ABSTRACT
Background: In recent years, many adolescents have fled their home countries due to war and human rights violations, consequently experiencing various traumatic events and putting them at risk of developing mental health problems. The symptomatology of refugee youth was shown to be multifaceted and often falling outside of traditional diagnoses.
Objective: The present study aimed to investigate the symptomatology of this patient group by assessing the network structure of a wide range of symptoms. Further, we assessed clinicians’ perceptions of symptoms relations in order to evaluate the clinical validity of the empirical network.
Methods: Empirical data on Post-Traumatic Stress Disorder (PTSD), depression and other trauma symptoms from N = 366 refugee youth were collected during the routine diagnostic process of an outpatient centre for refugee youth in Germany. Additionally, four clinicians of this outpatient centre were asked how they perceive symptom relations in their patients using a newly developed tool. Separate networks were constructed based on 1) empirical symptom data and 2) clinicians’ perceived symptom relations (PSR).
Results: Both the network based on empirical data and the network based on clinicians’ PSR showed that symptoms of PTSD and depression related most strongly within each respective cluster (connected mainly via sleeping problems), externalizing symptoms were somewhat related to PTSD symptoms and intrusions were central. Some differences were found within the clinicians’ PSR as well as between the PSR and the empirical network. Still, the general PSR-network structure showed a moderate to good fit to the empirical data.
Conclusion: Our results suggest that sleeping problems and intrusions play a central role in the symptomatology of refugee children, which has tentative implications for diagnostics and treatment. Further, externalizing symptoms might be an indicator for PTSD-symptoms. Finally, using clinicians’ PSR for network construction offered a promising possibility to gain information on symptom networks and their clinical validity.
HIGHLIGHTS
Symptom networks were investigated to understand better refugee youth’s symptomatology.
Intrusions were central; PTSD and depression symptoms related mainly to symptoms in their own domain.
Despite differences, clinicians’ perceptions showed moderate fit to the empirical data.
Antecedentes: En los últimos años, muchos adolescentes han huido de sus países de origen debido a la guerra y a violaciones contra los derechos humanos experimentando, como consecuencia, diversos eventos traumáticos y situándose en riesgo de desarrollar problemas de salud mental. La sintomatología de los refugiados jóvenes mostró ser multifacética y, por lo general, quedando fuera de los diagnósticos tradicionales.
Objetivos: Este estudio tiene por objetivo investigar la sintomatología de este grupo de pacientes evaluando la estructura de redes de un amplio rango de síntomas. Además, evaluamos la percepción de los clínicos respecto a la relación de los síntomas para evaluar la validez clínica del trabajo de redes empírico.
Métodos: Se recolectaron datos empíricos sobre el trastorno de estrés postraumático (TEPT), la depresión y otros síntomas de trauma de N = 366 jóvenes refugiados durante los procesos diagnósticos de rutina de un centro ambulatorio para refugiados jóvenes en Alemania. Además, a cuatro clínicos de este centro ambulatorio se les preguntó sobre cómo percibían las relaciones de los síntomas de sus pacientes empleando una herramienta recientemente desarrollada. Se construyeron redes separadas sobre la base de 1) datos empíricos de síntomas y 2) la percepción de la relación de los síntomas (PRS) de los clínicos.
Resultados: Tanto las redes basadas en datos empíricos como las redes basadas en la PRS de los clínicos mostraron que los síntomas del TEPT y de la depresión se relacionaban con más fuerza con cada racimo respectivo de síntomas (conectados principalmente a través de los problemas de sueño). Los síntomas externalizantes estaban algo relacionados con los síntomas asociados al TEPT mientras que las intrusiones se constituyeron en algo central. Se encontraron algunas diferencias entre las propias PRS’s de los clínicos, así como entre la PRS y las redes empíricas. Aun así, la restructura general de redes basadas en la PRS mostró una correspondencia moderada a buena con los datos empíricos.
Conclusión: Nuestros resultados sugieren que los problemas de sueño y las intrusiones tienen un papel central en la sintomatología de los niños refugiados, lo que tiene implicancias tentativas para el diagnóstico y el tratamiento. Además, los síntomas externalizantes podrían ser un indicador de síntomas asociados al TEPT. Finalmente, empleando la PRS de los clínicos para la construcción de redes brindó una posibilidad prometedora para obtener información sobre las redes de síntomas y su validez clínica.
背景: 近年来, 许多青少年由于战争和侵犯人权行为而逃离了本国, 因此遭受了各种创伤事件, 使他们处于发展出精神健康问题的风险中。难民青年的症状被证明是多方面的, 通常不属于传统诊断范围。
目的: 本研究旨在通过评估各种症状的网络结构来考查此患者群体的症状。此外, 我们评估临床医生对症状关系的看法, 以评估经验网络的临床有效性。
方法:在德国一家难民青年门诊中心的例行诊断过程中, 收集了来自366名难民青年的创伤后应激障碍 (PTSD), 抑郁和其他创伤症状的经验数据。此外, 还询问了该门诊中心的四名临床医生如何使用新开发的工具来了解患者的症状关系。基于1) 经验症状数据和2) 临床医生的感知症状关系 (PSR) 构建了单独的网络。
结果: 基于经验数据的网络和基于临床医生的PSR的网络都表明, PTSD和抑郁症状在各自簇中关系最密切 (主要通过睡眠问题连接), 外化症状在一定程度上与PTSD症状相关, 闯入是核心症状。在临床医生的PSR内以及PSR和经验网络之间发现了一些差异。尽管如此, 一般的PSR网络结构仍显示出与经验数据中等到良好的契合度。
结论: 我们的结果表明, 睡眠问题和闯入在难民儿童的症状中起着核心作用, 这对于诊断和治疗具有探索性意义。此外, 外化症状可能是PTSD症状的指标。最后, 利用临床医生的PSR进行网络构建, 为获得有关症状网络及其临床有效性的信息提供了一个有希望的可能性。
1. Introduction
In 2019, 26 million refugees left their home countries due to violence, conflicts and human rights violations with about half of them being children under the age of 18 (UNHCR, Citation2019). Exposure to war, the flight itself and staying in overcrowded camps put refugee youth at risk of experiencing potentially traumatic events (Hodes & Vostanis, Citation2019). Although a lot of youth show resilience to these experiences, many develop mental health problems, frequently symptoms of post-traumatic stress disorder (PTSD) or depression (Bronstein & Montgomery, Citation2011; Hodes & Vostanis, Citation2019). Repeated interpersonal traumata, e.g. being mistreated or neglected, were shown to cause more complex psychological problems than a single-incident, naturalistic trauma, e.g. experiencing a hurricane (van der Kolk, Ford, & Spinazzola, Citation2019). Further, children and adolescents who experienced repeated interpersonal traumata often show symptoms that fall outside of the classical PTSD domains (D’Andrea, Ford, Stolbach, Spinazzola, & van der Kolk, Citation2012). Additional to symptoms from the classical PTSD symptom clusters (hyperarousal, avoidance, re-experiencing), these youth often display emotional, behavioural, attentional, and physiological regulation difficulties as well as problems in self- and relational regulation (D’Andrea et al., Citation2012; van der Kolk et al., Citation2009). These developmental considerations were acknowledged by the proposed Developmental Trauma Disorder (DTD) (van der Kolk et al., Citation2009), however, not or only partly incorporated in current diagnoses in the ICD-11 and DSM-5 (American Psychiatric Association, Citation2013; Maercker et al., Citation2013). In consequence, children and adolescents who experienced repeated interpersonal traumata often do not fulfil the diagnostic criteria for PTSD and go undetected in clinical practice (D’Andrea et al., Citation2012).
Understanding the complex symptomatology of youth with repeated interpersonal traumata is vital to develop appropriate diagnostics and treatment. Here, the network theory provides a promising approach (Borsboom, Citation2017). Instead of solely investigating the presence or absence of a diagnosis, individual symptoms and their relations can be explored (Borsboom & Cramer, Citation2013). More specifically, network characteristics such as the connections between symptoms (called edges) and symptom centrality, i.e. how many connections a symptom has with other symptoms, are investigated (Borsboom & Cramer, Citation2013). A systematic review of 35 network analyses (Birkeland, Greene, & Spiller, Citation2020) and a network meta-analysis (Isvoranu, Epskamp, & Cheung, Citation2020) of post-traumatic stress symptoms found that, despite some similarities, relations between symptoms and their centrality differed across studies. Such heterogeneous results can be explained by the fact that samples and trauma types varied between studies. Furthermore, previous research showed that experiences of different adverse life events may result in different inter-relations of psychological symptoms (Burger et al., Citation2020; Cramer, Borsboom, Aggen, & Kendler, Citation2012). De Haan et al. (Citation2020) investigated a symptom network of dysfunctional post-traumatic cognitions, post-traumatic stress and depression symptoms of trauma-exposed children and adolescents. They found the symptoms strong or overwhelming emotions, strong physical sensations and difficulty concentrating as most central. However, trauma types varied widely between children in their sample and, therefore, it seems unlikely that specific features of this network are applicable to refugee children, a patient group with specific trauma experiences. To this date, only one study investigated the network of adolescents with experiences of repeated interpersonal traumata (Pfeiffer et al., Citation2019). They found nightmares, psychological reactivity, physiological reactivity and concentration problems as the most central symptoms of refugee youth (Pfeiffer et al., Citation2019). One limitation of this study is that it solely included PTSD symptoms. As potentially traumatic experiences during childhood can lead to a variety of symptoms outside of the classic PTSD symptom cluster, this network analysis might not capture all symptoms that are relevant for refugee youth.
Moreover, despite some research on network-based predictions of psychopathology and treatment effects (Fried & Cramer, Citation2017), information on the clinical validity of empirical networks is limited. It is unclear how networks estimated from empirical data relate to the actual dynamics found in clinical practice, which impedes their applicability to clinical practice. A way forward is to assess how clinicians perceive symptom relations in their patients and comparing these to networks estimated with empirical data (Deserno et al., Citation2020). Due to their direct contact with patients, clinicians likely accumulate many observations of symptoms (relations) and have a good understanding of their patients’ symptoms. Hence, clinicians’ perceived symptom relations (PSR) could provide a valuable source of information for network construction and an overlap between networks estimated with empirical data and clinicians’ PSR could offer initial evidence on their clinical validity.
Although network analysis provides a tool for analysing empirical symptom data, we see a gap: a) in the extent to which current empirical networks of PTSD can be generalized to refugee youth in specific and b) in the extent to which current data models are able to capture important clinical considerations (Burger et al., Citation2020). The present study aimed to gain information on how symptoms of refugee youth relate across a variety of symptoms using the network approach. Furthermore, we aimed to assess clinicians’ PSR and compare these to the network estimated with empirical data in order to investigate the clinical validity of the empirical network.
2. Methods
2.1. Procedure
Empirical data on psychological symptoms were collected during the diagnostic process of the Outpatient Health-Care Center for Refugee Children and Adolescents (OCR) of the University Medical Center Hamburg-Eppendorf. The diagnostic process and the data-collection procedure were approved by the ethics committee of the Chamber of Psychotherapists, Hamburg (05/2017-PTK-HH), and have been described in detail elsewhere (Barthel, Ravens-Sieberer, Schulte-Markwort, Zindler, & Klasen, Citation2019). All refugee children and adolescents who sought treatment in the OCR took part in the standard diagnostic process which was conducted by psychologists, often with the help of interpreters. Their diagnostic data were used for the present study if patients gave their informed consent, if they were at least eleven years old and had experienced at least one interpersonal trauma. The exclusion criteria for the diagnostic process were severe psychological instability (being in acute risk of harming themselves or others or being in direct need of inpatient treatment), alcohol or drug intoxication or acute symptoms of psychosis or suicidality as established by an initial medical consultation.
For collecting information on symptom relations as perceived by clinicians, all four clinicians (psychotherapist or psychiatrists) of the OCR who provided psychotherapy to refugee youth were asked to participate in the study.Footnote1 Clinicians were instructed about the procedure over the phone and entered their Perceived Symptom Relations (PSR) via a newly developed online tool on their computer.
2.2. Materials
Based on Birkeland and colleagues’ recommendation (Birkeland et al., Citation2020) that including similar items should be avoided as they may measure the same latent construct, we chose 20 specific items relevant for refugee youth (see Appendix AFootnote2 for the decision procedure).
2.2.1. PTSD symptoms
Eleven out of the 13 items from the Children’s Revised Impact of Event Scale-13 (Children and War Foundation, Citationn.d.) were included in the network analysis. These items assess self-reported PTSD symptoms of avoidance, intrusion and arousal in the last week on a 4-point Likert scale with answer categories ranging from ‘never’ to ‘often’. The CRIES-13 has been shown to have good internal reliability (Verlinden et al., Citation2014). To assess intrusions and avoidance, the mean score of two intrusion items and mean score of three avoidance items were calculated (resulting in eight PTSD symptoms in total, see Appendix A for details).
2.2.2. Depression symptoms
We chose five out of 14 items from the paediatric PROMIS-depression Item Bank, a self-report screening instrument evaluating depressive symptoms in children and adolescents in the past 7 days (Irwin et al., Citation2010). Items are scored on a 5-point Likert scale from ‘never’ to ‘nearly always’. Good internal reliability and external validity have been shown for this questionnaire (Varni et al., Citation2014).
2.2.3. Externalizing symptoms
Four items from the Strength and Difficulties Questionnaire – Externalizing Scale (SDQ) (Goodman, Citation1997) assessing deviant behaviour, irritability, restlessness and impulsivity over the last 6 months on a 3-point Likert scale were included to incorporate symptoms related to the DTD.
2.2.4. Other trauma symptoms
The sum-score of the Somatic-Symptom Scale-8 (SSS-8) (Gierk et al., Citation2014) which evaluates impairments due to somatic complaints on a 5-point Likert scale in the last week was included. The SSS-8 has been shown to have good reliability as well as construct and factor validity (Gierk et al., Citation2014). Finally, one item assessing the frequency of suicidal thoughts and one item assessing the frequency of self-injury on a 3-point scale (‘never’, ‘sometimes’, ‘often’) in the last 6 months from the Child Behaviour Checklist (CBCL) (Achenbach, Citation1991) were included.
2.2.5. Perceived symptom relations (PSR)
To measure the PSR from clinicians, we developed a new tool utilizing the R package shinyFootnote3 (Chang, Cheng, Allaire, Xie, & McPherson, Citation2020). This tool assessed the relation between all possible 190 symptom pairs of the 20 symptoms that were also included in the empirical network. Clinicians were asked to indicate how two specific symptoms relate to each other. They could choose on a 9-point scale ranging from ‘relate very strongly negative’ to ‘do not relate’ to ‘relate very strongly positive’. When the clinicians indicated a relationship between symptoms, the resulting network was displayed in an adjacent window. An image of the tool is displayed in . Clinicians were asked to base their perception on an ‘average patient’ they would treat in the OCR, emphasizing that we are interested in how symptoms relate in general.
2.3. Statistical analysis
2.3.1. Empirical network
A Gaussian Graphical Model (GGM, i.e. a partial correlation network) was estimated for the empirical data using Spearman correlations between symptoms (Epskamp & Fried, Citation2018). Missing data was handled using pairwise estimation. The gLASSO regularization was applied with a tuning parameter of 0.5 and the Extended Bayesian Information Criterion (EBIC) (Chen & Chen, Citation2008; Friedman, Hastie, & Tibshirani, Citation2008). The strength and expected influence of each symptom were considered. Finally, the stability of the network parameters and centrality indices were calculated using bootstrapping methods (Epskamp, Borsboom, & Fried, Citation2018).
2.3.2. Constructing and comparing PSR-networks
Based on clinicians’ PSR, one network per clinician was constructed (four in total). Clinicians’ indications on the strength of the relation between a symptom pair were used as edges weights (‘do not relate’ = 0, ‘relate weakly positive/negative’ = (−)0.2, ‘relate positive/negative’ = (−)0.5, ‘relate strongly positive/negative’ = (−)0.7, ‘relate very strongly positive/negative’ = (−)1). Through averaging edge weights for each symptom pair an average PSR-network was created. Strength and expected influence of each symptom were investigated for all PSR-networks. To assess the similarity of the individual PSR-networks, the edge weights and centrality indices (strength and expected influence) of all PSR-networks were correlated with each other. Further, to inspect the similarity of the general structure of these networks, we assessed the number of edges on which the individual PSR-networks agreed regarding the presence or absence of the edge.
2.3.3. Comparison of the empirical network and the PSR-networks
The empirical network and the PSR-networks were compared in several analyses. First, edge weights of the PSR-networks were correlated with edge weights of the empirical network (indicating partial correlations). Second, edge weights of the PSR-networks were correlated with the symptom correlations of the empirical data. Third, to compare their general structure, we looked at the agreement regarding the presence versus absence of an edge between the empirical and the PSR-network. Here, the overlap between edges was explored 1) for the empirical network and the average PSR-network including all edges and 2) for the empirical network and the average PSR-network only including edges, with a weight larger than 0.5. Finally, the empirical data were fit to the binary adjacency matrix of each PSR-network (indicating the presence or absence of an edge) using the R packages psychonetrics (Epskamp, Citation2020) and the model fit of each PSR structure was investigated. All analyses were done in R 3.6.1. Code for the complete analysis can be found here https://osf.io/hkdrb/?view_only=b9a7f04dc9684a45a1bed9d1c119b5dd.
3. Results
3.1. Sample description
Between 10/2016 and 06/2020, 398 refugee children and adolescents participated in the diagnostic process in the OCR. Twenty-three of these were younger than 11 years old and nine indicated to have never experienced an interpersonal traumatic event, resulting in a final sample of N = 366. On average, participants were 15.8 years old (SD = 1.93, range = 11–20) and the majority was male (75.7%, n = 277). Nearly half of the adolescents were born in Afghanistan (43.7%, n = 159), and 17% (n = 62) were born in Syria. Almost two-thirds of the adolescents (64.8%, n = 236) fled unaccompanied, whereby the average duration of the flight was 8.7 months (SD = 14.96). When entering the diagnostic process, refugee youth had lived in Germany on average for 20.6 months (SD = 10.65). displays the most common interpersonal traumatic events that adolescents were exposed to. Regarding other types of trauma, 16.25% (n = 58) of the refugee youth also experienced at least one life-threatening disease in their home country, 10.2% (n = 36) during flight and 3.28% (n = 12) in Germany. An accident or a natural disaster was experienced at least once by 39.08% (n = 136) of the sample in their home country, 14.99% (n = 52) during flight and 3.71% (n = 13) in Germany. The number of ICD-10 PTSD and depression diagnoses is shown in and the frequency of each symptom in . In regard to the empirical symptom data, 4.99% of all data points were missing, with the symptom somatic complaints having the most missing data points (n = 99, 27.05%). The exact number of data points per symptom is described in .
Table 1. Number of adolescents exposed to interpersonal-traumatic events
Table 2. Number of adolescents with a PTSD and depression diagnosis
Table 3. Mean scores and standard deviations of included symptoms
The four clinicians of the OCR were on average 38.5 years old and mainly female (75%, n = 3). Two were psychiatrists and two psychotherapists, all four specialized in children and youth therapy and further educated in trauma therapy. Three clinicians were educated in psychodynamic psychotherapy whereas one specialized in behavioural therapy. Their experience with therapy of refugee youth was 1.5 years, 3 years, 5 years and 17 years.
3.2. Empirical network
The network estimated with the empirical data is displayed in . Fifty-four (of 190) edges were present and the average edge weight was 0.02. The network consisted of solely positive edges. Stability assessments showed large confidence intervals for the edge weights and a CS-coefficient of 0.20 (see Appendix B for corresponding graphs). Consequently, only the general structure of the network should be interpreted and centrality indices are not reported (Epskamp & Fried, Citation2018). PTSD and depression symptoms seem to be mostly connected within each cluster and only somewhat between each other. Except defiant behaviour, no externalizing symptom was connected to the network.Footnote4 In an additional exploratory analysis, the network was estimated without regularization using the model selection procedure. In this network, symptoms related most strongly within each cluster, externalizing symptoms were least strongly connected to the network and the symptom intrusions was most central. In a second exploratory analysis, the network was estimated with only the PTSD and depression symptoms (this was done as the stability analysis showed too low power to estimate a stable network for all 20 symptoms). This network showed smaller confidence intervals for the edge weights and a CS-coefficient of 0.44; thus, this estimation seemed somewhat more stable. Again, PTSD and depression symptoms were most strongly connected within their cluster and intrusions had the highest strength. See Appendix C for more information on both additional analyses.
3.3. PSR-networks
The four resulting networks from the clinicians as well as the average PSR-network are displayed in . Clinician A indicated 128/190 edges as present, Clinician B 183/190, Clinician C 173/190 and Clinician D 109/190. The average edge weight of each PSR-network ranged from 0.28 to 0.52, and the median was 0.20 for clinician A and D and 0.50 for B and C. Although the majority of edges were indicated as positive, between one and 16 symptom-pairs per PSR-network were indicated as relating negatively to each other. In the average PSR-network, the average edge weight was 0.36. The symptoms sleeping problems and intrusion of pictures and thoughts showed the highest strength and expected influence whereas the symptoms with the lowest strength and expected influence were somatic complaints and defiant behaviour in the average PSR-network.
Figure 3. PSR-networks. Layout was averaged across networks to ensure comparability. Maximum of edge weights was set to 1
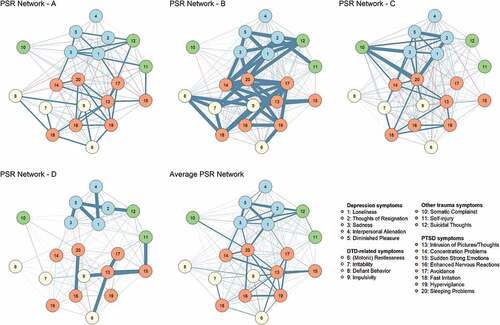
The average correlation between edge weights of the four PSR-networks was r = 0.43. The average correlation of the centrality indices strength and expected influence was r = 0.32 and r = 0.31, respectively. With regard to the presence or absence of an edge, the PSR-networks agreed on average on the absence of 12 edges and the presence of 119 edges of a total of 190 edges. Correlations between each specific PSR-network for edge weights, strength and expected influence are depicted in Appendix B, .
3.4. Comparison of the empirical network and PSR-networks
The edge weights of the average PSR-network (as indicated by clinicians) and the empirical network (indicating partial correlations between symptoms) correlated with r = 0.29. The correlation between the edge weights of the average PSR-network and the symptom correlation of the empirical data was slightly stronger, r = 0.41. displays the comparison between the empirical network and each individual PSR-network. When comparing the presence versus absence of edges, the average PSR-network and the empirical network agreed on the presence of 53 edges and the absence of six edges of the total of 190 edges. The average PSR-network including only strong edges (edge weight >0.5) agreed with the empirical network on the presence of 24 edges and on the absence of 101 of 190 edges. See Appendix B, , for the overlap of edges for each individual PSR-network and the empirical network. When estimating the empirical network without regularization, the edge weights correlated with r = 0.34 with the average PSR-network. Due to the conceptual differences between PSR-networks and empirically estimated networks, it is generally not possible to establish a 1:1 scaling relationship. Clinicians were in principle able to select the maximum value for each possible relationship between any variable while the empirical networks were based on partial-correlations, and therefore generally exhibit less strong relationships.
Table 4. Spearman correlation of the edge weights of the PSR-networks with the edge weights of the empirical network and with the correlation of the empirical data
When the empirical data were fitted on the binary adjacency matrix of the average PSR-network including all indicated edges, most fit indices indicated good fit. The binary adjacency matrix of the average PSR-network including only edges with an edge weight >0.2 showed moderate fit. Poor to moderate fit to the empirical data was shown for the binary adjacency matrix of the average PSR-network including only edges with a weight >0.5. displays the fit indices for the binary adjacency matrix of the average PSR-network and the fit indices of the binary adjacency matrix of each individual PSR-network.
Table 5. Fit indices of binary adjacency matrix of the average PSR-network
Table 6. Fit indices of binary adjacency matrix of each individual PSR-network including all indicated edges
4. Discussion
The complex symptomatology of youth who experienced repeated interpersonal traumata and the limitation of current diagnoses for these patients emphasize the need to better understand the symptomatology of this highly vulnerable group. We aimed to address this need by assessing the symptom network of refugee youth. To assess the clinical validity of the empirical network, we aimed to integrate clinical expertise in this study. In the empirical and in nearly all PSR-networks, it became visible, that PTSD and depression symptoms related most strongly to symptoms within their own domain. Further, externalizing symptoms were somewhat connected to PTSD-symptoms in the PSR-networks and deviant behaviour next to somatic complaints was least central in the average PSR-network. In contrast, intrusions and sleeping problems were shown to be related to many symptoms, i.e. were highly central, both in the empirical and in the PSR-networks. Finally, the low to moderate associations between the edge weights of the empirical and PSR-networks indicated differences between these networks. More specifically, while their general structure seems to be roughly similar, the PSR-networks had, for example, a much higher density than the empirical network.
4.1. Symptoms network of refugee youth
The empirical and PSR-networks showed that depression and PTSD symptoms related most strongly within their respective cluster, which aligns with previous findings in trauma-exposed children (De Haan et al., Citation2020). This contrasts the often-reported high comorbidity of depression and PTSD (Stander, Thomsen, & Highfill-McRoy, Citation2014) which would suggest that depression and PTSD symptoms are highly related (Borsboom, Citation2017). From the network perspective, an explanation for a high comorbidity of both disorders despite separate symptom clusters are bridge symptoms, i.e. symptoms that connect different symptom clusters with each other. The PSR-networks suggest sleeping problems as a potential bridge symptom, since it is highly central and connected to both symptom clusters. These exploratory results could suggest the following mechanisms: A traumatic event might activate different PTSD symptoms, possibly leading to the onset of sleeping problems, which might lead to the activation of depression symptoms. This would align with theories proposing a causal relationship between PTSD and depression as the reason for their comorbidity. Note, however, that causal interpretations regarding treatment effects remain putative, given that the present study is based on cross-sectional between-subject data. These interpretations need to be tested with future longitudinal within-person designs. In their review, Stander and colleagues found fairly consistent evidence that PTSD is a risk factor for subsequent depression (Stander et al., Citation2014). In contrast, some theories suggest common risk factors between both depression and PTSD (Stander et al., Citation2014). This could mean that sleeping problems might sustain both PTSD and depression symptoms. Supporting this speculation, a longitudinal study showed that insomnia preceded and predicted both PTSD and depression symptoms in a later timepoint in combat-experienced soldiers (Wright et al., Citation2011). In regard to clinical implications, one possible interpretation is that the assessment and early treatment of sleeping problems might be helpful to reduce the activation of depression symptoms and/or alleviate both PTSD and depression symptoms. A meta-analysis showed that sleep-specific cognitive behavioural therapy reduced both PTSD and depression symptoms (Ho, Chan, & Tang, Citation2016).
We also found that clinicians perceived externalizing symptoms as connecting mainly to PTSD symptoms but generally as only weakly connected to the symptom network with deviant behaviour being the symptom with the lowest strength in the PSR-networks. Importantly, this does not imply that externalizing symptoms are not severe in this sample. Externalizing problems were still related to PTSD symptomsFootnote5 (in contrast to nearly no relation to depression symptoms), i.e. PTSD and externalizing symptoms seem to co-occur. Consequently, it might be helpful if refugee youth who display externalizing problems would be screened for PTSD symptoms. Since externalizing symptoms are likely to be more visible to others (e.g. to teachers or classmates), taking these symptom relations into account could offer an early indication of PTSD symptoms. Interestingly, next to deviant behaviour, somatic complaints were least central in the PSR-networks. This suggests that clinicians do not perceive somatic complaints co-occurring often with PTSD and depression symptoms and therefore, information on somatic complaints seems to give little information on the likelihood of PTSD symptoms and vice versa.
Finally, according to PSR-networks, sleeping problems and intrusions were most central in the symptom network of refugee youth. As discussed above, sleeping problems might be an important bridge symptom between depression and PTSD symptoms. Intrusions were also central in the empirical network. This aligns with the review of network studies on post-traumatic stress symptoms which found ‘recurrent thought of trauma’ as one of the most central symptoms in a majority of their studies (Birkeland et al., Citation2020). The importance of intrusions is also pointed out in cognitive theories of PTSD, stating that intrusions can cause strong negative emotional reactions which further leads to avoidance and an increased sensitivity to trauma cues (Ehlers & Clark, Citation2000; Rubin, Berntsen, & Bohni, Citation2008). As intrusions seem to relate to many other symptoms, reducing intrusions during therapy might be helpful to reduce activation of other relating symptoms. However, our centrality findings contrast to the previous network analysis of refugee youth (Pfeiffer et al., Citation2019). This emphasizes that future studies need to replicate current findings and/or test them in a confirmatory way. More generally, due to the novelty of the network analytic tools and the fact that concepts like centrality need to be further validated, practical clinical implications need to be formulated with great caution (Blanchard & Heeren, Citation2020). Still, symptom networks can provide a better understanding of psychopathology in specific groups beyond DSM-based diagnostic categories. Using clinical expertise alongside empirical networks was an initial step for the validation of network concepts within clinical practice and enabled us to provide some cautious implication for clinical practice.
4.2. Clinical perceptions and empirical networks
The individual PSR-networks agreed on the presence/absence on more than 60% of edges, providing some evidence for similarity between these networks. However, the low to moderate association between edge weights of the individual PSR-networks also suggests differences in how clinicians perceived symptom relations. Such differences could be, for example, caused by different specializations or years of working experience and should be explored in future studies with more clinicians.
The structure of the empirical network and the PSR-networks were only weakly associated, suggesting dissimilarity between these networks and might question the clinical validity of the empirical network. Deserno and colleagues (Deserno et al., Citation2020) also found a low association between edge weights of the clinician rated Perceived Causal Relations between symptoms and their empirical network. This could have several reasons. First, in our study, the empirical network was instable which means that power was possibly too low to recover the ‘true’ underlying model. Consequently, differences could be due to false positive or false negative edges in the empirical network. Second, as LASSO regularization leads to a sparse structure in the estimation of networks regardless of the true underlying model and this opposes the strong density of the PSR-networks, network differences could be caused by this regularization method (Deserno et al., Citation2020; Epskamp, Kruis, Marsman, & Marinazzo, Citation2017). However, using model selection instead of regularization for network estimation did not meaningfully increase the correlation between the PSR- and the empirical networks. Third, it is unclear what exactly the PSR from clinicians represent. Our results suggest that the PSR might represent unconditional compared to conditional relationships as PSR were more similar to the symptom correlations (i.e. unconditional relations), than to partial correlations (i.e. conditional relations). Further, instructing clinicians to think of an average patient might have triggered them to think of symptom relations within a patient. As the empirical network in our study assesses between-person-processes, differences between the PSR- and the empirical networks might be due to the fact that they assess differing concepts. Thus, future studies need to closer investigate whether PSR conceptualize within- or between-person-processes. Finally, investigating the model fit of the general structures from the PSR-networks showed that these were somewhat consistent with the empirical data offering initial support that PSRs indicate relations that are also observable in empirical data. Taken together, it stays somewhat unclear how empirical networks relate to clinical perceptions. Still, assessing PSR was shown valuable as important clinical considerations could be investigated.
4.3. Limitations
Various limitations have to be considered when interpreting this study. First, due to the sample size, the empirical network showed some instability; therefore, it should be interpreted with caution. Second, because of its novelty, the reliability and validity of assessing PSR with the new tool remain somewhat unclear and need to be investigated in future studies. Third, we only assessed four clinicians which limits the generalizability of the PSR-networks. Fourth, the networks did not include dissociative symptoms and symptoms from every domain of the DTD despite their clinical relevance. This was due to the low reliability of the instrument assessing dissociation and the absences of an instrument that assesses all DTD-related symptoms. Finally, as we solely analysed cross-sectional data and undirected symptom relations, no causality can be inferred.
4.4. Conclusion and future outlook
Investigating symptom networks of refugee youth based on empirical data and clinicians’ perceptions enabled us to gain insight into the symptomatology of youth with experiences of repeated interpersonal traumata. PTSD and depression symptoms were shown to mainly relate to symptoms within their cluster, sleeping problems could be a bridge symptom between both clusters and intrusions were shown to be central. Considering clinical validity through assessing clinicians’ PSR was shown to be promising as information from the instable empirical network could be enriched with clinical knowledge. Future studies need to investigate the reliability and validity of the proposed PSR and their assessment. Moreover, as the current study was exploratory, findings in regard to symptomatology of refugee youth need to be replicated and tested in a confirmatory way. An important next step is to formulate a theory on the effects of repeated, interpersonal traumata in adolescents (Robinaugh, Hoekstra, Toner, & Borsboom, Citation2020) and using sources of information beyond network-based empirical symptom data can play an important role here (Burger et al., Citation2020). A thorough understanding of symptomatology of refugee youth will help to further develop better diagnostics and therapies, which can hopefully help to reduce suffering of this vulnerable group.
Open-materials
This article has earned the Center for Open Science badge for Open Materials. The materials are openly accessible at https://osf.io/hkdrb/?view_only=b9a7f04dc9684a45a1bed9d1c119b5dd.
Acknowledgments
We would like to thank all refugee children and adolescents that took part in this study. Further, we would like to thank all four clinicians of the OCR for taking their time to participate in this study. We thank the psychologists of the OCR who conducted the diagnostic interviews and the students who entered the empirical data from these interviews.
Disclosure statement
No potential conflict of interest was reported by the author(s).
Data availability statement
Since participants did not give written consent for providing their data to researchers who are not part of this study group, data are available upon request for researchers after consultation with the corresponding author, Lea Schumacher.
Additional information
Funding
Notes
1. Of note, common considerations regarding sample-size do not apply to this type of modelling, since each clinician likely based their estimates on many accumulated observations.
2. All appendices can be found under ‘Appendices’ here https://osf.io/hkdrb/?view_only=b9a7f04dc9684a45a1bed9d1c119b5dd
3. Available here: https://ls-apps.shinyapps.io/Symptom-Netzwerk/; Code and clinicians’ manual for this tool are available here https://osf.io/hkdrb/?view_only=b9a7f04dc9684a45a1bed9d1c119b5dd
4. Of note, the assessment timeframe differed between the externalizing symptoms, self-injury and suicidal thought (‘last six months’) and all the other symptoms (‘past seven days’). Although data were collected at one cross-section of time, it needs to be noted that the interpretation of relationships between symptoms reflects retrospective self-reports spanning across different scales, i.e. relationships indicate associations between symptoms that occurred within the last six months and symptoms that occurred within the past seven days.
5. The lack of relations between the externalizing symptoms and all other symptoms in the empirical network might be due to low power.
References
- Achenbach, T. M. (1991). Manual for the child behavior checklist/4-18 and 1991 profile. Burlington, VT: University of Vermont.
- American Psychiatric Association. (2013). Diagnostic and statistical manual of mental disorders (5th ed.). Washington, DC: Author. doi:10.1176/appi.books.9780890425596.
- Barthel, D., Ravens-Sieberer, U., Schulte-Markwort, M., Zindler, A., & Klasen, F. (2019). Klinisch-psycologische Diagnostik in einer Flüchtlingsambulanz für Kinder und Jugendliche. Kindheit und Entwicklung, 28(3), 160–12. doi:10.1026/0942-5403/a000286
- Birkeland, M. S., Greene, T., & Spiller, T. R. (2020). The network approach to posttraumatic stress disorder: A systematic review. European Journal of Psychotraumatology, 11(1), 1700614. doi:10.1080/20008198.2019.1700614
- Blanchard, M. A., & Heeren, A. (2020). Ongoing and future challenges of the network approach to psychopathology: From theoretical conjectures to clinical translations. In G. Asmundson (Ed.), Reference module in neuroscience and biobehavioral psychology (2nd ed., pp. B9780128186978000000). Elsevier. doi:10.1016/B978-0-12-818697-8.00044-3.
- Borsboom, D. (2017). A network theory of mental disorders. World Psychiatry, 16(1), 5–13. doi:10.1002/wps.20375
- Borsboom, D., & Cramer, A. O. (2013). Network analysis: An integrative approach to the structure of psychopathology. Annual Review of Clinical Psychology, 9(1), 91–121. doi:10.1146/annurev-clinpsy-050212-185608
- Bronstein, I., & Montgomery, P. (2011, March 1). Psychological distress in refugee children: A systematic review. Clinical Child and Family Psychology Review, 14(1), 44–56. doi:10.1007/s10567-010-0081-0
- Burger, J., Stroebe, M. S., Perrig-Chiello, P., Schut, H. A., Spahni, S., Eisma, M. C., & Fried, E. I. (2020, April 15). Bereavement or breakup: Differences in networks of depression. Journal of Affective Disorders, 267, 1–8. doi:10.1016/j.jad.2020.01.157
- Burger, J., van der Veen, D. C., Robinaugh, D. J., Quax, R., Riese, H., Schoevers, R. A., & Epskamp, S. (2020, December). Bridging the gap between complexity science and clinical practice by formalizing idiographic theories: A computational model of functional analysis. BMC Medicine, 18(1), 99. doi:10.1186/s12916-020-01558-1
- Chang, W., Cheng, J., Allaire, J. J., Xie, Y., & McPherson, J. (2020). Shiny: Web application framework for R [Internet]. Retrieved from https://CRAN.R-project.org/package=shiny
- Chen, J., & Chen, Z. (2008). Extended Bayesian information criteria for model selection with large model spaces. Biometrika, 95(3), 759–771. doi:10.1093/biomet/asn034
- Children and War Foundation. (n.d.). Measures: Cries-13 [Internet]. Retrieved from https://www.childrenandwar.org/projectsresources/measures/
- Cramer, A. O. J., Borsboom, D., Aggen, S. H., & Kendler, K. S. (2012, May). The pathoplasticity of dysphoric episodes: Differential impact of stressful life events on the pattern of depressive symptom inter-correlations. Psychological Medicine, 42(5), 957–965. doi:10.1017/S003329171100211X
- D’Andrea, W., Ford, J., Stolbach, B., Spinazzola, J., & van der kolk, B. A. (2012). Understanding interpersonal trauma in children: Why we need a developmentally appropriate trauma diagnosis. American Journal of Orthopsychiatry, 82(2), 187. doi:10.1111/j.1939-0025.2012.01154.x
- De Haan, A., Landolt, M. A., Fried, E. I., Kleinke, K., Alisic, E., Bryant, R., … Meiser‐Stedman, R. (2020). Dysfunctional posttraumatic cognitions, posttraumatic stress and depression in children and adolescents exposed to trauma: A network analysis. Journal of Child Psychology and Psychiatry, 61(1), 77–87. doi:10.1111/jcpp.13101
- Deserno, M., Borsboom, D., Begeer, S., van Bork, R., Hinne, M., Geurts, H., & Santana, G. L. (2020, December). Highways to happiness for autistic adults? Perceived causal relations among clinicians. PLoS One, 15(12), e0243298. doi:10.1371/journal.pone.0243298
- Ehlers, A., & Clark, D. M. (2000). A cognitive model of posttraumatic stress disorder. Behaviour Research and Therapy, 38(4), 319–345. doi:10.1016/S0005-7967(99)00123-0
- Epskamp, S. (2020). Psychonetrics: Structural equation modeling and confirmatory network analysis [Internet]. Retrieved from https://CRAN.R-project.org/package=psychonetrics
- Epskamp, S., Borsboom, D., & Fried, E. I. (2018). Estimating psychological networks and their accuracy: A tutorial paper. Behavior Research Methods, 50(1), 195–212. doi:10.3758/s13428-017-0862-1
- Epskamp, S., & Fried, E. I. (2018, December). A tutorial on regularized partial correlation networks. Psychological Methods, 23(4), 617–634. doi:10.1037/met0000167
- Epskamp, S., Kruis, J., Marsman, M., & Marinazzo, D. (2017, June). Estimating psychopathological networks: Be careful what you wish for. PLoS ONE, 12(6), e0179891. doi:10.1371/journal.pone.0179891
- Fried, E. I., & Cramer, A. O. J. (2017). Moving forward: Challenges and directions for psychopathological network theory and methodology. Perspectives on Psychological Science, 12(6), 999–1020. doi:10.1177/1745691617705892
- Friedman, J., Hastie, T., & Tibshirani, R. (2008). Sparse inverse covariance estimation with the graphical lasso. Biostatistics, 9(3), 432–441. doi:10.1093/biostatistics/kxm045
- Gierk, B., Kohlmann, S., Kroenke, K., Spangenberg, L., Zenger, M., Brähler, E., & Löwe, B. (2014). The somatic symptom scale–8 (SSS-8): A brief measure of somatic symptom burden. JAMA Internal Medicine, 174(3), 399–407. doi:10.1001/jamainternmed.2013.12179
- Goodman, R. (1997). The strengths and difficulties questionnaire: A research note. Journal of Child Psychology and Psychiatry, 38(5), 581–586. doi:10.1111/j.1469-7610.1997.tb01545.x
- Ho, F. Y. Y., Chan, C. S., & Tang, K. N. S. (2016, February 1). Cognitive-behavioral therapy for sleep disturbances in treating posttraumatic stress disorder symptoms: A meta-analysis of randomized controlled trials. Clinical Psychology Review, 43, 90–102. doi:10.1016/j.cpr.2015.09.005
- Hodes, M., & Vostanis, P. (2019, July). Practitioner review: Mental health problems of refugee children and adolescents and their management. Journal of Child Psychology and Psychiatry, 60(7), 716–731. doi:10.1111/jcpp.13002
- Irwin, D. E., Stucky, B., Langer, M. M., Thissen, D., DeWitt, E. M., Lai, J.-S., & DeWalt, D. A. (2010). An item response analysis of the pediatric PROMIS anxiety and depressive symptoms scales. Quality of Life Research, 19(4), 595–607. doi:10.1007/s11136-010-9619-3
- Isvoranu, A.-M., Epskamp, S., & Cheung, M. (2020). Network models of post-traumatic stress disorder: A meta-analysis [Internet]. PsyArXiv. Retrieved from https://doi.org/10.31234/osf.io/8k4u6
- Maercker, A., Brewin, C. R., Bryant, R. A., Cloitre, M., van Ommeren, M., Jones, L. M., & Reed, G. M. (2013). Diagnosis and classification of disorders specifically associated with stress: Proposals for ICD-11. World Psychiatry, 12(3), 198–206. doi:10.1002/wps.20057
- Pfeiffer, E., Sukale, T., Müller, L. R. F., Plener, P. L., Rosner, R., Fegert, J. M., … Unterhitzenberger, J. (2019). The symptom representation of posttraumatic stress disorder in a sample of unaccompanied and accompanied refugee minors in Germany: A network analysis. European Journal of Psychotraumatology, 10(1), 1675990. doi:10.1080/20008198.2019.1675990
- Robinaugh, D. J., Hoekstra, R. H., Toner, E. R., & Borsboom, D. (2020). The network approach to psychopathology: A review of the literature 2008–2018 and an agenda for future research. Psychological Medicine, 50(3), 353–366. doi:10.1017/S0033291719003404
- Rubin, D. C., Berntsen, D., & Bohni, M. K. (2008, October). A memory-based model of posttraumatic stress disorder: Evaluating basic assumptions underlying the PTSD diagnosis. Psychological Review, 115(4), 985–1011. doi:10.1037/a0013397
- Stander, V. A., Thomsen, C. J., & Highfill-McRoy, R. M. (2014). Etiology of depression comorbidity in combat-related PTSD: A review of the literature. Clinical Psychology Review, 34(2), 87–98. doi:10.1016/j.cpr.2013.12.002
- UNHCR. (2019). Global trends: Forced displacement in 2019 [Internet]. Retrieved from https://www.unhcr.org/5ee200e37.pdf
- van der Kolk, B., Ford, J. D., & Spinazzola, J. (2019, December 31). Comorbidity of developmental trauma disorder (DTD) and post-traumatic stress disorder: Findings from the DTD field trial. European Journal of Psychotraumatology, 10(1), 1562841. doi:10.1080/20008198.2018.1562841
- van der Kolk, B. A., Pynoos, R. S., Cicchetti, D., Cloitre, M., D’Andrea, W., Ford, J. D., Lieberman, A. F., Putnam, F. W., Saxe, G., Spinazzola, J., Stolbach, B. C., & Teicher, M. (2009). Proposal to include a developmental trauma disorder diagnosis for children and adolescents in DSM-V [Internet]. Retrieved from http://www.cathymalchiodi.com/dtd_nctsn_pdf
- Varni, J. W., Magnus, B., Stucky, B. D., Liu, Y., Quinn, H., Thissen, D., & DeWalt, D. A. (2014). Psychometric properties of the PROMIS® pediatric scales: Precision, stability, and comparison of different scoring and administration options. Quality of Life Research, 23(4), 1233–1243. doi:10.1007/s11136-013-0544-0
- Verlinden, E., van Meijel, E. P., Opmeer, B. C., Beer, R., de Roos, C., Bicanic, I. A., & Lindauer, R. J. L. (2014). Characteristics of the children’s revised impact of event scale in a clinically referred Dutch sample. Journal of Traumatic Stress, 27(3), 338–344. doi:10.1002/jts.21910
- Wright, K. M., Britt, T. W., Bliese, P. D., Adler, A. B., Picchioni, D., & Moore, D. (2011). Insomnia as predictor versus outcome of PTSD and depression among Iraq combat veterans. Journal of Clinical Psychology, 67(12), 1240–1258. doi:10.1002/jclp.20845