ABSTRACT
We previously reported that autologous-fecal-microbiota-transplantation (aFMT), following 6 m of lifestyle intervention, attenuated subsequent weight regain and insulin rebound for participants consuming a high-polyphenol green-Mediterranean diet. Here, we explored whether specific changes in the core (abundant) vs. non-core (low-abundance) gut microbiome taxa fractions during the weight-loss phase (0–6 m) were differentially associated with weight maintenance following aFMT. Eighty-two abdominally obese/dyslipidemic participants (age = 52 years; 6 m weightloss = −8.3 kg) who provided fecal samples (0 m, 6 m) were included. Frozen 6 m’s fecal samples were processed into 1 g, opaque and odorless aFMT capsules. Participants were randomly assigned to receive 100 capsules containing their own fecal microbiota or placebo over 8 m-14 m in ten administrations (adherence rate > 90%). Gut microbiome composition was evaluated using shotgun metagenomic sequencing. Non-core taxa were defined as ≤ 66% prevalence across participants. Overall, 450 species were analyzed. At baseline, 13.3% were classified as core, and Firmicutes presented the highest core proportion by phylum. During 6 m weight-loss phase, abundance of non-core species changed more than core species (P < .0001). Subject-specific changes in core and non-core taxa fractions were strongly correlated (Jaccard Index; r = 0.54; P < .001). Following aFMT treatment, only participants with a low 6 m change in core taxa, and a high change in non-core taxa, avoided 8–14 m weight regain (aFMT = −0.58 ± 2.4 kg, corresponding placebo group = 3.18 ± 3.5 kg; P = .02). In a linear regression model, low core/high non-core 6 m change was the only combination that was significantly associated with attenuated 8–14 m weight regain (P = .038; P = .002 for taxa patterns/treatment intervention interaction). High change in non-core, low-abundance taxa during weight-loss might mediate aFMT treatment success for weight loss maintenance.
ClinicalTrials.gov: NCT03020186
Introduction
One of the early ways to characterize the microbiome in a structured manner was by studying the “core microbiome,” defined most simply and broadly as microbial features shared across a population.Citation1,Citation2 While evidence suggests there is no single core or non-core microbiome in the sense of a shared set of taxa across all healthy individuals,Citation3 studies with different hosts and habitats have reported a role for taxa with low abundance,Citation4–6 including driving a community structure change following a new diet in a model organism.Citation5 We have reported a similar phenomenon in a human trial, for participants consuming a high-polyphenols plant-based Mediterranean diet.Citation7 However, the significance of low-abundance taxa in the context of obesity has yet to be further addressed in humans.
Fecal microbiota transplantation (FMT) has offered a direct way to utilize the microbiome as a therapeutic tool. It is an effective treatment for recurrent Clostridioides difficile infectionCitation8 and is being investigated for various conditions.Citation9–14 Due to the increasing prevalence of obesity,Citation15 and reports supporting the role of the gut microbiome in obesity,Citation16–19 FMT has garnered attention as a possible treatment for obesity. To date, few studies have examined FMT’s therapeutic potential for obesity.Citation20–23 Those studies show partial improvement of metabolic markers, with little to no benefit on body weight; however, they did not include dietary interventions. Kootte et al.Citation20 reported differences in baseline microbiota composition between responders and non-responders, raising the possibility that personal microbiome characteristics are associated with FMT success. A novel approach of FMT is autologous FMT (aFMT), i.e., obtaining a person’s fecal sample at a ‘healthier’ time and self-transplanting later, with the advantage of eliminating the potential of inter-individual pathogen transmission. In a mouse-model study that compared the effects of aFMT, allogenic FMT, and no FMT on obesity induced by 12w high fat diet, followed by 6w of caloric restriction, aFMT processed before obesity induction and administered in the last 2 weeks of the caloric restriction period proved most effective at potentiating weight loss induced by calorie restriction.Citation24
Recently, we have used aFMT in humans, by processing participants’ fecal samples after an optimization period (nadir of weight-loss) and transplanting them during the expected weight-regain phase. We showed that aFMT attenuated the expected weight regain and insulin rebound for participants consuming a high-polyphenol “green” Mediterranean diet,Citation25 as well as maintaining decreased levels of leptin, C-reactive protein, Interleukin-6, and total cholesterol that were achieved in the weight-loss phase.Citation26
In this exploratory analysis, we hypothesized that distinct responses of the prevalent (core) and low-abundance (non-core) fractions of the gut microbiome during the weight loss phase might contribute to aFMT treatment success.
Results
Baseline characteristics and 6 months of weight-loss
Of 90 participants who began the intervention (aFMT; n = 44, placebo; n = 46), 83 were eligible for microbiome analysis and 82 for weight maintenance analysis. Ineligibility reasons and study design are presented in . The 82 participants included in the analysis did not differ from the 8 participants who were not included in their weight at recruitment to the lifestyle trial (included = 92.4 ± 14.39 kg, not included = 98.7 ± 11.69 kg, P = 0.19) and weight at recruitment to the aFMT trial (included = 84.4 ± 11.9 kg, not included = 87.23 ± 13.23 kg, P = 0.58). The mean age of participants was 52.6, and most were male (90.2%), reflecting the nature of the workplace. The baseline and 6- months characteristics of the participants included in the analysis are presented in .
Figure 1. Trial flow chart. ‘Microbiome analysis’ refers to Figure 2. ‘Weight maintenance analysis’ refers to Figure 3.
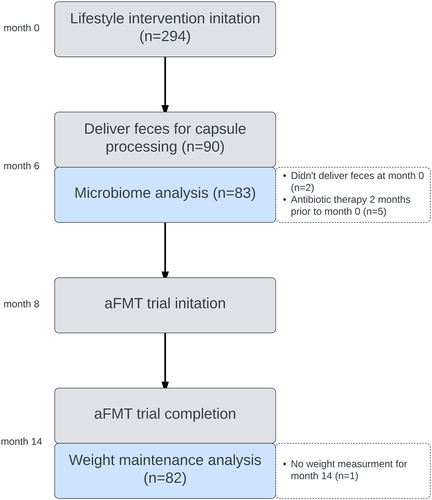
Table 1. Baseline and 6 months characteristics of the study population
Core and non-core species at baseline and response to lifestyle intervention
Overall, 450 species were included in the analysis (, appendix). At baseline, 60 were classified as core (13.3%), defined as prevalent in at least 66% of participants. Firmicutes was the phylum with the highest proportion of core species (47/263; 17.87%), followed by Actinobacteria (10/62; 16.12%) and Bacteroidetes (3/79; 3.79%). We further evaluated species division to the core and non-core fractions based on all available samples (including 6 m, 173 samples). Of 450 species, 445 (98.9%) matched their original classification (three non-core and two core species changed classification), and of all 701 available taxa, 695 (99.1%) matched their original classification (1 additional non-core taxa changed classification; overall, 1 taxon that changed classification was from the Firmicutes phylum), demonstrating that the division to the core and non-core fractions was robust in our study population. There was a strong correlation between the prevalence across participants and mean relative abundance at baseline across all species (Spearman’s r = 0.89, P < .0001), a correlation that remained strong both for the non-core (Spearman’s r = 0.84, P < .0001) and core (Spearman’s r = 0.61, P < .0001) fractions, as well as within the four major phyla (>1 species; Spearman’s r > 0.68, P < .0001). The mean prevalence of core species was found to be 90.4%, whereas non-core species exhibited a mean prevalence of 11.6%. Furthermore, the median relative abundance of core species was observed to be 6.9%, while non-core species displayed a median relative abundance of 0.8%. Next, we calculated the median 6 m relative abundance fold change for each species across participants () and found that non-core species changed more than core species (all species core vs. non-core: P < .0001), we further observed this pattern within Firmicutes species (Firmicutes core vs. Firmicutes non-core: P < .0001).
Figure 2. Bacterial species, stratified by phyla, are presented as a singular bar along the X-axis, as the same vertical bar along the entire figure represents the same species. (a). presence/absence matrix at baseline, red indicates presence. Each row represents a participant. classification to the core and non-core taxa based on the presence/absence matrix, green represents core. (b). species prevalence across all participants. (c). species mean relative abundance across all participants. (d). median log2 species 6m change across all participants. (e). T-test comparison of core and non-core 6m absolute median log2 change.
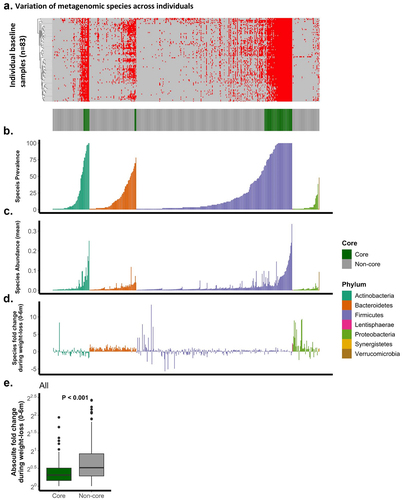
Core and non-core subject-specific fractions and aFMT weight maintenance
To evaluate person-specific changes in the core and non-core taxa during the weight-loss phase, we calculated the within-person Jaccard dissimilarity index using the R package VeganCitation27 for each subject’s core and non-core microbiome communities, assessing the intra-individual distance between the baseline and 6 m time points. A correlation was observed between the Jaccard index in core and non-core taxa fractions (Pearson’s r = .54, P < .001; ). By employing various cutoff thresholds to define the core microbiome, it is expected that the correlation strength would be most pronounced at the extreme ends of these thresholds. We observed an upward trend in the correlation starting at a cutoff of ~ 50% (Fig. S1), which led us to set this threshold as the minimum for our analysis. Subsequently, we computed the individual ratio between the Jaccard index for the core taxa and the Jaccard index for all taxa, aiming to establish an upper threshold for our analysis (Fig. S2), as we later utilized the changes in the core fraction to estimate the expected change in the non-core fraction. Although the proportion initially showed a relatively high value, we observed a downward trend at approximately 75%. This finding justified our choice of setting the threshold at 66% as it aligned with the observed trends in our dataset and our analytical framework. We further performed linear regression, with the non-core change as the dependent variable and the core change as the independent variable. Next, we divided participants according to high/low 6 m change in each fraction of the microbiome. High core microbiome change was considered as an above-median Jaccard dissimilarity index value. As the two fractions were highly correlated, high non-core microbiome change was considered a greater change than expected by the change in the core fraction (positive residual value in the linear regression model), generating four groups of high/low change in core/non-core fraction. Within each sub-group classified by its change in the core and non-core fractions, we compared the weight regain in 8-14 m between aFMT participants and placebo (). First, we found that there was no association between the core/non-core sub-groups and the lifestyle intervention arms (as previously described;Citation25 P = 0.6; Table S1). Prior to the aFMT, following 8 months of dietary intervention, no difference in 0-8 m weight loss between aFMT and placebo was observed within any of the sub-groups (Fig. S3). Following 6 months of administration of aFMT, between 8–14 months, a significant difference in weight change was observed only within the low core/high non-core group, with aFMT participants successfully maintaining their weight as compared to placebo (aFMT – low core/high non-core group = −0.58 ± 2.4 kg, placebo – low core/high non-core group = 3.18 ± 3.5 kg, P = 0.02; ). A near-significant opposite trend was observed in the complementary group, high core/low non-core (aFMT – high core/low non-core = 2.4 ± 1.5 kg, placebo – high core/low non-core = 0.87 ± 1.4 kg, P = .052). We further compared 8-14 m weight regain between aFMT participants only and found that core/non-core 6 m change pattern was associated with weight regain (high core/low non-core = 2.4 ± 1.5 kg, high core/high non-core = 2.28 ± 1.8 kg, low core/low non-core = 0.63 ± 1.9 kg, low core/high non-core = −0.58 ± 2.4 kg; P = 0.03 between groups; Fig. S4). In a linear regression model testing the effect of core/non-core group and treatment allocation on 8-14 m weight regain, as well as their interaction, the only significant pattern combination was the low core/high non-core group, and its interaction with aFMT treatment (P = .038 and P = .002, respectively; high core/high non-core; P = .914, low core/low non-core; P = .917, aFMT; P = .185, high core/low non-core and placebo were the references groups). Furthermore, aFMT participants from the low core/high non-core group were able to maintain their weight attenuation, as compared to placebo until the end of the lifestyle trial (8-18 m weight change: aFMT – low core/high non-core = −0.22 ± 2.1 kg, placebo – low core/high non-core = 3.77 ± 5.4 kg, P = .047; Fig. S5). Low core/high non-core group, and its interaction with aFMT treatment, remained significant in a linear regression model testing 8-18 m weight regain (P = .04 an P = .011, respectively).
Figure 3. (a). subject-specific Jaccard index for the core and non-core microbiome fractions, their correlation, and division to four “high/low core/non-core change” groups based on linear regression. (b). 8-14m weight regain comparison between aFMT and placebo within each “core/non-core” sub-group. T-tests were used to compare weight maintenance between treatment and placebo within each group.
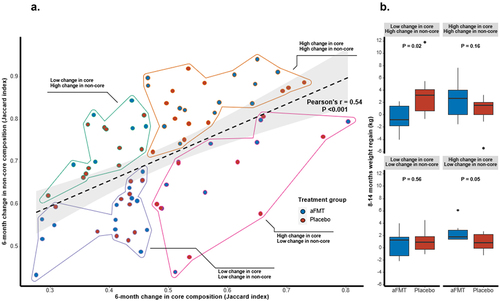
Discussion
In this exploratory analysis of a 14-month aFMT randomized placebo-controlled trial including 82 participants, we showed, using a person-specific Jaccard index metric, that participants who underwent a relatively small change in their “core microbiome” and a notable change in their “non-core microbiome” during a weight-loss phase uniquely maintained their weight loss after aFMT, up to 4 months following intervention completion. While the core and non-core fractions of the microbiome 6 m change were strongly correlated, the non-core fraction changed more than the core fraction.
The trial had several limitations. Similar to our previous trials,Citation28,Citation29 most participants were male. However, a previous notable study reported only a small effect size of gender on the overall difference in microbiome composition at the population level.Citation30 In addition, recruitment of participants from the same isolated workplace impose a limitation on the generalizability of our findings. In our analysis, very low-abundance taxa were excluded, possibly hindering our ability to pinpoint the effects of the very rare non-core taxa. We took that conservative approach due to the zero-inflated nature of microbiome data, trying to avoid the identification of spurious correlations. Strengths of the study include the rigorous double-blinded placebo-controlled design with supervised capsule administration and the use of bacterial species resolution characterized by shotgun metagenomics for microbiome analysis.
We report that substantial changes in low-abundance taxa across a shared-workplace population may contribute to the success of aFMT treatment in reducing weight regain. Investigators have applied different definitions and approaches to defining the core microbiome,Citation31,Citation32 but reports of the importance of rare taxa in different habitats and contextsCitation5,Citation6,Citation33 motivated us to perform this analysis. There is a wide range of variability in threshold values reported in the literature, ranging from 30% up to 100%.Citation31,Citation32 While we acknowledge that any choice of cutoff is somewhat arbitrary, our selection was based on the correlation between the core and non-core fractions and the changes observed in the core fraction, which helped us evaluate the expected changes in the non-core fraction. Furthermore, our selected threshold does not align with the extremes reported in the literature, which provides some support for our decision. It is noteworthy that all participants were recruited from a single, isolated research center, where they reside in the same geographical area and share a communal workplace dining room for their meals. Geography explains some of the inter-individual variation in the microbiome.Citation34 The induction of a lifestyle intervention was sufficient to change the taxa with low prevalence in our study population. The observed correlation between the core and non-core fractions might be expected due to the nature of microbiome data as compositional, and due to the rigorous classification of taxa as core/non-core after including samples following 6 months of lifestyle intervention. While we can’t definitively rule out any potential negative impacts of high changes in the core fraction, our analysis, which establishes a dependency of the change in the non-core fraction on the change in the core fraction, allows us to draw the conclusion that, within the context of low change in the core fraction, a high change in low-abundance taxa may be beneficial in the context of obesity.
While gut microbiome differ substantially between individuals,Citation3 variation at a given point in time (e.g., baseline of intervention) was reported to be linked with obesity, response to dietary intervention, and response to FMT treatment.Citation20,Citation35–37 In our analysis, variation of the gut microbiome response to a lifestyle intervention was associated with aFMT treatment success. Our results further imply the possible importance of personal microbiome features with maintenance of body weight, with features representing “core” and “non-core” microbial community dynamic during a perturbation in our analysis.
Firmicutes, known to be a highly abundant phylum in the human gut microbiome, has been suggested to be related to human obesity, although this claim is still controversial.Citation38 The high stability of core species from this phylum in our analysis, in conjunction with the relatively high core proportion, might signify the known role of Firmicutes as a stable component of the human gut microbiome.
In conclusion, our results suggest that the effectiveness of aFMT treatment to reduce weight regain following intervention-induced weight loss might be related to changes in the gut microbiome during the weight loss phase, with a significant role for the changes in low-abundance species.
Patients and methods
Study design
The aFMT and DIRECT-PLUS (Dietary Intervention Randomized Controlled Trial Polyphenols-Unprocessed; ClinicalTrials.gov Identifier: NCT03020186) lifestyle trial methods have been extensively described previously.Citation25 In short, the trial included two phases, each phase began at the same time for all participants. In the first phase, 294 eligible participants, employees of the nuclear research center in Dimona, Israel, were randomized to one of three unique diets; all received free gym membership and physical activity guidance. Inclusion and exclusion criteria are detailed in a previous report.Citation25 The main inclusion criteria were abdominal obesity (waist circumference: men >102 cm, women >88 cm) or dyslipidemia (Triglycerides >150 mg/dL and HDL cholesterol ≤40 mg/dL for men, ≤ 50 for women); exclusion criteria included major health impairments. In the second phase, 90 participants with ≥ 3.5% weight loss following 6 months of the first phase lifestyle intervention, and without antibiotic use within the prior two months, gave consent to enroll in an aFMT randomized, double-blinded, placebo-controlled intervention. The full fecal sample provided by participants at recruitment to the aFMT trial was processed into frozen, opaque, and odorless capsules. Participants were randomized in a 1:1 ratio to consume 100 g of their own processed fecal matter or an identical placebo dose over 10 sessions, over 6 months, starting at month 8 of the DIRECT-PLUS trial. An investigator supervised each capsule administration session. During months 6–14, participants still adhered to their allocated lifestyle intervention up to month 18.Citation39 The Soroka University Medical Center human subject committee approved and monitored the study. All participants provided written informed consent and received no financial compensation.
Weight and microbiome assessments
Participants were weighed without shoes to the nearest 0.1 kg at baseline, following the weight loss phase (6 m), and at the beginning (8 m) and the end (14 m) of the aFMT administration phase, as well as following additional 4 months of adherence to their randomized diet and physical activity (18 m). Fecal samples were provided by participants at baseline and after 6 months of lifestyle intervention at the study site, immediately frozen at −20°C for 1–3 days before storage at −80°C. For all microbiome analysis, fecal DNA was extracted, sequenced, and normalized using shotgun metagenomic. The average sequencing depth was 15.4 ± 2.6 million reads per sample. DNA sequences were aligned using an accelerated version of the Needleman-Wunsch algorithm to a curated database containing all representative genomes in RefSeq, version 86.Citation40 Each input sequence was assigned the lowest common ancestor that was consistent across at least 80% of all reference sequences tied for best hit. The number of counts for each taxon was then normalized to the average genome length. Additional details regarding the metagenomic pipeline are provided in a previous report.Citation25 Taxa prevalent in less than 2% of baseline samples and without phylum-level annotation were excluded from all analyses. Fecal samples obtained in the 2-months’ time window following antibiotics intake were excluded from the analysis.
Statistical analysis
Taxa with species-level annotation, prevalent in > 2% of samples and with relative abundance higher than 0.02% in at least one sample were included in the baseline analysis (m0). Prevalence was calculated based on the occurrence of species across all participants. A taxon was determined to be part of the core microbiome if, across participants, it was prevalent in ≥ 66% of the samples and non-core otherwise; prevalent classification was given to taxa with > 0.02% mean relative abundance. Pearson correlation was calculated to test the association between the subject-specific change in the core and non-core microbiome fractions. T-tests were used to compare core and non-core species change during the weight-loss phase and weight maintenance between treatment and placebo, Chi-squared test was used to evaluate the association between categorical variables. Analysis of variance (ANOVA) was used to compare the body weight outcome between the four microbiome groups. We tested the interaction between aFMT treatment and each of the core/non-core groups in a linear regression model, with weight regain in 8-14 m/8-18 m as the dependent variable; high core/low non-core was the reference group, in the python package statsmodels.Citation41 The cutoff for statistical significance was set to P < 0.05. Statistical analyses were performed using R software, version 3.5.3, and in the python package Pingouin.Citation42
Supplemental Material
Download Zip (544.3 KB)Acknowledgments
We thank Prof. Assaf Rudich for his review of the manuscript of this work.
Disclosure statement
Iris Shai advises the nutritional committee of Hinoman, Ltd. Ilan Youngster is an advisor for Mybiotics Ltd. Dan Knights is CEO of, and holds equity in, CoreBiome, Inc.
Data availability statement
As previously reported7, shotgun metagenomic sequencing data have been deposited in the European Nucleotide Archive with accession number PRJEB49147 (https://www.ebi.ac.uk/ena/browser/view/PRJEB49147)
Supplementary material
Supplemental data for this article can be accessed online at https://doi.org/10.1080/19490976.2023.2264457
Additional information
Funding
References
- Turnbaugh PJ, Ley RE, Hamady M, Fraser-Liggett CM, Knight R, Gordon JI. The human microbiome project. Nature [Internet]. 2007;449(7164):804–10. [accessed 2022 Aug 5]. doi:10.1038/nature06244.
- Shade A, Handelsman J. Beyond the venn diagram: the hunt for a core microbiome. Environ Microbiol [Internet]. 2012;14:4–12. [accessed 2022 Aug 5]. doi:10.1111/j.1462-2920.2011.02585.x.
- Lloyd-Price J, Abu-Ali G, Huttenhower C. The Healthy Human Microbiome Genome Medi [Internet]. 2016;8(1):1–11. [accessed 2022 Jul 27]. doi:10.1186/s13073-016-0307-y.
- Van Der Gast CJ, Walker AW, Stressmann FA, Rogers GB, Scott P, Daniels TW, Carroll MP, Parkhill J, Bruce KD. Partitioning core and satellite taxa from within cystic fibrosis lung bacterial communities. ISME J [Internet]. 2011;5:780–791. [accessed 2022 Aug 6]. doi:10.1038/ismej.2010.175.
- Benjamino J, Lincoln S, Srivastava R, Graf J. Low-abundant bacteria drive compositional changes in the gut microbiota after dietary alteration. Microbiome [Internet]. 2018;6(1):86. [accessed 2022 Aug 6]. doi:10.1186/s40168-018-0469-5.
- Velazquez EM, Nguyen H, Heasley KT, Saechao CH, Gil LM, Rogers AWL, Miller BM, Rolston MR, Lopez CA, Litvak Y, et al. Endogenous Enterobacteriaceae underlie variation in susceptibility to Salmonella infection. Nat microbiol [Internet]. 2019;4(6):1057–1064. [accessed 2022 Aug 6]. doi:10.1038/s41564-019-0407-8.
- Rinott E, Meir AY, Tsaban G, Zelicha H, Kaplan A, Knights D, Tuohy K, Scholz MU, Koren O, Stampfer MJ, et al. The effects of the green-Mediterranean diet on cardiometabolic health are linked to gut microbiome modifications: a randomized controlled trial. Genome Med. 2022;14(1):14. doi:10.1186/s13073-022-01015-z.
- Baunwall SMD, Lee MM, Eriksen MK, Mullish BH, Marchesi JR, Dahlerup JF, Hvas CL. Faecal microbiota transplantation for recurrent clostridioides difficile infection: an updated systematic review and meta-analysis. EClinicalMedicine. 2020;29-30:29–30. doi:10.1016/j.eclinm.2020.100642.
- Craven L, Rahman A, Nair Parvathy S, Beaton M, Silverman J, Qumosani K, Hramiak I, Hegele R, Joy T, Meddings J, et al. Allogenic fecal microbiota transplantation in patients with nonalcoholic fatty liver disease improves abnormal small intestinal permeability: a randomized control trial. Am J Gastroenterol [Internet]. 2020;115(7):1055–1065. [accessed 2022 Aug 7]. doi:10.14309/ajg.0000000000000661.
- Sokol H, Landman C, Seksik P, Berard L, Montil M, Nion-Larmurier I, Bourrier A, Le Gall G, Lalande V, De Rougemont A, et al. Fecal microbiota transplantation to maintain remission in Crohn’s disease: a pilot randomized controlled study. Microbiome [Internet]. 2020;8(1): [accessed 2022 Aug 7]. doi:10.1186/s40168-020-0792-5.
- Serrano-Villar S, Talavera-Rodríguez A, Gosalbes MJ, Madrid N, Pérez-Molina JA, Elliott RJ, Navia B, Lanza VF, Vallejo A, Osman M, et al. Fecal microbiota transplantation in HIV: a pilot placebo-controlled study. Nat Commun [Internet]. 2021;12(1): [accessed 2022 Aug 7]. doi:10.1038/s41467-021-21472-1.
- Costello SP, Hughes PA, Waters O, Bryant RV, Vincent AD, Blatchford P, Katsikeros R, Makanyanga J, Campaniello MA, Mavrangelos C, et al. Effect of fecal microbiota transplantation on 8-week Remission in patients with ulcerative colitis: a randomized clinical trial. JAMA [Internet]. 2019;321:156–164. [accessed 2022 Aug 7]. doi:10.1001/jama.2018.20046.
- De Groot P, Nikolic T, Pellegrini S, Sordi V, Imangaliyev S, Rampanelli E, Hanssen N, Attaye I, Bakker G, Duinkerken G, et al. Faecal microbiota transplantation halts progression of human new-onset type 1 diabetes in a randomised controlled trial. Gut. 2021;70(1):92–105. doi:10.1136/gutjnl-2020-322630.
- Davar D, Dzutsev AK, McCulloch JA, Rodrigues RR, Chauvin JM, Morrison RM, Deblasio RN, Menna C, Ding Q, Pagliano O, et al. Fecal microbiota transplant overcomes resistance to anti–PD-1 therapy in melanoma patients. Sci [Internet]. 2021;371(1979):595–602. [accessed 2022 Aug 7]. doi:10.1126/science.abf3363.
- Ward ZJ, Bleich SN, Cradock AL, Barrett JL, Giles CM, Flax C, Long MW, Gortmaker SL. Projected U.S. State-level prevalence of adult obesity and severe obesity. N Engl J Med [Internet]. 2019;381:2440–2450. [accessed 2022 Aug 7]. doi:10.1056/NEJMsa1909301.
- Chassaing B, Koren O, Goodrich JK, Poole AC, Srinivasan S, Ley RE, Gewirtz AT. Dietary emulsifiers impact the mouse gut microbiota promoting colitis and metabolic syndrome. Nature [Internet]. 2015;519(7541):92–96. [accessed 2022 Jun 25]. doi:10.1038/nature14232.
- Thaiss CA, Itav S, Rothschild D, Meijer MT, Levy M, Moresi C, Dohnalová L, Braverman S, Rozin S, Malitsky S, et al. Persistent microbiome alterations modulate the rate of post-dieting weight regain. Nature [Internet]. 2016;540(7634):544–551. [accessed 2022 May 7]. doi:10.1038/nature20796
- Peters BA, Shapiro JA, Church TR, Miller G, Trinh-Shevrin C, Yuen E, Friedlander C, Hayes RB, Ahn J. A taxonomic signature of obesity in a large study of American adults. Sci Rep [Internet]. 2018;8(1). [accessed 2022 Aug 7]. doi:10.1038/s41598-018-28126-1.
- Menni C, Jackson MA, Pallister T, Steves CJ, Spector TD, Valdes AM. Gut microbiome diversity and high-fibre intake are related to lower long-term weight gain. Int J Obes. 2017;41(7):1099–1105. doi:10.1038/ijo.2017.66.
- Kootte RS, Levin E, Salojärvi J, Smits LP, Hartstra AV, Udayappan SD, Hermes G, Bouter KE, Koopen AM, Holst JJ, et al. Improvement of insulin sensitivity after lean donor feces in metabolic syndrome is Driven by baseline intestinal microbiota composition. Cell Metab. 2017;26:611–619.e6. doi:10.1016/j.cmet.2017.09.008.
- Vrieze A, Van Nood E, Holleman F, Salojärvi J, Kootte RS, Bartelsman JFWM, Dallinga-Thie GM, Ackermans MT, Serlie MJ, Oozeer R, et al. Transfer of intestinal microbiota from lean donors increases insulin sensitivity in individuals with metabolic syndrome. Gastroenterology. 2012;143:913–916.e7. doi:10.1053/j.gastro.2012.06.031.
- Yu EW, Gao L, Stastka P, Cheney MC, Mahabamunuge J, Soto MT, Ford CB, Bryant JA, Henn MR, Hohmann EL, et al. Fecal microbiota transplantation for the improvement of metabolism in obesity: the fmt-trim double-blind placebo-controlled pilot trial. PLoS Med. 2020;17(3):17. doi:10.1371/journal.pmed.1003051.
- Allegretti JR, Kassam Z, Mullish BH, Chiang A, Carrellas M, Hurtado J, Marchesi JR, McDonald JAK, Pechlivanis A, Barker GF, et al. Effects of fecal microbiota transplantation with oral capsules in obese patients. Clin Gastroenterol Hepatol. 2020;18(4):855–863.e2. doi:10.1016/j.cgh.2019.07.006.
- Pérez-Matute P, Íñiguez M, de Toro M, Recio-Fernández E, Oteo JA. Autologous fecal transplantation from a lean state potentiates caloric restriction effects on body weight and adiposity in obese mice. Sci Rep [Internet]. 2020;10(1) [accessed 2022 Aug 7] doi:10.1038/s41598-020-64961-x.
- Rinott E, Youngster I, Yaskolka Meir A, Tsaban G, Zelicha H, Kaplan A, Knights D, Tuohy K, Fava F, Scholz MU, et al. Effects of diet-modulated autologous fecal microbiota transplantation on weight regain. Gastroenterology. 2021;160(1):158–173.e10. doi:10.1053/j.gastro.2020.08.041.
- Rinott E, Youngster I, Meir AY, Tsaban G, Kaplan A, Zelicha H, Rubin E, Koren O, Shai I. Autologous fecal microbiota transplantation can retain the metabolic achievements of dietary interventions. Eur J Intern Med. 2021;92:17–23. doi:10.1016/j.ejim.2021.03.038.
- Oksanen J, Simpson G, Blanchet F, Kindt R, Legendre P, Minchin P, O’Hara R, Solymos P, Stevens M, Szoecs E, et al. The vegan: Community ecology package. R package version 2.6-4. 2013. https://CRAN.R-project.org/package=vegan
- Shai I, Schwarzfuchs D, Henkin Y, Shahar DR, Witkow S, Greenberg I, Golan R, Fraser D, Bolotin A, Vardi H, et al. Weight loss with a low-carbohydrate, Mediterranean, or low-fat diet. N Engl J Med [Internet]. 2008;359:229–241. [accessed 2022 Aug 8]. doi:10.1056/nejmoa0708681.
- Gepner Y, Shelef I, Schwarzfuchs D, Zelicha H, Tene L, Meir AY, Tsaban G, Cohen N, Bril N, Rein M, et al. Effect of distinct lifestyle interventions on mobilization of fat storage pools CENTRAL magnetic resonance imaging randomized controlled trial. Circulation [Internet]. 2018;137:1143–1157. [accessed 2022 Jun 21]. doi:10.1161/CIRCULATIONAHA.117.030501.
- Falony G, Joossens M, Vieira-Silva S, Wang J, Darzi Y, Faust K, Kurilshikov A, Bonder MJ, Valles-Colomer M, Vandeputte D, et al. Population-level analysis of gut microbiome variation. Sci [Internet]. 2016;352(6285):560–564. [accessed 2022 Oct 31]. doi:10.1126/science.aad3503.
- Risely A, Tate A. Applying the core microbiome to understand host–microbe systems. J Animal Eco. 2020;89(7):1549–1558. doi:10.1111/1365-2656.13229.
- Neu AT, Allen EE, Roy K. Defining and quantifying the core microbiome: challenges and prospects. Proc Natl Acad Sci USA [Internet]. 2021;118(51). [accessed 2022 Jun 1]. doi:10.1073/pnas.2104429118.
- Pust MM, Tümmler B. Bacterial low-abundant taxa are key determinants of a healthy airway metagenome in the early years of human life. Comput Struct Biotechnol J. 2022;20:175–186. doi:10.1016/j.csbj.2021.12.008.
- Yatsunenko T, Rey FE, Manary MJ, Trehan I, Dominguez-Bello MG, Contreras M, Magris M, Hidalgo G, Baldassano RN, Anokhin AP, et al. Human gut microbiome viewed across age and geography. Nature. 2012;486(7402):222–227. doi:10.1038/nature11053.
- Palmas V, Pisanu S, Madau V, Casula E, Deledda A, Cusano R, Uva P, Vascellari S, Loviselli A, Manzin A, et al. Gut microbiota markers associated with obesity and overweight in Italian adults. Sci Rep [Internet]. 2021;11(1):1–14. [accessed 2022 Nov 21]. doi:10.1038/s41598-021-84928-w.
- Jian C, Silvestre MP, Middleton D, Korpela K, Jalo E, Broderick D, de Vos WM, Fogelholm M, Taylor MW, Raben A, et al. Gut microbiota predicts body fat change following a low-energy diet: a PREVIEW intervention study. Genome Med [Internet]. 2022;14:1–18. [accessed 2022 Jun 16]. doi:10.1186/s13073-022-01053-7.
- Jie Z, Yu X, Liu Y, Sun L, Chen P, Ding Q, Gao Y, Zhang X, Yu M, Liu Y, et al. The baseline gut microbiota directs dieting-induced weight loss trajectories. Gastroenterology [Internet]. 2021;160(6):2029–2042.e16. [accessed 2022 May 21]. doi:10.1053/j.gastro.2021.01.029.
- Magne F, Gotteland M, Gauthier L, Zazueta A, Pesoa S, Navarrete P, Balamurugan R. The firmicutes/bacteroidetes ratio: a relevant marker of gut dysbiosis in obese patients? Nutrients. 2020;12:1474. doi:10.3390/nu12051474.
- Meir AY, Rinott E, Tsaban G, Zelicha H, Kaplan A, Rosen P, Shelef I, Youngster I, Shalev A, Blüher M, et al. Effect of green-Mediterranean diet on intrahepatic fat: the DIRECT plus randomised controlled trial. Gut. 2021;70(11):2085–2095. doi:10.1136/gutjnl-2020-323106.
- O’Leary NA, Wright MW, Brister JR, Ciufo S, Haddad D, McVeigh R, Rajput B, Robbertse B, Smith-White B, Ako-Adjei D, et al. Reference sequence (RefSeq) database at NCBI: current status, taxonomic expansion, and functional annotation. Nucleic Acids Res [Internet]. 2016;44(D1):D733–45. [accessed 2022 Oct 28]. doi:10.1093/nar/gkv1189.
- Seabold S, Perktold J Statsmodels: econometric and Statistical modeling with Python [Internet]. 2010. http://statsmodels.sourceforge.net/
- Vallat R. Pingouin: statistics in Python. J Open Source Softw [Internet]. 2018;3(31):1026. [accessed 2022 Oct 2]. doi:10.21105/joss.01026.