) has very high precision in identifying differentially expressed genes (and transcripts) for 16S rRNA data and has high recall, given sufficient sample sizes;Citation166 and 3) has the potential to be generalized for use in any type of high-throughput sequencing data.Citation167 However, ALDEx2 has several drawbacks, including: 1) It uses a value from valid Dirichlet distribution to replace zero read counts,Citation161 thus it cannot address the zero-inflation problem. 2) The performance of ALDEx2 statistical testing is dependent on transformations; when the log-ratio transformation cannot sufficiently approximate an unchanged reference, it is difficult to interpret the testing results of ALDEx2.Citation168 At this point, the inter-quartile range log-ratio (iqlr) transformation outperforms the centered log-ratio (clr) transformation, and was recommended for use as the default setting for ALDEx2.Citation166 3) Because ALDEx2 performs statistical hypothesis testing using nonparametric methods (Wilcoxon rank sum test or Kruskal-Wallis test for comparisons of two or more than two groups, respectively), it reduces statistical power and hence requires large sample sizes.Citation166,Citation168–171 For example, it was reported that ALDEx2 is difficult to control FDRCitation156 and to maintain statistical power compared to competing differential abundance methods (e.g., ANCOM, ANCOM-BC, edgeR, and DESeq2).Citation156,Citation172 Centered log-ratio transformation was used in the gut microbiome and cancer immunotherapy studiesCitation105,Citation173 to account for the compositional nature of microbial sequencing data.
alr- transformation and ANCOM
ANCOMCitation98 is an alr (additive log-ratio)-based method because it was developed based on alr- transformation, to account for the compositional structure of microbiome data. ANCOM repeatedly uses alr-transformation to choose each of the taxa in the data set as a reference taxon, at a time, and builds its statistical tests on point estimates of transformed OTU counts. After the alr- transformation, the different ratios revealed between the groups can be analyzed by standard statistical tools.Citation174 The use of ANCOM has these benefits: 1) It can well control the FDR while maintaining power comparable to other methods.Citation70,Citation175 2) Especially, compared to RioNorm2 and RAIDA, it has very low FDR (close to 0) and hence is able to detect differential abundant OTUs.Citation71
However, like ALDEx2, in ANCOM, log-ratio transformations play a role in normalization (log-ratio ‘normalizations’). Thus, ANCOM suffers from some similar drawbacks as ALDEx2: 1) Its appropriateness majorly depends on whether the log-ratio transformation sufficiently approximates an unchanged reference.Citation168 The compositional transformed data when using alr, clr, and ilr transformations not only do not improve raw counts tables, but also often make the performance of statistical analysis worse. In other words, the methods that do not require using log-ratio ‘normalizations’ are more appropriate.Citation168 2) Compositional analysis approach cannot address zero-inflation problem; rather it fails in the presence of zero values.Citation176 (p.389). ANCOM could increase the rate of false positives rather than control the FDR due to its improper handling of the zero counts.Citation177 3) The nonparametric nature of compositional analysis approach requires a large number of samples and is underpoweredCitation166,Citation168 as well as it decreases sensitivity on small data sets (e.g., less than 20 samples per group).Citation46,Citation168 Additionally, ANCOM has the following drawbacks: 1) It is computationally intensive to repeatedly apply alr transformation to each taxon in the data set as a reference taxon and it is also a challenge in choosing the reference taxon when the data set has larger number of taxa. 2) No consistent conclusions have been achieved regarding whether ANCOM can control FDR well or not: some studies reported that ANCOM can control FDR reasonably well under various scenarios,Citation46,Citation70 whereas others showed that ANCOM could generate a potentially false-positive result.Citation172 3) ANCOM performs statistical testing for significance using the quantile of its test statistic, W, rather than the P-values, which not only results in the analysis results difficult to interpret,Citation156 but also does not improve the performance of ANCOM by filtering taxa before analysis. ANCOM was reported to have reduced the number of detected differential abundant taxa. This is most likely related to the way that W statistics are calculated and used for significance in ANCOM.Citation178 4) ANCOM does not provide the P-value for individual taxon and cannot provide standard errors or confidence intervals of differential abundance analysis for each taxon.Citation70 5) The differential abundance testing results are difficult to interpret because ANCOM uses presumed invariant features to guide the log-ratio transformation.Citation168 Examples of microbiome cancer studies using ANCOM are available from these reports.Citation179–181
ANCOM-BC
ANCOM-BCCitation70 was proposed for differential abundance analysis (DDA) of microbiome data, with bias correction to ANCOM. ANCOM-BC assumes that 1) the observed taxon abundance is proportional to the unobservable absolute taxon abundance in a unit ecosystem; and 2) the estimation bias is caused by the sampling fraction of variation. ANCOM-BC is a two-stage method that begins with a normalization step and followed by the linear model framework for log-transformed OTU counts data. The goal of ANCOM-BC normalization is to eliminate the differential sampling fractions between each group and allow inferencing the absolute abundance from relative abundance. To address the problem of unequal sampling fractions, it uses a sample-specific offset term to serve as the bias correction.Citation70 The offset term is usually used in the generalized linear models to normalize or adjust for the library size across samples.Citation182 Here,ANCOM-BC uses a sample-specific offset term in a linear regression framework. However, here the sample-specific sampling fractions include both the library size of the corresponding sample and the microbial load in a unit volume of the ecosystem. Thus, in ANCOM-BC, both the library size across samples and the differences in the microbial loads are normalized or adjusted for.
ANCOM-BC has some advantages,Citation70 including: 1) More sample biases are corrected than other library size adjustment approaches. 2) It can well control the FDR while maintaining adequate power, compared to other count-based methods such as edgeR and DESeq2. In RNA-seq studies, the zero-inflated Gaussian mixture model is used in metagenomeSeq.
However, ANCOM-BC also has some weaknesses: 1) It cannot well control the FDR for sample sizes less than 5 per group.Citation70 2) It assumes that the compositional data on the covariates is linear, which is not often true and it cannot control the FDR well when this linear assumption is violated.Citation183 3) In practice, it uses the pseudo-count approach to impute the zeros and excludes those taxa that are associated with structural zeros in the analysis,Citation184 resulting in decreased performance. 4) It has a better FDR control than TSS-based methods. However, it still has low performance under strong compositional effects with Type I error inflation or low statistical power.Citation155 Please note that although ANCOM-BC attempts to provide further scale adjustments for compositionality and sparsity, it uses log- transformation of OTU counts data rather than log-ratio transformation. Thus, its methodology is not a typical compositional data analysis in the sense of Aitchison’s approach.Citation69 To solve the problem of compositionality, it uses the sum of ecosystem as reference to offset the data. This kind of normalization is not the typical Aitchison’s approach-based normalization. ANCOM-BC was reported to compare with other methods, in association with human microbiome, in the colorectal cancer study.Citation185
Hybrid-based normalization methods
In microbiome literature, some kinds of hybrid normalization methods have been developed in terms of explicitly combining or aggregating normalization procedures and statistical analysis modeling.
Network- and zero-inflated model-based normalization(RioNorm2)
The scaling or size factor normalization methods are developed under the assumption that the sampling counts are equivalently distributed up to a certain percentile, and hence the counts on different scales can be adjusted to a common scale using size factors. However, one common limitation of this kind of methods is that it may be ineffective to detect low to medium abundance level of taxa because they could result in false positives, and have lower power to detect taxa with small effect size.
RioNorm2Citation71 was developed along with a two-stage zero-inflated mixture count regression model, aiming to find a group of relatively invariant microbiome species across samples and conditions to construct the size factor for normalization, where the normalized count data is analyzed by a two-stage zero-inflated mixture count regression model: First, OTUs will be divided into two groups, with or without over-dispersion, using a score testCitation186 or bootstrap parametric test.Citation187 Then, OTUs, without and with over-dispersion, will be modeled using zero-inflated Poisson (ZIP) and ZINB distributions, respectively. RioNorm2 method works best in the case of absolute abundance (count) over the relative abundance (i.e., proportion). The benefits of using RioNorm2Citation71 are: 1) It takes account of under-sampling and over-dispersion of data. 2) It consistently yields high power while controlling the FDR, and is robust with small to medium effect size, and different library size and sample size. However, RioNorm2 also has limitations: 1) Like GMPR, since the ratio or log ratio is undefined, RioNorm2 only uses nonzeros in calculating the variance of log-ratio. The appropriateness of excluding zero values is difficult to be evaluated. 2) Only calculating the pairwise dissimilarity among the top abundant OTUs (i.e., the OTUs observed in at least 80% of samples with average count larger than 5 is recommend to be kept for constructing the network of OTUs) is obviously a practical but arbitrary procedure. 3) The fixed threshold value of 0.03th quantile of dissimilarity distribution in search for invariant OTUs for an edge connecting two OTUs needs to be further validated. RioNorm2 is implemented with the R package RioNorm2. The performance of RioNorm2 was demonstrated by a study on microbiome and metastatic melanoma to find the relationship between the microbiome species and the cancer treatment efficiency.Citation19
Cube root(cbrt)-based data normalization and normalization method aggregation
To improve deep neural network (DNN)-based classification of CRC using gut microbiome stool sample data, Mulenga et al.Citation72 proposed a cbrt-based data normalization method and a method that combines a feature extension technique, based on aggregating data normalization methods with data augmentation. The proposed method aims to reduce the effect of dominant features, which is achieved through two steps: 1) It first rescales data points in the data set by multiplying each of them by the standard deviation of the entire raw data set, and then the cube root on the product is computed. 2) It combines the individual normalization methods to extend features of a data set through a data augmentation technique to synthetically produce and combine new samples with the original dataCitation188 and hence to reduce data variability (i.e., variations in the abundance of a specific gene across samples).
It was demonstratedCitation72 that: 1) Aggregation of the normalization methods can improve the classification performance of a DNN model. 2) Combining normalization with data augmentation leverages the strength of the combined normalization methods and provides robust modeling results, although the proposed cbrt method does not outperform other single normalization methods all the time. However, this method also has limitations: 1) It results in significantly increased features in the transformed dataset. The quality is obviously arguable. 2) It also poses the challenge to carefully select the normalization methods to be used in the data transformation technique.Citation72 Therefore, users find it difficult to apply the proposed method to their real data. The proposed cbrt-based data normalization and the normalization method by aggregation were reported to improve CRC classification performance of Deep Neural Network (DNN) algorithms.Citation72 The integration of feature engineering and data augmentation for CRC identification was also discussed in the study on deep learning-based fusion model for biomedical image classification.Citation189
Evaluation of normalization methods in 16S RNA-seq and shotgun metagenomic data
Most normalization methods work best with their accompanying (i.e., built-in) statistical models or tests and their targeting data, while having worse performances using other statistical models or tests or different data. This scenario is a major common limitation of the so far proposed normalization methods. The performances of most normalization methods were evaluated based on visualization effects, by clustering, ordination techniques and differential abundance analysis. Here, we describe how these normalization methods have been evaluated, and comment on the questions behind them.
Is normalization necessary?
Does microbiome data really need to be normalized by an independent procedure? This question is related to another question: whether count-based approach or compositional (relative)-based approach, which are the two largely available approaches in microbiome literature, is more appropriate for analysis of microbiome data? Usually, the compositional (relative)-based approach is preferred to relative-based or compositional methods, such as ALDEX2,Citation162 and ANCOMCitation98 which prefers to use a data transformation or normalization method to normalize microbial taxa abundances. In contrast, the count-based approach, such as edgeR-TMMCitation121 and DESeq-RLE,Citation127 include an offset (a built-in normalization procedure) in their count models to adjust for the library size (sequencing depth). Some count-based methods advocatesCitation190 showed that transforming the microbiome count data could potentially decrease the power in detecting significant taxa.
Is rarefying necessary?
As a normalization procedure, rarefaction resamples an OTU(ASV/gene) table such that all samples have the same sequencing depths. Are corrections for unequal library sizes really needed to be applied? In 16S RNA-seq literature, this topic has been evaluated by McMurdie and Holmes,Citation40 Weiss et al.,Citation46 and McKnight et al.Citation54 However, there is no consensus regarding whether it is appropriate or not to be used in microbiome data. Some researchers still think rarefaction is an effective normalization method.Citation46,Citation54 In 2005, rarefying was first recommended for microbiome counts to attenuate the sensitivity of the UniFrac distanceCitation191 to library size, and later, especially to differences in the presence of rare OTUs.Citation192 In microbiome literature until 2013, microbiome researchers often start their data analysis with an ad hoc library size normalization procedure, so-called rarefying by random subsampling without replacement.Citation40,Citation193–195 Particularly, although in general, both rarefaction and the size factor-based methods (e.g., TSS, edgeR-TMM, DESeq2-RLE, CSS, and GMPR) have their own weaknesses and strengths for particular applications,Citation66 compared to the size factor-based methods, rarefaction is useful and is recommended for alpha- and beta-diversity analysis, especially for unweighted measures and for confounded scenarios, where the sequencing depth correlates with the variable of interest.Citation46,Citation66 The rationale is that most taxa in microbiome data are low abundant and their presence/absence strongly depends on the sequencing depth; in such cases, rarefaction can ensure comparison of alpha- and beta-diversity on an equal basis. In contrast, the size factor-based normalization cannot handle this issue. Other researchersCitation54 made similar points and recommended that community-level comparisons should generally use proportions (preferably) or rarefied data rather than use methods for variance stabilization transformations (e.g., UQ, CSS, edgeR-TMM, and DESeq-VS) because these methods involve a log transformation that distort communities and alter species evenness.
However, other researchers do not advocate using rarefaction as a normalization procedure.Citation48,Citation66 Rarefying procedure throws away sequences from the larger libraries so that all have the same, smallest size, which not only often discards samples that can be accurately clustered by other methods, but also results in a high rate of false positives in tests for differentially abundant species across samples.Citation40 Therefore, rarefying biological count data is statistically inadmissible.Citation40 There are several reasons for this: First, rarefying (rarefaction) wastes or loses available valid data but does not equalize variances; instead of inflating the variances in all samples, it adds noise (artificial uncertainty) by random subsampling. Second, rarefying (rarefaction) was previously often used in the macro-ecology and early microbiome studies, where their principal objective is to explore or descriptively compare species or samples from different environmental/biological sources. However, current microbiome study has moved beyond exploratory/descriptive comparison of microbiome samples to multivariate analysis of microbiome, in which sample covariates can be adjusted for sample-wise distance matrices. Thus, as a normalization procedure, “rarefying to even sampling depth” is not necessary or is not important for detecting differential abundance of OTUs/ASVs between different samples. Third, rarefying or using rarefied counts is not optimal for downstream clustering analysis and does not improve the sensitivity and specificity of differential abundance analysis of microbiome data. In summary, rarefying or using rarefied counts was evaluated not only with performance costs in both sample-clustering and differential abundance analysis, but also with increased Type-I and Type-II errors.Citation40
Rarefying has also been commonly used in shotgun metagenomic data.Citation195–197 However, it was shownCitation91 that rarefying had a relatively low performance for normalization of metagenomic gene abundance data, both in terms of true positive rate (TPR), false positive rate (FPR), and the ability to control the FDR, as well as the resultant inflated gene-gene correlation. In contrast, increasing the number of DNA fragments will increase the ability to correctly identify differentially abundant genes (DAGs).Citation91 Therefore, Pereira et al.Citation91 recommended avoiding the use of the rarefying method to correct the differences in sequencing depth for the identification of DAGs.
In summary, although generally rarefying/rarefaction holds promise as a reliable method for comparing microbial diversity or community-level comparisons, when interpreting rarefying, we should consider two points:Citation196 1) rarefaction is used to compare observed richness among samples for a given level of sampling effort rather than attempt to estimate true richness of a community; 2) rarefaction analyses require larger samples; so rarefaction on small samples may yield the incorrect order of the true richness of the sample. Additionally, the concept of rarefying or rarefaction originated in macro-ecology. It may be suitable for exploratory study of macro species, but may be not necessary or equally important in microbiome study. In microbiome study, alpha diversity tends to be less important than beta diversity and functional study. Thus, the methods or models that can address heterogeneity of data and studies to detect core microbial taxa and integrate multi-omics data are more important. Therefore, we weigh generalized models over rarefying or rarefaction to mitigate heterogeneity of data and studies, and recommend using generalized linear mixed models and multivariate analysis for microbiome study.Citation38,Citation198–201
Does variance stabilization matter in normalization?
Mitigating variability matters in normalization
Variability in microbiome study includes variations in the abundance of a specific gene across samples due to data sequencingCitation202 and heterogeneity of studies such as batch effects.Citation203,Citation204 High variability can result in reducing the sensitivity of a model and challenge the data integration and meta analysis to provide convincing analysis results.Citation202,Citation204–206 Thus, mitigating variability via normalization matters.
Negative binomial (NB) versus poisson model?
Rarefying has some rooting in Poisson model, while the question of variance stabilization is directly relevant to whether the Poisson model or the NB model is more appropriate for the microbiome data. The appropriateness of using variance stabilizing transformations to detect DAGs highlights that the dependence between the mean and variance is not trivial. In contrast to the Poisson model, the NB model can use variance stabilizing transformations to decouple the dependence between mean and variance.Citation207
McMurdie and Holmes,Citation40 based on their evaluation results and well-established statistical theory, advocated that microbiome investigations should avoid using rarefying altogether. Instead, they advocated that microbiome investigations should use the relevant variance stabilizing transformations to normalize their microbiome data and should use a hierarchical mixture such as the Poisson-Gamma or Binomial-Beta models to model uncertainty. McMurdie and Holmes’ recommendationCitation48 for the potential of normalization techniques of DESeq2Citation62 and metagenomeSeqCitation42,Citation145 have been recognized and incorporated into QIIME, since version 2.0.Citation46
Rarefying versus variance stabilization transformations?
In 2017, Weiss et al.Citation46 compared six existing normalization methods and differential abundance analyses to raw data (none using any methods) using simulation and real 16S rRNA amplicon sequencing data to evaluate the performance of rarefying and variance stabilization transformations methods. The six evaluated normalization methods are proportion, rarefy, logUQ,Citation50 CSS,Citation42 DESeq-VS(variance stabilization),Citation62,Citation127 and edgeR-TMM(Trimmed Mean by M-Values).Citation121 On one hand, Weiss et al.Citation46 agreed with McMurdie and HolmesCitation48 that 1) when the groups differ substantially, rarefying and most normalization methods are clustering well for ordination metrics, based on the presence or absence; and 2) rarefying results in a loss of sensitivity due to elimination of a portion of available data. On the other hand, Weiss et al.Citation46 concluded that rarefying remains a useful technique for sample normalization because it can cluster samples more clearly, according to the biological origin, and rarefying itself does not increase the FDR in differential abundance testing. Instead, Weiss et al.Citation46 stated that DESeq2 and other alternative methods for variance stabilization transformations are potentially vulnerable to artifacts due to thelibrary size.
The contrast between rarefying and the methods of variance stabilization transformations actually highlights the issues of how to effectively use sample sizes and appropriate statistical models to deal with the high variability of microbiome data. Both rarefying and the variance stabilization transformations methods have limitations. On one hand, discarding data and using a nonparametric test (e.g., Wilcoxon rank-sum test) could lead to false negatives (lower sensitivity).Citation46 The severity of the power decrease caused by rarifying depends upon how much data has been thrown away and how many samples were collected. Thus, a general guideline is needed to guide how to rarefy to obtain the highest depth possibleCitation208 and a justification should be put forth if the samples are discarded. On the other hand, the methods for variance stabilization transformations (e.g., UQ, CSS, edgeR-TMM, and DESeq-VS) relies on log transformations as a mechanism to standardize variances, i.e., usually via a log2 adding a pseudocount. The log transformations are typically used to reduce 1) the skewness of the data and 2) the variability due to the outliers, with the assumption that the log transformation can make data conform more closely to the normal distribution.Citation110,Citation111 Log transformations facilitate data analysis by making multiplicative models additive and perfectly removing heteroscedasticity when the relative standard deviation is constant.Citation109 However, unfortunately, log transformations have at least three disadvantages:Citation209 1) limited effect on values with a large relative standard deviation; 2) cannot deal with the zero value because log zero is undefined; and 3) tend to inflate the variance of values near zeroCitation114 although it can reduce the large variance of large values. In microbiome literature, the log transformation approach is also criticizedCitation46,Citation54 because log transformation cannot stabilize variances or heterogeneity; instead, could result in distorting the Bray-Curtis dissimilarity values to poorly match the original values and hence make the interpretation of data difficult.Citation54 However, in contrast to rarefying, the negative binomial(NB)-based models that are implemented in DESeq2Citation127 and in edgeRCitation121 with RLE normalization were able to accurately and specifically detect differential abundance, regardless of the effect sizes, replicate numbers, and library sizes.Citation40 Thus, overall, we can consider the normalization methods, along with their NB-based models in the packages edgeR and DESeq2, as mitigating over-dispersion normalization methods. These two models and the other over-dispersed models have been applied in the analysis of microbiome data.Citation200,Citation201
Total count versus median or upper quartile?
In RNA-seq gene expression data, total counts (TC) can be heavily dominated by the most common genes. Thus, theoretically median (Med) and upper quartile (UQ) methods are more robust than the TC method, and the gene count distributions with the 50th (Med method) or 75th (UQ method) percentile are often used as a scaling factor to replace the sum of TC. Med and UQ methods also have been evaluated as more robust and hence better than the TC method for the normalization of RNA-seq data.Citation50,Citation97,Citation210
In early microbiome studies, similar data situations have been considered by some microbiome researches, and Med and UQ normalization methods have been often seen applied in microbiome data. However, due to the controversial results that have been already found in RNA-seq literature and the common belief, early in microbiome studies, Pereira et al.Citation91 showed that the TC method not only had overall similar or higher performance than Med and UQ normalization methods but also had an overall higher TPR and a lower FPR. Moreover, when DAGs have unbalanced effects, UQ and quantile-quantile (QQ) methods were unable to control the FDR.Citation91 There are several reasons for the TC method overall outperforming the Med and UQ methods as also the disparity between shotgun metagenomic and RNA-seq data. First, the estimated scaling factors of the TC method, and the Med and UQ methods are very highly correlated;Citation211 thus they have similar overall performance. Second, the differences in the data structure between shotgun metagenomics and transcriptomics, at least partially contribute to the disparity.Citation91 The upper quartile was developed specifically for transcriptomics; thus it is not necessarily suitable for shotgun metagenomic data. Third, the highly biased P-values and hence high FPR in the identification of DAGs for shotgun metagenomics using UQ and QQ normalization methods are due to invalid underlying model assumptions.Citation212 This is because these models do not incorporate gene-specific variability and hence high over-dispersion has been incorrectly interpreted as biological effects.Citation63,Citation213 Thus, the problem of the skewed P-value distributions generated by improper normalization cannot be addressed by replacing the parametric model (e.g., the over-dispersed Poisson model) with a nonparametric or a permutation-based method.Citation91 The above findings were demonstrated by shotgun metagenomic data. More studies are needed to confirm these results by 16S rRNA data because, compared to the shotgun metagenomic data, 16S rRNA data are more over-dispersed and zero-inflated; hence, are less close to RNA-seq data.
Does over-dispersion matter in normalization?
Emphasis on variance stabilization transformations highlights the importance of over-dispersion in normalization matters. The statistical theory of rarefied counts is based on a hypergeometric model,Citation53 which was originally designed to compare a pair of lanes in RNA-seq data, where the individual gene counts in each lane are assumed to follow a hypergeometric distribution and a pair of lanes are compared using a hypergeometric model. In other words, the hypergeometric distribution assumes that the given lane effect will be absent, after accounting for the different total gene counts in each lane. Under the null of absence of a lane effect, the P-values across genes are uniformly distributed and thus a lane effect is the deviation from uniformity. It can be assessed using a QQ-plot, and the gene expression effects can be analyzed via a Poisson model to compare the multiple lanes for a lane effect.Citation53
Poisson model is not appropriate for microbiome data because the microbiome data are over-dispersed and zero-inflated. The original DESeq-VSCitation62,Citation127 and edgeR-TMMCitation121 were proposed in the background of the microarray gene expression study. As discussed above, it is still debatable whether these original NB-based models that were proposed for RNA-seq data are appropriate for microbiome data. However, it is obvious that they are more suitable than rarefying to fit microbiome data because mitigating over-dispersion matters in microbiome data analysis, whereas sample rarefying and proportion approaches are the Poisson based-methods. Thus, whether the sample rarefying and proportion approaches are appropriate is relevant to whether the Poisson model or any other relevant model for modeling uncertainty is appropriate. It is obvious that after removing low-depth samples, including zero counts from sample, the Poisson based-rarefying and NB-based models have no significant difference in clustering, ordination, and differential abundance analysis. However, it does not mean that NB-based models are not better than the Poisson based-rarefying methods.
Through shotgun metagenomic data, Pereira et al.Citation91 evaluated that edgeR-TMMCitation61 and DESeq-RLECitation127 had the overall best performance, both in terms of TPR and FPR. Especially, they had a higher advantage in the unbalanced case, compared to other methods. At the unbalanced DAGs, only edgeR-TMM, DESeq-RLE and metagenomeSeq-CSS were able to correctly control the FDR or only had a moderate bias when the effects of DAGs are lightly or heavily-unbalanced. In addition, both methods estimated the effect size more accurately and showed high correlations of their estimated scaling factors. Compared to DESeq-RLE, edgeR-TMM, in most cases, had overall better performance in terms of slightly higher TPR and lower FPR, making it the highest performing method in this comparison study. The better performance of edgeR-TMM and DESeq-RLE observed in the shotgun metagenomic data is consistent with previous evaluations on RNA-seq dataCitation51 and 16S rRNA-seq count data.Citation40
Two reasons could contribute to the better performance of edgeR-TMM and DESeq-RLE on differential abundance analysis: 1) these two methods do not explicitly adjust for count distributions across samples, while allowing samples to be different in library composition; and 2) these two methods are built on a NB model so that they are able to model over-dispersion of the data, compared to other scaling methods. Although the NB model was criticized for having very low power in detecting differential abundance for small or medium effect sizes and its overall performance is highly sensitive to the library size,Citation71 we think mitigating over-dispersion is the main reason that edgeR-TMM and DESeq-RLE outperform other scaling methods.
Does zero-inflation matter in normalization?
Accounting for zero values in normalization matters in microbiome study.
Microbiome data have many zeros
As we described in Section 2, one of the important and unique characteristics of microbiome data is that microbiome data often have many zeros. Compared to RNA-seq data, microbiome sequencing data are more over-dispersed and zero-inflated.Citation66,Citation201,Citation214 Excess zeros in microbiome taxa abundance, especially at lower taxonomic levels or OUT/ASV counts (e.g., genus and species), result in only a few taxa or OTUs/ASVs, often dominating across samples, and any given taxa or OTUs/ASVs presenting in all samples is rare.Citation36
The issues of zeros are not appropriately addressed in the RNA-seq data-based normalization methods
RNA-seq data-based normalization methods typically calculate the size factor after excluding genes with zero values. For example, edgeR-TMM identifies a trimmed set of genes for each sample without including genes with zero values.Citation61 DESeq-RLE does not well define the geometric means of genes for genes with zeros.Citation127 When the housekeeping genes is used for normalization, only the housekeeping genes with nonzero expression values are considered.Citation215 Thus, using these methods that are specifically designed for normalizing RNA-seq data to microbiome data will exclude OTUs with zero values in calculating the size factor, leading to OTUs with zero values that are not well defined.Citation66 Thus, both edgeR-TMM and DESeq-RLE use only a small fraction of the “ubiquitous genes” to calculate the size factor after excluding the genes with zero values. When the “ubiquitous genes” were defined by excluding those genes with zero values in all samples, and excluding most, if not all, differentially expressed genes,Citation131,Citation215 there is only a small common fraction of data to calculate the size factor. For example, edgeR-TMM sets a reference sample for the size factor calculation, which restricts the size factor calculation to a specific gene set that the reference sample harbors. DESeq-RLE becomes less stable when the gene data become more sparse and even fails if there are no “ubiquitous genes’’. Because the DESeq method sets the negative values resulting from the log-like transformation to zero, it actually ignores many rare species completely.Citation46 Moreover, DESeq normalization method was developed mainly for use with Euclidean metrics. Thus, it was shown that this method does not work well with non-Euclidean measures or ecological metrics (e.g., Bray-Curtis dissimilarity), and will result in misleading results, except when using weighted UniFrac.Citation46 DESeq and edgeR-TMM assumes that most microbes are not differentially abundant, and among those differentially abundant microbes, the amount of increased/decreased abundance is approximately balanced.Citation50 Because microbial environments are extremely variable, DESeq and edgeR-TMM normalization assumptions are likely not appropriate for highly diverse microbial environments. It was shown that edgeR-TMM and DESeq-RLE normalization methods are only able to normalize a small fraction of the available OTUs in real 16S-rRNA data sets.Citation66
In summary, both DESeq and edgeR-TMM normalization methods are not informative and hence are not optimal for microbiome data.
The issues of zeros cannot be appropriately addressed by replacing zeros with a small pseudo-count value
In practice, one convenient way to deal with the zero problem and therefore to avoid the zero-inflation problem is to replace zero with a small pseudo-count value. This practical strategy is often conducted in compositional dataCitation98,Citation143,Citation144,Citation216,Citation217 to ensure log(0) to be defined. Not only in compositional-based approach, other normalization and statistical methods, including DESeq and CSS, logUQ methods, also add a constant or pseudo-count (i.e., one) to the count matrix prior to transformation to avoid log(0) undefined. The pseudo-counts are often generated by a Bayesian model.Citation218 However, this is not an ideal way to address the zero problem, regardless of whether the pseudo-count was directly added or generated by a model. There are several reasons: 1) Actually, the appropriateness of replacing zero with a small pseudo-count value is built on the implicit assumption that all the zeros are due to under-sampling.Citation40 This assumption is one major limitation of this approach because it does not differentiate between structural zeros and sampling zeros.Citation214 2) There is no clear consensus on how to choose the pseudo-count; and hence it is often chosen very arbitrarily. 3) Log transform is nonlinear. Thus, the choice of pseudo-count is sensitive.Citation143,Citation144 4) The clustering results can be highly influenced by the chosen pseudo-counts.Citation143. 5) The Bayesian formulation assumes a Dirichlet-multinomial framework, and hence imposes a negative correlation structure on every pair of taxa.Citation40,Citation46,Citation98,Citation219 In summary, by replacing zero values with pseudo-counts, not only is the appropriateness not guaranteed, but it also ignores interpreting the different zero values of microbiome data in terms of appropriate concepts and sources of zeros. Therefore, we should carefully interpret the analysis results when the zero values are replaced.Citation176
Does compositionality matter in normalization?
Some researchers do not consider that microbiome data are compositional or the compositional effects are attenuated due to its larger numbers of taxa, whereas others really think microbiome data are compositional (details in Section 3.4.3). Here, we summarize three main points regarding the mitigating compositionality matters in normalizing microbiome data:
The traditional normalization methods are not optimal for compositional data. RNA-seq approach assumes that the majority of genes (in the case of microbiome, OTUs/ASVs/taxa) are not differentially abundantCitation51 and the developed models were used to estimate over-dispersion.Citation42 RNA-seq approaches,Citation162 including the DESeq2 methodCitation46 and the traditional proportion normalization,Citation220 have poor performance in the analysis of compositional data due to high FDR.
RNA-seq approach (e.g., DESeq2) was designed to provide increased sensitivity on smaller datasets (<20 samples per group), when the library sizes are larger and/or very uneven; this kind of method tends to increase FDR. And
The practice of manually adding a pseudo-count to the matrix prior to DESeq2 transformation also increases the FDR.Citation46,Citation162
Put together, from the perspective of compositional analysis, microbiome data should be normalized and analyzed by a compositionally aware method such as ANCOM because it is not only very sensitive (for >20 samples per group) but it is also critical to have a good control over FDR and increase its power.Citation46 However, as we reviewed in Section 4.6, compositional-based approach cannot solve the zero problem.
Are different normalization methods needed for 16s rRNA-seq data and shotgun metagenomic data?
Although rare studies have evaluated this topic, it is certain that normalization methods for both 16S rRNA-seq data and shotgun metagenomic data should be evaluated, based on their unique data characteristics. As we reviewed in Section 2, these two kinds of data share unique data characteristics: over-dispersion, sparse with many zeros, and compositionality and heterogeneity. There are also some differences. For example, shotgun metagenomic data may be less over-dispersed and zero-inflated compared to 16S rRNA-seq data, and may be closer to RNA-seq data.
In 2018, Pereira et al.Citation91 evaluated nine normalization methods that are available for high-dimensional count data using the gene abundance data generated by shotgun metagenomic sequencing in terms of: 1) ability to identify differentially abundant genes (DAGs) using an over-dispersed Poisson generalized linear model (OGLM)Citation212,Citation221 and 2) correctly calculating unbiased P-values and controlling the FDR. In general, most normalization methods that have been evaluated by the shotgun metagenomic dataCitation91 have similar performances to previously using RNA-seq data.Citation50,Citation51
In summary, most normalization methods: 1) could satisfactorily normalize metagenomic gene abundance data when the DAGs were equally distributed between the groups, while the performance was substantially reduced when the distribution of DAGs were more unbalanced; 2) had a reduced true positive rate (TPR) and a high false positive rate (FPR) as well as was unable to control the FDR. 3) The relative normalization results also had been majorly impacted by the size of the groups with several methods even underperforming when only few samples were present. Particularly, among the nine normalization methods: 1) The edgeR-TMM and DESeq-RLE overall had the highest performance, which are therefore recommended for the analysis of gene abundance data;2) CSS also showed satisfactory performance when sample sizes are larger; 3) Normalization using quantile-quantile, median and upper quartile, as well as rarefying the data, overall had the lowest performance, resulting in high FPRs and in many cases FPRs reached unacceptable levels. Thus, using quantile-quantile, median, upper quartile, and rarefying to normalize metagenomic gene abundance data was not recommended by Pereira et al.Citation91
Overall, Pereira et al.Citation91 demonstrated that improper methods may result in unacceptably high levels of false positives and hence lead to incorrect biological interpretation. Thus, this study highlighted the importance of selecting the suitable normalization methods in the analysis of data from shotgun metagenomics.
Does evaluation method matter in evaluating the performance of normalization?
To evaluate the performance of normalization, generally five kinds of evaluation methods have been used: 1) receiver operator characteristic (ROC) curve; 2) correlation and association analyses; 3) sample-wise distance or dissimilarity metrics; 4) clustering and ordination techniques; and 5) statistical tests.
ROC curve
ROC curveCitation222,Citation223 is a visual representation of the diagnostic capability of binary classifiers. It is usually used to reveal the sensitivity – true positive rate (TPR) and specificity (1 – false positive rate (FPR)). ROC curve is most often used to evaluate statistical and machine learning methods including normalization methods.
Correlation and association analyses
The performance of normalization methods are also often evaluated by Pearson’s correlation analysisCitation224 and Spearman’s correlation analysis,Citation225 intersample variability,Citation60 Matthews correlation coefficient (MCC),Citation226 and intraclass correlation coefficients (ICC).Citation227
Sample-wise distance or dissimilarity metrics
Sample-wise distance/dissimilarity measures are often used to measure the performance of normalization. Bray-Curtis dissimilarity,Citation228 which has one favorable nature of not requiring equal variances, is most commonly used in microbiome studies. Other distance/dissimilarity metrics also have been used in microbiome studies including binary Jaccard, Euclidean distance to measure each OTU as a dimension, Poisson distance to measure sample-wise distance implemented in the PoiClaClu package,Citation229 mean squared difference of top OTUs implemented in edgeR,Citation121 unweighted UniFrac distance,Citation191 and weighted UniFrac distance.Citation230
Clustering and ordination techniques
Clustering and ordination are used along with sample-wise distance/dissimilarity metrics. The four most commonly used hierarchical clustering analysis (HCA) methods are single linkage,Citation231 complete linkage,Citation232,Citation233 average linkage.Citation234 and Ward hierarchical grouping.Citation235 Among the ordination methods, principal component analysis (PCA),Citation236–238 principal coordinate analysis (PCoA),Citation239 nonmetric multidimensional scaling (NMDS),Citation240–243 and especially PCoA are most often used in microbiome literature.
Statistical tests
Various statistical tests have been used to evaluate normalization methods in various proposed methods for normalization of microbiome data, including parametric Welch t-test implemented in the packages phyloseq and multtest,Citation244 nonparametric Wilcoxon rank-sum test (or Mann-Whitney U test), the differential abundance analysis, and particularly edgeR-exactTest,Citation121,Citation245 DESeq-nbinomTest,Citation127 DESeq2-nbinomWaldTest,Citation62 Voom,Citation138 metagenomeSeq,Citation42,Citation145 ANCOM,Citation98 and multivariate analysis, such as PERMANOVA.Citation246
Usually in microbiome studies, one or more of above five categorical criteria are often combined together to evaluate normalization methods. The effects of normalization from the proposed or compared normalization methods are evaluated based on the performances of standardizing within-sample variance across samples, data separation in clustering and/or in ordination plots between groups and in differential abundance analysis.Citation40,Citation42,Citation46,Citation50,Citation97,Citation247 Although the microbiome normalized data evaluated by different normalization methods have different qualities and could lead to different conclusions on the evaluated normalization methods, and different downstream statistical results could be obtained when using the microbiome data normalized by different normalization methods, some preliminary conclusions on the performance of normalization evaluation can be achieved.
1) overall RNA-seq data-based normalization methods have better performances than ecology data-based and traditional normalization methods. In general, CSS, DESeq-VS, and edgeR-TMM normalization methods overperform the proportions and rarefying in normalizing 16S rRNA-seq data.Citation40 and shotgun metagenomic sequencing data.Citation54
2) due to targeting unique microbiome data characteristics, overall microbiome data-based normalization methods have better performances than RNA-seq data-based as well as ecology data-based and traditional normalization methods. It was shownCitation154 that the specifically designed normalization methods for microbiome data including wrench edgeR-TMM (also using wrench normalization), wrench Hurdle, ANCOM-BC are clustered together but are separated from the methods designed for RNA-Seq data, which are clustered together including CSS, CPM, DESeq-VS. Other studyCitation155 also demonstrated that metagenomeSeq-wrench normalization method had controlled FDR well across settings, while maintaining decent power. This suggests that the normalization methods that are specifically designed to handle specific biases associated with microbiome data is important.
3) any normalization methods including microbiome data-based and RNA-seq data-based normalization methods cannot simultaneously address all the issues caused by microbiome unique data characteristics in terms of controlling FDR while maintaining power and flexibility. None of the normalization methods can address all the challenges of microbiome data. This has been demonstrated in so far the most comprehensive evaluation of microbial differential abundance analysis(DAA) methods.Citation155 This study evaluated most available microbial DAA methods with their default normalization methods including Aldex2, ANCOM-BC, metagenomeSeq, DESeq2, edgeR-TMM, Omnibus test (using GMPR normalization), and RAIDA, and found that none of the DAA methods are able to be simultaneously robust, powerful, and flexible. Here, we briefly summarize some main arguments:Citation155 1) The DAA methods that explicitly address compositional effects including ANCOM-BC, Aldex2, and metagenomeSeq did have reduced false-positive rates, but they are still not optimal due to Type I error inflation or low statistical power. 2) ANCOM-BC, Aldex2, and Omnibus test did outperform the TSS-based methods in FDR control, but their performances are still not satisfactory for strong compositional effects. 3) Both ANCOM-BC and Omnibus test did not work well under a small sample size. 4) Some other methods offered the best FDR control, but at the cost of low power and especially for rare taxa. 5) Most methods failed to control the FDR when the sequencing depths differed cross groups, indicating rarefaction may be still required for these methods.
4) proportions and rarefying methods may be still suitable for conducting comparisons of entire communities, but are not important for microbial function study.The roles that proportions and rarefying methods play in microbiome study are still controversial. For ecological reasons or perspective, and specifically when comparing communities, three measures have been considered as important:Citation54 1) fully standardize reads in using the Bray – Curtis (BC) dissimilarity and other distance and dissimilarity metrics to measure beta diversity; 2) the species evenness; and 3) the community functionality of dominant species.
On one hand, prior to calculating distance or dissimilarity measures, proportions or rarefying may be the most suitable methods for transforming ecological data to produce accurate comparisons among entire communities (i.e., beta diversity).Citation54 On the other hand, the UQ,CSS, edgeR-TMM, and DESeq-VS methods have potential problems in calculating all the above three measures to compare communities.Citation54 Here, three arguments can be summarized: 1) These normalization methods (e.g., UQ, CSS, edgeR-TMM, and DESeq-VS) do not guarantee equal number of reads across samples, which may raise serious concerns about their applicability for community-level comparisons. 2) These normalization methods focus on standardizing the within-sample variance across samples,Citation97,Citation247 which suppress differences in species evenness. 3) these methods relie on log transformations as a mechanism to standardize variances, i.e., usually via a log2 adding a pseudo-count. The behind algorithm is to reduce the effect of highly abundant OTUs so that the effects of rare OTUs (species) can be detected. Given both rare OTUs (species) and the dominant OTUs (species) in an ecological community can play important functional roles, reducing the importance of dominant OTUs (species) and amplifying the importance of rare OTUs(species) may provide misleading insight into the differences among communities. However, as we discussed in above Sections 4.2 and 4.3, microbial function study has been weighted more important than microbial/ecological community analysis, suggesting that detecting whether specific OTUs/taxa differ across groups is a more important topic compared to just describing alpha and beta diversities in current microbiome study.
5) the basic distinction of statistical analysis between count-based and compositional (relative)-based approaches could also lead to the different evaluation results of normalization methods. In general, it is agreed that microbiome data are structured as multiple taxonomic levels and encoded as phylogenetic tree, high-dimensional, sparse, and often have many zeros. However, some microbiome researchers think microbiome data are discrete and real counts should be analyzed using a count-based method, whereas others consider that microbiome data are compositional (relative) and should be analyzed using a compositional (relative)-based approach. When inferencing microbial taxa, both count-based and compositional(relative)-based approaches will face the statistical issues of dependency, sparsity, over-dispersion, and zero-inflation. However, these two approaches weigh the importance of microbiome normalization methods differently for both 16S rRNA sequencing and shotgun metagenomic sequencing data and use different strategies to address these challenges.
The count-based approach advocates consider that the microbiome data are not mainly compositional. Their arguments have been discussed in Xia:Citation36 1) Compared to ecology data, microbiome data usually have large number of taxa and hence have more high dimensions, but the compositional effect on the large diversity of samples is mild or is attenuated if more taxa are included in this study. Thus, the spurious correlation concern due to compositionality that is originated in ecology study is not a big deal. 2) Composition-based approach interprets biological differences based on the ratio of taxa rather than to directly detect which taxa are associated with the outcome of interest. This does not make sense biologically. 3) Microbiome data compositionality may be corrected when the absolute cellular abundances can be estimated as microbiome sequencing data science technologies advance. Overall the issues of overdispersion and zero inflation as well as dependency of taxa are more considered in count-based approach. In the count-based approach, the taxa abundance is normalized using an offset in a standard count model (e.g., zero-inflated and zero-hurdle models) and variations are adjusted via covariates. In contrast, the direct challenges of how to replace the zero values prior to normalization and how to deal with the boundary [0, 1] are mainly faced to be addressed by composition(relative)-based approach.
In summary, several unique characteristics (i.e., multidimensionality, compositionality, sparsity with many zeros, and heterogeneity) exist simultaneously in one microbiome data/study. The real challenges are due to the factor that none of normalization and DAA methods can simultaneously address the issues caused by these unique microbiome data characteristics.
Conclusions and perspectives
Microbiomes are associated with various gastrointestinal (GI) tract/and non-GI tract cancers. However, the challenge is that microbiome data have unique characteristics, which often make standard statistical data analysis methods less effective. Normalizing microbiome data has been evaluated as a necessary step for statistical analysis microbiome data.
Limitations of normalization methods
Microbiome normalization methods were initially adopted from other research fields such as microarray gene expression, RNA sequencing, and ecology studies. Later microbiome researchers and statisticians developed their own specifically designed methods to target microbiome unique data characteristics. Adoption of methods from the well-established fields is a good starting point and often a necessary stage to develop more appropriate methods for microbiome data. But the available methods from other fields (“old bottles”) are not necessarily fitted to fill the new microbiome data (“new wines”). Thus, there is a need to develop new normalization methods appropriate for microbiome unique data.
Currently normalization methods in 16S rRNA-seq and shotgun metagenomic sequencing studies are still few and most normalization methods originated in RNA-seq and microarray studies.
The normalization methods developed in 16S rRNA-seq and shotgun metagenomic studies both have limitations. First, although newly specifically designed methods were proposed to deal with microbiome unique data characteristics (e.g., sparsity, compositionality, over-dispersion, and zero inflation), they are often evaluated on weighing one characteristic over another. The development of methods that can be used to address multiple microbiome unique data characteristics is still challenging. Second, the overall lowest performance of quantile-quantile, median and upper quartile normalization methods, and rarefying in general have been recognized. However, this method and especially rarefying are still advocated by some microbiome researchers. Rarefying originated in rarefaction, in which Poisson modeling and ecology sampling are used. Based on our experience on 16S rRNA-seq and shotgun metagenomics studies, rarefaction on the one hand can facilitate comparisons of alpha- and beta-diversity, but on the other hand results in loss sensitivity due to elimination of a portion of available data. When the normalization methods were applied in cancer research (and also in other research fields), usually the advantages and disadvantages of these methods were not mentioned or recognized. For example, the concept of rarefication was adopted from macro-ecology; however, its limitation was rarely discussed when it was applied. Third, normalization of microbiome data is a complicated topic. Many sources of unwanted variation can affect read counts, such as multiple library preparation protocols, sequencing platforms, sequencing technology (e.g., read length, paired- versus single-end reads). Most currently proposed methods focus on addressing one of the microbiome unique data characteristics and/or within one dataset. The methods that aim to move beyond only adjusting for one simple difference such as the sequencing depth and therefore are able to adjust for other often unknown and more complex effects are expected.
Challenges and future directions
No large differences between microbiome cancer research and other microbiome research fields have been identified so far. We noticed that a few normalization methods were developed using microbiome cancer data. But few studies have clearly described whether or not there are differences between cancer microbiome data and other microbiome data. Therefore, we can expect that normalization methods that were developed in other microbiome data can be applied into microbiome cancer study and the methods that were developed based on microbiome cancer data can also be employed in other microbiome studies. The critical point is that whether these methods are fitted to the microbiome data to address the unique data characteristics such as to mitigate the sparsity, overdispersion and zero inflation, as well as other heterogeneities and unwanted biases.
While the overall normalization methods have been evaluated to be necessary for both 16S rRNA-seq and shotgun metagenomic data and some of the newly specifically designed methods are promising, their applications in real microbiome studies are still challenging. We expect more works to be done for evaluating these available methods and developing new specifically designed methods to target unique characteristics of microbiome data.
However, challenges remain in microbiome research field. On the one hand, much work still needs to be done to characterize microbiome data such as what are the differences and their respective advantages and disadvantages between 16S rRNA and shotgun metagenomic data? What roles will microbiome normalization methods play in 16S rRNA and shotgun metagenomic data? Whether or not different normalization methods are required when microbiome data were generated by 16S rRNA and shotgun metagenomic sequencing techniques? What total number of sequenced reads (or sampling depths) should always be considered when evaluating sequencing data, regardless of method used to generate it?Citation33 Or do16S rRNA and shotgun metagenomic sequencing methods require different sequenced count reads to obtain effective statistical analysis? Generally, compared to the data generated by 16S rRNA sequencing method, the unique data characteristics of shotgun metagenomic data are closer to RNA-seq data. It was reviewedCitation248 that analysis of RNA-seq data does not require “sophisticated normalization.” The question is whether it is true for shotgun metagenomic data? Whether deeper sequencing is better or some levels of sequencing are sufficient for effective statistical data analysis to provide insight into microbiome differences between groups. On the other hand, we are sure that several microbiome unique characteristics simultaneously exist in one dataset; thus one cannot rely on one method to address or correct all the heterogeneities or biases. Hence, integrating strategy may be a good approach such as through accommodating multiple potential normalization methods in microbiome association analysis.Citation249 However, we need to keep in mind that the more appropriate methods should be based on study design and the heterogeneities and biases that specifically dominate the data and hence need to be addressed and corrected.
Consent for publication
The author approved the submission.
Acknowledgement
Thanks to Yuxuan Xia for proofreading this article.
Disclosure statement
No potential conflict of interest was reported by the author.
Additional information
Funding
References
- Hong M, Tao S, Zhang L, Diao L-T, Huang X, Huang S, Xie S-J, Xiao Z-D, Zhang H, RNA sequencing: new technologies and applications in cancer research. J Hematol Oncol 2020, 13 (1), 1–41. doi:10.1186/s13045-020-01005-x
- Ahn H, Min K, Lee E, Kim H, Kim S, Kim Y, Kim G, Cho B, Jeong C, Kim Y, Whole-transcriptome sequencing reveals characteristics of cancer microbiome in Korean patients with GI tract cancer: fusobacterium nucleatum as a Therapeutic target. Microorganisms 2022, 10 (10), 1896. doi:10.3390/microorganisms10101896
- Newsome RC, Yang Y, Jobin C, The microbiome, gastrointestinal cancer, and immunotherapy. J Gastroen Hepatol 2022, 37 (2), 263–272. doi:10.1111/jgh.15742
- Ajayi TA, Cantrell S, Spann A, Garman KS, Leong JM, Barrett’s esophagus and esophageal cancer: links to microbes and the microbiome. PLoS Pathog 2018, 14 (12), e1007384. doi:10.1371/journal.ppat.1007384
- Stewart OA, Wu F, Chen Y, The role of gastric microbiota in gastric cancer. Gut Microbes 2020, 11 (5), 1220–1230. doi:10.1080/19490976.2020.1762520
- Janney A, Powrie F, Mann EH, Host–microbiota maladaptation in colorectal cancer. Nature 2020, 585 (7826), 509–517. doi:10.1038/s41586-020-2729-3
- Yang D, Wang X, Zhou X, Zhao J, Yang H, Wang S, Morse MA, Wu J, Yuan Y, Li S, Blood microbiota diversity determines response of advanced colorectal cancer to chemotherapy combined with adoptive T cell immunotherapy. Oncoimmunology 2021, 10 (1), 1976953. doi:10.1080/2162402X.2021.1976953
- Yang Y, Gharaibeh RZ, Newsome RC, Jobin C, Amending microbiota by targeting intestinal inflammation with TNF blockade attenuates development of colorectal cancer. Nature Cancer 2020, 1 (7), 723–734. doi:10.1038/s43018-020-0078-7
- Riquelme E, Zhang Y, Zhang L, Montiel M, Zoltan M, Dong W, Quesada P, Sahin I, Chandra V, San Lucas A, et al., Tumor microbiome diversity and composition influence pancreatic cancer outcomes. Cell 2019, 178 (4), 795–806.e12. doi:10.1016/j.cell.2019.07.008
- Geller LT, Barzily-Rokni M, Danino T, Jonas OH, Shental N, Nejman D, Gavert N, Zwang Y, Cooper ZA, Shee K, Potential role of intratumor bacteria in mediating tumor resistance to the chemotherapeutic drug gemcitabine. Sci 2017, 357 (6356), 1156–1160. doi:10.1126/science.aah5043
- Chakladar J, Kuo SZ, Castaneda G, Li WT, Gnanasekar A, Yu MA, Chang EY, Wang XQ, Ongkeko WM, The pancreatic microbiome is associated with carcinogenesis and worse prognosis in males and smokers. Cancers 2020, 12 (9), 2672. doi:10.3390/cancers12092672
- Ponziani FR, Bhoori S, Castelli C, Putignani L, Rivoltini L, Del Chierico F, Sanguinetti M, Morelli D, Paroni Sterbini F, Petito V, Hepatocellular carcinoma is associated with gut microbiota profile and inflammation in nonalcoholic fatty liver disease. Hepatology 2019, 69 (1), 107–120. doi:10.1002/hep.30036
- Ren Z, Li A, Jiang J, Zhou L, Yu Z, Lu H, Xie H, Chen X, Shao L, Zhang R, Gut microbiome analysis as a tool towards targeted non-invasive biomarkers for early hepatocellular carcinoma. Gut 2019, 68 (6), 1014–1023. doi:10.1136/gutjnl-2017-315084
- Behary J, Amorim N, Jiang X-T, Raposo A, Gong L, McGovern E, Ibrahim R, Chu F, Stephens C, Jebeili H, et al., Gut microbiota impact on the peripheral immune response in non-alcoholic fatty liver disease related hepatocellular carcinoma. Nat Commun 2021, 12 (1), 187. doi:10.1038/s41467-020-20422-7
- Zheng Y, Fang Z, Xue Y, Zhang J, Zhu J, Gao R, Yao S, Ye Y, Wang S, Lin C, Specific gut microbiome signature predicts the early-stage lung cancer. Gut Microbes 2020, 11 (4), 1030–1042. doi:10.1080/19490976.2020.1737487
- Zhu J, Liao M, Yao Z, Liang W, Li Q, Liu J, Yang H, Ji Y, Wei W, Tan A, Breast cancer in postmenopausal women is associated with an altered gut metagenome. Microbiome 2018, 6 (1), 1–13. doi:10.1186/s40168-018-0515-3
- Liss MA, White JR, Goros M, Gelfond J, Leach R, Johnson-Pais T, Lai Z, Rourke E, Basler J, Ankerst D, et al., Metabolic biosynthesis pathways identified from fecal microbiome associated with prostate cancer. Eur Urol 2018, 74 (5), 575–582. doi:10.1016/j.eururo.2018.06.033
- Gopalakrishnan V, Spencer CN, Nezi L, Reuben A, Andrews M, Karpinets T, Prieto P, Vicente D, Hoffman K, Wei SC, Gut microbiome modulates response to anti–PD-1 immunotherapy in melanoma patients. Sci 2018, 359 (6371), 97–103. doi:10.1126/science.aan4236
- Matson V, Fessler J, Bao R, Chongsuwat T, Zha Y, Alegre M-L, Luke JJ, Gajewski TF, The commensal microbiome is associated with anti–PD-1 efficacy in metastatic melanoma patients. Sci 2018, 359 (6371), 104–108. doi:10.1126/science.aao3290
- Routy B, Le Chatelier E, Derosa L, Duong CP, Alou MT, Daillère R, Fluckiger A, Messaoudene M, Rauber C, Roberti MP, Gut microbiome influences efficacy of PD-1–based immunotherapy against epithelial tumors. Sci 2018, 359 (6371), 91–97. doi:10.1126/science.aan3706
- Xia Y, Sun J, Chen DG, Bioinformatic analysis of microbiome data. Stat Anal Of Microbiome Data With R. Singapore: Springer; 2018. doi:10.1007/978-981-13-1534-3_1
- Xia Y, Sun J, Statistical data analysis of microbiomes and metabolomics. American Chemical Society, 2022.
- Handelsman J, Rondon MR, Brady SF, Clardy J, Goodman RM, Molecular biological access to the chemistry of unknown soil microbes: a new frontier for natural products. Chem Biol 1998, 5 (10), R245–R249. doi:10.1016/S1074-5521(98)90108-9
- Raes J, Foerstner KU, Bork P, Get the most out of your metagenome: computational analysis of environmental sequence data. Curr Opin Microbiol 2007, 10 (5), 490–498. doi:10.1016/j.mib.2007.09.001
- Hallam SJ, Putnam N, Preston CM, Detter JC, Rokhsar D, Richardson PM, DeLong EF, Reverse methanogenesis: testing the hypothesis with environmental genomics. Sci 2004, 305 (5689), 1457–1462. doi:10.1126/science.1100025
- Tyson GW, Chapman J, Hugenholtz P, Allen EE, Ram RJ, Richardson PM, Solovyev VV, Rubin EM, Rokhsar DS, Banfield JF, Community structure and metabolism through reconstruction of microbial genomes from the environment. Nature 2004, 428 (6978), 37–43. doi:10.1038/nature02340
- Venter JC, Remington K, Heidelberg JF, Halpern AL, Rusch D, Eisen JA, Wu D, Paulsen I, Nelson KE, Nelson W, Environmental genome shotgun sequencing of the sargasso sea. Sci 2004, 304 (5667), 66–74. doi:10.1126/science.1093857
- Sharpton TJ, An introduction to the analysis of shotgun metagenomic data. Front Plant Sci 2014, 5, 209. doi:10.3389/fpls.2014.00209
- Schloss PD, Handelsman J, Metagenomics for studying unculturable microorganisms: cutting the gordian knot. Genome Biol 2005, 6 (8), 1–4. doi:10.1186/gb-2005-6-8-229
- Albertsen M, Hugenholtz P, Skarshewski A, Nielsen KL, Tyson GW, Nielsen PH, Genome sequences of rare, uncultured bacteria obtained by differential coverage binning of multiple metagenomes. Nat Biotechnol 2013, 31 (6), 533–538. doi:10.1038/nbt.2579
- Kuczynski J, Lauber CL, Walters WA, Parfrey LW, Clemente JC, Gevers D, Knight R, Experimental and analytical tools for studying the human microbiome. Nat Rev Genet 2012, 13 (1), 47–58. doi:10.1038/nrg3129
- Boulund F, Sjören A, Kristiansson E, Tentacle: distributed quantification of genes in metagenomes. GigaScience 2015, 4 (1), s13742-015-0078–1. doi:10.1186/s13742-015-0078-1
- Xia Y, Sun J, An integrated analysis of microbiomes and metabolomics. Am Chemi Soci 2022.
- Xia Y, Sun J, Hypothesis testing and statistical analysis of microbiome. Genes & Dis 2017, 4 (3), 138–148. doi:10.1016/j.gendis.2017.06.001
- Xia Y, Sun J, Chen D-G, Statistical analysis of microbiome data with R. Springer Singapore: 2018; Vol. 847. doi:10.1007/978-981-13-1534-3
- Xia Y, Chaptereleven - correlation and association analyses in microbiome study integrating multiomics in health and disease. In: Progress in molecular biology and translational science, Sun J, editor Academic Press: 2020; Vol. 171, pp. 309–491. doi:10.1016/bs.pmbts.2020.04.003
- Proctor L, Priorities for the next 10 years of human microbiome research. Nat Publ Group: 2019. 569 7758 623–625 doi:10.1038/d41586-019-01654-0
- Xia Y, Sun J, Bioinformatic and statistical analysis of microbiome data: from raw sequences to advanced modeling with QIIME 2 and R. Springer International Publishing: 2023. doi:10.1007/978-3-031-21391-5
- Xia Y, Correlation and association analyses in microbiome study integrating multiomics in health and disease. Prog Mol Biol Transl Sci 2020, 171, 309–491.
- McMurdie PJ, Holmes S, McHardy AC, Waste not, want not: why rarefying microbiome data is inadmissible. PLoS Comput Biol 2014, 10 (4), e1003531. doi:10.1371/journal.pcbi.1003531
- White JR, Nagarajan N, Pop M, Ouzounis CA, Statistical methods for detecting differentially abundant features in clinical metagenomic samples. PLoS Comput Biol 2009, 5 (4), e1000352. doi:10.1371/journal.pcbi.1000352
- Paulson JN, Stine OC, Bravo HC, Pop M, Differential abundance analysis for microbial marker-gene surveys. Nat Methods 2013, 10 (12), 1200–1202. doi:10.1038/nmeth.2658
- Beszteri B, Temperton B, Frickenhaus S, Giovannoni SJ, Average genome size: a potential source of bias in comparative metagenomics. ISME J 2010, 4 (8), 1075–1077. doi:10.1038/ismej.2010.29
- Frank JA, Sørensen SJ, Quantitative metagenomic analyses based on average genome size normalization. Appl Environ Microb 2011, 77 (7), 2513–2521. doi:10.1128/AEM.02167-10
- Sanders HL, Marine benthic diversity: a comparative study. Am Nat 1968, 102 (925), 243–282. doi:10.1086/282541
- Weiss S, Xu ZZ, Peddada S, Amir A, Bittinger K, Gonzalez A, Lozupone C, Zaneveld JR, Vázquez-Baeza Y, Birmingham A, et al., Normalization and microbial differential abundance strategies depend upon data characteristics. Microbiome 2017, 5 (1), 27. doi:10.1186/s40168-017-0237-y
- Colwell RK, Chao A, Gotelli NJ, Lin S-Y, Mao CX, Chazdon RL, Longino JT, Models and estimators linking individual-based and sample-based rarefaction, extrapolation and comparison of assemblages. J Plant Ecol 2012, 5 (1), 3–21. doi:10.1093/jpe/rtr044
- McMurdie PJ, Holmes S, Watson M, Phyloseq: an R package for reproducibleinteractive analysis and graphics of microbiome census data. PLoS One 2013, 8 (4), e61217. doi:10.1371/journal.pone.0061217
- Hughes JB, Hellmann JJ, The application of rarefaction techniques to molecular inventories of microbial diversity. Methods Enzymol 2005, 397, 292–308.
- Bullard JH, Purdom E, Hansen KD, Dudoit S, Evaluation of statistical methods for normalization and differential expression in mRNA-seq experiments. BMC Bioinform 2010, 11 (1), 94. doi:10.1186/1471-2105-11-94
- Dillies M-A, Rau A, Aubert J, Hennequet-Antier C, Jeanmougin M, Servant N, Keime C, Marot G, Castel D, Estelle J, et al., A comprehensive evaluation of normalization methods for illumina high-throughput RNA sequencing data analysis. Brief Bioinform 2012, 14 (6), 671–683. doi:10.1093/bib/bbs046
- Mitra S, Klar B, Huson DH, Visual and statistical comparison of metagenomes. Bioinformatics 2009, 25 (15), 1849–1855. doi:10.1093/bioinformatics/btp341
- Marioni JC, Mason CE, Mane SM, Stephens M, Gilad Y, RNA-seq: an assessment of technical reproducibility and comparison with gene expression arrays. Genome Res 2008, 18 (9), 1509–1517. doi:10.1101/gr.079558.108
- McKnight DT, Huerlimann R, Bower DS, Schwarzkopf L, Alford RA, Zenger KR, Methods for normalizing microbiome data: an ecological perspective. Methods Ecology Evol 2019, 10 (3), 389–400. doi:10.1111/2041-210X.13115
- McCafferty J, Mühlbauer M, Gharaibeh RZ, Arthur JC, Perez-Chanona E, Sha W, Jobin C, Fodor AA, Stochastic changes over time and not founder effects drive cage effects in microbial community assembly in a mouse model. ISME J 2013, 7 (11), 2116–2125. doi:10.1038/ismej.2013.106
- Bolstad BM, Irizarry RA, Åstrand M, Speed TP, A comparison of normalization methods for high density oligonucleotide array data based on variance and bias. Bioinformatics 2003, 19 (2), 185–193. doi:10.1093/bioinformatics/19.2.185
- Irizarry RA, Hobbs B, Collin F, Beazer‐Barclay YD, Antonellis KJ, Scherf U, Speed TP, Exploration, normalization, and summaries of high density oligonucleotide array probe level data. Biostatistics 2003, 4 (2), 249–264. doi:10.1093/biostatistics/4.2.249
- Hansen KD, Irizarry RA, Wu Z, Removing technical variability in RNA-seq data using conditional quantile normalization. Biostatistics 2012, 13 (2), 204–216. doi:10.1093/biostatistics/kxr054
- Hicks SC, Okrah K, Paulson JN, Quackenbush J, Irizarry RA, Bravo HC, Smooth quantile normalization. Biostatistics 2017, 19 (2), 185–198. doi:10.1093/biostatistics/kxx028
- Fortin J-P, Labbe A, Lemire M, Zanke BW, Hudson TJ, Fertig EJ, Greenwood CM, Hansen KD, Functional normalization of 450k methylation array data improves replication in large cancer studies. Genome Biol 2014, 15 (11), 503. doi:10.1186/s13059-014-0503-2
- Robinson MD, Oshlack A, A scaling normalization method for differential expression analysis of RNA-seq data. Genome Biol 2010, 11 (3), R25. doi:10.1186/gb-2010-11-3-r25
- Love MI, Huber W, Anders S, Moderated estimation of fold change and dispersion for RNA-seq data with DESeq2. Genome Biol 2014, 15 (12), 550. doi:10.1186/s13059-014-0550-8
- Jonsson V, Osterlund T, Nerman O, Kristiansson E, Variability in metagenomic count data and its influence on the identification of differentially abundant genes. J Computer Biological 2017, 24 (4), 311–326. doi:10.1089/cmb.2016.0180
- Sohn MB, Du R, An L, A robust approach for identifying differentially abundant features in metagenomic samples. Bioinformatics 2015, 31 (14), 2269–2275. doi:10.1093/bioinformatics/btv165
- Smyth GK, Limma: linear models for microarray data. In Bioinformatics and computational biology solutions using r and bioconductor, Springer: New York, 2005; pp. 397–420. doi:10.1007/0-387-29362-0_23
- Chen L, Reeve J, Zhang L, Huang S, Wang X, Chen J, GMPR: a robust normalization method for zero-inflated count data with application to microbiome sequencing data. PeerJ 2018, 6, e4600. doi:10.7717/peerj.4600
- Kumar MS, Slud EV, Okrah K, Hicks SC, Hannenhalli S, Corrada Bravo H, Analysis and correction of compositional bias in sparse sequencing count data. Bmc Genom 2018, 19 (1), 799. doi:10.1186/s12864-018-5160-5
- Aitchison J, A concise guide to compositional data analysis. In: 2nd Compositional Data Analysis Workshop. Girona, Italy 20032003.
- Aitchison J, The statistical analysis of compositional data. Chapman and Hall Ltd. Reprinted in 2003 with additional material by The Blackburn Press.: London, 1986.
- Lin H, Peddada SD, Analysis of compositions of microbiomes with bias correction. Nat Commun 2020, 11 (1), 3514. doi:10.1038/s41467-020-17041-7
- Ma Y, Luo Y, Jiang H, Valencia A, A novel normalization and differential abundance test framework for microbiome data. Bioinformatics 2020, 36 (13), 3959–3965. doi:10.1093/bioinformatics/btaa255
- Mulenga M, Kareem SA, Sabri AQM, Seera M, Govind S, Samudi C, Mohamad SB, Feature extension of gut microbiome data for deep neural network-based colorectal cancer classification. IEEE Access 2021, 9, 23565–23578. doi:10.1109/ACCESS.2021.3050838
- Singh D, Singh B, Investigating the impact of data normalization on classification performance. Appl Soft Comput 2020, 97, 105524. doi:10.1016/j.asoc.2019.105524
- Gotelli N, Colwell R, Quantifying biodiversity: procedures and pitfalls in the measurement and comparison of species richness. Ecol Lett 2001, 4, 379–391. 4 doi:10.1046/j.1461-0248.2001.00230.x
- Mao CX, Colwell RK, Estimation of species richness: mixture models, the role of rare species, and inferential challenges. Ecology 2005, 86 (5), 1143–1153. doi:10.1890/04-1078
- Brewer A, Williamson M, A new relationship for rarefaction. Biodivers Conserv 1994, 3 (4), 373–379. doi:10.1007/BF00056509
- Horner-Devine MC, Lage M, Hughes JB, Bohannan BJM, A taxa–area relationship for bacteria. Nature 2004, 432 (7018), 750–753. doi:10.1038/nature03073
- Jernvall J, Wright PC, Diversity components of impending primate extinctions. Proc Natl Acad Sci U S A 1998, 95 (19), 11279–11283. doi:10.1073/pnas.95.19.11279
- Schloss PD, Westcott SL, Ryabin T, Hall JR, Hartmann M, Hollister EB, Lesniewski RA, Oakley BB, Parks DH, Robinson CJ, et al., Introducing mothur: open-source, platform-independent, community-supported software for describing and comparing microbial communities. Appl Environ Microbiol 2009, 75 (23), 7537–7541. doi:10.1128/AEM.01541-09
- Schloss PD, Westcott SL, Ryabin T, Hall JR, Hartmann M, Hollister EB, Lesniewski RA, Oakley BB, Parks DH, Robinson CJ, et al., QIIME allows analysis of high-throughput community sequencing data. Nat Methods 2010, 7 (5), 335–336. doi:10.1038/nmeth.f.303
- Jari Oksanen FGB, Friendly M, Kindt R, Legendre P, McGlinn D, Minchin PR, O’Hara RB, Simpson GL, Peter Solymos MHHS, Szoecs E, et al., Vegan: community ecology package ordination methods, diversity analysis and other functions for community and vegetation ecologists. http://CRAN.R-project.org/package=vegan. 2019.
- Leo Lahti SSEA, Tools for microbiome analysis in R. Version 1.9.95. 2017.
- Lauber CL, Hamady M, Knight R, Fierer N, Pyrosequencing-based assessment of soil pH as apredictor of soil bacterial community structure at the continental scale. Appl Environ Microb 2009, 75 (15), 5111–5120. doi:10.1128/AEM.00335-09
- Lauber CL, Zhou N, Gordon JI, Knight R, Fierer N, Effect of storage conditions on the assessment of bacterial community structure in soil and human-associated samples. FEMS Microbiol Lett 2010, 307 (1), 80–86. doi:10.1111/j.1574-6968.2010.01965.x
- Aguirre de Cárcer D, Denman SE, McSweeney C, Morrison M, Evaluation of subsampling-based normalization strategies for tagged high-throughput sequencing data sets from gut microbiomes. Appl Environ Microb 2011, 77 (24), 8795–8798. doi:10.1128/AEM.05491-11
- Kuczynski J, Liu Z, Lozupone C, McDonald D, Fierer N, Knight R, Microbial community resemblance methods differ in their ability to detect biologically relevant patterns. Nat Methods 2010, 7 (10), 813–819. doi:10.1038/nmeth.1499
- Hamady M, Knight R, Microbial community profiling for human microbiome projects:tools, techniques, and challenges. Genome Res 2009, 19 (7), 1141–1152. doi:10.1101/gr.085464.108
- Kuczynski J, Costello EK, Nemergut DR, Zaneveld J, Lauber CL, Knights D, Koren O, Fierer N, Kelley ST, Ley RE, et al., Direct sequencing of the human microbiome readily reveals community differences. Genome Biol 2010, 11 (5), 210. doi:10.1186/gb-2010-11-5-210
- Hillmann B, Al-Ghalith GA, Shields-Cutler RR, Zhu Q, Gohl DM, Beckman KB, Knight R, Knights D, Rawls JF, Evaluating the information content of shallow shotgun metagenomics. mSystems 2018, 3 (6), e00069–18. doi:10.1128/mSystems.00069-18
- Papadimitriou K, Anastasiou R, Georgalaki M, Bounenni R, Paximadaki A, Charmpi C, Alexandraki V, Kazou M, Tsakalidou E, Comparison of the microbiome of artisanal homemade and industrial feta cheese through amplicon sequencing and shotgun metagenomics. Microorganisms 2022, 10 (5), 1073. doi:10.3390/microorganisms10051073
- Pereira MB, Wallroth M, Jonsson V, Kristiansson E, Comparison of normalization methods for the analysis of metagenomic gene abundance data. Bmc Genom 2018, 19 (1), 274. doi:10.1186/s12864-018-4637-6
- Xuan C, Shamonki JM, Chung A, DiNome ML, Chung M, Sieling PA, Lee DJ, Takabe K, Microbial dysbiosis is associated with human breast cancer. PLoS One 2014, 9 (1), e83744. doi:10.1371/journal.pone.0083744
- Sze MA, Baxter NT, Ruffin MT, Rogers MA, Schloss PD, Normalization of the microbiota in patients after treatment for colonic lesions. Microbiome 2017, 5 (1), 1–10. doi:10.1186/s40168-017-0366-3
- Baxter NT, Ruffin MT, Rogers MA, Schloss PD, Microbiota-based model improves the sensitivity of fecal immunochemical test for detecting colonic lesions. Genome Med 2016, 8 (1), 1–10. doi:10.1186/s13073-016-0290-3
- Dai Z, Coker OO, Nakatsu G, Wu WKK, Zhao L, Chen Z, Chan FKL, Kristiansen K, Sung JJY, Wong SH, et al., Multi-cohort analysis of colorectal cancer metagenome identified altered bacteria across populations and universal bacterial markers. Microbiome 2018, 6 (1), 70. doi:10.1186/s40168-018-0451-2
- Bergemann TL, Wilson J, Proportion statistics to detect differentially expressed genes: a comparison with log-ratio statistics. BMC Bioinform 2011, 12, 228–228. 1 doi:10.1186/1471-2105-12-228
- Dillies MA, Rau A, Aubert J, Hennequet-Antier C, Jeanmougin M, Servant N, Keime C, Marot G, Castel D, Estelle J, et al., A comprehensive evaluation of normalization methods for illumina high-throughput RNA sequencing data analysis. Brief Bioinform 2013, 14 (6), 671–683. doi:10.1093/bib/bbs046
- Mandal S, Van Treuren W, White RA, Eggesbø M, Knight R, Peddada SD, Analysis of composition of microbiomes: a novel method for studying microbial composition. Microb Ecol Health Dis 2015, 26 (1), 27663. doi:10.3402/mehd.v26.27663
- Tsilimigras MC, Fodor AA, Compositional data analysis of the microbiome: fundamentals, tools, and challenges. Ann Epidemiol 2016, 26 (5), 330–335. doi:10.1016/j.annepidem.2016.03.002
- Morton JT, Sanders J, Quinn RA, McDonald D, Gonzalez A, Vázquez-Baeza Y, Navas-Molina JA, Song SJ, Metcalf JL, Hyde ER, et al., Balance trees reveal microbial niche differentiation. mSystems 2017, 2 (1). doi:10.1128/mSystems.00162-16
- Jackson DA, COMPOSITIONAL DATA in COMMUNITY ECOLOGY: THE PARADIGM or PERIL of PROPORTIONS? Ecology 1997, 78 (3), 929–940. doi:10.1890/0012-9658(1997)078[0929:CDICET]2.0.CO;2
- Rezasoltani S, Aghdaei HA, Jasemi S, Gazouli M, Dovrolis N, Sadeghi A, Schlüter H, Zali MR, Sechi LA, Feizabadi MM, Oral microbiota as novel Biomarkers for colorectal cancer screening. Cancers 2023, 15 (1), 192. doi:10.3390/cancers15010192
- Poore GD, Kopylova E, Zhu Q, Carpenter C, Fraraccio S, Wandro S, Kosciolek T, Janssen S, Metcalf J, Song SJ, Microbiome analyses of blood and tissues suggest cancer diagnostic approach. Nature 2020, 579 (7800), 567–574. doi:10.1038/s41586-020-2095-1
- Hasan R, Bose S, Roy R, Paul D, Rawat S, Nilwe P, Chauhan NK, Choudhury S, Tumor tissue-specific bacterial biomarker panel for colorectal cancer: bacteroides massiliensis, alistipes species, alistipes onderdonkii, bifidobacterium pseudocatenulatum, Corynebacterium appendicis. Arch Microbiol 2022, 204 (6), 1–10. doi:10.1007/s00203-022-02954-2
- Simpson RC, Shanahan ER, Batten M, Reijers ILM, Read M, Silva IP, Versluis JM, Ribeiro R, Angelatos AS, Tan J, et al., Diet-driven microbial ecology underpins associations between cancer immunotherapy outcomes and the gut microbiome. Nat Med 2022, 2344–2352. doi:10.1038/s41591-022-01965-2
- Huber W, Von Heydebreck A, Sültmann H, Poustka A, Vingron M, Variance stabilization applied to microarray data calibration and to the quantification of differential expression. Bioinformatics 2002, 18 (suppl_1), S96–S104. doi:10.1093/bioinformatics/18.suppl_1.S96
- Parsons HM, Ludwig C, Günther UL, Viant MR, Improved classification accuracy in 1- and 2-dimensional NMR metabolomics data using the variance stabilising generalised logarithm transformation. BMC Bioinform 2007, 8 (1), 234. doi:10.1186/1471-2105-8-234
- van den Berg RA, Hoefsloot HC, Westerhuis JA, Smilde AK, van der Werf MJ, Centering, scaling, and transformations: improving the biological information content of metabolomics data. Bmc Genom 2006, 7 (1), 142. doi:10.1186/1471-2164-7-142
- Kvalheim OM, Brakstad F, Liang Y, Preprocessing of analytical profiles in the presence of homoscedastic or heteroscedastic noise. Anal Chem 1994, 66 (1), 43–51. doi:10.1021/ac00073a010
- Feng C, Wang H, Lu N, Chen T, He H, Lu Y, Tu XM, Log-transformation and its implications for data analysis. Shanghai Arch Psychiatry 2014, 26 (2), 105–109. doi:10.3969/j.issn.1002-0829.2014.02.009
- Feng C, Wang H, Lu N, Tu XM, Log transformation: application and interpretation in biomedical research. Stat Med 2013, 32 (2), 230–239. doi:10.1002/sim.5486
- Xia Y, Sun J, Pretreating and normalizing metabolomics data for statistical analysis. Genes & Dis 2023. doi:10.1016/j.gendis.2023.04.018
- De Livera AM, Dias DA, De Souza D, Rupasinghe T, Pyke J, Tull D, Roessner U, McConville M, Speed TP, Normalizing and integrating metabolomics data. Anal Chem 2012, 84 (24), 10768–10776. doi:10.1021/ac302748b
- Durbin BP, Hardin JS, Hawkins DM, Rocke DM, A variance-stabilizing transformation for gene-expression microarray data. Bioinformatics 2002, 18 (suppl_1), S105–S110. doi:10.1093/bioinformatics/18.suppl_1.S105
- Oresta B, Braga D, Lazzeri M, Frego N, Saita A, Faccani C, Fasulo V, Colombo P, Guazzoni G, Hurle R, et al., The microbiome of catheter collected urine in males with bladder cancer according to disease stage. J Urol 2021, 205 (1), 86–93. doi:10.1097/JU.0000000000001336
- Choi H, Kim S, Fermin D, Tsou C-C, Nesvizhskii AI, QPROT: statistical method for testing differential expression using protein-level intensity data in label-free quantitative proteomics. J Proteomics 2015, 129, 121–126. doi:10.1016/j.jprot.2015.07.036
- Li P, Piao Y, Shon HS, Ryu KH, Comparing the normalization methods for the differential analysis of illumina high-throughput RNA-Seq data. BMC Bioinform 2015, 16 (1), 347. doi:10.1186/s12859-015-0778-7
- Abrams ZB, Johnson TS, Huang K, Payne PRO, Coombes K, A protocol to evaluate RNA sequencing normalization methods. BMC Bioinform 2019, 20 (24), 679. doi:10.1186/s12859-019-3247-x
- Smyth GK, Limma: linear models for microarray data. In Bioinformatics and computational biology solutions using R and bioconductor, Springer: 2005; pp. 397–420. doi:10.1007/0-387-29362-0_23
- Wang Q, Ye J, Fang D, Lv L, Wu W, Shi D, Li Y, Yang L, Bian X, Wu J, et al., Multi-omic profiling reveals associations between the gut mucosal microbiome, the metabolome, and host DNA methylation associated gene expression in patients with colorectal cancer. BMC Microbiol 2020, 20 (S1), 83. doi:10.1186/s12866-020-01762-2
- Robinson MD, McCarthy DJ, Smyth GK, edgeR: a Bioconductor package for differential expression analysis of digital gene expression data. Bioinformatics 2010, 26 (1), 139–140. doi:10.1093/bioinformatics/btp616
- Boulund F, Pereira MB, Jonsson V, E K, Computational and statistical considerations in the analysis of metagenomic data. In: Metagenomics: perspectives, methods and applications, M N, editor Academic Press: Cambridge, 2018pp. 81–102 doi:10.1016/B978-0-08-102268-9.00004-5
- Sakurai T, De Velasco MA, Sakai K, Nagai T, Nishiyama H, Hashimoto K, Uemura H, Kawakami H, Nakagawa K, Ogata H, et al., Integrative analysis of gut microbiome and host transcriptomes reveals associations between treatment outcomes and immunotherapy-induced colitis. Mol Oncol 2022, 16 (7), 1493–1507. doi:10.1002/1878-0261.13062
- Lin Y, Lau HC-H, Liu Y, Kang X, Wang Y, Ting NL-N, Kwong TN-Y, Han J, Liu W, Liu C, et al., Altered mycobiota signatures and enriched pathogenic aspergillus rambellii are associated with colorectal cancer based onmulticohort fecal metagenomic analyses. Gastroenterology 2022, 163 (4), 908–921. doi:10.1053/j.gastro.2022.06.038
- Jin C, Lagoudas GK, Zhao C, Bullman S, Bhutkar A, Hu B, Ameh S, Sandel D, Liang XS, Mazzilli S, et al., Commensal microbiota promote lung cancer development via γδ Tcells. Cell 2019, 176 (5), 998–1013.e16. doi:10.1016/j.cell.2018.12.040
- Parhi L, Alon-Maimon T, Sol A, Nejman D, Shhadeh A, Fainsod-Levi T, Yajuk O, Isaacson B, Abed J, Maalouf N, Breast cancer colonization by fusobacterium nucleatum accelerates tumor growth and metastatic progression. Nat Commun 2020, 11 (1), 1–12. doi:10.1038/s41467-020-16967-2
- Anders S, Huber W, Differential expression analysis for sequence count data. Genome Biol 2010, 11 (10), R106. doi:10.1186/gb-2010-11-10-r106
- Mortazavi A, Williams BA, McCue K, Schaeffer L, Wold B, Mapping and quantifying mammalian transcriptomes by RNA-Seq. Nat Methods 2008, 5 (7), 621–628. doi:10.1038/nmeth.1226
- Thompson KJ, Ingle JN, Tang X, Chia N, Jeraldo PR, Walther-Antonio MR, Kandimalla KK, Johnson S, Yao JZ, Harrington SC, et al., A comprehensive analysis of breast cancer microbiota and host gene expression. PLoS One 2017, 12 (11), e0188873. doi:10.1371/journal.pone.0188873
- Lopes-Ramos CM, Kuijjer ML, Ogino S, Fuchs CS, DeMeo DL, Glass K, Quackenbush J, Gene regulatory network analysis identifies sex-linked differences in colon cancer drug metabolism. Cancer Res 2018, 78 (19), 5538–5547. doi:10.1158/0008-5472.CAN-18-0454
- Kadota K, Nishiyama T, Shimizu K, A normalization strategy for comparing tag count data. Algorithms Mol Biol 2012, 7 (1), 5–5. doi:10.1186/1748-7188-7-5
- Fu L, Luo K, Lv J, Wang X, Qin S, Zhang Z, Sun S, Wang X, Yun B, He Y, Integrating expression data-based deep neural network models with biological networks to identify regulatory modules for lung adenocarcinoma. Biology 2022, 11 (9), 1291. doi:10.3390/biology11091291
- Anders S, McCarthy DJ, Chen Y, Okoniewski M, Smyth GK, Huber W, Robinson MD, Count-based differential expression analysis of RNA sequencing data using R and Bioconductor. Nat Protoc 2013, 8 (9), 1765. doi:10.1038/nprot.2013.099
- Anders S, McCarthy DJ, Chen Y, Okoniewski M, Smyth GK, Huber W, Robinson MD, Count-based differential expression analysis of RNA sequencing data using R and Bioconductor. Nat Protoc 2013, 8 (9), 1765–1786. doi:10.1038/nprot.2013.099
- Maza E, In papyro comparison of TMM (edgeR), RLE (DESeq2), and MRN normalization methods for asimple two-conditions-without-replicates rna-seq experimental design. Front Genet 2016, 7 (164). doi:10.3389/fgene.2016.00164
- Wu Z, Liu W, Jin X, Ji H, Wang H, Glusman G, Robinson M, Liu L, Ruan J, Gao S, NormExpression: an R package to normalize gene expression data using evaluated methods. Front Genet 2019, 10 (400). doi:10.3389/fgene.2019.00400
- Klann E, Williamson JM, Tagliamonte MS, Ukhanova M, Asirvatham JR, Chim H, Yaghjyan L, Mai V, Microbiota composition in bilateral healthy breast tissue and breast tumors. Cancer Cause Control 2020, 31 (11), 1027–1038. doi:10.1007/s10552-020-01338-5
- Law CW, Chen Y, Shi W, Smyth GK, Voom: precision weights unlock linear model analysis tools for RNA-seq read counts. Genome Biol 2014, 15 (2), R29. doi:10.1186/gb-2014-15-2-r29
- Schmidt BL, Kuczynski J, Bhattacharya A, Huey B, Corby PM, Queiroz EL, Nightingale K, Kerr AR, DeLacure MD, Veeramachaneni R, Changes in abundance of oral microbiota associated with oral cancer. PLoS One 2014, 9 (6), e98741. doi:10.1371/journal.pone.0098741
- Behary J, Amorim N, Jiang X-T, Raposo A, Gong L, McGovern E, Ibrahim R, Chu F, Stephens C, Jebeili H, Gut microbiota impact on the peripheral immune response in non-alcoholic fatty liver disease related hepatocellular carcinoma. Nat Commun 2021, 12 (1), 1–14. doi:10.1038/s41467-020-20422-7
- Smyth GK, Linear models and empirical bayes methods for assessing differential expression in microarray experiments. Stat Appl Genet Mol Biol 2004, 3 (1). 1–25 doi:10.2202/1544-6115.1027
- Lee C, Lee S, Park T In A comparison study of statistical methods for the analysis metagenome data, 2017 IEEE International Conference on Bioinformatics and Biomedicine (BIBM); Kansas City, United States. IEEE. 2017; pp 1777–1781.
- Costea PI, Zeller G, Sunagawa S, Bork P, A fair comparison. Nat Methods 2014, 11 (4), 359–359. doi:10.1038/nmeth.2897
- Paulson JN, Bravo HC, Pop M, A fair comparisonreply. Nat Methods 2014, 11 (4), 359–360. doi:10.1038/nmeth.2898
- Paulson JN, Olson ND, Braccia DJ, Wagner J, Talukder H, Pop M, HC B, metagenomeSeq: statistical analysis for sparse high-throughput sequncing. Bioconductor Package, http://www.cbcb.umd.edu/software/metagenomeSeq. Version 1.28.2. 2013.
- Norouzi-Beirami MH, Marashi S-A, Banaei-Moghaddam AM, Kavousi K, Beyond taxonomic analysis of microbiomes: a functional approach for revisiting microbiome changes in colorectal cancer. Front Microbiol 2020, 10, 3117. doi:10.3389/fmicb.2019.03117
- Wang Q, Ye J, Fang D, Lv L, Wu W, Shi D, Li Y, Yang L, Bian X, Wu J, Multi-omic profiling reveals associations between the gut mucosal microbiome, the metabolome, and host DNA methylation associated gene expression in patients with colorectal cancer. BMC Microbiol 2020, 20 (S1), 1–13. doi:10.1186/s12866-020-01762-2
- Alshawaqfeh M, Rababah S, Hayajneh A, Gharaibeh A, Serpedin E, MetaAnalyst: a user-friendly tool for metagenomic biomarker detection and phenotype classification. BMC Med Res Methodol 2022, 22 (1), 1–14. doi:10.1186/s12874-022-01812-5
- Hughes JB, Hellmann JJ, Ricketts TH, Bohannan BJ, Counting the uncountable: statistical approaches to estimating microbial diversity. Appl Environ Microbiol 2001, 67 (10), 4399–4406. doi:10.1128/AEM.67.10.4399-4406.2001
- Ai D, Pan H, Li X, Gao Y, Liu G, Xia LC, Identifying gut microbiota associated with colorectal cancer using a zero-inflated lognormal model. Front Microbiol 2019, 10, 826. doi:10.3389/fmicb.2019.00826
- Abbas-Aghababazadeh F, Li Q, Fridley BL, Lin H, Comparison of normalization approaches for gene expression studies completed with high-throughput sequencing. PLoS One 2018, 13 (10), e0206312. doi:10.1371/journal.pone.0206312
- Lee W-H, Chen K-P, Wang K, Huang H-C, Juan H-F, Characterizing the cancer-associated microbiome with small RNA sequencing data. Biochem Bioph Res Co 2020, 522 (3), 776–782. doi:10.1016/j.bbrc.2019.11.166
- Kharofa J, Apewokin S, Alenghat T, Ollberding NJ, Metagenomic analysis of the fecal microbiome in colorectal cancer patients compared to healthy controls as a function of age. Cancer Med 2022. 12 3 2945–2957 doi:10.1002/cam4.5197
- Swift D, Cresswell K, Johnson R, Stilianoudakis S, Wei X, A review of normalization and differential abundance methods for microbiome counts data. Wiley Interdiscip Rev Comput Stat 2022, e1586. 2023 1 doi:10.1002/wics.1586
- Yang L, Chen J, A comprehensive evaluation of microbial differential abundance analysis methods: current status and potential solutions. Microbiome 2022, 10 (1), 130. doi:10.1186/s40168-022-01320-0
- Lin H, Peddada SD, Analysis of microbial compositions: a review of normalization and differential abundance analysis. NPJ Biofilms Microbiomes 2020, 6 (1), 60. doi:10.1038/s41522-020-00160-w
- Muthiah S; H, C. B. Wrench:wrench normalization for sparse count data. R pack-age version 1.16.0. https://github.com/HCBravoLab/Wrench.
- Pawlowsky-Glahn V, Egozcue JJ, Tolosana-Delgado R, Modeling and analysis of compositional data. John Wiley & Sons: London UK, 2015. doi:10.1002/9781119003144
- Aitchison J, The statistical analysis of compositional data. J R Stat Soc Series B Stat Methodol 1982, 44 (2), 139–160. doi:10.1111/j.2517-6161.1982.tb01195.x
- Egozcue JJ, Isometric logratio transformations for compositional data analysis. Math Geol 2003, 35 (3), 279–300. doi:10.1023/A:1023818214614
- Fernandes AD, Macklaim JM, Linn TG, Reid G, Gloor GB, ANOVA-like differential expression (ALDEx) analysis for mixed population RNA-seq. PLoS One 2013, 8. 8 7 doi:10.1371/journal.pone.0067019
- Fernandes AD, Reid JN, Macklaim JM, McMurrough TA, Edgell DR, Gloor GB, Unifying the analysis of high-throughput sequencing datasets: characterizing RNA-seq, 16S rRNA gene sequencing and selective growth experiments by compositional data analysis. Microbiome 2014, 2 (1), 15. doi:10.1186/2049-2618-2-15
- Morton JT, Sanders J, Quinn RA, McDonald D, Gonzalez A, Vázquez-Baeza Y, Navas-Molina JA, Song SJ, Metcalf JL, Hyde ER, et al., Balance trees reveal microbial niche differentiation. mSystems 2017, 2 (1), e00162–16. doi:10.1128/mSystems.00162-16
- Silverman JD, Washburne AD, Mukherjee S, David LA, A phylogenetic transform enhances analysis of compositional microbiota data. Elife 2017, 6, e21887. doi:10.7554/eLife.21887
- van den Boogaart KG, Tolosana-Delgado R, Analyzing compositional data with R. Springer-Verlag: Berlin Heidelberg, 2013. doi:10.1007/978-3-642-36809-7
- Quinn TP, Crowley TM, Richardson MF, Benchmarking differential expression analysis tools for RNA-Seq: normalization-based vs. log-ratio transformation-based methods. BMC Bioinform 2018, 19 (1), 274. doi:10.1186/s12859-018-2261-8
- Urbaniak C, Angelini M, Gloor GB, Reid G, Human milk microbiota profiles in relation to birthing method, gestation and infant gender. Microbiome 2016, 4 (1), 1. doi:10.1186/s40168-015-0145-y
- Quinn TP, Erb I, Richardson MF, Crowley TM, Wren J, Understanding sequencing data as compositions: an outlook and review. Bioinformatics 2018, 34 (16), 2870–2878. doi:10.1093/bioinformatics/bty175
- Seyednasrollah F, Laiho A, Elo LL, Comparison of software packages for detecting differential expression in RNA-seq studies. Brief Bioinform 2013, 16 (1), 59–70. doi:10.1093/bib/bbt086
- Tarazona S, Furió-Tarí P, Turrà D, Pietro AD, Nueda MJ, Ferrer A, Conesa A, Data quality aware analysis of differential expression in RNA-seq with NOISeq R/Bioc package. Nucleic Acids Res 2015, 43 (21), e140–e140. doi:10.1093/nar/gkv711
- Williams CR, Baccarella A, Parrish JZ, Kim CC, Empirical assessment of analysis workflows for differential expression analysis of human samples using RNA-Seq. BMC Bioinform 2017, 18 (1), 38. doi:10.1186/s12859-016-1457-z
- Morton JT, Marotz C, Washburne A, Silverman J, Zaramela LS, Edlund A, Zengler K, Knight R, Establishing microbial composition measurement standards with reference frames. Nat Commun 2019, 10 (1), 2719. doi:10.1038/s41467-019-10656-5
- Peng Z, Cheng S, Kou Y, Wang Z, Jin R, Hu H, Zhang X, Gong J-F, Li J, Lu M, The gut microbiome is associated with clinical response to anti–PD-1/PD-L1 immunotherapy in gastrointestinal cancer. Cancer Immunol Res 2020, 8 (10), 1251–1261. doi:10.1158/2326-6066.CIR-19-1014
- Thomas C, Aitchison J, Log-ratios and geochemical discrimination of scottish dalradian limestones: a case study. Geol Soc London, Spec Publ 2006, 264 (1), 25–41. doi:10.1144/GSL.SP.2006.264.01.03
- Mandal S, Treuren W, White RA, Eggesbo M, Knight R, Peddada SD, Analysis of composition of microbiomes: a novel method for studying microbial composition. Microb Ecol Health Dis 2015, 26. 26 doi:10.3402/mehd.v26.27663
- Xia Y, Sun J, Chen D-G, Compositional analysis of microbiome data. In Statistical analysis of microbiome data with R, Springer Singapore: Springer Singapore, 2018; pp. 331–393. doi:10.1007/978-981-13-1534-3_10
- Brill B, Amir A, Heller R, Testing for differential abundance in compositional counts data, with application to microbiome studies. arXiv preprint arXiv 2019, 1904.08937.
- Wallen ZD, Comparison study of differential abundance testing methods using two large parkinson disease gut microbiome datasets derived from 16S amplicon sequencing. BMC Bioinform 2021, 22 (1), 1–29. doi:10.1186/s12859-021-04193-6
- Bai J, Jhaney I, Daniel G, Bruner DW Inpilot study of vaginal microbiome using QIIME 2™ in women with gynecologic cancer before and after radiation therapy, Oncol Nurs Forum, 2019. 46 2 E48–E59 doi:10.1188/19.ONF.E48-E59
- Cheung MK, Yue GGL, Tsui KY, Gomes AJ, Kwan HS, Chiu PWY, San Lau CB, Discovery of an interplay between the gut microbiota and esophageal squamous cell carcinoma in mice. Am J Cancer Res 2020, 10 (8), 2409.
- Debelius JW, Huang T, Cai Y, Ploner A, Barrett D, Zhou X, Xiao X, Li Y, Liao J, Zheng Y, et al., Subspecies niche specialization in the oral microbiome is associated with nasopharyngeal carcinoma risk. mSystems 2020, 5 (4), e00065–20. doi:10.1128/mSystems.00065-20
- Xia Y, Sun J, Chen D-G, Modeling Zero-Inflated Microbiome Data. In: Statistical analysis of microbiome data with R, Xia Y, Sun J Chen D-G, editors Springer Singapore: Springer Singapore, 2018; pp. 453–496. doi:10.1007/978-981-13-1534-3_12
- Wang S, Robust differential abundance test in compositional data. arXiv preprint arXiv 2021, 2101.08765.
- Kaul A, Mandal S, Davidov O, Peddada SD, Analysis of microbiome data in the presence of excess zeros. Front Microbiol 2017, 8 (2114). doi:10.3389/fmicb.2017.02114
- Dai W, Li C, Li T, Hu J, Zhang H, Super-taxon in human microbiome are identified to be associated with colorectal cancer. BMC Bioinform 2022, 23 (1), 243. doi:10.1186/s12859-022-04786-9
- Ridout M, Hinde J, Demétrio CG, A score test for testing a zero‐inflated Poisson regression model against zero‐inflated negative binomial alternatives. Biometrics 2001, 57 (1), 219–223. doi:10.1111/j.0006-341X.2001.00219.x
- Jung BC, Jhun M, Lee JW, Bootstrap tests foroverdispersion in a zero‐inflated Poisson regression model. Biometrics 2005, 61 (2), 626–628. doi:10.1111/j.1541-0420.2005.00368.x
- Sayyari E, Kawas B, Mirarab S, TADA: phylogenetic augmentation of microbiome samples enhances phenotype classification. Bioinformatics 2019, 35 (14), i31–i40. doi:10.1093/bioinformatics/btz394
- Mansour RF, Alfar NM, Abdel‐Khalek S, Abdelhaq M, Saeed RA, Alsaqour R, Optimal deep learning based fusion model for biomedical image classification. Expert Syst 2022, 39 (3), e12764. doi:10.1111/exsy.12764
- Zhang X, Yi N, Valencia A, Fast zero-inflated negative binomial mixed modeling approach for analyzing longitudinal metagenomics data. Bioinformatics 2020, 36 (8), 2345–2351. doi:10.1093/bioinformatics/btz973
- Lozupone C, Knight R, UniFrac: a new phylogenetic method for comparing microbial communities. applied and environmental microbiology 2005, 71 (12), 8228–8235. Appl Environ Microb doi:10.1128/AEM.71.12.8228-8235.2005
- Lozupone C, Lladser ME, Knights D, Stombaugh J, Knight R, UniFrac: an effective distance metric for microbial community comparison. ISME J 2011, 5 (2), 169–172. doi:10.1038/ismej.2010.133
- Di Bella JM, Bao Y, Gloor GB, Burton JP, Reid G, High throughput sequencing methods and analysis for microbiome research. J Microbiol Methods 2013, 95 (3), 401–414. doi:10.1016/j.mimet.2013.08.011
- Segata N, Boernigen D, Tickle TL, Morgan XC, Garrett WS, Huttenhower C, Computational meta’omics for microbial community studies. Mol Syst Biol 2013, 9 (1), 666. doi:10.1038/msb.2013.22
- Navas-Molina JA, Peralta-Sánchez JM, González A, McMurdie PJ, Vázquez-Baeza Y, Xu Z, Ursell LK, Lauber C, Zhou H, Song SJ, Advancing our understanding of the human microbiome using QIIME. Vol. 531, Methods Enzymol. Elsevier; 2013; pp 371–444.
- Hughes JB, Hellmann JJ, The application of rarefaction techniques to molecular inventories of microbial diversity. In Methods in enzymology, Academic Press: 2005; Vol. 397, pp. 292–308. doi:10.1016/S0076-6879(05)97017-1
- Koren O, Knights D, Gonzalez A, Waldron L, Segata N, Knight R, Huttenhower C, Ley RE, Eisen JA, A guide toenterotypes across the human body: meta-analysis of microbial community structures in human microbiome datasets. PLoS Comput Biol 2013, 9 (1), e1002863. doi:10.1371/journal.pcbi.1002863
- Xia Y, Morrison-Beedy D, Ma J, Feng C, Cross W, Tu X, Modeling count outcomes from HIV risk reduction interventions: a comparison of competing statistical models for count responses. AIDS Res Treat 2012, 2012, 593569–593569. doi:10.1155/2012/593569
- Feng C, Wang H, Han Y, Xia Y, Lu N, Tu XM, Some theoretical comparisons of negative binomial and zero-inflated Poisson distributions. Commun In Stat- Theory And Methods 2015, 44 (15), 3266–3277. doi:10.1080/03610926.2013.823203
- Xia Y, Sun J, C DG, Modeling over-dispered microbiome data. In Statistical analysis of microbiome data with R, Springer: Singapore, 2018; pp. 395–451. doi:10.1007/978-981-13-1534-3_11
- Xia Y, Sun J, C DG, Modeling Zero-Inflated Microbiome Data. In Statistical analysis of microbiome data with R, Springer Singapore: Singapore, 2018; pp. 453–496. doi:10.1007/978-981-13-1534-3_12
- Jonsson V, Österlund T, Nerman O, Kristiansson E, Variability in metagenomic count data and its influence on the identification of differentially abundant genes. J Comput Biol 2017, 24 (4), 311–326. doi:10.1089/cmb.2016.0180
- Wang Y, Lêcao K-A, Managing batch effects in microbiome data. Brief Bioinform 2019. 21 6 1954–1970 doi:10.1093/bib/bbz105
- Ma S, Shungin D, Mallick H, Schirmer M, Nguyen LH, Kolde R, Franzosa E, Vlamakis H, Xavier R, Huttenhower C, Population structure discovery in meta-analyzed microbial communities and inflammatory bowel disease using MMUPHin. Genome Biol 2022, 23 (1), 1–31. doi:10.1186/s13059-022-02753-4
- Ling W, Lu J, Zhao N, Lulla A, Plantinga AM, Fu W, Zhang A, Liu H, Song H, Li Z, et al., Batch effects removal for microbiome data via conditional quantile regression. Nat Commun 2022, 13 (1), 5418. doi:10.1038/s41467-022-33071-9
- Dai Z, Wong SH, Yu J, Wei Y, Birol I, Batch effects correction for microbiome data with Dirichlet-multinomial regression. Bioinformatics 2019, 35 (5), 807–814. doi:10.1093/bioinformatics/bty729
- Anscombe FJ, The transformation of Poisson, binomial and negative-binomial data. Biometrika 1948, 35 (3/4), 246–254. doi:10.1093/biomet/35.3-4.246
- de Cárcer DA, Denman SE, McSweeney C, Morrison M, Evaluation of subsampling-based normalization strategies for tagged high-throughput sequencing data sets from gut microbiomes. Appl Environ Microb 2011, 77 (24), 8795–8798. doi:10.1128/AEM.05491-11
- Xia Y, Sun J, Pretreating and normalizing metabolomics data for statistical analysis. Genes & Dis in press, 2023. doi:10.1016/j.gendis.2023.04.018
- Boulund F, Pereira MB, Jonsson V, Kristiansson E, Chapter 4 - computational and statistical considerations in the analysis of metagenomic data. In: Metagenomics, Nagarajan M, editor Academic Press: 2018; pp. 81–102. doi:10.1016/B978-0-08-102268-9.00004-5
- Paulson JN Normalization and differential abundance analysis of metagenomic biomarker-gene surveys. University of Maryland, College Park, 2015.
- Jonsson V, Österlund T, Nerman O, Kristiansson E, Statistical evaluation of methods for identification of differentially abundant genes in comparative metagenomics. Bmc Genom 2016, 17, 78–78. 1 doi:10.1186/s12864-016-2386-y
- Parks DH, Tyson GW, Hugenholtz P, Beiko RG, STAMP: statistical analysis of taxonomic and functional profiles. Bioinformatics 2014, 30 (21), 3123–3124. doi:10.1093/bioinformatics/btu494
- Xia Y, Sun J, Chen D-G, What Are Microbiome Data? In Statistical analysis of microbiome data with R, Springer Singapore: Springer Singapore, 2018; pp. 29–41. doi:10.1007/978-981-13-1534-3_2
- Glusman G, Caballero J, Robinson M, Kutlu B, Hood L, Jordan IK, Optimal scaling ofdigital transcriptomes. PLoS One 2013, 8 (11), e77885. doi:10.1371/journal.pone.0077885
- Egozcue J, Pawlowsky-Glahn V, Mateu-Figueraz G, Barceló-Vidal C, doi:10.1023/A:1023818214614. Math Geol 2003, 35, 279–300. 3
- Greenacre M, Measuring Subcompositional Incoherence. Math Geosci, 43, 681–693. 2011. 6 doi:10.1007/s11004-011-9338-5
- Martín-Fernández J-A, Hron K, Templ M, Filzmoser P, Palarea-Albaladejo J, Bayesian-multiplicative treatment of count zeros in compositional data sets. Stat Modelling 2015, 15 (2), 134–158. doi:10.1177/1471082X14535524
- Mosimann JE, On the compound multinomial distribution, the multivariate β-distribution, and correlations among proportions. Biometrika 1962, 49 (1/2), 65–82. doi:10.1093/biomet/49.1-2.65
- Lovell D, Pawlowsky-Glahn V, Egozcue JJ, Marguerat S, Bähler J, Dunbrack Jr RL, Proportionality: a valid alternative to correlation for relative data. PLoS Comput Biol 2015, 11 (3), e1004075. doi:10.1371/journal.pcbi.1004075
- Kristiansson E, Hugenholtz P, Dalevi D, ShotgunFunctionalizeR: an R-package for functional comparison of metagenomes. Bioinformatics 2009, 25 (20), 2737–2738. doi:10.1093/bioinformatics/btp508
- Hanley JA, McNeil BJ, The meaning and use of the area under a receiver operating characteristic (ROC) curve. Radiology 1982, 143 (1), 29–36. doi:10.1148/radiology.143.1.7063747
- Bradley AP, The use of the area under the ROC curve in the evaluation of machine learning algorithms. Pattern Recogn 1997, 30 (7), 1145–1159. doi:10.1016/S0031-3203(96)00142-2
- Pearson KI, Mathematical contributions to the theory of evolution.—VII. On the correlation of characters not quantitatively measurable. Philos Trans of the R Soc of London Ser A Containing Pap of a Math or Phys Charact 1900, 195 (262–273), 1–47.
- Spearman C, The Proof and Measurement of Association between Two Things. Am J Psychol 1904, 15 (1), 72–101. doi:10.2307/1412159
- Matthews BW, Comparison of the predicted and observed secondary structure of T4 phage lysozyme. Biochim et Biophysica Acta (BBA)-Protein Struct 1975, 405 (2), 442–451. doi:10.1016/0005-2795(75)90109-9
- Müller R, Büttner P, A critical discussion of intraclass correlation coefficients. Stat Med 1994, 13 (23–24), 2465–2476. doi:10.1002/sim.4780132310
- Bray JR, Curtis JT, An ordination of the upland forest communities of southern Wisconsin. Ecol Monogr 1957, 27 (4), 326–349. doi:10.2307/1942268
- Witten DM, Classification and clustering of sequencing data using a Poisson model. The Annals of Applied Statistics 2011, 5 (4), 2493–2518. Ann Appl Stat doi:10.1214/11-AOAS493
- Lozupone CA, Hamady M, Kelley ST, Knight R, Quantitative and qualitative β diversity measures lead to different insights into factors that structure microbial communities. Appl Environ Microb 2007, 73 (5), 1576–1585. doi:10.1128/AEM.01996-06
- Sneath PH, The application of computers to taxonomy. Microbiology 1957, 17 (1), 201–226. doi:10.1099/00221287-17-1-201
- McQuitty LL, Hierarchical linkage analysis for the isolation of types. Educ Psychol Meas 1960, 20 (1), 55–67. doi:10.1177/001316446002000106
- Sokal R, Sneath PHA Principles of numerical taxonomy. WH: Freeman, San Francisco: 1963.
- Sokal RR, A statistical method for evaluating systematic relationships. Univ Kansas Sci Bull. 1958, 38, 1409–1438.
- Ward JH Jr, Hierarchical grouping to optimize an objective function. J Am Stat Assoc 1963, 58 (301), 236–244. doi:10.1080/01621459.1963.10500845
- Pearson KL, LIII. On lines and planes of closest fit to systems of points in space. London, Edinburgh Dublin Phil Mag J Sci 1901, 2 (11), 559–572. doi:10.1080/14786440109462720
- Hotelling H, Analysis of a complex of statistical variables into principal components. J Educ Psychol 1933, 24 (6), 417. doi:10.1037/h0071325
- Hotelling H, Relations Between Two Sets of Variates. Biometrika 1936, 28 (3/4), 321–377. doi:10.1093/biomet/28.3-4.321
- Gower JC, Some distance properties of latent root and vector methods used in multivariate analysis. Biometrika 1966, 53 (3–4), 325–338. doi:10.1093/biomet/53.3-4.325
- Shepard RN, The analysis of proximities: Multidimensional scaling with an unknown distance function. I. Psychometrika 1962, 27 (2), 125–140. doi:10.1007/BF02289630
- Shepard RN, Metric structures in ordinal data. J Math Psychol 1966, 3 (2), 287–315. doi:10.1016/0022-2496(66)90017-4
- Kruskal JB, Multidimensional scaling by optimizing goodness of fit to a nonmetric hypothesis. Psychometrika 1964, 29 (1), 1–27. doi:10.1007/BF02289565
- Kruskal JB, Nonmetric multidimensional scaling: A numerical method. Psychometrika 1964, 29 (2), 115–129. doi:10.1007/BF02289694
- Pollard K, Gilbert H, Ge Y, Taylor S, Dudoit S , Multtest: Resampling-Based Multiple Hypothesis Testing 2023. http://CRAN.Rproject.org/package=multtest,rpackageversion2.57.0
- Robinson MD, Smyth GK, Small-sample estimation of negative binomial dispersion, with applications to SAGE data. Biostatistics 2008, 9 (2), 321–332. doi:10.1093/biostatistics/kxm030
- Anderson MJ, Walsh DC, PERMANOVA, ANOSIM, and the Mantel test in the face of heterogeneous dispersions: what null hypothesis are you testing? Ecological monographs 2013, 83 (4), 557–574. doi:10.1890/12-2010.1
- Lin Y, Golovnina K, Chen Z-X, Lee HN, Negron YLS, Sultana H, Oliver B, Harbison ST, Comparison of normalization and differential expression analyses using RNA-Seq data from 726 individual Drosophila melanogaster. Bmc Genom 2016, 17 (1), 28. doi:10.1186/s12864-015-2353-z
- Wang Z, Gerstein M, Snyder M, RNA-Seq: a revolutionary tool for transcriptomics. Nature reviews genetics 2009, 10 (1), 57–63. Nat Rev Genet doi:10.1038/nrg2484
- Song H, Ling W, Zhao N, Plantinga AM, Broedlow CA, Klatt NR, Hensley-McBain T, Wu MC, Accommodating multiple potential normalizations in microbiome associations studies. BMC Bioinform 2023, 24 (1), 22. doi:10.1186/s12859-023-05147-w