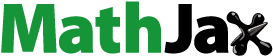
ABSTRACT
Objective: The effects of maternal exposure to adverse childhood experiences (ACEs) may be transmitted to subsequent generations through various biopsychosocial mechanisms. However, studies tend to focus on exploring one or two focal pathways with less attention paid to links between different pathways. Using a network approach, this paper explores a range of core prenatal risk factors that may link maternal ACEs to infant preterm birth (PTB) and low birthweight (LBW).
Methods: We used data from the Avon Longitudinal Study of Parents and Children (ALSPAC) (n = 8379) to estimate two mixed graphical network models: Model 1 was constructed using adverse infant outcomes, biopsychosocial and environmental risk factors, forms of ACEs, and sociodemographic factors. In Model 2, ACEs were combined to represent a threshold ACEs score (≥4). Network indices (i.e., shortest path and bridge expected influence [1-step & 2-step]) were estimated to determine the shortest pathway from ACEs to infant outcomes, and to identify the risk factors that are vital in activating other risk factors and adverse outcomes.
Results: Network analyses estimated a mutually reinforcing web of childhood and prenatal risk factors, with each risk connected to at least two other risks. Bridge influence indices suggested that childhood physical and sexual abuse and multiple ACEs were highly interconnected to others risks. Overall, risky health behaviours during pregnancy (i.e., smoking & illicit drug use) were identified as ‘active’ risk factors capable of affecting (directly and indirectly) other risk factors and contributing to the persistent activation of the global risk network. These risks may be considered priority candidate targets for interventions to disrupt intergenerational risk transmission. Our study demonstrates the promise of network analysis as an approach for illuminating the intergenerational transmission of adversity in its full complexity.
HIGHLIGHTS
We took a network approach to assessing links between ACEs and birth outcomes.
ACEs, other prenatal risk factors, and birth outcomes had complex inter-connections
Health behaviours in pregnancy were indicated as optimal intervention targets.
Objetivo: Los efectos de la exposición materna a experiencias adversas en la infancia (ACEs, en sus siglas en inglés) pueden ser transmitidos a las generaciones posteriores a través de varios mecanismos biopsicosociales. Sin embargo, los estudios tienden a centrarse en la exploración de una o dos vías focales, prestando menos atención a los vínculos entre diferentes vías. Utilizando un abordaje de red, este trabajo explora una serie de factores de riesgo prenatales centrales que pueden vincular las ACEs maternas con el nacimiento prematuro (PTB, en sus siglas en inglés) y el bajo peso al nacer (LBW, en sus siglas en inglés) de los bebés.
Métodos: Se utilizaron datos del Estudio Longitudinal de Padres e Hijos de Avon (ALSPAC) (n = 8.379) para estimar dos modelos de red gráfica mixta: El modelo 1 se construyó utilizando los resultados adversos del lactante, los factores de riesgo biopsicosociales y ambientales, las formas de las ACE y los factores sociodemográficos. En el modelo 2, las ACEs se combinaron para representar una puntuación de ACEs umbral (≥ 4). Se estimaron los índices de red (es decir, el camino más corto y la influencia esperada del puente [1 y 2 pasos]) para determinar el camino más corto desde las ACEs hasta los resultados infantiles, y para identificar los factores de riesgo que son vitales para activar otros factores de riesgo y resultados adversos.
Resultados: Los análisis de redes estimaron una red de factores de riesgo prenatales y de la infancia que se refuerzan mutuamente, y cada riesgo está conectado con al menos otros dos riesgos. Los índices de influencia de los puentes sugirieron que el abuso físico y sexual en la infancia y los múltiples ACEs estaban altamente interconectados con otros riesgos. En general, las conductas de riesgo para la salud durante el embarazo (es decir, el tabaquismo y el consumo de drogas ilícitas) se identificaron como factores de riesgo "activos" capaces de afectar (directa e indirectamente) a otros factores de riesgo y de contribuir a la activación persistente de la red de riesgo global. Estos riesgos pueden considerarse objetivos candidatos prioritarios para las intervenciones destinadas a interrumpir la transmisión intergeneracional del riesgo. Nuestro estudio demuestra la promesa del análisis de redes como abordaje para iluminar la transmisión intergeneracional de la adversidad en toda su complejidad.
目的:母亲暴露于不良童年期经历 (ACE) 的影响可能会通过各种生物心理社会机制传递给后代。然而,研究往往侧重于探索一两个焦点途径,而较少关注不同途径之间的联系。使用网络方法,本文探讨了一系列可能将母体 ACE 与婴儿早产 (PTB) 和低出生体重 (LBW) 联系起来的核心产前风险因素。
方法:我们使用来自雅芳父母和儿童纵向研究 (ALSPAC) (n = 8,379) 的数据来估计两个混合图形网络模型:模型 1 是使用不良婴儿结果、生物心理社会和环境风险因素、ACE 形式以及社会人口因素。在模型 2 中,ACE 被组合以表示阈值 ACE 分数(≥ 4)。估计了网络指标(即最短路径和桥梁预期影响 [1 步和 2 步])以确定从 ACE 到婴儿结果的最短路径,并确定对激活其他风险因素和不利因素至关重要的风险因素结果。
结果:网络分析估计了一个由童年期和产前风险因素组成的相互加强的网络,每个风险都与至少两个其他风险相关。桥影响指标表明,童年期身体和性虐待以及多个 ACE 与其他风险高度相关。总体而言,怀孕期间的风险健康行为(即吸烟和非法药物使用)被确定为能够(直接和间接)影响其他风险因素并有助于全局风险网络持续激活的‘活跃’风险因素。这些风险可被视为干扰代际风险传递干预措施的优先候选目标。我们的研究证明了网络分析作为一种阐明逆境代际传递全部复杂性的前景。
1. Background
One of the United Nations’ sustainable development goals (target indicator 3.2) for 2030 is to reduce preventable deaths of neonates and children under 5 years of age globally (United Nations Department of Economic and Social Affairs, Citation2016). Preterm birth (PTB) (births before 37 weeks of gestation) and low birthweight (LBW) (birthweight <2500 grams) remain among the leading causes of child morbidity and mortality (Vogel et al., Citation2018; World Health Organization, Citation1977) and are associated with both short- and long-term consequences throughout the lifespan (Goldenberg & Culhane, Citation2007). The pathogenesis of these adverse birth outcomes has been linked to a number of interconnected and potentially interacting prenatal risk factors, such as maternal exposure to teratogenic agents (e.g. tobacco, illicit substances, air pollutants), psychological distress, psychosocial stressors (e.g. financial difficulties), and biological complications (e.g. pre-eclampsia, infections) (Goldenberg & Culhane, Citation2007; Goldenberg, Culhane, Iams, & Romero, Citation2008; Macdonald-Wallis et al., Citation2015; Padula, Rivera-Núñez, & Barrett, Citation2020). Prenatal life is a critical phase for foetal programming and a highly sensitive period for both maternal and foetal biology (Buss et al., Citation2017; Moog et al., Citation2016). Thus, exposure to the aforementioned risk factors may cause alterations in the gestational environment which could result in deficits in foetal development (Buss et al., Citation2017; Goldenberg et al., Citation2008; Goldenberg & Culhane, Citation2007; Moog et al., Citation2016).
Maternal exposure to adverse childhood experiences (ACEs), characterised by forms of maltreatment (e.g. physical abuse, neglect) and household dysfunction (e.g. parental incarceration), have also been directly linked to both infant outcomes; however, findings are inconsistent (Benedict, Paine, Paine, Brandt, & Stallings, Citation1999; Blackmore et al., Citation2016; Gavin, Thompson, Rue, & Guo, Citation2012; Smith, Gotman, & Yonkers, Citation2016). The prenatal risk factors mentioned above, along with genetic, epigenetic and other environmental factors, are posited as pathways of transmission of ACE-related sequelae from mother to infant (Asmussen, Fischer, Drayton, & McBride, Citation2020; Buss et al., Citation2017). However, studies tend to focus on exploring one or two focal pathways with less attention paid to the interconnectivity and co-occurrence of these individual-, interpersonal-, and macro-level risks.
Drawing from graph theory and network science, network analysis is a statistical technique in which conditional independence relationships of all variables are modelled and visualised in the form of a graph with nodes depicting variables and edges representing the relations between variables (Haslbeck and Waldorp, Citation2015; Epskamp & Fried, Citation2018). Through this, the interrelationships between exposure variables can be estimated which may lead to the identification of unique interrelations among variables that cannot be identified in traditional analyses. Such relations are, for example, more challenging to discern in techniques such as multiple regression analyses, which tend to examine the relationships between one focal outcome and multiple predictor variables while largely ignoring the shared relations between predictors (Epskamp & Fried, Citation2018). Compared to regression models, network analysis is a more integrative approach through which complex multidimensional chains of risks can be analysed. For instance, network analysis allows for the identification of factors that overall have the biggest potential for shaping the rest of the network (the most central and/or most well-connected nodes sharing the most edges with other nodes in the model). These factors potentially represent valuable intervention targets, as positive changes in these risk factors may subsequently have positive influences on other factors analysed in the model.
Network models are commonly used in psychology to abstract connections among symptoms (Robinaugh, Hoekstra, Toner, & Borsboom, Citation2020). They are also used in epidemiologic studies to identify risk factors for disease outcomes (Jin, Zhou, Xu, & An, Citation2017; Khan, Uddin, & Srinivasan, Citation2019; Pereira-Morales, Adan, & Forero, Citation2019; Speyer et al., Citation2021) and predict the spread of infectious diseases (Loyal & Chen, Citation2020). Despite its promise, thus far, only a few studies have used a network approach to investigate the interrelatedness of ACEs (Vial, van der Put, Stams, Kossakowski, & Assink, Citation2020) and the relationships between ACEs and poor health outcomes in adulthood (Breuer, Greggersen, Kahl, Schweiger, & Westermair, Citation2020; Haselgruber, Knefel, Sölva, & Lueger-Schuster, Citation2021; Kheirabad et al., Citation2021; Schouw, Verkes, Schene, & Schellekens, Citation2020; Vial et al., Citation2020). For instance, Breuer and colleagues examined the direct relations between ACEs and adult mental ill health. They found high connectivity between all ACEs with each node positively associated with at least two other ACEs. They also identified neglect and domestic violence exposure as influential factors that mediate connections between ACEs and that childhood sexual abuse was the only link between ACEs and adult mental ill health (Breuer et al., Citation2020). The authors inferred that neglect and domestic violence exposure could be optimal candidate targets for interventions against ACEs while interventions against sexual abuse may increase the likelihood of preventing negative long-term mental ill health. Similarly, network analysis has been used to unravel complex relationships between ACEs and differential effects of distinct ACEs on various personality dimensions (Schouw et al., Citation2020). The authors found that childhood physical abuse and maternal rejection played a central role in shaping personality. Similarly, Pereira-Morales and colleagues examined the relations between multiple risk factors for mental illness in adulthood (Pereira-Morales et al., Citation2019). They found a positive association between childhood sexual abuse and higher levels of perceived distress in adulthood, and a positive association between emotional neglect and problematic alcohol consumption in adulthood. In contrast with previous studies that used a selective approach (single ACE) or a cumulative approach (using the de facto ≥ 4 ACEs threshold score) to examine relations between ACEs and outcomes, network models have demonstrated a much sparser association pattern and at the same time illuminated complex relations between multiple risks and outcomes (Breuer et al., Citation2020; Pereira-Morales et al., Citation2019).
The aforementioned studies (Breuer et al., Citation2020; Pereira-Morales et al., Citation2019; Schouw et al., Citation2020) used centrality metrics (i.e. strength, betweenness, closeness) which are typically used in network analysis to quantify the relative importance of each node in relation to the whole network structure (Epskamp, Borsboom, & Fried, Citation2018; Opsahl, Agneessens, & Skvoretz, Citation2010). Nodes with high centrality are thought to be influential nodes and it is suggested that identification and accurate characterisation of these central nodes may be vital in developing intervention strategies (Jones, Ma, & McNally, Citation2021; Robinaugh et al., Citation2020). However, other studies have shown that the use of these metrics has limited utility, betweenness and closeness centrality, in particular, have been shown to be ill-suited to most psychological networks (Bringmann et al., Citation2019). As such, it is crucial that the centrality measures used are tailored to the network context so that the conclusions drawn are fair representations of the process under study (Bringmann et al., Citation2019). This study aims to explore the interrelationships between maternal childhood and prenatal risk factors and poor infant outcomes.
2. Methods
2.1. Study design and population
This study was a secondary data analysis of the Avon Longitudinal Study of Parents and Children (ALSPAC) dataset. Pregnant women residents in Avon, UK with expected dates of delivery 1st April 1991 to 31st December 1992 were invited to take part in the study. The initial number of pregnancies enrolled is 14,541 (for these at least one questionnaire has been returned or a ‘Children in Focus’ clinic had been attended by 19/07/99). Of these initial pregnancies, there was a total of 14,676 foetuses, resulting in 14,062 live births and 13,988 children who were alive at 1 year of age (Boyd et al., Citation2013; Fraser et al., Citation2013). After excluding participants without obstetric records, with multiple pregnancies (twin, triplet, etc.), and still births, the final sample was 8379. Project details, data documentation, and specific details on ethical approval are available on the ALSPAC study website: https://www.bristol.ac.uk/alspac. Further, please note that the study website contains details of all the data that is available through a fully searchable data dictionary and variable search tool: http://www.bristol.ac.uk/alspac/researchers/our-data/.
2.2. Variables and measurement
2.2.1. Outcomes
Infant preterm birth (PTB) and low birthweight (LBW) were derived from obstetric records and recoded into dichotomous variables (yes/no).
2.2.2. ACEs
Maternal ACEs were measured using a 31-item checklist and 12-item checklist as part of self-reported questionnaires gathered at 32 weeks gestation and 33-months postpartum, respectively (Ellis, Iles-Caven, Northstone, & Golding, Citation2020; The ALSPAC Study Team, Citation2018). Ten items were used to represent types of ACEs: physical, emotional, and sexual abuse, physical neglect, emotional neglect, parental incarceration, parental death, parental separation, parental divorce, and having had a parent with mental illness. The ALSPAC Study Team recoded these items into dichotomous variables (yes/no) (The ALSPAC Study Team, Citation2018). For this analysis, parental death, separation, or divorce were aggregated into one dichotomous variable (yes = if ≥ 1 domain was experienced), which follows the World Health Organization guidelines for coding ACEs (World Health Organization, Citation2014). Finally, a threshold (≥ 4) ACEs variable was derived by computing the ACE sum score and collapsing it into a dichotomous variable (yes/no). This was done for comparison with previous research that commonly take this approach to defining ACE exposure e.g. (Hughes et al., Citation2017).
2.2.3. Prenatal risk factors
Fifteen variables, gathered between 18- and 32-weeks of gestation through self-completion questionnaires, were used to represent potential prenatal risk factors.
2.2.3.1. Psychosocial risk factors
Two items were used to represent psychosocial risk factors: exposure to discrimination and financial difficulties. Mothers were asked if they think they had been unfairly treated in the past year due to their sex, skin colour, family background, religion, the way they dress, the way they speak, or other reasons, respectively. The discrimination score was recoded into a dichotomous variable (yes/no) (The ALSPAC Study Team, Citation2018). To represent financial difficulties, mothers were asked to rate the difficulty of affording food, clothing, heating, rent, and things they need for the baby, respectively, using a 4-point scale (‘very difficult’ to ‘not difficult’). Sum scores were derived with values ranging from 0 = ‘no financial difficulties’ to 15 = ‘maximum financial difficulties’. Sum score values were coded as missing if any of the 10 component items were missing (The ALSPAC Study Team, Citation2018).
2.2.3.2. Psychological risk factors
The 10-item Edinburgh Postnatal Depression Scale (EPDS) was used to evaluate depressive symptoms in the past week. This measure has been validated and has demonstrated high sensitivity and specificity (Thorpe, Citation1993) and the Cronbach’s alpha for the current sample exceeded 0.80 (Heron, O’Connor, Evans, Golding, & Glover, Citation2004). The response categories for each item ranged from 0 = ‘quite often’ to 3 = ‘never’. Sum scores were derived with values ranging from 0 = ‘not depressed’ to 29 = ‘very depressed’. Sum score values were coded as missing if any of the 10 component items were missing (The ALSPAC Study Team, Citation2018). Prenatal anxiety was measured using the 8-item anxiety subscale (feeling panicky, upset for no obvious reason, strung-up inside, like going to pieces, as though they might faint, uneasy and restless, worrisome, and having upsetting bad dreams) of the validated Crown Crisp Experiential Index (CCEI). Item-level response categories ranged from 0 = ‘never’ to 4 = ‘very often’. A composite measure (ranged from 0 = ‘not anxious’ to 16 = ‘very anxious’) was derived with values coded as missing if at least one of the component items was missing.
2.2.3.3. Environmental risk factors
Three items were used to represent environmental risk factors. At 18 weeks gestation, mothers were asked if their partner smoked (yes/no) and if any other household member smoked (yes/no). Additionally, one item measured passive smoke exposure at 32 weeks gestation (‘how often during the day [are you] in a room or enclosed place where other people are smoking?’) using a 6-point scale with response categories ranging from: ‘all the time’, ‘>5 hrs’, ‘3-5 hrs’, ‘1-2 hrs’, and ‘not at all’ (The ALSPAC Study Team, Citation2018). This variable was recoded into a dichotomous variable (yes/no).
2.2.3.4. Medical risk factors
Five items were used to examine medical risk factors during pregnancy: urinary tract infection (UTI), genital herpes, gestational hypertension, pre-eclampsia, and gestational diabetes. At 32 weeks gestation, mothers were asked if they had UTI (yes/no) or genital herpes (yes/no) during the past three months (The ALSPAC Study Team, Citation2018). Obstetrics records showed: (i) gestational hypertension, defined as having elevated blood pressure (systolic blood pressure >139 mmHg [measured in millimetres of mercury] or diastolic blood pressure > 89 mmHg) on at least two occasions after 20 weeks gestation; (ii) pre-eclampsia (yes/no), defined as being diagnosed with gestational hypertension and concurrent proteinuria; and (iii) gestational diabetes (yes/no), defined as having traces of glycosuria (2 + or more) in the urine on at least two occasions (Lawlor, Macdonald-Wallis, Fraser, & Tilling, Citation2011).
2.2.3.5. Risky health behaviours
Three items were used to investigate risky health behaviours during pregnancy: prenatal smoking, alcohol, and illicit drug use. At 18 weeks gestation, mothers were asked how often during pregnancy they have taken amphetamine, barbiturate, crack, cocaine, heroin, methadone, ecstasy, or other drugs. This item was recoded into a dichotomous variable (The ALSPAC Study Team, Citation2018).
At 32 weeks gestation, mothers were asked how many cigarettes they smoked per day. This item was recoded into four categories (0 = ‘none’, 1 = ‘1-9’, 2 = ‘10-19’, 3 = ‘20+’) (The ALSPAC Study Team, Citation2018). Finally, the total alcoholic units consumed per week were derived by combining the number of half-pints of beer, glasses of wine, drinks of spirits, and other alcohol drinks consumed per week (ranging from 0 to 65) (The ALSPAC Study Team, Citation2018).
2.4. Covariates
The following a priori covariates were included in the analyses: age, educational attainment (ranging from lowest [‘none’] to highest [‘degree’]), ethnicity, and parity (Christiaens, Hegadoren, & Olson, Citation2015; Cowell et al., Citation2021). Highest level of educational attainment ranged from lowest (‘none/CSE’) to highest (‘Degree’). Ethnicity was recoded into a dichotomous variable (1 = ‘White’, 0 = ‘Other ethnicities’), given that 97.3% were White. Finally, parity was recoded into a dichotomous variable (0 = first-time pregnancy). See Additional File 1 (supplementary data) for the item questions and response categories.
3. Analytic strategy
Pairwise Markov Random Field (PMRF) models (Epskamp et al., Citation2018) were estimated to explore the underlying mechanisms of intergenerational transmission of ACEs to PTB and LBW. PMRF models consist of nodes which represent observed variables and edges which represent relations between two nodes after conditioning on all other nodes (Epskamp et al., Citation2018). When represented visually, the thickness of an edge represents the magnitude of the association (strong = thick, weak = thin) while the colour (positive = blue, negative = red) represents the direction of covariance. The analyses were conducted using R (Team RC, Citation2013). Two network models were estimated: the first model (29 nodes) included all variables outlined above, while the second model (22 nodes) included a node which represented the threshold ACEs score instead of individual ACEs. For network estimation, we used the mgm (Haslbeck & Waldorp, Citation2021) method as implemented in the estimateNetwork function in the bootnet package (Epskamp & Fried, Citation2020). Mixed graphical models (MGM) were constructed given that the data contained both dichotomous and continuous variables, and were estimated and selected via regularised LASSO (least absolute shrinkage and selection operator) nodewise regressions (Burger et al., Citation2020; Haslbeck & Waldorp, Citation2021). The LASSO neighbourhood regression limits false positive findings (or spurious edges) by reducing weak edges to zero which leads to a sparse network structure. To further limit spurious edges, a penalty approach was utilised by setting the tuning hyperparameter to , selected using the Extended Bayesian Information Criterion (EBIC), which is typically set between 0 and 0.5 (Foygel & Drton, Citation2010). Setting the hyperparameter to 0.5 provides conservative estimates that err on the side of parsimony (Epskamp & Fried, Citation2018). A drawback of this high specificity is that some edges are potentially not represented in the network.
Edges in MGM models are combined estimates from neighbourhood regression (i.e. estimates from regressing node A on B, and vice versa). As such, the ruleReg argument of the model estimation function was set to ‘OR’ to specify that an edge should be included if at least one estimate is non-zero (Haslbeck & Waldorp, Citation2021). Edge estimates, also called edge weights, give information on the strengths and directions of the relationships between nodes with higher magnitudes representing stronger associations between variables. Edge weight values are reported in the results section and are made available in Additional File 1 (supplementary data). Missing data were imputed via chained equations using the mice package (5 imputations) (van Buuren et al., Citation2015). This method provides unbiased parameter estimates on the assumption of missing at random. Node predictability, or how well a node can be predicted by all remaining nodes, were estimated using the mgm package (Haslbeck & Waldorp, Citation2021) and visualised in the network plot. Node predictability is based on variance explained for continuous variables and classification accuracy for categorical variables. The networks were visualised using the qgraph package (Epskamp et al., Citation2021) which uses the Fruchterman–Reingold algorithm to place nodes that are more strongly connected closer together. To test the accuracy of edge weights, 1,000 bootstraps were performed using the bootnet package (Epskamp & Fried, Citation2020).
The bridge expected influence (1-step and 2-step) in the networktools package were used as bridge centrality metrics to identify nodes that are most vital in communication between communities (Jones & Jones, Citation2021). Much like bridge strength centrality, the bridge expected influence (1-step) quantifies a node’s total connectivity with nodes in other communities. While the former is estimated by summing the absolute value of all edges that lie between node A and all nodes that are not in the same community as node A, the latter does not take the absolute value of edges before summing them (Jones & Jones, Citation2021). This statistic is useful for networks with positive and negative edges and is thought to be of importance for researchers that aim to target influential nodes for clinical treatment (Jones et al., Citation2021; Robinaugh, Millner, & McNally, Citation2016). The 2-step is similar to the 1-step bridge expected influence, but also takes into account the indirect effects that node A has on other communities via other nodes (Jones et al., Citation2021; Jones & Jones, Citation2021). The main difference between extant centrality and bridge centrality metrics is that, in the latter, communities are defined by the researchers via a guiding theory while in the former, they are defined by features internal to the network structure (Jones et al., Citation2021; Robinaugh et al., Citation2016). In this study, communities were defined as the various childhood and prenatal risk factors and infant outcomes. To test the stability of the bridge centrality metrics, 1000 bootstraps were performed using the bootnet package (Epskamp & Fried, Citation2020). To note, previous simulation studies report that the use of reasonably large sample sizes (>1500) consistently showed great robustness of bridge expected influence metrics (Jones et al., Citation2021), and, more broadly, high specificity, high sensitivity, and high correlations of edge weights in the estimated network structure (Constantin, Schuurman, & Vermunt, Citation2021). Finally, the shortest pathways that link ACEs to PTB and LBW were visualised using the qgraph package (Epskamp et al., Citation2021).
4. Results
Sociodemographic characteristics and prevalence of the maternal childhood and prenatal risk factors and adverse infant outcomes are shown in .
4.1. Network analysis
For both models, 8379 participants were included. In general, bootstrapped confidence intervals (CIs) around the edge estimates for the two models were narrow, indicating that the estimates are fairly accurate (see bootstrapped accuracy plots in Additional File 1). Network visualisation is presented in and . To facilitate interpretability, only edges above 0.2 are visualised. Note that edge weights of MGM models represent regression weights based on standardised data, and the scales of edges differ depending on the type of node. For instance, edges between two continuous nodes represent partial correlations and thus cannot be greater than 1, while edges between binary nodes represent logistic regressions and therefore have no upper limit (Burger et al., Citation2020; Liu et al., Citation2021). As such, the magnitudes of the binary and continuous edges cannot be directly compared for strength. Finally, node predictability measures were visualised using node rings, with blue borders indicating the proportion of explained variance for continuous nodes and purple borders indicating the accuracy of intercept model for dichotomous nodes. The red bordering indicates additional accuracy achieved by all remaining variables for binary nodes (Haslbeck & Waldorp, Citation2018).
Figure 1. Network displaying the interrelationships between ACEs, a wide array of prenatal risk factors for preterm birth and low birthweight, and the outcomes of interest. Carnation nodes represent ACEs, purple nodes represent the outcomes, teal nodes indicate biological risks, blue nodes indicate risky behaviours, orange nodes indicate psychosocial risks, green nodes represent environmental risks, and pink nodes represent covariates. Blue edges suggest positive association while red edges (dashed) represent negative associations. Node predictability measures visualised using node rings, with blue rings indicating the proportion of explained variance for continuous nodes while purple rings indicate accuracy of intercept model for dichotomous nodes. The red rings indicate additional accuracy achieved by all remaining variables.
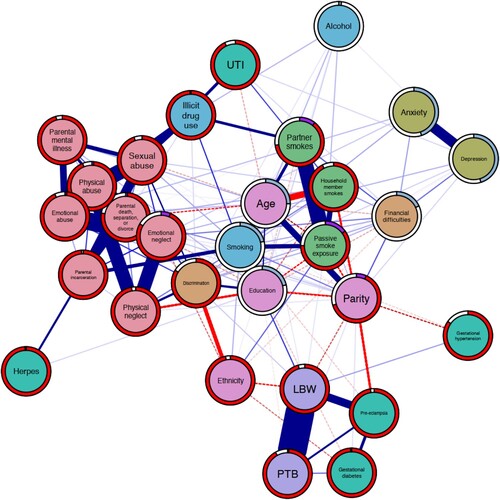
Figure 2. Network displaying the interrelationships between ACEs, a wide array of prenatal risk factors for preterm birth and low birthweight, and the outcomes of interest. Carnation nodes represent ACEs, purple nodes represent the outcomes, teal nodes indicate biological risks, blue nodes indicate risky behaviours, orange nodes indicate psychosocial risks, green nodes represent environmental risks, and pink nodes represent covariates. Blue edges suggest positive association while red edges (dashed) represent negative associations. Node predictability measures visualised using node rings, with blue rings indicating the proportion of explained variance for continuous nodes while purple rings indicate accuracy of intercept model for dichotomous nodes. The red rings indicate additional accuracy achieved by all remaining variables.
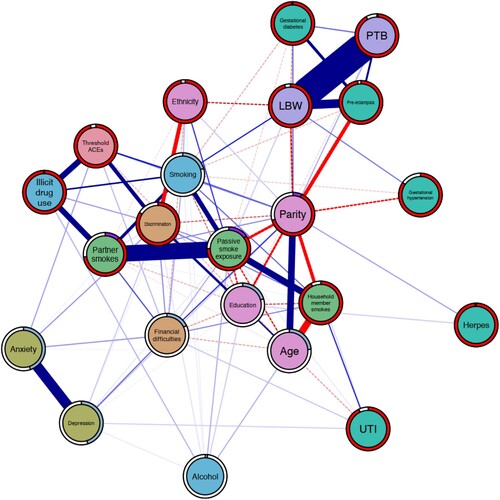
Table 1. Sociodemographic characteristics of the sample (n = 8379).
4.1.1. Model 1: individual ACEs
Model 1 estimated high interconnectivity between forms of ACEs, with each ACE positively associated with at least five other ACEs. The ACEs characterised by household dysfunction, apart from parental mental illness, were positively associated with at least one medical risk, at least two environmental risks, and two risky health behaviour nodes. The neglect node was positively associated with psychosocial, psychological, and risky health behaviour nodes while the abuse nodes were positively associated with at least one psychological, environmental, medical, and risky health behaviour nodes, respectively. Overall, there is high interrelatedness among risk nodes with each one associated with at least one other risk. For instance, depression was positively associated with exposure to discrimination (edge weight [w] = 0.35), financial difficulties (w = 0.12), UTI (w = 0.03), anxiety (w = 0.60), passive smoke exposure (w = 0.01), and alcohol use (w = 0.02). Similarly, both psychosocial risk nodes were positively associated with all the environmental and psychological risks and at least one risky health behaviour node, respectively. The 1-step bridge expected influence identified sexual abuse (z-score [z] = 0.85) as the most influential ACE while the 2-step variant identified physical abuse (z = 2.23). Overall, smoking (z = 1.47) followed by illicit drug use (z = 1.02) were the most influential risk nodes as estimated by the 1-step variant, and vice versa for the 2-step variant (illicit drug use = 3.84; smoking = 3.51). The results of the case-dropping bootstrap indicated that this centrality metric is relatively stable (correlation stability coefficient = .75).Footnote1 See Additional File 2 (supplementary data) for bridge centrality plots and post-hoc stability plots.
In relation to the infant outcomes, physical abuse was positively associated with LBW (w = 0.04) but not PTB. In addition, the LBW node was positively associated with passive smoke exposure (w = 0.02), gestational hypertension (w = 0.08), smoking (w = 0.16), and age (w = 0.02) nodes, and negatively associated with the ethnicity (w = −0.21), and parity (w = −0.14) nodes. Further, the PTB node was positively associated with the gestational diabetes (w = 0.12) and parity nodes (w = 0.02), and negatively associated with the education (w = −0.02) node. Only the pre-eclampsia node was positively associated with both PTB (w = 0.23) and LBW (w = 0.50). Other forms of ACEs were not directly linked to either outcome but conditionally linked through various pathways. Apart from physical neglect and emotional neglect which both estimated discrimination and ethnicity as the shortest pathway to the LBW node, the shortest pathway is through the smoking and education nodes (see Additional file 2 for shortest pathway plots).
4.1.2. Model 2: threshold ACEs
Model 2 demonstrates an approximately similar pattern to the previous model. The global network structure suggests high interconnectivity among nodes, with all nodes connected to at least two other nodes. The threshold ACEs node was not associated with either infant outcome, but the shortest pathway determined was through the smoking node. Further, results showed that PTB was positively associated with gestational diabetes (w = 0.12) and having had previous pregnanc(ies) (w = 0.07), and negatively associated with education (w = 0.03). On the other hand, the LBW node was positively associated with the exposure to second-hand smoke (w = 0.04), gestational hypertension (w = 0.11), and age (0.02) nodes while negatively associated with the ethnicity (w = −0.19), gestational diabetes (w = −0.08), and parity (w = –0.22) nodes. Only pre-eclampsia was positively associated with both PTB (0.23) and LBW (0.53). In both variants of the bridge expected influence, smoking was identified as the most influential bridge node (1-step z-score = 1.11; 2-step = 2.29), followed by threshold ACEs (1-step = 1.09; 2-step = 1.78), and illicit drug use (1-step = 0.88; 2-step = 1.78). Post-hoc stability analysis indicated that this centrality metric is relatively stable (correlation stability coefficient = 0.75).
5. Discussion
Adopting a network approach, we explored the underlying mechanisms that may link maternal adverse childhood experiences to infant preterm birth and low birthweight, while accounting for sociodemographic factors. We found that there were high levels of interrelatedness among childhood and prenatal risk factors in both models. This expands on evidence that found that ACEs tend to co-occur (Gilbert et al., Citation2009; Hindley, Citation2006) and are linked to a wide range of persistent biopsychosocial health risks (Ford & Delker, Citation2018; Hughes et al., Citation2017). Consistent with previous studies, the bridge centrality metrics also indicated that threshold ACEs played a central role in activating directly and indirectly connected risk and adverse outcome nodes (Hein & Monk, Citation2017; Herzog & Schmahl, Citation2018; Hughes et al., Citation2017).
Physical abuse demonstrated a direct relationship with LBW. In addition, the bridge expected influence (2-step) estimated this form of ACEs to be the most influential when accounting for indirect effects. On the other hand, the shortest pathway plots demonstrated that sexual abuse was vital in linking other forms of ACEs to adverse infant outcomes. In addition, the 1-step bridge expected influence estimated it to be the most influential ACEs that activate the other risk nodes. Considered to be one of the more severe forms of ACEs (Lesco, Citation2018), physical abuse and sexual abuse are toxic stressors and their far-reaching consequences on health and development have been well-established (Finkelhor, Citation2020). It has been shown that physically and sexually abused children exhibit elevated C-reactive protein levels (a biomarker of inflammation), possibly as a way for the body to adapt to physical insults (Danese & McEwen, Citation2012). Exposure to prolonged or acute toxic stress during this significant developmental phase may lead to long-term deficits in the immune-inflammatory physiology and may carry over to the gestational biology and cascade to embryonic and foetal development (Moog et al., Citation2016). These forms of ACEs have been associated with an increased likelihood of exposure to other ACEs and have been identified as the most synergistically reactive form of ACEs, meaning that interactions with other adversities in childhood or adulthood substantially increased health consequences (Briggs, Amaya-Jackson, Putnam, & Putnam, Citation2021). Thus, consistent with previous studies, physical and sexual abuse can be considered as optimal targets for intervention because their prevention may increase the likelihood of positively destabilising the entire ACE network and may prevent the occurrence of long-term health consequences (Breuer et al., Citation2020).
We also found that multiple individual ACEs were linked to LBW and PTB through various pathways, and in general, the most common and most influential pathways were via risky health behaviours (i.e. smoking & illicit drug use). Exposure to physical abuse and sexual abuse in childhood are risk factors for higher smoking frequency and initiation of drug use in adolescence and adulthood (Dube et al., Citation2003; Kristman-Valente, Brown, & Herrenkohl, Citation2013). Further, there is corroborative evidence suggesting that prenatal smoking is a mediator between ACEs and PTB and LBW, respectively (Gavin & Morris, Citation2017; Smith et al., Citation2016). Various chains of risk have been posited; for instance, early stressors such as ACEs may negatively impact the development of the neurobiological system (disruption of the dopamine circuit and impairment of stress-regulatory circuits, inter alia) (Hein & Monk, Citation2017). To compensate, ACE-exposed children may turn to substances (such as nicotine) to stimulate dopaminergic neurons or to modulate stress or negative affect. In turn, early smoking initiation may contribute to drug-seeking behaviour, nicotine dependence, and relapse vulnerability that may carry over to pregnancy (Blalock et al., Citation2011; Kane, Harris, & Siega-Riz, Citation2018). Pregnancy is a psychologically taxing process and demands a lot from women’s bodies, it is possible that difficulties with smoking cessation are associated with inadequate social support and limited ways of mitigating stress, which can further be exacerbated when the women’s reference network enacts as a social stressor (Cnattingius, Lindmark, & Meirik, Citation1992; Homish, Eiden, Leonard, & Kozlowski, Citation2012; Woodby, Windsor, Snyder, Kohler, & Diclemente, Citation1999).
Along with PTB and LBW, the 2017 Global Burden of Diseases, Injuries, and Risk Factors Study identified smoking as one of the top risk factors for burdens of disease and mortality among women (Stanaway et al., Citation2018). Relatedly, prenatal smoking has been linked to suboptimal utero-placental oxygen and blood flow and is considered as one of the major risk factors for a wide array of gestational complications, including PTB and LBW (Janbakhisov, Emekçi, Ça, & Altunyurt, Citation2013). Aside from being the most influential risk node, we found that prenatal smoking was associated with LBW. This underscores that prenatal smoking is a candidate target for interventions to disrupt intergenerational risk transmission. Smoking cessation during pregnancy is one of the key action points laid out in the UK Government’s commissioned report on early intervention (Powell, Gheera, Foster, Long, & Kennedy, Citation2021); in order to address this issue, concerted action is necessary. There is some evidence that pharmacological treatments, coupled with psychosocial interventions, help promote smoking cessation during pregnancy; however, its impact on gestational outcomes is still unknown (Claire et al., Citation2020). Behavioural change policies and interventions, which involve addressing negative mental habits or altering the stimuli within the micro-environments to influence health-related behaviours have also been found to be beneficial (Diamanti et al., Citation2019; Vlaev, King, Dolan, & Darzi, Citation2016). For instance, evidence suggests that behavioural counselling can support smoking cessation by helping women develop strategies for managing stress or cravings to smoke (Diamanti et al., Citation2019). Given that socioecological factors like having friends or a partner who smokes are barriers for smoking cessation in pregnancy (Diamanti et al., Citation2019; Homish et al., Citation2012), addressing norms as cues for behavioural change may be beneficial not only for maternal-foetal health but broader public health. Policymakers should consider investing in cessation services that are integrated into antenatal care services, where educational materials to stop smoking are provided to women who smoke and routine carbon monoxide testing are offered along with other routine tests (e.g. urine, blood pressure, growth check) (National Institute for Care and Clinical Excellence, Citation2021). Healthcare services should embed trauma-informed principles to their organisational culture and practices, where the impact of traumatic stress is viewed through a socioecological lens (Abuse, Citation2014), emphasising the safety of users and providers, and the focus lies on enhancing support rather than blame.
Third, consistent with previous evidence (Goldenberg et al., Citation2008; Goldenberg & Culhane, Citation2007; Valero de Bernabé et al., Citation2004), we found that environmental risks (exposure to second-hand smoke) and sociodemographic factors (ethnicity, age, education) were positively associated with either PTB or LBW. Our results further indicated that women who were not White were more often neglected in childhood, exposed to discrimination, and experienced financial difficulties during pregnancy. Further, women who had lower educational attainment were more often exposed to multiple ACEs, had experienced discrimination, financial difficulties, and/or depression were exposed to second-hand smoke, and used tobacco during pregnancy. As Marmot (Citation2020) reports, even after accounting for socioeconomic disadvantage, minority ethnic groups are more likely than White British people to report poor health. Ethnic minority populations, compared to their more advantaged counterparts, are more likely to be subjected to institutional and cultural racism and discrimination; have less access to material resources, education, and health and welfare services, and have poorer living and working conditions (Williams & Mohammed, Citation2013). These are intersecting and intricately linked social determinants that exacerbate health inequities.
Finally, we found that gestational hypertension was linked to LBW while gestational diabetes was associated with PTB. Pre-eclampsia is the severest hypertensive disorder in pregnancy, and women with pre-existing diabetes are at an increased risk (Weissgerber & Mudd, Citation2015). In both models, we found that pre-eclampsia was a risk factor for both PTB and LBW, which is consistent with previous studies (Macdonald-Wallis et al., Citation2015; Valero de Bernabé et al., Citation2004). It is found to decrease utero-placental blood flow and subsequently increase maternal (e.g. cardiovascular and cerebrovascular diseases) and child (e.g. foetal growth restriction, attention-deficit/hyperactivity disorder) health complications (Pittara, Vyrides, Lamnisos, & Giannakou, Citation2021). Antihypertensive pharmacological treatment and routine blood pressure measure at each antenatal appointment are recommended (National Institute for Care and Clinical Excellence, Citation2020; Valero de Bernabé et al., Citation2004).
In order to disrupt the intergenerational transmission of adversity and diminished life opportunities, we need to go beyond individual-level programmes focussed on behaviour change to one focussed on providing immediate support and structural changes that improve their contexts and environments (Metzler, Merrick, Klevens, Ports, & Ford, Citation2017). The World Health Organization’s INSPIRE framework, a technical package aimed at preventing and responding to all forms of violence against children, acknowledges that children’s vulnerability to violence is a result of the interplay of individual, relationship, community, and societal factors and therefore recommends seven synergistic and evidence-based strategies: (i) Implementation and enforcement of laws; (ii) Norms and values; (iii) Safe environments; (iv) Parent and caregiver support; (v) Income and economic strengthening; (vi) Response and support services; and (vii) Education and life skills (World Health Organization, Citation2016). There are emerging research studies from South Africa that provide evidence of the additive effect of INSPIRE-aligned protective factors (e.g. positive parenting, parental monitoring, food sufficiency) in improving children’s health outcomes and adversity exposure across multiple sustainable development goals (SDG) targets (Cluver et al., Citation2020).
6. Strengths and limitations
This paper has a number of strengths. First, our study demonstrates the promise of network analysis as an approach for illuminating the intergenerational transmission of adversity in its full complexity. Identification of influential bridge nodes could aid in preventing comorbidity and inform the development of prevention strategies, yet, cross-sectional networks are not causal models, and as such, no causal claims can be made. Second, we have used conservative estimates to err on the side of parsimony and using various techniques, we have shown that our edge estimates are fairly accurate and stable. In addition, we have used a dataset with a large sample size. Finally, we have followed the Burger and colleagues’ guidelines to ensure that our reporting is systematic and transparent (Burger et al., Citation2020).
However, this study is subject to a number of limitations. First, items obtained from self-completion questionnaires are vulnerable to a variety of biases including social desirability bias, recall bias, demand characteristics, and/or expectancy effects (Holden, Gower, & Chmielewski, Citation2020). Second, the items related to ACEs were not part of a validated measure nor did they measure other important parameters of risk exposure (i.e. severity, duration, chronicity) which would have provided a more accurate representation. Third, the World Health Organization’s coding guidelines for analysis were followed, this includes parcelling the items on parental separation/divorce and parental death (World Health Organization, Citation2014). This could be problematic because as evidenced in the analyses, individual ACEs have unequal weight of effect on later health outcomes and the trauma of losing a parent during childhood may not be the same as the impact of parental divorce or separation. Fourth, data on sensitive topics such as ACEs or prenatal substance use may be underreported due to associated stigma, fear of repercussions, and limited measures to elicit honest responses (Bakhireva, Leeman, Roberts, Rodriguez, & Jacobson, Citation2021). Fifth, although maternal sociodemographic characteristics during pregnancy were included as covariates in all the analyses, (epi)genetics, which may be important confounding factors in many of the relationships explored, were not accounted for.
7. Conclusion
By using network analysis, we were able to map out the web of relationships between ACEs and found that when one ACE is present, other types of ACEs are likely to be present as well. Our network also demonstrated the complex interplay between ACEs, prenatal risk factors, and infant preterm birth and low birthweight. Physical abuse was the only childhood adversity linked directly to low birthweight. Finally, childhood physical and sexual abuse, and risky health behaviours during pregnancy were identified as ‘active’ risk factors capable of affecting (directly and indirectly) other risk factors and contributing to the persistent activation of the global risk network. Thus, they may represent optimal targets for the prevention of intergenerational risk transmission.
Availability of data and materials
The study website contains details of all the data that is available through a fully searchable data dictionary and variable search tool.
Supplemental Material
Download MS Word (552 KB)Supplemental Material
Download MS Word (2.9 MB)Acknowledgements
We are extremely grateful to all the families who took part in this study, the midwives for their help in recruiting them, and the whole ALSPAC team, which includes interviewers, computer and laboratory technicians, clerical workers, research scientists, volunteers, managers, receptionists and nurses. All authors were engaged in the overall conceptualisation, study design, investigation, and findings interpretation. CLH, LGS, and ALM were involved in the analysis. All authors contributed to provide critical review and revision of the manuscript. All authors have reviewed and approved this manuscript. Ethics approval and consent to participate: Ethical approval for the study was obtained from the ALSPAC Ethics and Law Committee and the Local Research Ethics Committees. Informed consent for the use of data collected via questionnaires and clinics was obtained from participants following the recommendations of the ALSPAC Ethics and Law Committee at the time. The study was carried out in accordance with the Declaration of Helsinki regarding the ethical conduct of medical research involving human subjects.
Disclosure statement
No potential conflict of interest was reported by the author(s).
Additional information
Funding
Notes
1 For a metric to be considered stable, it should meet the 0.5 threshold value. For a substantive discussion of this stability test, we refer the reader to the 2018 paper by Epskamp and Fried (Citation2018). Stability testing for the 2-step variant has yet to be implemented because simulation studies indicate a high correlation between the two variants (r = >.9) (Jones, Citation2019).
References
- Abuse, S. (2014). SAMHSA’s concept of trauma and guidance for a trauma-informed approach. HHS Publication No.(SMA) 14–4884. Rockville, MD: Substance Abuse and Mental Health Services Administration.
- The ALSPAC Study Team. (2018). The ALSPAC study: Data collected from the questionnaires. University of Bristol.
- Asmussen, K., Fischer, F., Drayton, E., & McBride, T. (2020). Adverse childhood experiences: What we know, what we don’t know, and what should happen next. Early intervention foundation.
- Bakhireva, L. N., Leeman, L., Roberts, M., Rodriguez, D. E., & Jacobson, S. W. (2021). You didn’t drink during pregnancy, Did You? Alcoholism Clinical and Experimental Research, 45(3), 543–547. doi:10.1111/acer.14545
- Benedict, M. I., Paine, L. L., Paine, L. A., Brandt, D., & Stallings, R. (1999 July). The association of childhood sexual abuse with depressive symptoms during pregnancy, and selected pregnancy outcomes. Child Abuse & Neglect, 23(7), 659–670. doi:10.1016/S0145-2134(99)00040-X
- Blackmore, E. R., Putnam, F. W., Pressman, E. K., Rubinow, D. R., Putnam, K. T., Matthieu, M. M., … O'Connor, T. G. (2016 June). The effects of trauma history and prenatal affective symptoms on obstetric outcomes. Journal of Traumatic Stress, 29(3), 245–252. doi:10.1002/jts.22095
- Blalock, J. A., Nayak, N., Wetter, D. W., Schreindorfer, L., Minnix, J. A., Canul, J., Cinciripini, P. M., et al. (2011). The relationship of childhood trauma to nicotine dependence in pregnant smokers. Psychology of Addictive Behaviors, 25(4), 652–663. doi:10.1037/a0025529
- Boyd, A., Golding, J., Macleod, J., Lawlor, D. A., Fraser, A., Henderson, J., et al. (2013 February). Cohort Profile: the ‘children of the 90s’ – the index offspring of the Avon longitudinal study of parents and children. International Journal of Epidemiology, 42(1), 111–127. doi:10.1093/ije/dys064
- Breuer, F., Greggersen, W., Kahl, K. G., Schweiger, U., & Westermair, A. L. (2020 September). Caught in a web of trauma: Network analysis of childhood adversity and adult mental ill-health. Child Abuse & Neglect, 107, 104534. doi:10.1016/j.chiabu.2020.104534
- Briggs, E. C., Amaya-Jackson, L., Putnam, K. T., & Putnam, F. W. (2021 February). All adverse childhood experiences are not equal: The contribution of synergy to adverse childhood experience scores. The American Psychologist, 76(2), 243–252. doi:10.1037/amp0000768
- Bringmann, L. F., Elmer, T., Epskamp, S., Krause, R. W., Schoch, D., Wichers, M., … Snippe, E. (2019). What do centrality measures measure in psychological networks? Journal of Abnormal Psychology, 128(8), 892–903. doi:10.1037/abn0000446
- Burger, J., Isvoranu, A. M., Lunansky, G., Haslbeck, J. M. B., Epskamp, S., Hoekstra, R. H. A., et al. (2020 November). Reporting standards for psychological network analyses in cross-sectional data [Internet]. PsyArXiv. https://osf.io/4y9nz.
- Buss, C., Entringer, S., Moog, N. K., Toepfer, P., Fair, D. A., Simhan, H. N., … Wadhwa, P. D. (2017 May 1). Intergenerational transmission of maternal childhood maltreatment exposure: Implications for fetal brain development. Journal of the American Academy of Child & Adolescent Psychiatry, 56(5), 373–382. doi:10.1016/j.jaac.2017.03.001
- Christiaens, I., Hegadoren, K., & Olson, D. M. (2015 December). Adverse childhood experiences are associated with spontaneous preterm birth: A case–control study. BMC Medicine, 13(1), 124. doi:10.1186/s12916-015-0353-0
- Claire, R., Chamberlain, C., Davey, M. A., Cooper, S. E., Berlin, I., Leonardi-Bee, J., et al. (2020). Pharmacological interventions for promoting smoking cessation during pregnancy. Cochrane Database Syst Rev [Internet], 3. https://www.readcube.com/articles/10.1002%2F14651858.CD010078.pub3.
- Cluver, L., Shenderovich, Y., Meinck, F., Berezin, M. N., Doubt, J., Ward, C. L., et al. (2020 October). Parenting, mental health and economic pathways to prevention of violence against children in South Africa. Social Science & Medicine (1982), 262, 113194. doi:10.1016/j.socscimed.2020.113194
- Cnattingius, S., Lindmark, G., & Meirik, O. (1992 June 1). Who continues to smoke while pregnant? Journal of Epidemiology & Community Health, 46(3), 218–221. doi:10.1136/jech.46.3.218
- Constantin, M., Schuurman, N. K., & Vermunt, J. (2021). A General Monte Carlo method for sample size analysis in the context of network models [Internet]. PsyArXiv. https://psyarxiv.com/j5v7u/.
- Cowell, W., Taing, L., Askowitz, T., Bosquet Enlow, M., Hacker, M. R., & Wright, R. J. (2021 April). Associations of maternal trait anger expression and lifetime Traumatic and non-traumatic experiences with preterm birth. Maternal and Child Health Journal, 25(4), 635–644. doi:10.1007/s10995-020-03026-x
- Danese, A., & McEwen, B. S. (2012 April). Adverse childhood experiences, allostasis, allostatic load, and age-related disease. Physiology & Behavior, 106(1), 29–39. doi:10.1016/j.physbeh.2011.08.019
- Diamanti, A., Papadakis, S., Schoretsaniti, S., Rovina, N., Vivilaki, V., Gratziou, C., & Katsaounou, P. A. (2019). Smoking cessation in pregnancy: An update for maternity care practitioners. Tobacco Induced Diseases, 17, 57. doi:10.18332/tid/109906
- Dube, S. R., Felitti, V. J., Dong, M., Chapman, D. P., Giles, W. H., & Anda, R. F. (2003 Mar 1). Childhood abuse, neglect, and household dysfunction and the risk of illicit drug use: The adverse childhood experiences study. Pediatrics, 111(3), 564–572. doi:10.1542/peds.111.3.564
- Ellis, G., Iles-Caven, Y., Northstone, K., & Golding, J. (2020 April 8). Traumatic childhood events of parents enrolled in the Avon Longitudinal Study of Parents and Children (ALSPAC). Wellcome Open Res, 5(65). doi:10.12688/wellcomeopenres.15804.1
- Epskamp, S., Borsboom, D., & Fried, E. I. (2018 February). Estimating psychological networks and their accuracy: A tutorial paper. Behavior Research Methods, 50(1), 195–212. doi:10.3758/s13428-017-0862-1
- Epskamp, S., Constantini, G., Haslbeck, J., Isvoranu, A., Waldorp, L. J., & Schmittmann, V. D. (2021). Package ‘qgraph’. [Internet]. https://mran.microsoft.com/snapshot/2017-12-11/web/packages/qgraph/qgraph.pdf.
- Epskamp, S., & Fried, E. I. (2018 December). A tutorial on regularized partial correlation networks. Psychological Methods, 23(4), 617–634. doi:10.1037/met0000167
- Epskamp, S., & Fried, E. I. (2020). Package ‘bootnet’ [Internet]. https://cran.r-project.org/web/packages/bootnet/bootnet.pdf.
- Finkelhor, D. (2020 Oct). Trends in adverse childhood experiences (ACEs) in the United States. Child Abuse & Neglect, 108, 104641. doi:10.1016/j.chiabu.2020.104641
- Ford, J. D., & Delker, B. C. (2018 May 27). Polyvictimization in childhood and its adverse impacts across the lifespan: Introduction to the special issue. Journal of Trauma & Dissociation, 19(3), 275–288. doi:10.1080/15299732.2018.1440479
- Foygel, R., & Drton, M. (2010 November 30). Extended Bayesian Information criteria for Gaussian graphical models. Advances in Neural information Processing Systems, 23, 2020–2028.
- Fraser, A., Macdonald-Wallis, C., Tilling, K., Boyd, A., Golding, J., Davey Smith, G., et al. (2013 February 1). Cohort profile: the Avon Longitudinal Study of Parents and Children: ALSPAC mothers cohort. International Journal of Epidemiology, 42(1), 97–110. doi:10.1093/ije/dys066
- Gavin, A. R., & Morris, J. (2017 May). The association between maternal early life forced sexual intercourse and offspring birth weight: The role of socioeconomic status. Journal of Women's Health / the Official Publication of the Society for the Advancement of Women's Health Research, 26(5), 442–449. doi:10.1089/jwh.2016.5789
- Gavin, A. R., Thompson, E., Rue, T., & Guo, Y. (2012 April). Maternal early life risk factors for offspring birth weight: Findings from the add health study. Prevention Science, 13(2), 162–172. doi:10.1007/s11121-011-0253-2
- Gilbert, R., Widom, C. S., Browne, K., Fergusson, D., Webb, E., & Janson, S. (2009 January). Burden and consequences of child maltreatment in high-income countries. The Lancet, 373(9657), 68–81. doi:10.1016/S0140-6736(08)61706-7
- Goldenberg, R. L., & Culhane, J. F. (2007 February 1). Low birth weight in the United States. American Journal of Clinical Nutrition, 85(2), 584S–590S. doi:10.1093/ajcn/85.2.584S
- Goldenberg, R. L., Culhane, J. F., Iams, J. D., & Romero, R. (2008 January 5). Epidemiology and causes of preterm birth. The Lancet, 371(9606), 75–84. doi:10.1016/S0140-6736(08)60074-4
- Haselgruber, A., Knefel, M., Sölva, K., & Lueger-Schuster, B. (2021 March). Foster children’s complex psychopathology in the context of cumulative childhood trauma: The interplay of ICD-11 complex PTSD, dissociation, depression, and emotion regulation. Journal of Affective Disorders, 282, 372–380. doi:10.1016/j.jad.2020.12.116
- Haslbeck, J. M. B., & Waldorp, L. J. (2018 April). How well do network models predict observations? On the importance of predictability in network models. Behavior Research Methods, 50(2), 853–861. doi:10.3758/s13428-017-0910-x
- Haslbeck, J. M. B., & Waldorp, L. J. (2015). Structure estimation for mixed graphical models in high-dimensional data. Retrieved from: http://arxiv.org/abs/1510.05677.
- Haslbeck, J., & Waldorp, L. (2021). Package “mgm” [Internet]. https://cran.r-project.org/web/packages/mgm/mgm.pdf.
- Hein, T. C., & Monk, C. S. (2017 March). Research review: Neural response to threat in children, adolescents, and adults after child maltreatment – a quantitative meta-analysis. Journal of Child Psychology and Psychiatry, and Allied Disciplines, 58(3), 222–230. doi:10.1111/jcpp.12651
- Heron, J., O’Connor, T. G., Evans, J., Golding, J., & Glover, V. (2004 May). The course of anxiety and depression through pregnancy and the postpartum in a community sample. Journal of Affective Disorders, 80(1), 65–73. doi:10.1016/j.jad.2003.08.004
- Herzog, J. I., & Schmahl, C. (2018 September 4). Adverse childhood experiences and the consequences on neurobiological, psychosocial, and somatic conditions across the lifespan. Frontiers in Psychiatry, 9, 420. doi:10.3389/fpsyt.2018.00420
- Hindley, N. (2006 Sep 1). Risk factors for recurrence of maltreatment: A systematic review. Archives of Disease in Childhood, 91(9), 744–752. doi:10.1136/adc.2005.085639
- Holden, G. W., Gower, T., & Chmielewski, M. (2020). Methodological considerations in ACEs research. In Adverse Childhood Experiences [Internet]. Elsevier. Retrieved from: https://linkinghub.elsevier.com/retrieve/pii/B9780128160657000094.
- Homish, G. G., Eiden, R. D., Leonard, K. E., & Kozlowski, L. T. (2012 January). Social–environmental factors related to prenatal smoking. Addictive Behaviors, 37(1), 73–77. doi:10.1016/j.addbeh.2011.09.001
- Hughes, K., Bellis, M. A., Hardcastle, K. A., Sethi, D., Butchart, A., Mikton, C., et al. (2017 August). The effect of multiple adverse childhood experiences on health: A systematic review and meta-analysis. Lancet Public Health, 2(8), e356–e366. doi:10.1016/S2468-2667(17)30118-4
- Janbakhisov, T., Emekçi, Ö, Ça, E., & Altunyurt, S. (2013). The effect of maternal smoking during pregnancy on Doppler parameters. Perinatal Journal/Perinatoloji Dergisi, 21(3), 5. doi:10.2399/prn.13.0213004
- Jin, J., Zhou, S., Xu, Q., & An, J. (2017 May). Identification of risk factors in epidemiologic study based on ROC curve and network. Scientific Reports, 7(1), 46655. doi:10.1038/srep46655
- Jones, P. (2019). Bootstrapping bridge centrality using the bootnet package. Paytonjjones.wordpress.com. Retrieved 3 June 2022, from: https://paytonjjones.wordpress.com/2019/02/01/bootstrapping-bridge-centrality-using-the-bootnet-package/.
- Jones, P. J., Ma, R., & McNally, R. J. (2021 March 4). Bridge centrality: A network approach to understanding comorbidity. Multivariate Behavior Research, 56(2), 353–367. doi:10.1080/00273171.2019.1614898
- Jones, P., & Jones, M. P. (2021). Package ‘networktools.’ [Internet]. Retrieved from: https://cran.microsoft.com/snapshot/2018-07-04/web/packages/networktools/networktools.pdf.
- Kane, J. B., Harris, K. M., & Siega-Riz, A. M. (2018 June). Intergenerational pathways linking maternal early life adversity to offspring birthweight. Social Science & Medicine (1982), 207, 89–96. doi:10.1016/j.socscimed.2018.04.049
- Khan, A., Uddin, S., & Srinivasan, U. (2019 Dec). Chronic disease prediction using administrative data and graph theory: The case of type 2 diabetes. Expert Systems With Applications, 136, 230–241. doi:10.1016/j.eswa.2019.05.048
- Kheirabad, M. K., Yun, J. Y., Nguyen, T. B., Rami, F. Z., Piao, Y. H., Li, L., et al. 2021. Network analysis of trauma in patients with early-stage psychosis [Internet]. In Review. https://www.researchsquare.com/article/rs-748297/v1.
- Kristman-Valente, A. N., Brown, E. C., & Herrenkohl, T. I. (2013 Oct). Child physical and sexual abuse and Cigarette smoking in adolescence and adulthood. The Journal of Adolescent Health : Official Publication of the Society for Adolescent Medicine, 53(4), 533–538. doi:10.1016/j.jadohealth.2013.06.003
- Lawlor, D., Macdonald-Wallis, C., Fraser, A., & Tilling, K. (2011). Data abstracted from antenatal medical records [Internet]. University of Bristol. Retrieved from: http://www.bris.ac.uk/alspac/researchers/access/.
- Lesco, G. (2018). ACEs and associated health-risk behaviours in university students from the Republic of Moldova. Copenhagen: World Health Organization Regional Office in Europe.
- Liu, D., Epskamp, S., Isvoranu, A. M., Chen, C., Liu, W., & Hong, X. (2021 November). Network analysis of physical and psychiatric symptoms of hospital discharged patients infected with COVID-19. Journal of Affective Disorders, 294, 707–713. doi:10.1016/j.jad.2021.07.043
- Loyal, J. D., & Chen, Y. (2020). Statistical network analysis: A review with applications to the coronavirus disease 2019 pandemic. International Statistical Review = Revue internationale De Statistique, 88(2), 419–440. doi:10.1111/insr.12398
- Macdonald-Wallis, C., Silverwood, R. J., De Stavola, B. L., Inskip, H., Cooper, C., Godfrey, K. M., … Tilling, K. (2015, November 17). Antenatal blood pressure for prediction of pre-eclampsia, preterm birth, and small for gestational age babies: Development and validation in two general population cohorts. Bmj, 351. doi:10.1136/bmj.h5948
- Marmot, M. (2020 February 24). Health equity in England: The Marmot review 10 years on. BMJ, m693. doi:10.1136/bmj.m693
- Metzler, M., Merrick, M. T., Klevens, J., Ports, K. A., & Ford, D. C. (2017 January). Adverse childhood experiences and life opportunities: Shifting the narrative. Children and Youth Services Review, 72, 141–149. doi:10.1016/j.childyouth.2016.10.021
- Moog, N. K., Buss, C., Entringer, S., Shahbaba, B., Gillen, D. L., Hobel, C. J., & Wadhwa, P. D. (2016 May 15). Maternal exposure to childhood trauma is associated during pregnancy with placental-fetal stress physiology. Biological Psychiatry, 79(10), 831–839. doi:10.1016/j.biopsych.2015.08.032
- National Institute for Care and Clinical Excellence. (2020). Hypertension in pregnancy: diagnosis and management [Internet]. https://www.nice.org.uk/guidance/ng133/resources/hypertension-in-pregnancy-diagnosis-and-management-pdf-66141717671365.
- National Institute for Care and Clinical Excellence. (2021). Antenatal care: routine care for healthy pregnant women: NICE clinical guidelines [Internet]. https://www.nice.org.uk/guidance/ng201/resources/antenatal-care-pdf-66143709695941.
- Opsahl, T., Agneessens, F., & Skvoretz, J. (2010). Node centrality in weighted networks: Generalizing degree and shortest paths. Social Networking, 7. doi:10.1016/j.socnet.2010.03.006
- Padula, A. M., Rivera-Núñez, Z., & Barrett, E. S. (2020 June). Combined impacts of prenatal environmental exposures and psychosocial stress on offspring health: Air pollution and metals. Current Environmental Health Reports, 7(2), 89–100. doi:10.1007/s40572-020-00273-6
- Pereira-Morales, A. J., Adan, A., & Forero, D. A. (2019 March 4). Network analysis of multiple risk factors for mental health in young Colombian adults. Journal of Mental Health (Abingdon, England), 28(2), 153–160. doi:10.1080/09638237.2017.1417568
- Pittara, T., Vyrides, A., Lamnisos, D., & Giannakou, K. (2021). Pre-eclampsia and long-term health outcomes for mother and infant: An umbrella review. BJOG: An International Journal of Obstetrics & Gynaecology, 128(9), 1421–1430. doi:10.1111/1471-0528.16683
- Powell, T., Gheera, M., Foster, D., Long, R., & Kennedy, S. (2021). Early intervention: policy and provision [Internet]. House of Commons Library. https://researchbriefings.files.parliament.uk/documents/CBP-7647/CBP-7647.pdf.
- Robinaugh, D. J., Hoekstra, R. H. A., Toner, E. R., & Borsboom, D. (2020 February). The network approach to psychopathology: A review of the literature 2008–2018 and an agenda for future research. Psychological Medicine, 50(3), 353–366. doi:10.1017/S0033291719003404
- Robinaugh, D. J., Millner, A. J., & McNally, R. J. (2016). Identifying highly influential nodes in the complicated grief network. Journal of Abnormal Psychology, 125(6), 747–757. doi:10.1037/abn0000181
- Schouw, J. E. M. C., Verkes, R. J., Schene, A. H., & Schellekens, A. F. A. (2020 January). The relationship between childhood adversity and adult personality revealed by network analysis. Child Abuse & Neglect, 99, 104254. doi:10.1016/j.chiabu.2019.104254
- Smith, M. V., Gotman, N., & Yonkers, K. A. (2016 Apr). Early childhood adversity and pregnancy outcomes. Maternal and Child Health Journal, 20(4), 790–798. doi:10.1007/s10995-015-1909-5
- Speyer, L. G., Hall, H. A., Ushakova, A., Murray, A. L., Luciano, M., & Auyeung, B. (2021). Links between perinatal risk factors and maternal psychological distress: A network analysis. Acta Obstetricia et Gynecologica Scandinavica, 100(5), 917–926. doi:10.1111/aogs.14056
- Stanaway, J. D., Afshin, A., Gakidou, E., Lim, S. S., Abate, D., Abate, K. H., et al. (2018). Global, regional, and national comparative risk assessment of 84 behavioural, environmental and occupational, and metabolic risks or clusters of risks for 195 countries and territories, 1990–2017: A systematic analysis for the Global Burden of Disease Study 2017. The Lancet, 392(10159), 1923–1994. doi:10.1016/S0140-6736(18)32225-6
- Team RC. (2013). R: A language and environment for statistical computing.
- Thorpe, K. (1993 Apr). A study of the use of the Edinburgh postnatal depression scale with parent groups outside the postpartum period. Journal of Reproductive And infant Psychology, 11(2), 119–125. doi:10.1080/02646839308403204
- United Nations Department of Economic and Social Affairs. (2016). Transforming our world: The 2030 agenda for sustainable development.
- Valero de Bernabé, J., Soriano, T., Albaladejo, R., Juarranz, M., Calle, M. E., Martínez, D., et al. (2004 September). Risk factors for low birth weight: A review. European Journal of Obstetrics, Gynecology, and Reproductive Biology, 116(1), 3–15. doi:10.1016/j.ejogrb.2004.03.007
- van Buuren, S., Groothuis-Oudshoorn, K., Robitzsch, A., Vink, G., Doove, L., & Jolani, S. (2015). Package ‘mice.’ Comput Softw.
- Vial, A., van der Put, C., Stams, G. J., Kossakowski, J., & Assink, M. (2020 Sep 1). Exploring the interrelatedness of risk factors for child maltreatment: A network approach. Child Abuse & Neglect, 107, 104622. doi:10.1016/j.chiabu.2020.104622
- Vlaev, I., King, D., Dolan, P., & Darzi, A. (2016). The theory and practice of “nudging”: changing health behaviors. Public Administration Review, 76(4), 550–561. doi:10.1111/puar.12564
- Vogel, J. P., Chawanpaiboon, S., Moller, A. B., Watananirun, K., Bonet, M., & Lumbiganon, P. (2018 October 1). The global epidemiology of preterm birth. Best Practice & Research Clinical Obstetrics & Gynaecology, 52, 3–12. doi:10.1016/j.bpobgyn.2018.04.003
- Weissgerber, T. L., & Mudd, L. M. (2015 March). Preeclampsia and diabetes. Current Diabetes Reports, 15(3), 579. doi:10.1007/s11892-015-0579-4
- Williams, D. R., & Mohammed, S. A. (2013 August 1). Racism and health I: Pathways and scientific evidence. The American Behavioral Scientist, 57(8). doi:10.1177/0002764213487340
- Woodby, L. L., Windsor, R. A., Snyder, S. W., Kohler, C. L., & Diclemente, C. C. (1999). Predictors of smoking cessation during pregnancy. Addiction, 94(2), 283–292. doi:10.1046/j.1360-0443.1999.94228311.x
- World Health Organization. (1977 January 1). Who: Recommended definitions, terminology and format for statistical tables related to the perinatal period and use of a new certificate for cause of perinatal deaths. Acta Obstetricia et Gynecologica Scandinavica, 56(3), 247–253.
- World Health Organization. (2014). Adverse childhood experiences international questionnaire (ACE-IQ) guidance for analysing ACE-IQ. Geneva: World Health Organization.
- World Health Organization. (2016). INSPIRE: Seven strategies for ending violence against children [Internet]. Geneva, Switzerland: World Health Organization. Retrieved from: http://www.who.int/violence_injury_prevention/violence/inspire/en.