ABSTRACT
Controversy exists regarding the processes involved in creative thinking with the Remote Associates Test (RAT) and the Compound Remote Associates Test (CRAT). We report three experiments that aimed to shed light on the component processes underpinning CRAT performance by using the mere presence of task-irrelevant sound as a key theoretical tool. Experiments 1 and 2 revealed that CRAT performance was impaired relative to a quiet condition by the presence of sequences of changing letters and tones, respectively. In both experiments a non-changing sound (a repeated letter or a repeated tone) produced no disruption relative to quiet. Experiment 3 established that additional disruption was engendered by having to ignore meaningful speech as compared to meaningless speech. These experiments demonstrate that both semantic activation and subvocalisation are important determinants of successful creative thinking with CRAT problems. We suggest that semantic activation underpins solution-generation processes whereas subvocalisation underpins solution-evaluation processes.
Research on human thinking in the field of problem solving has produced accounts that specify numerous component cognitive processes that, when engaged, may facilitate or thwart the coming-to-mind of a solution (Goldstein & Levin, Citation1987; Newell & Simon, Citation1972; Weisberg, Citation2015). For example, nonconscious, implicit processes of spreading activation within semantic memory can lead to activation converging on a problem solution (Ohlsson, Citation2011). Attentional control processes (e.g. as measured by working memory capacity; WMC), however, can have opposing effects on problem solving (Wiley & Jarosz, Citation2012). On the one hand a high level of attentional control can facilitate a narrow search through the problem space, but on the other hand too much attentional control can impair problem solving if the solution requires a less constrained, broader search. Narrow searches can result in the production of a dominant response that is incorrect and yield a failure (impasse) and unless attentional control can then be used to inhibit the inappropriate response candidate, accessing the problem solution is hindered (Wiley & Jarosz, Citation2012).
Multi-component accounts of problem solving arise in microcosm in the context of work on creative problem solving. Many of the component processes underpinning creative problem solving can be observed using laboratory-based tasks such as the compound remote associates test (CRAT; Bowden & Jung-Beeman, Citation2003b). The CRAT is a variant of the older remote associates test (RAT; Mednick, Citation1962) and both are amongst the most frequent and influential tasks used to assess creative problem solving in historical studies (e.g. Ansburg, Citation2000; Arden et al., Citation2010; Bowden & Jung-Beeman, Citation2003b; Dallob & Dominowski, Citation1993; Mednick, Citation1962; Schooler & Melcher, Citation1995; Smith & Blankenship, Citation1989) and in contemporary research (e.g. Davelaar, Citation2015; Howe et al., Citation2016; Olteţeanu et al., Citation2018; Salvi et al., Citation2016; Smith et al., Citation2012; Smith et al., Citation2013; Wu & Chen, Citation2017). In the current study we present CRAT problems in the presence of concurrent task-irrelevant sound and use their distraction susceptibility profiles as a function of task-irrelevant sound parameters (i.e. acoustic variability, Beaman & Jones, Citation1997; and meaningfulness, Marsh et al., Citation2009), to glimpse the component processes underlying their solution.
The conceptual antecedent of the CRAT, the RAT, was originally developed to determine creative thought that was independent of any domain-specific knowledge (Mednick, Citation1962). For each RAT problem, participants are presented with three seemingly unrelated cue-words (e.g. same, tennis, head) and requested to produce as a solution a fourth word that meaningfully links or mediates the three cue-words (in this case the fourth word would be “match”). This solution word may be associated with one or more of the cue-words through semantic association (tennis match), synonymy (same = match) or compounding (matchhead). In contrast to the RAT, the CRAT (e.g. Bowden & Jung-Beeman, Citation2003b) involves problems for which the semantic association between the cue-words (e.g. cake, swiss, cottage) and the solution word (i.e. cheese) is restricted to compound words or phrases, with the solution word acting as either a prefix (cheese-cake) or a suffix (swiss-cheese, cottage-cheese) to the cue-words. Forging new associations between unrelated concepts in the way that is tapped by RAT and CRAT problems is often thought to be reflective of the processes underpinning the genesis of original, useful and adaptive ideas to meet specified task-requirements (Martindale, Citation1989).
In the present research we opted to use CRAT problems as they have advantages for research on creative cognition over RAT problems, including the existence of normative data on solution rates and solution times (e.g. Bowden & Jung-Beeman, Citation2003b), which enables the generation of problem sets that vary in difficulty according to established parameters. It was also advantageous in the present research that CRAT problems only require the identification of a solution word that forms compound words or phrases with the cue-words (and not the arguably different processes that underpin associative processing, polysemic processing and compounding). Arguably, then, the use of CRAT problems may, to our advantage, restrict the set of processes under investigation. In addition, CRAT problems can more easily be constructed to yield unambiguous solutions than RAT items (cf. Bowden & Jung-Beeman, Citation2003b). Nevertheless, since RAT problems do involve compounding and are sufficiently alike to CRAT problems in terms of requiring generation and testing of solutions, we discuss the body of work undertaken using RAT problems where relevant for our current investigation.
The component processes involved in solving CRAT and RAT problems
In terms of the component solution processes involved in solving CRAT and RAT problems, phenomenological reports from participants tackling such items (e.g. Salvi et al., Citation2016) suggest that they are often solved through a process of insight, which involves non-conscious, implicit thinking that gives rise to a sudden and clear realisation of a likely solution (i.e. an “Aha!” experience or “Eureka!” Moment; e.g. Bowden & Beeman, Citation1998; Bowden & Jung-Beeman, Citation2003b). These flashes of insight that arise from largely tacit processes may follow a period of impasse (a feeling of being stuck) whereby previous, repeated attempts at discovering a solution have proved unsuccessful (Bowden et al., Citation2005; Bowden & Jung-Beeman, Citation2003a). While it has been debated (e.g. Cranford & Moss, Citation2012) whether an insight response necessarily requires a standard “insight sequence” (i.e. problem presentation → repeated failure → impasse → incubation → restructuring → Aha! + solution; Bowden et al., Citation2005; Ohlsson, Citation1992), the reliable correlation that is observed between CRAT/RAT performance and measures of creativity (Datta, Citation1964; Mednick, Citation1962) and performance on “classic” insight problems (Dallob & Dominowski, Citation1993; Huang et al., Citation2012; Schooler & Melcher, Citation1995), suggests that CRAT and RAT problems share at least some processes and characteristics with other tasks that are used to study creative problem solving.
It should be noted, however, that there is also evidence from participants’ phenomenological reports that CRAT and RAT problems may be solved by a more analytic process (e.g. Salvi et al., Citation2016), which involves conscious, explicit thinking that takes the solver closer to a solution in an incremental, step-by-step manner (see also Ball & Stevens, Citation2009; Fleck & Weisberg, Citation2004). In addition, it is also possible that the solution to CRAT and RAT problems may at times involve a mix of both conscious analysis and non-conscious insight (Barr et al., Citation2015; for theoretical arguments concerning the interplay between implicit and explicit processes in creative problem solving see Barr, Citation2018; Gilhooly et al., Citation2015; Sowden et al., Citation2015; Weisberg, Citation2015, Citation2018).
If we accept the growing evidence that CRAT and RAT problems can be solved either via implicit, insight processes or explicit, analytic processes (and potentially sometimes a combination of both) then this raises deeper questions regarding the specific underlying nature of these key processes. Indeed, evidence implicates the involvement of a wide range of underpinning cognitive mechanisms in the production of solutions to CRAT and RAT problems, including lexical-semantic, associative operations (e.g. Marko et al., Citation2019; Mednick, Citation1962), working memory processes (e.g. Ball & Stevens, Citation2009; Chein et al., Citation2010; Threadgold et al., Citation2019) and executive functions (e.g. Storm et al., Citation2011).
If such a variety of fundamental processes indeed underpin CRAT and RAT performance, then it should be possible to interrogate these processes separately through empirical manipulations. To this end, in the current study we investigate how CRAT performance is affected by the mere presence of task-irrelevant sound comprising different acoustic and semantic properties. Much is known about the nature of focal task processing that is disrupted by different properties of task-irrelevant sound (Beaman & Jones, Citation1997; Jones & Tremblay, Citation2000; Jones et al., Citation2012; Marsh et al., Citation2008, Citation2009). For example, only tasks that are underpinned by subvocal serial rehearsal are susceptible to disruption via the acoustic, changing-state properties of task-irrelevant sound (Beaman & Jones, Citation1997), tasks that require search of semantic memory, such as category fluency, are particularly impaired by the meaning of task-irrelevant sound (e.g. comprehensible against incomprehensible speech; Jones et al., Citation2012), and task-irrelevant sound regardless of its meaningfulness, does not impair executive processes (Jones et al., Citation2012; Morris & Jones, Citation1990). Thus, auditory distraction may be a key theoretical tool offering a window on the component processes underpinning successful CRAT performance.
Associative, semantic processes as a determinant of CRAT and RAT performance
Given that the RAT was modelled on the premise that creative ideas are realised through novel combinations of remotely associated elements, it is not surprising that numerous studies focus on associative, semantic or lexical-semantic processing as a determinant of effective CRAT and RAT performance (e.g. Davelaar, Citation2015; Kenett et al., Citation2018; Kounios & Beeman, Citation2009; Marko et al., Citation2019; Marupaka et al., Citation2012; Mednick, Citation1962; Smith et al., Citation2012). Such studies have revealed that individuals who are better able to solve RAT problems have more interconnected, flexible and robust lexical-semantic and associative networks that facilitate the spread of activation throughout these networks (Kenett et al., Citation2014; Kenett et al., Citation2018). We return to the role of associative and semantic process in yielding CRAT and RAT solutions in Experiment 3.
Retrieval and rehearsal processes as determinants of CRAT and RAT performance
The importance of verbally-mediated working memory processes in CRAT solutions has been reported in a number of studies (Ball & Stevens, Citation2009; Chein & Weisberg, Citation2014). For example, Chein and Weisberg (Citation2014) found that measures of verbal working memory capacity (i.e. operation span) and attentional control (i.e. anti-saccade ability) explained unique variance in overall CRAT solution rates as well as in solution rates for problems that participants self-reported as being solved via insight, but not those that were solved via analysis. Chein and Weisberg also showed that a visuo-spatial working memory capacity measure (symmetry span), although being weakly correlated with overall CRAT solution rates and solution rates for CRAT problems solved via insight, nevertheless failed to explain any unique variance once shared variance with verbal working memory capacity was accounted for (see also Lee et al., Citation2014; Lee & Therriault, Citation2013). This suggests that domain-specific measures of working memory capacity may be exclusively associated with verbally-mediated creative problem-solving performance as measured by CRAT and RAT problems. However, given that working memory capacity tasks likely tap many component processes (e.g. updating, monitoring, shifting and inhibition; Miyake et al., Citation2000), its relationship with CRAT and RAT performance is arguably not very informative.
Of relevance to the current investigation is the fact that working memory span is dependent on covert retrieval and rehearsal. For example, the version of working memory span that is referred to as “operation span” (OSPAN; Turner & Engle, Citation1989) involves interleaving to-be-recalled words or letters with simple to-be-solved mathematical equations (the processing phase). The OSPAN score is based upon the number of words/letters that can be recalled in the correct sequence. It is thought that individuals covertly retrieve and rehearse the to-be-recalled items throughout the task, including during the processing phases (e.g. McCabe, Citation2008). Such covert retrieval and rehearsal might entail refreshing (Barrouillet et al., Citation2004), mental search (Cowan, Citation1992) or subvocal rehearsal (Baddeley, Citation1986). It is possible, therefore, that the efficiency of covert rehearsal may explain the association between verbal working memory capacity and performance with CRAT and RAT problems.
In support of this latter view, numerous studies have shown that preventing subvocalisation can impair performance on tasks involving working memory (Murray, Citation1968), and problem solving (Wallace et al., Citation2017; for reviews, see Alderson-Day & Fernyhough, Citation2015; Winsler et al., Citation2009). Pertinent to the current study is Ball and Stevens’s (Citation2009) finding that concurrent articulatory suppression (repeating the sequence 1 through 6 at one item per second) reduced performance accuracy for easy and difficult CRAT problems relative to a control condition. However, instructing participants to verbalise all thoughts (i.e. to “think aloud”) impaired performance for easy CRAT items but facilitated performance for difficult CRAT items relative to a control condition. This general impairment of CRAT performance by articulatory suppression suggests that solving CRAT problems may benefit from inner-speech production.
Similarly, limiting opportunities for subvocalisation by presenting task-irrelevant sounds also hampers concurrent CRAT performance (Ball et al., Citation2018; Threadgold et al., Citation2019). For example, Threadgold et al. (Citation2019) demonstrated that meaningless irrelevant sound (Spanish vocal music presented to monolingual English-speaking participants; Experiment 1) or non-vocal, instrumental music (Experiment 2) impaired solution accuracy for CRAT problems relative to quiet. Furthermore, sound with little acoustic variation (i.e. library noise) produced no impact relative to quiet, while an upbeat song with lyrics produced disruption relative to quiet and library noise (Experiment 3). Threadgold et al. (Citation2019) concluded that the disruptive impact of task-irrelevant sound on CRAT performance was a consequence of the presence of changing-state irrelevant sound conflicting with subvocal processes required for CRAT problem solving – an interference-by-process (Jones & Tremblay, Citation2000) – yet the authors did not include the more typical device of comparing a repeated single sound (e.g. letter or tone; steady-state) with an acoustically varying sequence of sounds (e.g. different letters or tones; changing-state). Therefore, the necessary checks have not yet been undertaken to support the notion that the disruption to CRAT performance by task-irrelevant sound is attributable to an interference-by-process.
The increased disruption produced by changing-state as compared with steady-state sounds has been coined the “changing-state effect” and is the key empirical referent of the “irrelevant-sound effect” in the context of short-term memory for serial order (Jones et al., Citation1992). Here, the mere presence of a changing sequence of speech (different verbal tokens) or non-speech sounds (sequence of tones of different frequency) impairs serial recall of visual-verbal items (e.g. Jones & Macken, Citation1993). That it is the acoustic properties, rather than the phonological or post-categorical properties of to-be-ignored sound that determines disruption of serial recall is well-known. For example, to-be-ignored narrative that is incomprehensible to participants produces as much disruption as narrative presented in the participant’s mother tongue (Jones et al., Citation1990; Marsh et al., Citation2009, supplementary experiment), indicating that the semanticity of the sound does not contribute additional disruption.
The interference-by-process view holds that the pre-attentive processing of the serial order of changes within sound, as a by-product of the auditory-streaming process (Bregman, Citation1990), clashes with the deliberate serial rehearsal process that supports the ordered retention and recall of the visual memoranda (Jones & Tremblay, Citation2000). Specifically, the irrelevant order cues compete with the subvocal, motoric process ([inner-]speech planning) responsible for maintaining the to-be-remembered items in a sequence. On the interference-by-process account, both the influence of changing-state sound and articulatory suppression on serial recall performance are attributable to their impact on the speech-planning process: articulatory suppression blocks the speech-planning process while changing-state sound impairs its operation via threatening to assume the control of action of the process. Finding a changing-state effect in the context of CRAT problem solving would lend credence to the notion that verbal working memory – and particularly subvocalisation – is involved in CRAT performance (Ball & Stevens, Citation2009; Chein & Weisberg, Citation2014; Threadgold et al., Citation2019).
Support for the proposal that the manifestation of a changing-state effect indicates that subvocalisation is necessary for facilitating performance on a focal task has been gleaned from studies of task sensitivity. Only tasks that call for the seriation of information or for which rote rehearsal provides an efficient strategy are disrupted by the presence of task-irrelevant sound conveying changing-state properties (Baddeley & Salamé, Citation1986; Beaman & Jones, Citation1997, Citation1998; Boyle & Coltheart, Citation1996; Burani et al., Citation1991; Farley et al., Citation2007; Gisselgård et al., Citation2007; Henson et al., Citation2003; Jones & Macken, Citation1993; Richardson, Citation1984; Salamé & Baddeley, Citation1990). Arguably the most convincing demonstration of this has been via the comparison of two tasks that share many characteristics (e.g. items, rate of presentation, list length) but whose contents can be tested in one of two ways. For example, presenting all but one digit from a well-known set of digits in a random order and asking participants to report the missing digit can be completed largely without seriation. This is demonstrated by the fact that performance on the task survives articulatory suppression (Beaman & Jones, Citation1997) and is immune to the changing-state effect (Beaman & Jones, Citation1997; Jones & Macken, Citation1993). However, presenting a post-list probe and requesting the participant to report the item that followed it on the list is dependent on serial recall, as is evident by the susceptibility of the task to disruption via articulatory suppression (Beaman & Jones, Citation1997) and the changing-state effect (Beaman & Jones, Citation1997).
This task-process sensitivity is a key line of evidence against alternative accounts of auditory distraction that suppose the disruption produced by changing-state sound is due to the passive processing of that sound reducing the overall level of resource available for any task that is attentionally demanding (e.g. Bell et al., Citation2012; Bell et al., Citation2019; Cowan, Citation1995; see also Neath, Citation2000).
Experiment 1
The aim of Experiment 1 was to establish more convincingly than hitherto (e.g. Threadgold et al., Citation2019) whether changing-state properties of sound impair CRAT performance. To this end, we contrasted performance in a quiet condition to performance in a steady-state (repeated letter) and changing-state (changing letters) condition. We expected to observe a changing-state effect whereby CRAT performance is lower for problems tackled in a changing-state as compared to a steady-state condition. If the presence of a changing-state effect can be presented as ipso facto evidence that performance of the focal task is underpinned by subvocal-motor processing (Beaman & Jones, Citation1997; Jones & Macken, Citation1993), then we can infer that such processing underpins performance for at least some CRAT problems.
As noted earlier, it is widely held that CRAT problems can be solved either via sudden insight processes or via step-by-step, analytic processes (Bowden et al., Citation2005; Danek et al., Citation2016). Given that the use of subvocalisation, which is susceptible to disruption from changing-state sound, might suggest a step-by-step analytic strategy associated with CRAT problem solving, we also requested that a subset of participants give “feeling-of-insight” ratings for correctly solved problems. Using the prompt detailed by Chein and Weisberg (Citation2014), participants were asked whether they felt their solution to each problem was obtained through a step-by-step analytic process or a sudden coming-to-mind of the solution (i.e. an “Aha!” moment). If it is analytic solutions that are specifically impaired by changing-state sound, then we expected to observe more self-reported insight solutions than analytic solutions in the changing-state condition as compared to the quiet and steady-state conditions.
Method
Participants
Participants were 48 undergraduate students (34 female; Mage = 23.85 years, SDage = 5.32) at the University of Central Lancashire, UK, all reporting normal or corrected-to-normal vision and normal hearing, who took part in the experiment in return for course credit. From the total of 48 participants, 24 were randomly selected and asked to report their solution strategy (16 female; Mage = 25.50 years, SDage = 5.67), with the remaining 24 not being asked to report their solution strategy (18 female; Mage = 22.08 years, SDage = 4.47). All participants were native English speakers. The research protocol was approved by the Psychology and Social Work Ethics Committee of the University of Central Lancashire.
Design and materials
The experiment employed a 3 × 2 within-participants design, with Sound (Quiet vs. Steady-state speech vs. Changing-state speech) and CRAT problem difficulty (Easy vs. Difficult) as the factors.
Irrelevant sound
The irrelevant sound was either steady-state speech or changing-state speech. The steady-state speech comprised a single repeated letter that was sampled from the following set of 10 letters: A B C G J K L M Q S. The changing-state speech comprised a sequence of letters sampled from the same 10 letters. To prepare the irrelevant sound these 10 letters were spoken in an even-pitch female voice and recorded using Audacity Software with a 16-bit resolution at a sampling rate of 48 kHz. Each letter was edited to be 250 ms in spoken duration. Ten 30 s steady-state sequences were generated that consisted of 50 repetitions of the same letter. In addition, 19 changing-state sequences were constructed that consisted of pseudo-random presentations of letters drawn from the 10-letter set. Within these sequences, each of the 10 letters was sampled once before any given letter was sampled again from the set. A further constraint was that no given letter was presented twice in succession within the sequence. Within the changing-state sequences, each letter was sampled five times throughout the 30 s sequence duration. For both the steady-state and changing-state sequences, a 350 ms silent inter-stimulus-interval was presented between letters.
Compound Remote Associate Tasks
For this experiment CRAT problems were selected over RAT problems as for the latter, associative relatedness, synonymy and compounding involve the unavoidable complication of being processed by different cognitive systems (Weiland et al., Citation2014; Wu & Chen, Citation2017; but see Marko et al., Citation2019). Fifty-seven CRAT items were selected from the normative set of problems provided by Bowden and Jung-Beeman (Citation2003b), with all of the chosen problems being solved on average within 30 s. Problems, including solutions, were chosen on the basis of having no word overlap and were screened for their appropriateness in UK English. Consistent with previous studies (Ball & Stevens, Citation2009; Kizilirmak et al., Citation2018), the chosen problems were then divided using a median split based on solution rates, into a set of easy CRAT items (n = 30; mean solution rate: M = .68, SD = .16; mean solution time: M = 8.18 s, SD = 2.6) and a set of difficult CRAT items (n = 27; mean solution rate: M = .27, SD = .13; mean solution times: M = 11.9 s, SD = 2.9).
Using the programme “Match” (Van Casteren & Davis, Citation2007), the set of 57 CRAT problems were divided into three groups of 19 items that were matched for solution rate (Set 1: M = .49, SD = .26; Set 2: M = .48, SD = .27; Set 3: M = .48, SD = .26) and solution time (s) (Set 1: M = 9.98, SD = 3.56; Set 2: M = 10.13, SD = 3.34; Set 3: M = 9.61, SD = 3.34), with each being comprised of 10 easy and 9 difficult items. A one-way ANOVA confirmed no significant differences between normative solution rates (p = .985) and solution times (p = .893) for the three sets of problems. provides the mean solution rates and mean solution times (s) for the “difficult” and “easy” CRAT problems within each of the three matched sets.
Table 1. Mean normative solution rates and solution times (s), with standard deviations in parentheses, for the easy and difficult CRAT problems within each of the three matched sets of problems used in Experiment 1.
Self-reported solution strategies
A subset of 24 participants (randomly allocated to the self-report condition) were asked to report on their solution strategy after providing a response to each CRAT problem. It was emphasised to participants that most problems could be solved either via an analysis strategy or through sudden insight. For each solved item participants were asked to indicate on a four-point scale if their answer was derived completely by analysis, partially by analysis, partially by insight or completely by insight (cf. Chein & Weisberg, Citation2014). Following Chein and Weisberg (Citation2014), on producing their solution participants were to press the appropriately numbered key (1, 2, 3 or 4) to choose one of four solution strategies: Complete Strategy (key 1), Partial Strategy (key 2), Partial Insight (key 3) and Complete Insight (key 4). Participants were reminded that there were no right or wrong answers for the solution strategy and were provided with the brief reminder summary as follows:
1. Complete Strategy: When you thought of the word, at first you did not know whether it was the answer, but after thinking about it strategically (for example, trying to combine the single word with each of the three problem words) you figured out that it was the answer.
2. Partial Strategy: You did not immediately know the word was the answer, but you did not have to think about it much either. For example, after figuring out how the solution went with the first two stimulus words, you realized that it was the solution.
3. Partial Insight: You had a weaker feeling of insight (not as strong as a rating of 4): you felt that the word you thought of might have been the answer, but it was not as obvious as “Of course!” You might have had to check the solution with one of the words to make sure it was correct.
4. Complete Insight: As soon as you thought of the word you knew that it was the answer; the solution word came with a feeling that it was correct (“It popped into my head”; “Of course!”;“I had an Aha!”).
Half of the participants received a scale in which completely analytic solutions were rated as 1 and completely insightful solutions were rated as 4, while the other half of participants received a reverse version of the scale.
Procedure
Each participant provided written informed consent prior to taking part in the experiment. Participants completed the task individually on a laptop computer, with headphones attached. The allocation of CRAT groups was counter-balanced across participants. The task-irrelevant sound conditions were presented pseudo-randomly with the constraint being that no more than two consecutive problems were paired with the same sound condition. For steady-state trials, a sequence entailing repetition of a single letter was chosen pseudo-randomly from the 10 steady-state sequences with the exception that each repeated-letter sequence was sampled across all steady-state trials an approximately equal number of times and was not repeated across consecutive steady-state trials (even if quiet and changing-state trials intervened). For changing-state trials, a random sequence was chosen from the 19 changing-state sequences. Task-irrelevant, to-be-ignored sound was presented over Sennheiser HD-202 headphones at approximately 65–70 dB(A).
Participants were provided with instructions to ignore the background sound and were assured that they would not be asked anything about it later. They were introduced to CRAT problems, and an example CRAT item was presented along with its answer. To ensure familiarity with the task and computer programme, participants completed three additional practice problems, not drawn from the experimental set of 57 CRAT items, before undertaking the experimental problems.
Each participant was permitted up to 30 s to attempt to solve each CRAT problem, and they were asked to press the space bar on identifying the answer to reveal a text field in which to insert their answer before pressing the “enter” key. Participants were then presented with three fixation crosses on the screen for 1 s, with each cross being replaced by one word from the three-word problem. Participants could not go back to the same problem, or revise their answer, once they had entered a response. Cue-words were presented in 30-point Times New Roman font, and for each problem all three cue-words were presented simultaneously along the same horizontal plane.
Participants were allocated randomly to the strategy-report or no-strategy-report conditions. For those providing a solution-strategy rating, on completing each problem the participants were asked to indicate on a four-point scale whether their response was derived more by insight or analysis.
The experiment was executed on a PC running an E-Prime 2.0 programme (Psychology Software Tools, Sharpsburg, PA, USA) that controlled stimulus presentation and recorded participant responses. On completing the experiment, each participant received a written debrief and was thanked for their time.
Results
Supplementary materials and results, including data analysis scripts, can be found here: https://uclandata.uclan.ac.uk/274/.
Solution rates were analysed by means of generalised linear mixed effects (glme) models, using the “glmer” function from the lme4 package (Bates et al., Citation2015) and the binomial family, within the R environment for statistical computing (R Core Team, 2015). CRAT problem difficulty and sound conditions were coded as fixed factors and specified using the function “contr.sdif” from the MASS package (Venables & Ripley, Citation2002). Our general approach was to include a maximal random effects structure (Barr et al., Citation2013), with random intercept and slopes for all the fixed effects for participants, and random intercept and random slope for the sound conditions for items, and specify 10000 iterations with the optimiser “bobyqa” for glme models. If a model failed to converge or there were zero and/or perfectly correlated terms in the random structure (indicating data overfitting), we systematically trimmed the model starting with items and then participants, by first removing correlations and then interactions. If a model still failed to converge and/or overfitted the data, we removed each random slope. The p values were estimated using the lmerTest package (Kuznetsova et al., Citation2017). Fixed effect estimations are summarised in .
Table 2. Generalised linear mixed effects models for all experiments.
Self-reported solution strategies scores on the four-point scale were standardised so that “1” always indicated an analytic solution, and “4” always indicated an insight solution. These strategies were treated as ordinal responses and analysed with cumulative link mixed (clm) modelling in R, using the “clmm” function from the ordinal package (Christensen, Citation2015). Difficulty and sound conditions were specified as fixed factors using the default contr.treatment and using as baseline the Steady-State Sound condition for Experiment 1, and the Meaningless Sound condition for Experiment 3. We used this sound conditions as baseline to allow us to perform theoretically meaningful contrasts and have results easily comparable with the analyses on the solution rates. Similar to the analysis of solution rates, the models initially included a maximal random effects structure (Barr et al., Citation2013), with a random intercept and random slopes (CRAT problem difficulty and sound) per participants and random intercept and slope for sound conditions for items. If a model failed to converge or had correlations in the random structure equal to zero or one, we pruned the random structure by first removing correlations, interactions, and then slopes. The significance of all effects was assessed using the “summary” command, with the default p-values based on the Wald statistic. If any interaction reached significance, post hoc comparisons of estimated marginal means with Tukey adjustment were performed with the emmeans package (Lenth et al., Citation2018) in R. The model results are shown in .
Table 3. Cumulative link mixed effects models for Experiments 1 and 3.
Solution rates
displays the mean solution rates according to each level of Sound and each level of CRAT problem difficulty. As can be seen, the Changing-state speech condition resulted in fewer correct responses in comparison to either the Steady-state speech or Quiet conditions. In addition, solution rates for Easy CRAT problems were higher than for Difficult CRAT problems, but problem difficulty did not appear to moderate the effect of Sound on CRAT performance.
Figure 1. Mean solution rates for Experiment 1 according to Sound condition (Quiet, Steady-state speech and Changing-state speech) and CRAT problem difficulty (Easy vs. Difficult). Error bars represent the within-participant standard errors of the mean.
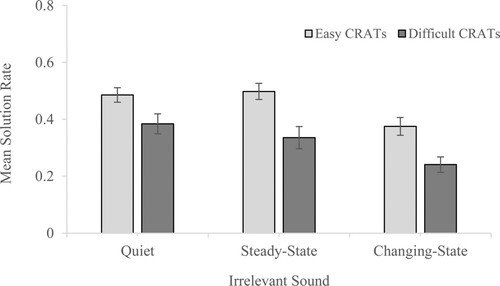
The final generalised linear mixed effects model for Experiment 1 reached convergence with random intercept for each item, and random intercept, slope for CRAT problem difficulty and their correlation for each participant. The analysis revealed a significant main effect of CRAT problem difficulty. As expected, solution rates were significantly higher for the Easy problems (M = .45, SE = .03) in comparison to the Difficult problems (M = .32, SE = .03). In addition, significantly more problems were solved in the Steady-state speech condition (M = .42, SE = .03) in comparison to the Changing-state speech condition (M = .31, SE = .03). There was no significant difference between the number of problems solved in the Quiet (M = .43, SE = .03) and the Steady-state speech conditions (M = .42, SE = .03). There was also no significant CRAT problem difficulty × Sound interaction.
Self-Reported solution strategies
The final model for Experiment 1 included a random intercept for items and a random structure with both fixed effects and their interaction for participants.
The self-reported scores for solution strategy were collated for CRAT problems that had been solved correctly. The analysis indicated that the self-reported solution strategy was not impacted by any of the experimental conditions. There was no significant main effect of CRAT problem difficulty and Sound conditions did not differ from each other. In addition, the CRAT problem difficulty × Sound interaction was not significant.
The percentage of participants self-reporting the four different solution strategies for a correct solution can be seen in . The mean percentage of problems self-reported as solved by Insight (“Aha!”) was greater than the percentage self-reported by Strategy. If it can be assumed that the “Complete Insight” and “Complete Strategy” self-reports can be dichotomised, then participants reported their correct solution being accompanied by an “Aha!” (Complete Insight) response more frequently than not (Complete Strategy). Self-reports of “Aha!” were less frequent than that reported by Danek et al. in the context of classical insight problem solving tasks (Citation2016; 51.9%) and that of Jung-Beeman et al. (Citation2004, p. 56%, Experiment 1) who used the CRAT, but comparable with that reported from the CRAT by Webb et al. (Citation2016, p. 30.66%, Experiment 1). However, caution should be exercised when drawing these comparison due to methodological differences in design, problems adopted and measurement scales. Consistent with previous findings (e.g. Kizilirmak et al., Citation2018) the frequency with which participants reported a Complete Insight, “Aha!” experience, over Complete Strategy was not influenced by CRAT problem difficulty.
Table 4. (a) Percentage of each self-reported strategy for correctly solved problems as a function of Sound condition (Quiet, Steady-state speech and Changing-state speech) and CRAT problem difficulty (Easy vs. Difficult); and (b) Percentage of each self-reported strategy for correctly solved problems as a function of Sound condition (Quiet, Steady-state speech and Changing-state speech) and collapsed across CRAT problem difficulty. Within-participant standard error of the means are presented in parentheses.
Discussion
Experiment 1 compared CRAT performance in a quiet condition to performance in task-irrelevant, steady-state and task-irrelevant, changing-state sound conditions. The key finding was a changing-state effect: significantly more CRAT problems were solved in the steady-state condition relative to the changing-state condition. As expected, a significant difference in solution rates also emerged for easy versus difficult CRAT problems and this pattern was unaffected by the presence of sound. The finding that task-irrelevant, changing-state sound impairs CRAT problem-solving performance regardless of problem difficulty coheres with the finding that articulatory suppression equally impairs easy and difficult CRAT problems (Ball & Stevens, Citation2009; Threadgold et al., Citation2019). Contrary to our hypothesis, however, the presence of task-irrelevant, changing-state sound did not increase self-reported ratings of insight solutions relative to analytic solutions.
The presence of a changing-state effect is typically presented as ipso facto evidence that a task is underpinned by subvocal motor processing (Beaman & Jones, Citation1997). This view has been shaped by its task-specific nature with a host of non-serial tasks (e.g. perceptual [Burani et al., Citation1991], lexical [Stokes & Arnell, Citation2012] and executive [Jones et al., Citation2012; Morris & Jones, Citation1990]) all failing to demonstrate a changing-state effect or disruption from task-irrelevant, changing-state sound. On this assumption, Experiment 1 appears to provide convincing evidence that at least the solving of some CRAT problems is underpinned, or facilitated, by subvocal processing (cf. Ball & Stevens, Citation2009; Threadgold et al., Citation2019).
We also note that the failure to find a specific reduction in self-reported analytic solutions to CRAT problems in the presence of changing-state sound undermines the assumption that analytic strategies (which may involve subvocalisation) are peculiarly disrupted by acoustical variation. However, it should be noted that whether solutions to CRAT problems demonstrate the standard characteristics of insight problem solving has been questioned. For example, using a think-aloud technique, Cranford and Moss (Citation2012) found that participants often report an “Aha!” experience in the absence of other components of the insight sequence, such as impasse. In addition, Weisberg (Citation2015) has suggested routes to insight that are analytically-based, suggesting that self-report measures of insight processing may have debatable diagnosticity.
Experiment 2
Prior to more detailed interpretation of the results of Experiment 1 we sought to address two issues that could potentially undermine our putative account of the data. The first issue is the outside possibility that the effects we observed were driven by the semantic properties of the task-irrelevant speech. The second issue concerned the possibility that the changing-state effect that we observed is attributable to “attentional capture”, rather than interference-by-process.
In Experiment 1, we presented letters as the task-irrelevant sound. One could argue that some letters are associated with semantic concepts (e.g. A with “a”, S with “snake”, T with “tea” and so on). If so, then the impact of changing-state sound that we observed might be attributable to the semantic properties of those sounds, although in our opinion such an explanation is unlikely. Nevertheless, in Experiment 2 we sought to replicate conceptually the findings of Experiment 1, but this time by minimising the potential semantic processing of irrelevant sound by using tones as the auditory material.
Another potential argument is that the changing-state effect observed in Experiment 1 is attributable to the presence of multiple tokens that, according to some accounts (e.g. Bell et al., Citation2019), might engender attentional capture from the focal CRAT problem. While we suggest that this is unlikely due to the insensitivity of non-serial tests such as the missing-item task to disruption via the changing-state effect (Jones & Macken, Citation1993), we nonetheless sought to reduce this possibility by adopting the minimum requirement to satisfy the criterion for changing-state sound: alternating tokens (Jones et al., Citation1992). Two alternating tones 5 semitones apart have been shown to produce an 8% disruption of serial recall performance relative to a single repeated tone (Jones et al., Citation1999) and according to Bell et al. (Citation2019) constitute a changing-state stimulus with the lowest probability of capturing attention.
In sum, Experiment 2 was largely a replication of Experiment 1 but here, participants tackled CRAT problems in the presence of alternating tones (changing-state), repeated tones (steady-state) or quiet. The requirement for participants to self-rate their solution strategies was dropped for Experiment 2, given its failure to distinguish changes in strategy as a function of acoustic variation within the task-irrelevant sound in Experiment 1.
Method
Participants
Thirty-six undergraduate students (18 female; Mage = 23.5 years, SDage = 5.3) at the University of Central Lancashire participated in the experiment in return for course credit. All participants reported normal or corrected-to-normal vision and normal hearing and were native English speakers. The research protocol was approved by the Psychology and Social Work Ethics Committee at the University of Central Lancashire. Participants had not previously taken part in Experiment 1.
Design, materials and procedure
The design was identical to Experiment 1, with the exception that Steady-state tones and Changing-state tones were adopted for the sound conditions. The Steady-state tone took the form of a single, repeated sine-tone, whereas the Changing-state tones comprised two alternating sine-tones with a 5-semitone separation. The to-be-solved CRAT problems were identical to those described in Experiment 1. The procedure for Experiment 2 was identical to that described for Experiment 1, with the exception that participants were not asked to self-report their solution strategy upon solving each problem.
To-be-ignored sounds
Two sine-tones (A4 [440 Hz] and E4 [329.628 Hz]) five semitones apart, were generated with Audacity software with 16-bit resolution at a sampling rate of 48 kHz. The tones were 200 ms in duration with rise and decay times of 20 ms. Sequences were created that were 30 s long wherein tones were separated by 100 ms of silence. Two steady-state sequences of tones were generated. One consisted of repeated presentations of A4 sine-tones and the other consisted of repeated presentations of E4 sine-tones. Two changing-state sequences were constructed for which one sequence started with the A4 sine-tone and alternated thereafter with the E4 sine-tone, and the other started with the E4 sine-tone and alternated thereafter with the A4 sine-tone. Each 30 s sequence comprised 100 tones. Steady-state and changing-state sequences were presented in a pseudo-random order with the constraints that the identical sequences were not presented more than twice in succession, no more than two steady-state or changing-state sequences were presented in succession and each sequence was presented an approximately equal number of times across the testing session.
Results
As in Experiment 1, we analysed solution rates with glme models in R, using the “glmer” function from the lme4 package (Bates et al., Citation2015) and the binomial family, and the self-reported solution strategies with clm models in R with the “clmm” function from the ordinal package (Christensen, Citation2015). The same fixed effects and approach to random structure were used when modelling. The results of the models are summarised in and .
Solution rates
shows the mean solution rates according to each level of Sound and each level of CRAT problem difficulty. The data indicate a reduction in CRAT solution performance for the Changing-state tone condition in comparison to both the Steady-state tone condition and the Quiet condition.
Figure 2. Mean solution rates for Experiment 2 according to Sound condition (Quiet, Steady-state tone and Changing-state tones) and CRAT problem difficulty (Easy vs. Difficult). Error bars represent the within-participant standard errors of the mean.
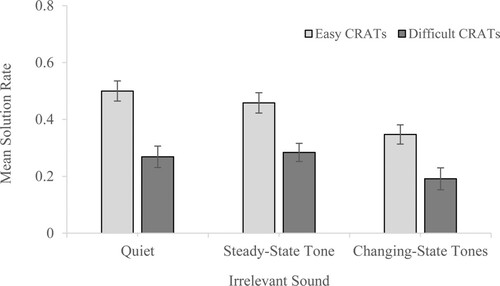
The model that reached convergence included a random intercept for item and a random structure with random intercept, slope for CRAT problem difficulty and their correlation for participants. The analysis revealed a significant main effect of CRAT problem difficulty, with significantly higher solution rates for the Easy CRAT problems (M = .44, SE = .03) in comparison to the Difficult CRAT problems (M = .25, SE = .03). Furthermore, significantly more problems were solved in the Steady-state tone condition (M = .37, SE = .03) in comparison to the Changing-state tone condition (M = .27, SE = .04). There was no significant difference in the solution rate for problems in the Quiet condition and Steady-state tone condition. There was also no significant CRAT problem difficulty × Sound interaction.
Discussion
Experiment 2 compared CRAT performance in a quiet condition to performance in task-irrelevant, steady-state sound and task-irrelevant, changing-state sound conditions using non-speech stimuli. Like Experiment 1, a changing-state effect emerged whereby CRAT performance was poorer in the presence of alternating tones compared to a repeated tone or quiet. Moreover, repeated tones failed to disrupt performance relative to quiet. As in Experiment 1, a significant difference in solution rates was observed for easy versus difficult CRAT problems and akin to Experiment 1, this pattern was not modulated by the presence of sound (cf. Ball & Stevens, Citation2009; Threadgold et al., Citation2019). Given that tones convey no semantic content (nor phonemes) and alternating tones have a low probability of capturing attention on attentional diversion accounts (Bell et al., Citation2019), the changing-state effect observed in Experiment 2 rules out a semantic interpretation of the apparent changing-state effect observed in Experiment 1, while also suggesting that an attentional capture explanation is unlikely.
Experiments 1 and 2 demonstrate that changing-state sound disrupts CRAT performance. Given the susceptibility of vocal motor processing to disruption via the changing-state properties of sound (Jones & Tremblay, Citation2000) a facilitatory role of subvocalisation in CRAT performance in implied. This view is further supported by the finding that preventing subvocalisation impairs CRAT performance (Ball & Stevens, Citation2009). Furthermore, the finding that changing-state irrelevant sound fails to impair executive processing (Jones et al., Citation2012; Morris & Jones, Citation1990) lends further support to the view that CRAT performance is susceptible to disruption due to changing-state sound interfering with subvocal processes.
Prior explanations of the processes involved in solving CRAT and RAT problems have been dominated by accounts wherein solutions are produced via a search through semantic networks (Davelaar, Citation2015; Kenett et al., Citation2014; Kenett et al., Citation2018; Marko et al., Citation2019; Smith et al., Citation2013). At first blush, however, it seems difficult to envisage how task-irrelevant sound without rich semantic properties can disrupt a task underpinned by ostensibly semantic search (Smith et al., Citation2013), given that task-irrelevant sound would not be expected to impact upon the (possibly implicit) semantic activation processes required to solve CRAT problems (Davelaar, Citation2015; Mednick, Citation1962; Smith et al., Citation2013). We note, however, that the solution of CRAT problems is often held to require not just a “generate” process but also a “test” process (see Cranford & Moss, Citation2012; Smith et al., Citation2013). The generate component involves a search within a semantic network that leads to activation converging on solution candidates, sometimes through executive control (Smith et al., Citation2013; but see Marko et al., Citation2019). The testing component, on the other hand, involves combining words within working memory to evaluate whether a viable solution has been discovered (e.g. Threadgold et al., Citation2019). We therefore suggest that changing-state sound impairs the subvocalisation required during the process of testing solutions but has little or no effect on semantic search.
Although the semantic search process underpinning CRAT performance might be inviolable to the acoustic, changing-state properties of a task-irrelevant sound, we concede that it may be vulnerable to impairment via the semantic properties of the task-irrelevant sound. Indeed, a growing body of literature shows that the semantic properties of task-irrelevant sound impact on focal task performance when efficient performance on that task also requires semantic processing (Beaman, Citation2004; Jones et al., Citation1990; Marsh et al., Citation2008, Citation2009; Marsh & Jones, Citation2010; Martin et al., Citation1988; Meng et al., Citation2020; Neely & LeCompte, Citation1999; Sörqvist et al., Citation2012). Such evidence suggests that distraction stems from a conflict between similar, concurrent semantic processing undertaken for both the focal task and for the obligatory processing of task-irrelevant sound: a semantic interference-by-process.
The instance of semantic interference-by-process most relevant to the current study is observed in the context of semantic fluency (Jones et al., Citation2012; Marsh et al., Citation2017), wherein meaningful, but not meaningless, task-irrelevant sound disrupts retrieval of category instances from semantic memory in response to a retrieval cue (e.g. “fruit”). Obvious similarities exist between the processes used in solving CRAT problems and semantic fluency tasks: both are semantic search tasks involving the use of retrieval cues (Davelaar, Citation2015). While the semantic fluency task initially involves the presentation of one cue-word (e.g. “animals”), CRAT problems entail the presentation of three cue-words. However, the generation of an item or items (e.g. cat, lion) in the context of semantic fluency can be used to search with multiple cues (e.g. “animals” and “cat”) and locate subcategories or clusters of items (e.g. pets, zoo animals, feline animals etc.). While the semantic fluency task requires divergent thinking and search (i.e. many responses are required), CRAT problems require divergent thinking and search followed by convergent thought, whereby a single response must be isolated from activated alternatives. Furthermore, unlike the semantic fluency task, wherein all items can be recalled from an accessed semantic cluster, many competing semantic clusters (e.g. in the case of polysemic words) may be accessed in the search for CRAT responses (Davelaar, Citation2015).
There is good evidence for the obligatory semantic processing of task-irrelevant speech (Röer et al., Citation2017; Vachon et al., Citation2020) that has consequences for activation within a semantic network, depending on the type of search process involved. According to spreading activation accounts, processing a word activates a concept node that corresponds to its meaning. In turn, activation spreads to concept nodes representing other semantically related words through learned associations. The result is a localised network of semantic associates. The presentation of a cue therefore leads to activation spreading throughout semantic networks, activating several concept nodes that may vie for retrieval. Activation of concepts within the network due to the obligatory semantic processing of task-irrelevant speech may disrupt the flow of activation of linkages between cue-related items and concepts that facilitate semantic fluency (Jones et al., Citation2012) and CRAT performance. Activation within the semantic memory system is dynamic, changing with each item retrieved. As such, network activation of concepts from the auditory modality may reduce semantic activation, and thus accessibility of, task-relevant words. One consequence of task-irrelevant speech activating the semantic network might be a reduction in the number of potentially relevant words that can be retrieved during processing, which will decrease the likelihood of generating unusual associations or solutions (Amabile et al., Citation2005). Since solving CRAT problems requires such remote associations, CRAT performance should suffer.
Given that both subvocalisation, which is disrupted by changing-state sound (Jones et al., Citation2000), and semantic search, which is disrupted by meaningful, task-irrelevant sound (Jones et al., Citation2012), may influence CRAT problem solving, it should be possible to observe an additive effect of the acoustic and semantic properties of sound on performance. Such an additive effect is not often observed in the literature, although Oswald et al. (Citation2000) reported that participants’ comprehension of sentences was impaired by both meaningful and meaningless task-irrelevant sound compared to quiet, with the meaningful relative to meaningless speech producing more. Thus, disruption to comprehension was primarily due to the sounds’ acoustical variation but the disruptive effect was strengthened by the additional property of the sound’s semanticity. Further evidence for such additivity in the disruptive effect of the acoustic and semantic properties of sound is scarce, with impairments attributable to the acoustic properties of task-irrelevant sound occurring independently of its semantic properties (Jones et al., Citation1990) and likewise with disruption attributable to the meaning of task-irrelevant sound occurring in the absence of impairment via its acoustic properties (e.g. Marsh et al., Citation2008, Citation2009; Meng et al., Citation2020).
Experiment 3
The received view is that CRAT problem solving entails “generate-and-test” processes (Davelaar, Citation2015; Marko et al., Citation2019; Smith et al., Citation2013), as is the case with many cognitive tasks (Forbus et al., Citation1995; Hunt et al., Citation2016; Thomas et al., Citation2008), particularly those involving word problems (Gupta et al., Citation2012). On this account, a local search process leads to the consideration of response candidates. When one candidate is selected it is tested against all the cue-words to verify its validity as a response. This search-test process is repeated until an acceptable answer is found (Smith et al., Citation2013). Moreover, due to the vast number of words that may be linked to at least one of the cue-words, search is probabilistic rather than exhaustive, which would be too time-consuming. There is some disagreement concerning whether search is singularly or multiplicatively constrained. Using a think-aloud technique and the RAT, Smith et al. (Citation2013) reported evidence for the latter. They showed that participants typically sample from response candidates that are activated by a single cue-word at a time and that consecutively produced candidates tend to be associatively related to previously considered responses, suggesting the search process is determined by spreading activation throughout a localised network.
The aim of Experiment 3 was to test the hypothesis that the semantic properties of task-irrelevant sound disrupt CRAT performance. Previous work suggests the semantic properties of task-irrelevant sound are obligatorily processed (Röer et al., Citation2017; Vachon et al., Citation2020) and disrupt semantic search, as revealed by reduced semantic fluency (Jones et al., Citation2012; Marsh et al., Citation2017). Since CRAT performance draws heavily on semantic search (Mednick, Citation1962; Smith et al., Citation2013), we expected the semantic properties of task-irrelevant sound to have a disruptive effect over and above the effect of its changing-state properties (as revealed in Experiments 1 and 2). To investigate this, we contrasted performance in a quiet condition to performance in a meaningless sound condition (spectrally-rotated speech) and a meaningful speech condition.
On the assumption that the acoustic and semantic properties of the task-irrelevant sound affect different component processes underpinning CRAT performance, we expected to observe an additive effect whereby meaningless sound produces disruption compared to quiet, with an additional disruption produced by meaningful sound. Moreover, since insight solutions have often been aligned with convergent semantic activation rather than step-by-step analytic thinking, we also took self-report measures for each correct solution. Our rationale was that self-reported insight solutions should be lower in the meaningful as compared to meaningless sound condition and the quiet condition. This is because the obligatory semantic processing of meaningful sound (and thus the activation of concepts within semantic memory) should disrupt the activation of remote associates to the cue-words, thereby impairing insight solutions.
Method
Participants
Participants were 30 undergraduate students (20 female; Mage = 23.43 years, SDage = 4.38) at the University of Central Lancashire, UK, all reporting normal or corrected-to-normal vision and normal hearing. Participants received course credit for taking part in the experiment. All participants were native English speakers, and none had previously participated in Experiments 1 or 2. The research protocol was approved by the Psychology and Social Work Ethics Committee at the University of Central Lancashire.
Design, materials and procedure
The experiment employed a 3 × 2 within-participants design, with Sound (Quiet vs. Meaningful speech versus Meaningless speech) and CRAT problem difficulty (Easy vs. Difficult) as the factors. The procedure was similar to Experiment 1 with the following exceptions: (1) the CRAT problems were taken from a different source of normed items; and (2) the Sound conditions were different. All participants were asked to self-report their solution strategies using the same four-point scale that was deployed in Experiment 1.
To-be-ignored sound
The auditory stimuli that were used as to-be-ignored sound comprised trivia sentences that were based on (and included) those used in a previous study by Lev-Ari and Keysar (Citation2010). The sentences were short statements such as, “Camels have three eyelids to protect themselves from blowing sand”. The sentences were recorded to at 16-bit resolution at a 22 kHz sampling rate with Sony Sound Forge Pro 10 software (Sony Creative Software). The sentences were spoken in an even-pitched male voice using a Sennheiser ME 65 microphone.
The 20 meaningful sound sequences comprised the individual sentences concatenated within a sound file to a duration of 30 s. Each sequence comprised a different set of sentences. The 20 meaningful sound sequences were inverted around 2 kHz to create spectrally-rotated, meaningless versions (Scott et al., Citation2009). Spectral rotation involves transforming the high-frequency energy in the signal into low-frequency energy, and the low frequency energy into high frequency energy. The acoustic properties of the spectrally-rotated signal are regarded as approximately equivalent to the normal speech signal. For example, pauses between words and sentences, intonation, and variation in sound pressure level across time are fairly equal in spectrally-rotated speech and normal speech. Spectrally-rotated speech also preserves some of the phonetic-cue features of normal speech. In sum, spectrally-rotated and normal speech possess very similar temporal and spectral complexity, with the principal difference between the normal speech signal and the rotated speech signal relating to its meaning: rotated speech is incomprehensible, giving the appearance of an “alien” language.
Compound remote associate tasks
A total of 60 CRAT problems were selected from norms provided by Sandkühler and Bhattacharya (Citation2008) using the same method adopted for Experiments 1 and 2. Based on solution accuracy data, these were divided via a median split into a set of 30 easy CRAT problems (mean solution rate: M = .65, SD = .09; mean solution time: M = 8.7 s, SD = 2.3) and 30 difficult CRAT problems (mean solution rate: M = .34, SD = .07; mean solution time: M = 10.5 s, SD = 2.9) on the basis of Sandkühler and Bhattacharya’s (Citation2008) normative data for solution rates and times for 30 s presentations of problems.
Using the programme “Match” (Van Casteren & Davis, Citation2007), the 60 CRAT problems were divided into three groups of 20 items matched for solution rate and solution time, each comprising 10 easy and 10 difficult CRAT problems. There were no significant differences between normative solution rates (p = .997) and solution times (p = .455) for each of the three sets of CRAT problems. As in Experiments 1 and 2, an additional three CRAT problems were selected for use as practice trials to familiarise participants with the experimental procedure. presents the mean normative solution rates and solution times for the easy and difficult CRAT problems within each of the three matched sets.
Table 5. Mean solution rates and solution times (s), with standard deviations in parentheses, for easy and difficult CRAT problems within each of the three matched sets of problems used in Experiment 3.
Results
Consistent with Experiment 1 and in Experiment 2, we analysed the data in R with glme models (“glmer” function from the lme4 package; Bates et al., Citation2015) for the solution rates, and clm models (“clmm” function from the ordinal package, Christensen, Citation2015) for the self-reported solution strategies. The same Difficulty and Sound conditions were treated as fixed effects, and the same general approach adopted to build and then trim the random structure. As in Experiment 1, post hoc comparisons of estimated marginal means with Tukey adjustment were performed with the emmeans package (Lenth et al., Citation2018) in R on any significant interaction observed in the clm model. The results of the models are shown in and .
Solution rates
shows the pattern of findings for the solution rates in Experiment 3. It is apparent that meaningful speech appeared to be detrimental to CRAT performance relative to the meaningless speech and quiet conditions. In addition, the data seem to indicate that meaningless speech also led to worse CRAT performance than the quiet condition.
Figure 3. Mean solution rates for Experiment 3 according to Sound condition (Quiet, Meaningless speech and Meaningful speech) and CRAT problem difficulty (Easy vs. Difficult). Error bars represent the within-participant standard errors of the mean.
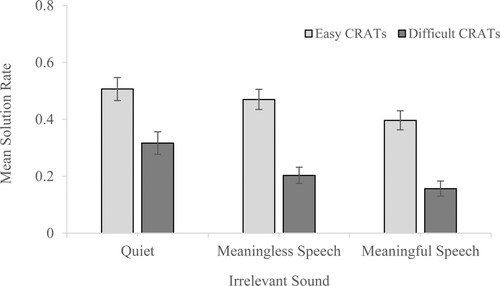
The final glme model that reached convergence included a random intercept for items, and random intercept, random slope for CRAT problem difficulty and their correlation for participants. Consistent with Experiments 1 and 2, there was a significant main effect of CRAT problem difficulty, with significantly higher solution rates for the Easy CRAT problems (M = .46, SE = .04) in comparison to the Difficult CRAT problems (M = .23, SE = .03). In addition, significantly more CRAT problems were solved in the Quiet condition (M = .41, SE = .04) in comparison to the Meaningless speech condition (M = .34, SE = .03). There was also a significant difference between the type of speech, with significantly fewer problems solved in the Meaningful speech condition (M = .28, SE = .03) in comparison to the Meaningless speech condition (M = .34, SE = .03). Finally, the analysis revealed a marginal CRAT problem difficulty × Sound interaction, such that the effect of CRAT problem difficulty was stronger in the Meaningless speech condition compared to the Quiet condition. Given the marginal significance of the interaction, we are cautious to (over-)interpret this finding.
Self-reported solution strategies
The self-reported solution strategy scores were collated for CRAT problems that had been solved correctly. The final model for Experiment 3 included a random intercept for items, and a random structure with both fixed effects and their interaction for participants. Contrary to our prediction, solution strategies did not differ significantly depending on the Sound conditions. However, CRAT problem difficulty did give rise to a significant main effect on self-reported solution strategy scores, with Easy CRAT problems (M = 2.98, SE = .11) resulting in significantly higher insight responses compared to with Difficult CRAT problems (M = 2.81, SE = .17). There was also a reliable interaction between the CRAT problem difficulty and the Meaningless and Meaningful Sound conditions. Pairwise comparisons revealed that for the Easy CRAT problems, Sound did not have any effect on self-reported solution strategy responses, as solution rates did not differ significantly between the Meaningless speech and Quiet conditions (b = 0.027, SE = 0.260, z ratio = 0.104, p = .994), and between the Meaningless and Meaningful speech (b = 0.169, SE = 0.281, z ratio = 0.603, p = .818) conditions. However, for the difficult CRAT problems, Sound did have an effect on self-reported solution strategy responses. The self-reported rating scores significantly differed between the Meaningless and Meaningful speech (b = −1.033, SE = 0.442, z ratio = −2.338, p = .051) conditions, resulting in significantly greater insight-based ratings for the difficult problems solved in the Meaningful speech condition. We did not observe any significant difference in the Difficult CRAT problems between the Meaningless speech and Quiet conditions (b = −0.125, SE = 0.437, z ratio = −0.286, p = .956).
The percentage of each self-reported strategy for correctly solved problems as a function of sound condition and problem difficulty can be seen in . The interesting picture that emerges from these data is the apparent switch to more analytic strategies for the difficult CRAT problems. This was not observed in Experiment 1, nor found previously (Kizilirmak et al., Citation2018). Clearly further investigation is necessary to examine this apparent shift and we speculate that it is caused by a context effect (the presence of more complex speech conditions in Experiment 3 than Experiment 1) or a change to a different set of CRAT problems from Experiments 1 and 2, to Experiment 3.
Table 6. (a) Percentage of each self-reported strategy for correctly solved problems as a function of Sound condition (Quiet, Meaningless speech and Meaningful speech) and CRAT problem difficulty (Easy vs. Difficult); and (b) Percentage of each self-reported strategy for correctly solved problems as a function of Sound condition (Quiet, Meaningless speech and Meaningful speech) and collapsed across CRAT problem difficulty. Within-participant standard error of the means are presented in parentheses.
Discussion
In Experiment 3 CRAT performance in a quiet condition was compared to performance in a meaningless, task-irrelevant sound condition and a meaningful task-irrelevant sound condition. Consistent with Experiments 1 and 2, CRAT performance was poorer in the presence of task-irrelevant sound relative to quiet. However, the key finding of Experiment 3 was that the presence of semanticity within the task-irrelevant sound augments its disruptive effect on CRAT performance. Consistent with Experiments 1 and 2, solution rates were greater for easy versus difficult CRAT problems (cf. Ball & Stevens, Citation2009; Threadgold et al., Citation2019). Moreover, like Experiments 1 and 2, the presence of task-irrelevant sound did not moderate the problem difficulty effect.
The data from Experiment 3 are consistent with the notion that the semantic properties of task-irrelevant sound are disruptive to CRAT performance because they produce a semantic-interference-by-process. The obligatory semantic processing of task-irrelevant sound (e.g. Röer et al., Citation2017; Vachon et al., Citation2020) gives rise to a spread of activation in a semantic network that disrupts the ordinary spread of semantic activation involved in the localised search processes underpinning CRAT performance (Davelaar, Citation2015; Smith et al., Citation2013). On the interference-by-process account, this semantic interference effect is additional to the disruptive impact attributable to the acoustic complexity (or changing-state) properties of the task-irrelevant sound, which is observed by the disruption produced by meaningless sound (see Experiments 1 and 2).
That the additional disruption produced by meaningful, task-irrelevant sound is qualitatively distinct from meaningless, task-irrelevant sound is supported by several empirical studies. For example, meaningless speech (whether reversed or presented in a language foreign to the participant) and meaningful speech are equally disruptive of serial short-term memory (e.g. Jones et al., Citation1990; Marsh et al., Citation2009; Röer et al., Citation2017). This not only rules out the notion that the semantic properties of sound are particularly attentional capturing, but also supports the idea that it is the semantic character of the focal task that determines its susceptibility to disruption via the semantic properties of the task-irrelevant sound (Marsh & Jones, Citation2010; see also Meng et al., Citation2020). That meaningful task-irrelevant sound did not influence self-reported solution ratings in any predicted way might suggest that the experience of insight is not diagnostic of the processes that underpin such insight in problem solving. However, an alternative account of the invulnerability of self-reported solution strategy to the presence of meaningful task-irrelevant sound is that subvocalisation underpins both analytic and insight-based solution strategies in different ways, subvocalisation facilitating solution evaluation processes but not generation processes. If this is the case, then other, non-subvocalisation processes, could still underpin analytic as compared to insight solution strategies.
General discussion
In the three experiments reported here we investigated the impact of the acoustic properties (Experiments 1 and 2) and the semantic properties (Experiment 3) of task-irrelevant sound on CRAT problem-solving performance. Experiments 1 and 2 demonstrated a changing-state effect whereby the presence of changing as compared to repeated letters (Experiment 1) and tones (Experiment 2) significantly impaired CRAT performance regardless of the difficulty of the problems. In Experiment 3, meaningful, task-irrelevant sound produced greater impairment of CRAT performance than meaningless task-irrelevant sound, which in turn reduced CRAT performance as compared with quiet. Again, CRAT difficulty did not modulate this disruption.
Across Experiments 1 and 2, the presence of changing-state sound did not affect self-reported solution strategies (i.e. insight vs. analysis). Experiment 3 gave rise to an interaction between meaningful sound and problem difficulty in relation to self-reported solution strategies, with only the difficult problems showing differences in self-reported strategies across sound conditions in that more insight-based ratings arose in the meaningful speech condition compared to the other conditions. This finding was not in line with our predictions and is challenging to explain, but it may serve as an interesting avenue for future research.
We argue that our findings relating to CRAT performance in the face of task-irrelevant sound are consistent with a generate-and-test account of CRAT problem solving (Cranford & Moss, Citation2012; Smith et al., Citation2013), whereby candidate responses are generated via a local search of semantic memory networks and are then tested against cue-words for their veracity as solutions. We suggest that meaningful, task-irrelevant sound produces activation within the semantic network, thereby disrupting the spread of activation and localised search for the solution word. Further, we argue that this semantic interference effect is qualitatively distinct from the disruptive impact that meaningless, task-irrelevant sound confers to CRAT problem solving. Inferring from the results of Experiments 1 and 2, we propose that the disruption produced by meaningless sound is a changing-state effect. This auditory distraction effect is purportedly the joint product of pre-attentive processes applied involuntarily to the sound and to processes underpinning the focal task (Jones & Tremblay, Citation2000). The cues derived from changes from one sound element to the next specify the order of those sounds and this order information specifically interferes with the goal-driven processes of keeping to-be-remembered items in sequence via the deliberate process of serial rehearsal (interference-by-process). While it is unlikely that serial rehearsal underpins CRAT performance, at least some verbal maintenance or subvocal planning may be necessary once a response candidate has been recovered (from the generation process) to test it against each cue-word. We assume that this testing process is susceptible to disruption via the changing acoustic properties of task-irrelevant sound (i.e. the changing-state effect).
We consider that the interference-by-process account is a better explanation of the disruption produced by changing-state task-irrelevant sound on CRAT problem solving than an attentional view (e.g. Bell et al., Citation2012; Bell et al., Citation2019; Cowan, Citation1995; see also Neath, Citation2000) for a number of reasons. According to the attentional view the consumption of capacity by task-irrelevant sound may surpass the total available capacity for the focal task at hand. On this view it would be assumed that changing-state sound, regardless of whether it conveys semantic properties, should produce distraction on any focal task, regardless of the nature of the processing (semantic or non-semantic) involved in that task. However, this prediction is not borne out by the available data. For example, as a class of tasks that make substantial demands on attention, executive tasks, should be susceptible to the disruptive effects of changing-state sound. However, several lines of evidence suggest that the mere presence of to-be-ignored sound does not appear to disrupt executive processing. Morris and Jones (Citation1990) reported that the presence of articulatory suppression and meaningless irrelevant speech impaired serial memory for the items regardless of the number of updates required. Thus, rehearsal, not the executive process of updating, was impaired by irrelevant speech disrupting speech-planning.
A further result at odds with the attentional account is that changing-state (meaningless) irrelevant speech fails to impair semantic fluency (i.e. the retrieval of category-exemplars to a semantic-category name such as “fruit”) or phonemic fluency (i.e. retrieval of words beginning with a cued first letter; Jones et al., Citation2012). Given the extensive involvement of executive processing in both of these fluency tasks (i.e. inhibition, updating and monitoring of working memory content, self-initiation, switching between clusters; Baldo et al., Citation2006; Rosen & Engle, Citation1997; Troyer et al., Citation1998), these latter findings suggests that changing-state sounds do not impair such executive processing (at least those involved in fluency and memory updating; Jones et al., Citation2012; Morris & Jones, Citation1990). If this conclusion is viable, then the disruption it produces to CRAT performance in Experiments 1 and 2 cannot be attributable to disruption of the putative functions of executive processing within the CRAT (Smith et al., Citation2013; Storm et al., Citation2011; but see Marko et al., Citation2019) which include, for example, use of the executive function of inhibition to remediate repeated solution failures and fixation when a candidate word (typically a prepotent response) coheres with one or even two of the three cue-words (cross, rain, tie), but not all three (e.g. crosswalk, raincoat; Storm, Citation2011; Storm et al., Citation2011; Storm & Angello, Citation2010; Storm & Koppel, Citation2012). Thus, we assert that the locus of the disruption produced by task-irrelevant sound is not at a level whereby inappropriate responses require inhibition to enable generation of novel, insightful, solutions (Gilhooly et al., Citation2007; Schooler & Melcher, Citation1995), but rather due to subvocal planning required in testing potential solutions.
In the next sections we first consider the possibility that subvocal planning, which is vulnerable to changing-state disruption, is necessary for solution testing with CRAT problems. The considerations that then follow deal with the potential susceptibility of semantic search to disruption via the automatic semantic processing of meaningful, task-irrelevant sound.
What role is played by working memory in solution testing?
If it is assumed that solution testing for CRAT problems entails working memory, then it is important to consider the nature of these processes. Unlike typical working memory tasks, wherein participants must actively maintain in mind a set of items, the cue-words for CRAT problems are always present, which makes it unlikely that a simple capacity constraint determines the disruption of CRAT performance in the presence of task-irrelevant sound (Jones & Tremblay, Citation2000). One possibility is that working memory is required to maintain semantic representations or multiple meanings of a concept that are active within the language system (e.g. in the case of polysemic words). For example, participants must remember and maintain the meaning of previously produced words to search locally within the same semantic space in memory. There is growing evidence that phonological and semantic representations contribute separately to working memory task performance (Haarmann et al., Citation2003; Nishiyama, Citation2013; Shivde & Thompson-Schill, Citation2004). Furthermore, semantic representations appear to be actively maintained independently from phonological representations (Nishiyama, Citation2013, Citation2018). Earlier we noted that the relationship between verbal working memory capacity measures (e.g. OSPAN) and CRAT performance (Chein & Weisberg, Citation2014) might be attributable to covert retrieval and rehearsal of items during the task (McCabe, Citation2008). Covert retrieval and rehearsal may be undertaken by refreshing (Barrouillet et al., Citation2004), mental search (Cowan, Citation1992) or subvocal rehearsal (Baddeley, Citation1986). Therefore, the relationship between working memory capacity and CRAT performance could be related to one or more forms of covert retrieval/rehearsal. However, some theoretical clarity on this issue can be gleaned from the relative sparing of attentional refreshing (which arguably supports semantic activations) under empirical conditions that eliminate or diminish opportunities for subvocal rehearsal. For example, Nishiyama (Citation2018) reports a study in which the secondary task of tapping (which demands attentional resources involved in refreshing) impaired synonym recognition performance while articulatory suppression had no effect. Thus, the activation of the semantic representations of words via long-term memory networks – via attentional refreshing – can be maintained in the presence of articulatory suppression (Nishiyama, Citation2018).
That refreshing can occur independently of the availability of subvocal processing might explain the pervasive influence that semantic representations continue to have on short-term memory performance under conditions of concurrent articulation (Besner & Davelaar, Citation1982; Haarmann & Usher, Citation2001; Saint-Aubin & Poirier, Citation1999), while so-called phonological effects are typically abolished (Baddeley et al., Citation1975; Murray, Citation1968). While it is possible that attentional refreshing may be involved in CRAT performance, it is unlikely that it is disrupted by task-irrelevant sound. This is because only attentionally demanding secondary tasks purportedly disrupt attentional refreshing (Nishiyama, Citation2018). Furthermore, the finding that articulatory suppression, which does not disrupt attentional refreshing, impairs CRAT performance (Ball & Stevens, Citation2009) implies that reducing the opportunity for speech-based planning (as is assumed to occur with both articulatory suppression and task-irrelevant sound), is unlikely to disrupt the semantic representations involved in CRAT performance. This suggests that some mechanism other than subvocal rehearsal supports the maintenance of semantic representations within the cognitive system.
Threadgold et al. (Citation2019) have previously argued that subvocalisation may be required to rehearse potential solutions during CRAT problem solving before converging on a solution word. For example, participants may generate a response candidate and use inner speech to test the viability of that word against the cue-words. In the case of the CRAT problem “cadet, deep, ship”, participants may rehearse “space-cadet” and “deep-space” while testing the validity of the generated word as a solution against “ship”. Subvocalisation might be particularly useful in the case of problems for which the solution word varies as a prefix or suffix for the cue-words (e.g. “space-cadet” [prefix], “deep-space” [suffix]), but is perhaps less useful for RAT problems, where the solution word has different conceptual relationships to the cue-words (associative relatedness, synonymy and compounding).
It is clear that subvocalisation underpins a number of tasks other than serial recall (Beaman, Citation2018; Miyake et al., Citation2004; Smith et al., Citation1995; for a review, see Alderson-Day & Fernyhough, Citation2015). For example, Smith et al. (Citation1995) report that subvocalisation plays a role in parsing meaningful letter strings (“NRG” = Energy) as revealed by susceptibility of the task to disruption via the mere presence of task-irrelevant sound or the demand for engagement in articulatory suppression. The notion here is that subvocalisation is required so that a judgment can be based on the auditory imagery it yields. Perhaps it is possible that processing multiple compound words for their veracity also requires auditory imagery? An alternative possibility is that task-irrelevant sound may impair subvocalisations important in set shifting (Miyake et al., Citation2004) and task switching (Emerson & Miyake, Citation2003) that may underpin CRAT performance. However, caution should be exercised when entertaining this possibility since putative executive functions appear to be resistant to disruption via task-irrelevant sound (Jones et al., Citation2012) and there is opposition to the view that CRAT performance requires such functions (e.g. Marko et al., Citation2019).
Whilst we have considered changing-state irrelevant sound to impact specifically on the subvocalisation required for the test process, rather than impairing any semantic processing involved in the task per se, it is possible that semantic representations could also affect active word maintenance. For example, articulatory rehearsal of words can give rise to their lexical-representations that can in turn feedback to facilitate their articulatory rehearsal. Thus, further work is required to establish whether the impairment to CRAT performance by task-irrelevant sound is specific to the subvocalisation process in the absence of semantic processing, or whether semantic processing is also disrupted due to the impairment of subvocal rehearsal via task-irrelevant sound. Assuming the disruption of CRAT performance by task-irrelevant sound taps a generation process (Smith et al., Citation2013; cf. Jones et al., Citation2012), it is of import to consider further the nature of semantic networks and processes therein.
Semantic networks and their robustness or susceptibility to (semantic) distraction
The classic view of semantic search as a generative process is that a printed (or heard) word activates a concept node corresponding to its meaning, with activation then spreading to concept nodes that are semantically related. The problem space for CRAT items is the localised network of semantic associates activated by the cue-words, one of which should be the solution word owing to convergent activation from the cue-words. As the solution word is a weak associate of the cue-words, its activation level may be low and thus a goal for the problem solver is to increase activation of the solution word relative to other words within the search space (Davelaar, Citation2015). The presence of task-irrelevant sound that also activates the concepts of words within the semantic network (Röer et al., Citation2017; Vachon et al., Citation2020) will reduce the likelihood of discovering words associated with the cue-words by driving down their activation (see also Jones et al., Citation2012). This would be particularly the case for weak associates that receive less activation than strong (prepotent) associates of one or more cue-words.
One reason why the disruptive impact of meaningful, task-irrelevant sound observed in Experiment 3 was modest may be due to the considerable variability between individuals in terms of their semantic networks (Kenett et al., Citation2018; Mednick, Citation1962). For example, Mednick (Citation1962) proposed that individual differences in the capability to solve RAT problems relates to an associative hierarchy that organises creative ideas. There is considerable variation between individuals in these lexical-semantic and associative structures. Individuals with steeper associative hierarchies connect more closely-related and fewer distally-related concepts. This may result in the production of a small number of frequent or stereotypical ideas or responses. Since the RAT requires processing uncommon, infrequent lexical-semantic associates that are distally-related (remote associates), these individuals are poorer at RAT problems than those individuals with a flatter organisation of semantic memory structure who can combine a wide range of weakly-activated concepts to generate novelty. Therefore, the flatter the organisation of semantic memory, the greater the links between concepts, including those that are remotely associated. Thus, individuals with flatter organisations of semantic memory can generate a large variety of associated responses to cue-words, including remote and uncommon associates. This is particularly useful in the context of RAT problems, wherein solutions entail greater remote association to cue-words.
Similar conclusions have been reached by other researchers (Kenett et al., Citation2014; Marko et al., Citation2019; Rossmann & Fink, Citation2010). For example, using semantic-network modelling, Kenett et al. (Citation2018) showed that the semantic network of highly creative participants is more spread out and cleaves into more partitions than that for low creative participants whose networks are more rigid. It follows that disruption of search within a network, as produced by meaningful, task-irrelevant sound might be a function of each participant’s network. For example, activation within semantic memory for individuals with steep associative hierarchies (for which remote associations are more difficult to activate) might be more susceptible to disruption via the presence of meaningful, task-irrelevant sound.
Can the think-aloud technique help delineate the CRAT processes that are affected by task-irrelevant sound?
We suggest that the deployment of the think-aloud technique (e.g. Ball & Stevens, Citation2009; Chein et al., Citation2010; Fleck & Weisberg, Citation2004; Gilhooly et al., Citation2010; Schooler et al., Citation1993; Schooler & Melcher, Citation1995) is likely to afford a deeper understanding of the roles of generate-and-test processes in solving CRAT problems as well as the susceptibility of these processes to disruption via the acoustic or semantic properties of task-irrelevant sound. Necessarily, articulatory suppression requires the use of the vocal-motor production system, thereby preventing the overt report of candidate items. However, changing-state, task-irrelevant sound, whose locus of disruption is arguably also via the vocal-motor production system (Jones et al., Citation2004), does not prevent the vocal production of candidate responses (Ball et al., Citation2015). Therefore, assuming that the think-aloud requirement changes neither the nature of the generate process (and thus the response candidates considered) nor the following testing process (e.g. Ericsson & Simon, Citation1993; Smith et al., Citation2013; Weisberg, Citation2015; but see Schooler et al., Citation1993), then any differential disruption to these processes produced by the acoustic/semantic properties of task-irrelevant sound could be observed. Further, Cranford and Moss (Citation2012) established a protocol for identifying the various components of insight, including impasse, restructuring and the “Aha!” experience that, if adopted, might further characterise the impact of task-irrelevant sound on the component processes underpinning CRAT performance.
Similar to the insights into CRAT problem solving offered by the think-aloud technique, other tasks involving the production of lexical entries from semantic memory may likewise permit inspection of alterations in the search process due to the presence of meaningful, task-irrelevant sound. For example, Gupta et al. (Citation2012) requested that participants produce a single “best guess” for RAT problems under time pressure. Participants who produced responses of low associative frequency, even if incorrect, had better RAT performance than those who tended to produce high associative frequency (incorrect) responses. If obligatory processing of task-irrelevant semantic information impacts on strong and weak linkages differentially, as one might predict, then it would be expected that guesses should be higher in associative frequency in the presence of task-irrelevant meaningful speech. This is because concurrent activation due to the obligatory processing of semantic activation should cause the activation of weakly-related items to drop off sooner (Sörqvist et al., 2010).
One complication for this latter proposal is that the difficulty of RAT problems, regardless of their psycholinguistic heterogeneity, is intricately related to “remoteness”, as defined by the associative and syntagmatic distance between the cue-words and the respective solutions. Indeed, Marko et al. (Citation2019) report that 80% of the variance in item difficulty is attributable to associative and syntagmatic distance. The upshot of this, is that the difficulty of RAT items stems from demands on the lexical-semantic search for remote solution candidates. If meaningful, task-irrelevant sound impairs activation of particularly uncommon and infrequent lexical-semantic associates, then it might be predicted that the disruptive impact of meaningful, as compared with meaningless, task-irrelevant sound would be more pronounced for the more difficult CRAT problems. However, contra to this pattern, the additional disruption from meaningful as compared with meaningless sound was not more pronounced for easy as compared with difficult CRAT items. On the face of it, this finding conflicts with the prediction that meaningful task-irrelevant sound impairs semantic search, particularly for weak associates. However, it should be noted that the difficulty measures used in our study (drawn from Bowden & Jung-Beeman, Citation2003b) may not relate specifically to the semantic remoteness of a solution and could, therefore, be influenced by other factors such as the length of the solution or the size of the problem space in terms of the total number of compound words that can be generated from the cue-words (Bowden et al., Citation2005).
Insight versus analytic processing: dichotomy and criterial issues
Although self-report ratings for CRAT problems demonstrated that they were mostly solved via insight, whether the occurrence of insight is criterial for classifying a response as creative, as suggested by some researchers (Ohlsson, Citation2011; Perkins, Citation2000; Wiley & Jarosz, Citation2012) has been debated (Weisberg, Citation2015). Moreover, multiple paths may give rise to insight (Cranford & Moss, Citation2012; Fleck & Weisberg, Citation2004, Citation2013), suggesting that the proposed sequence to an insight solution (problem presentation → repeated failure → impasse → incubation → restructuring → Aha! + solution) also cannot be criterial or definitive for a creative solution.
The latter observation rather undermines views that propose that special processes underpin insight problem solving (e.g. Schooler et al., Citation1993) and instead supports a “business-as-usual” view (cf. Ball & Stevens, Citation2009), according to which the cognitive processes underpinning insight and non-insight problem solving are essentially the same, despite subjective experiences accompanying insight differing from those accompanying analytic processes. On the business-as-usual view, there exists a continuum of analytic-to-insight problem solving rather than a dichotomy of analytic versus insight processes (but see Gilhooly & Murphy, Citation2005). If the business-as-usual view is accepted, then the failure to observe an impact of meaningful versus meaningless task-irrelevant sound on self-reported problem-solving strategies is unsurprising. This is because the processes arguably affected by the acoustic and by the semantic properties of background sound will underpin solutions that are self-reportedly solved through both non-insight and insight methods.
The cognitive control paradox in the domain of creative cognition
In this paper we have argued in favour of an interference-by-process account of the disruption to CRAT performance by task-irrelevant sound. We assert that the impairment produced by changing-state sound is attributable to the processing of that sound directly undermining the subvocal processing necessary for testing candidate solutions. Further, we ascribe the additional effect of meaningfulness to the semantic processing of irrelevant information impairing the semantic search underpinning the generation of candidate solutions. The former process appears to be indomitable: the magnitude of the changing-state effect is immune to manipulations of cognitive control (Hughes et al., Citation2013). However, it is not obvious whether semantic distraction effects can be overridden by the same cognitive-control manipulations (cf. Marsh et al., Citation2012; Vachon et al., Citation2020). The interference-by-process account eschews the notion that task-irrelevant sound disrupts performance because it captures attention from any attentionally-demanding task (Bell et al., Citation2012, Citation2019; Cowan, Citation1995). This account has been supported by the failure of task-irrelevant sound to impair executive processing (Jones et al., Citation2012; Morris & Jones, Citation1990) and by the task-specific nature of distraction (Jones & Macken, Citation1993).
If one persists in arguing that task-irrelevant sound indeed affects controlled attention, then it is not immediately obvious whether insight problem solving should benefit or suffer. Several studies suggest that controlled attention and executive functions (e.g. mental set-shifting, updating, monitoring and inhibition) facilitate creative problem solving (e.g. Gilhooly & Fioratou, Citation2009; Gilhooly et al., Citation2007; Nijstad et al., Citation2010; Nusbaum & Silvia, Citation2011; Benedek et al., Citation2012; De Dreu et al., Citation2012; Edl et al., Citation2014; Lee & Therriault, 2012; Silvia et al., Citation2013). However, this might be contingent upon whether a solution is obtained via insight or via analytic problem solving (assuming there is a distinction; Fleck, Citation2008; Weisberg, Citation2015), with insight processing being less reliant on controlled attention and executive functions.
Overall, the available data support the latter suggestion (Ash & Wiley, Citation2006; Fleck, Citation2008; Gilhooly & Fioratou, Citation2009; Gilhooly & Murphy, Citation2005; Lavric et al., Citation2000). For example, Wiley and Jarosz (Citation2012) suggest strong executive functions may even impair insight problem solving due to an increased focus on obvious solution possibilities limiting opportunities for restructuring. Further, Jarosz et al. (Citation2012) suggest that a more diffuse attentional state arising from mild alcohol intoxication facilitates CRAT performance (see also Ansburg & Hill, Citation2003; Finke et al., Citation1992; Martindale, Citation1995). However, it is unclear how Jarosz et al.’s manipulation makes attention diffuse in this study and no independent measure of “diffuse attention” was provided beyond CRAT performance.
If the view is sustained that insight problem solving is facilitated by reduced controlled attention and executive processing and that the presence of task-irrelevant sound usurps resources for such processing, then it would be expected that insight, but not analytic, problem solving should benefit. This view would imply that CRAT performance should either increase in the presence of task-irrelevant sound or that task-irrelevant sound should increase the likelihood of participants solving the problems through insightful processing. This pattern of results, however, was not observed in the current study.
Does the absence of such findings instead provide support for the notion that task-irrelevant sound is more likely to disrupt the goal-directed processes involved in creative problem solving that are tied to planning and mental manipulation (e.g. controlled effortful thinking) rather than the processes that come about through diffuse activation that may be underpinned by uncontrolled, spontaneous, associative thinking (Beaty et al., Citation2014; Benedek et al., Citation2012; Faust & Lavidor, Citation2003; Gruska & Necka, Citation2002; Merten & Fischer, Citation1999; Rossmann & Fink, Citation2010), assuming both are involved in creativity (Gabora, Citation2010; Mok, Citation2014)? To sustain this position, it would have to be assumed that the spontaneous and automatic activation of semantic associations does not interact with controlled processes (e.g. specifying combinations and constraints) to determine CRAT performance (cf. Chermahini et al., Citation2012; Jones & Estes, Citation2015; Lee & Therriault, Citation2013).
Conclusions
In conclusion, the findings reported here support the view that CRAT problem solving is based on a generate-and-test process. In addition to the semantic search process, that appears to be disrupted by the presence of meaningful, task-irrelevant sound, our findings also point to a role for subvocalisation in the testing of potential CRAT solutions, which is a process that is impaired by simple acoustic variation within the task-irrelevant sound. That these two mechanisms are indeed qualitatively distinct requires further investigation, as does their relationship to insight problem solving more generally. We nevertheless believe that our reported studies clearly demonstrate the value of studying the effects of distraction in problem solving as a way to shed new light on the old issue of understanding the component processes that underpin creative cognition.
Disclosure statement
No potential conflict of interest was reported by the author(s).
Additional information
Funding
References
- Alderson-Day, B., & Fernyhough, C. (2015). Inner speech: Development, cognitive functions, phenomenology, and neurobiology. Psychological Bulletin, 141(5), 931–965. https://doi.org/https://doi.org/10.1037/bul0000021
- Amabile, T. M., Barsade, S. G., Mueller, J. S., & Staw, B. M. (2005). Affect and creativity at work. Administrative Science Quarterly, 50(3), 367–403. https://doi.org/https://doi.org/10.2189/asqu.2005.50.3.367
- Ansburg, P. I. (2000). Individual differences in problem solving via insight. Current Psychology: A Journal for Diverse Perspectives on Diverse Psychological Issues, 19, 143–146. https://doi.org/https://doi.org/10.1007/s12144-000-1011-y
- Ansburg, P. I., & Hill, K. (2003). Creative and analytic thinkers differ in their use of attentional resources. Personality & Individual Differences, 34(7), 1141–1152. https://doi.org/https://doi.org/10.1016/S0191-8869(02)00104-6
- Arden, R., Chavez, R. S., Grazioplene, R., & Jung, R. E. (2010). Neuroimaging creativity: A psychometric review. Behavioural Brain Research, 214(2), 143–156. https://doi.org/https://doi.org/10.1016/j.bbr.2010.05.015
- Ash, I. K., & Wiley, J. (2006). The nature of restructuring insight: An individual differences approach. Psychonomic Bulletin & Review, 13(1), 66–73. https://doi.org/https://doi.org/10.3758/BF03193814
- Baddeley, A. (1986). Working memory. Oxford University Press.
- Baddeley, A., & Salamé, P. (1986). The unattended speech effect: Perception or memory? Journal of Experimental Psychology: Learning, Memory, & Cognition, 12(4), 525–529. https://doi.org/https://doi.org/10.1037/0278-7393.12.4.525
- Baddeley, A., Thomson, N., & Buchanan, M. (1975). Word length and the structure of short-term memory. Journal of Verbal Learning & Verbal Behavior, 14(6), 575–589. https://doi.org/https://doi.org/10.1016/S0022-5371(75)80045-4
- Baldo, J. V., Schwartz, S., Wilkins, D., & Dronkers, N. F. (2006). Role of front versus temporal cortex in verbal fluency as revealed by voxel-based lesion symptom mapping. Journal of the International Neuropsychological Society, 12((06|6)), 896–900. https://doi.org/https://doi.org/10.1017/S1355617706061078
- Ball, L. J., Marsh, J. E. M., Litchfield, D., Cook, R., & Booth, N. (2015). When distraction helps: Evidence that concurrent articulation and irrelevant speech can facilitate insight problem solving. Thinking & Reasoning, 21(1), 76–96. https://doi.org/https://doi.org/10.1080/13546783.2014.934399
- Ball, L. J., & Stevens, A. (2009). Evidence for a verbally-based analytic component to insight problem solving. In N. Taatgen, & H. Rijn (Eds.), Proceedings of the 31st Annual Conference of the Cognitive Science Society (pp. 1060–1065). Cognitive Science Society.
- Ball, L. J., Threadgold, E., Solowiej, A., & Marsh, J. E. (2018). Can intrinsic and extrinsic metacognitive cues shield against distraction in problem solving. Journal of Cognition, 1(1), 1–12. https://doi.org/10.5334/joc.9
- Barr, N. (2018). Intuition, reason, and creativity: An integrative dual-process perspective. In G. Pennycook (Ed.), The new reflectionism in cognitive psychology: Why reason matters (pp. 99–124). Routledge.
- Barr, D. J., Levy, R., Scheepers, C., & Tily, H. J. (2013). Random effects structure for confirmatory hypothesis testing: Keep it maximal. Journal of Memory & Language, 68(3), 255–278. https://doi.org/https://doi.org/10.1016/j.jml.2012.11.001
- Barr, N., Pennycook, G., Stolz, J. A., & Fugelsang, J. A. (2015). Reasoned connections: A dual-process perspective on creative thought. Thinking & Reasoning, 21(1), 61–75. https://doi.org/https://doi.org/10.1080/13546783.2014.895915
- Barrouillet, P., Bernardin, S., & Camos, V. (2004). Time constraints and resource sharing in adults’ working memory spans. Journal of Experimental Psychology: General, 133(1), 83–100. https://doi.org/https://doi.org/10.1037/0096-3445.133.1.83
- Bates, D., Mächler, M., Bolker, B., & Walker, S. (2015). Fitting linear mixed-effects models using lme4. Journal of Statistical Software, 67(1), 1–48. https://doi.org/https://doi.org/10.18637/jss.v067.i01
- Beaman, C. P. (2004). The irrelevant sound phenomenon revisited: What role for working memory capacity? Journal of Experimental Psychology: Learning, Memory, & Cognition, 30(5), 1106–1118. https://doi.org/https://doi.org/10.1037/0278-7393.30.5.1106
- Beaman, C. P. (2018). The literary and recent scientific history of the earworm: A review and theoretical framework. Auditory Perception & Cognition, 1(1-2), 42–65. https://doi.org/https://doi.org/10.1080/25742442.2018.1533735
- Beaman, C. P., & Jones, D. M. (1997). The role of serial order in the irrelevant speech effect: Tests of the changing-state hypothesis. Journal of Experimental Psychology: Learning, Memory, & Cognition, 23(2), 459–471. https://doi.org/https://doi.org/10.1037/0278-7393.23.2.459
- Beaman, C. P., & Jones, D. M. (1998). Irrelevant sound disrupts order information in free recall as in serial recall. Quarterly Journal of Experimental Psychology, 51A(3), 615–636. https://doi.org/https://doi.org/10.1080/713755774
- Beaty, R. E., Benedek, M., Wilkins, R. W., Jauk, E., Fink, A., Silvia, P. J., Hodges, D. A., Koschutnig, K., & Neubauer, A. C. (2014). Creativity and the default network: A functional connectivity analysis of the creative brain at rest. Neuropsychologia, 64, 92–98. https://doi.org/https://doi.org/10.1016/j.neuropsychologia.2014.09.019
- Bell, R., Röer, J. P., Dentale, S., & Buchner, A. (2012). Habituation of the irrelevant sound effect: Evidence for an attentional theory of short-term memory disruption. Journal of Experimental Psychology: Learning, Memory, & Cognition, 38(6), 1542–1557. https://doi.org/https://doi.org/10.1037/a0028459
- Bell, R., Röer, J. P., Lang, A.-G., & Buchner, A. (2019). Distraction by steady-state sounds: Evidence for a graded attentional model of auditory distraction. Journal of Experimental Psychology: Human Perception & Performance, 45(4), 500–512. https://doi.org/https://doi.org/10.1037/xhp0000623
- Benedek, M., Franz, F., Heene, M., & Neubauer, A. C. (2012). Differential effects of cognitive inhibition and intelligence on creativity. Personality & Individual Differences, 53(4), 480–485. https://doi.org/https://doi.org/10.1016/j.paid.2012.04.014
- Besner, D., & Davelaar, E. (1982). Basic processes in reading: Two phonological codes. Canadian Psychological Association, 36(4), 701–711. https://doi.org/https://doi.org/10.1037/h0080665
- Bowden, E. M., & Beeman, M. J. (1998). Getting the right idea: Semantic activation in the right hemisphere may help solve insight problems. Psychological Science, 9(6), 435–440. https://doi.org/https://doi.org/10.1111/1467-9280.00082
- Bowden, E. M., & Jung-Beeman, M. (2003a). Aha! insight experience correlates with solution activation in the right hemisphere. Psychonomic Bulletin & Review, 10(3), 730–737. https://doi.org/https://doi.org/10.3758/BF03196539
- Bowden, E. M., & Jung-Beeman, M. (2003b). Normative data for 144 compound remote associate problems. Behavior Research Methods, Instruments, & Computers, 35(4), 634–639. https://doi.org/https://doi.org/10.3758/BF03195543
- Bowden, E. M., Jung-Beeman, M., Fleck, J., & Kounios, J. (2005). New approaches to demystifying insight. Trends in Cognitive Science, 9(7), 322–328. https://doi.org/https://doi.org/10.1016/j.tics.2005.05.012
- Boyle, R., & Coltheart, V. (1996). Effects of irrelevant sounds on phonological coding in reading comprehension and short term memory. Quarterly Journal of Experimental Psychology, 49A(2), 398–416. https://doi.org/https://doi.org/10.1080/713755630
- Bregman, A. S. (1990). Auditory scene analysis: The perceptual organization of sound. MIT Press.
- Burani, C., Vallar, G., & Bottini, G. (1991). Articulatory coding and phonoligcal judgements of words and pictures: The role of the phonolgical output buffter. European Journal of Cognitive Psychology, 3(4), 379–398. https://doi.org/https://doi.org/10.1080/09541449108406235
- Chein, J. M., & Weisberg, R. W. (2014). Working memory and insight in verbal problems: Analysis of compound remote associates. Memory & Cognition, 42(1), 67–83. https://doi.org/https://doi.org/10.3758/s13421-013-0343-4
- Chein, J. M., Weisberg, R. W., Streeter, N. L., & Kwok, S. (2010). Working memory and insight in the nine-dot problem. Memory & Cognition, 38(7), 883–892. https://doi.org/https://doi.org/10.3758/MC.38.7.883
- Chermahini, S. A., Hickendorff, M., & Hommel, B. (2012). Development and validity of a Dutch version of the remote associates task: An item-response theory approach. Thinking Skills & Creativity, 7(3), 177–186. https://doi.org/https://doi.org/10.1016/j.tsc.2012.02.003
- Christensen, R. H. B. (2015). Ordinal - regression models for ordinal data. R Package Version, 2015, 6–28. http://www.cran.r-project.org/package=ordinal/
- Cowan, N. (1992). Verbal memory span and the timing of spoken recall. Journal of Memory & Language, 31(5), 668–684. https://doi.org/https://doi.org/10.1016/0749-596X(92)90034-U
- Cowan, N. (1995). Working memory capacity. Psychology Press.
- Cranford, E. A., & Moss, J. (2012). Is insight always the same? A protocol analysis of insight compound remote associate problems. The Journal of Problem Solving, 4(2), 128–153. https://doi.org/https://doi.org/10.7771/1932-6246.1129
- Dallob, P. I., & Dominowski, R. L. (1993, April). Erroneous solutions to verbal insight problems: Effects of highlighting critical material [Paper presentation] 73rd Annual Meeting of the Western Psychological Association, Portland, OR.
- Danek, A. H., Wiley, J., & Öllinger, M. (2016). Solving classical insight problems without Aha! experience: 9 dot, 8 coin, and matchstick arithmetic problems. The Journal of Problem Solving, 9(1), 47–57. https://doi.org/https://doi.org/10.7771/1932-6246.1183
- Datta, L. (1964). A note on the RAT, US culture and creativity. Journal of Applied Psychology, 48(3), 184–185. https://doi.org/https://doi.org/10.1037/h0048900
- Davelaar, E. J. (2015). Semantic search in the remote associates test. Topics in Cognitive Sciences, 7(3), 494–512. https://doi.org/https://doi.org/10.1111/tops.12146
- De Dreu, C. K., Nijstad, B. A., Baas, M., Wolsink, I., & Roskes, M. (2012). Working memory benefits creative insight, musical improvisation, and original ideation through maintained task-focused attention. Personality & Social Psychology Bulletin, 38(5), 656–669. https://doi.org/https://doi.org/10.1177/0146167211435795
- Edl, S., Benedek, M., Papousek, I., Weiss, E. M., & Fink, A. (2014). Creativity and the stroop interference effect. Personality & Individual Differences, 69, 38–42. https://doi.org/https://doi.org/10.1016/j.paid.2014.05.009
- Emerson, M. J., & Miyake, A. (2003). The role of inner speech in task switching: A dual-task investigation. Journal of Memory & Language, 48(1), 148–168. https://doi.org/https://doi.org/10.1016/S0749-596X(02)00511-9
- Ericsson, K. A., & Simon, H. A. (1993). Protocol analysis Verbal report as data. MIT Press.
- Farley, L. A., Neath, I., Allbritton, D. W., & Surprenant, A. M. (2007). Irrelevant speech effects and sequence learning. Memory & Cognition, 35(1), 156–165. https://doi.org/https://doi.org/10.3758/BF03195951
- Faust, M., & Lavidor, M. (2003). Semantically convergent and semantically divergent priming in the cerebral hemispheres: Lexical decision and semantic judgment. Cognitive Brain Research, 17(3), 585–597. https://doi.org/https://doi.org/10.1016/S0926-6410(03)00172-1
- Finke, R. A., Ward, T. B., & Smith, S. M. (1992). Creative cognition: Theory, research and applications. MIT Press.
- Fleck, J. I. (2008). Working memory demands in insight versus analytic problem solving. European Journal of Cognitive Psychology, 20(1), 139–176. https://doi.org/https://doi.org/10.1080/09541440601016954
- Fleck, J. I., & Weisberg, R. W. (2004). The use of verbal protocols as data: An analysis of insight in the candle problem. Memory & Cognition, 32(6), 990–1006. https://doi.org/https://doi.org/10.3758/BF03196876
- Fleck, J. I., & Weisberg, R. W. (2013). Insight versus analysis. Evidence for diverse methods in problem solving. Journal of Cognitive Psychology, 25(4), 436–463. https://doi.org/https://doi.org/10.1080/20445911.2013.779248
- Forbus, K. D., Gentner, D., & Law, K. (1995). MAC/FAC: A model of similarity-based retrieval. Cognitive Science, 19(2), 141–205. https://doi.org/https://doi.org/10.1207/s15516709cog1902_1
- Gabora, L. (2010). Revenge of the “neurds”: Characterizing creative thought in terms of the structure and dynamics of memory. Creativity Research Journal, 22(1), 1–13. https://doi.org/https://doi.org/10.1080/10400410903579494
- Gilhooly, K. J., Ball, L. J., & Macchi, L. (2015). Insight and creative thinking processes: Routine and special. Thinking & Reasoning, 21(1), 1–4. https://doi.org/https://doi.org/10.1080/13546783.2014.966758
- Gilhooly, K. J., & Fioratou, E. (2009). Executive functions in insight vs. non-insight problem solving: An individual differences approach. Thinking & Reasoning, 15(4), 355–376. https://doi.org/https://doi.org/10.1080/13546780903178615
- Gilhooly, K. J., Fioratou, E., Anthony, S. H., & Wynn, V. (2007). Divergent thinking: Strategies and executive involvement in generating novel uses for familiar objects. British Journal of Psychology, 98(4), 611–625. https://doi.org/https://doi.org/10.1111/j.2044-8295.2007.tb00467.x
- Gilhooly, K. J., Fioratou, E., & Henretty, N. (2010). Verbalization and problem solving: Insight and spatial factors. British Journal of Psychology, 101(1), 81–93. https://doi.org/https://doi.org/10.1348/000712609X422656
- Gilhooly, K. J., & Murphy, P. (2005). Differentiating insight from non-insight problems. Thinking & Reasoning, 11(3), 279–302. https://doi.org/https://doi.org/10.1080/13546780442000187
- Gisselgård, J., Uddén, J., Ingvar, M., & Petersson, K. M. (2007). Disruption of order information by irrelevant items: A serial recognition paradigm. Acta Psychologica, 124(3), 356–369. https://doi.org/https://doi.org/10.1016/j.actpsy.2006.04.002
- Goldstein, F. C., & Levin, H. S. (1987). Disorders of reasoning and problem-solving ability. In M. J. Meier, A. Benton, & L. Diller (Eds.), Neuropsychological rehabilitation (pp. 327–354). Guildford Press.
- Gruska, A., & Necka, E. (2002). Priming and acceptance of close and remote associations by creative and less creative people. Creativity Research Journal, 14, 174–192. https://doi.org/https://doi.org/10.1207/S15326934CRJ1402_6
- Gupta, N., Jang, Y., Mednick, S. C., & Huber, E. (2012). The road not taken: Creative solutions require avoidance of high-frequency responses. Psychological Science, 23(3), 288–294. https://doi.org/https://doi.org/10.1177/0956797611429710
- Haarmann, H., Davelaar, E. J., & Usher, M. (2003). Individual differences in semantic short-term memory capacity and reading comprehension. Journal of Memory & Language, 48(2), 320–345. https://doi.org/https://doi.org/10.1016/S0749-596X(02)00506-5
- Haarmann, H., & Usher, M. (2001). Maintentenance of semantic information in capacity-limited short-term memory. Psychonomic Bulletin & Review, 8(3), 568–578. https://doi.org/https://doi.org/10.3758/BF03196193
- Henson, R., Hartley, T., Burgess, N., Hitch, G., & Flude, B. (2003). Selective interference with verbal short-term memory for serial order information: A new paradigm and tests of a timing-signal hypothesis. Quarterly Journal of Experimental Psychology, 56A(8), 1307–1334. https://doi.org/https://doi.org/10.1080/02724980244000747
- Howe, M. L., Wilkinson, S., Garner, S. R., & Ball, L. J. (2016). On the adaptive function of children’s and adults’ false memories. Memory, 24(8), 1062–1077. https://doi.org/https://doi.org/10.1080/09658211.2015.1068335
- Huang, P.-S., Chen, H. C., & Liu, C. H. (2012). The development of Chinese word remote associates test for college students. Psychological Testing, 59, 581–607.
- Hughes, R. W., Hurlstone, M. J., Marsh, J. E., Vachon, F., & Jones, D. M. (2013). Cognitive control of auditory distraction. Impact of task difficulty, foreknowledge, and working memory capacity supports duplex mechanism account. Journal of Experimental Psychology: Human Perception & Performance, 39(2), 539–553. https://doi.org/https://doi.org/10.1037/a0029064
- Hunt, R. R., Smith, R. E., & Toth, J. P. (2016). Category cued recall evokes a generate-recognize retrieval process. Journal of Experimental Psychology: Learning, Memory, & Cognition, 42(3), 339–350. https://doi.org/https://doi.org/10.1037/xlm0000136
- Jarosz, A. F., Colflesh, G. J. H., & Wiley, J. (2012). Uncorking the muse: Alcohol intoxication facilitates creative problem solving. Consciousness & Cognition, 21(1), 487–493. https://doi.org/https://doi.org/10.1016/j.concog.2012.01.002
- Jones, D., Alford, D., Bridges, A., Tremblay, S., & Macken, B. (1999). Organizational factors in selective attention: The interplay of acoustic distinctiveness and auditory streaming in the irrelevant sound effect. Journal of Experimental Psychology: Learning, Memory, & Cognition, 25(2), 464–473. https://doi.org/https://doi.org/10.1037/0278-7393.25.2.464
- Jones, D. M., Alford, D., Macken, W. J., Banbury, S. P., & Tremblay, S. (2000). Interference from degraded auditory stimuli: Linear effects of changing state in the irrelevant sequence. The Journal of the Acoustical Society of America, 108(3), 1082–1088. https://doi.org/https://doi.org/10.1121/1.1288412
- Jones, L. L., & Estes, Z. (2015). Convergent and divergent thinking in verbal analogy. Thinking & Reasoning, 21(4), 473–500. https://doi.org/https://doi.org/10.1080/13546783.2015.1036120
- Jones, D. M., & Macken, W. J. (1993). Irrelevant tones produce an irrelevant speech effect: Implications for phonological coding in working memory. Journal of Experimental Psychology: Learning, Memory, & Cognition, 19(2), 369–381. https://doi.org/https://doi.org/10.1037/0278-7393.19.2.369
- Jones, D. M., Macken, W. J., & Nicholls, A. P. (2004). The phonological store of working memory: Is it phonological and is it a store? Journal of Experimental Psychology: Learning, Memory, & Cognition, 30(3), 656–674. https://doi.org/https://doi.org/10.1037/0278-7393.30.3.656
- Jones, D. M., Madden, C., & Miles, C. (1992). Privileged access by irrelevant speech to short-term memory: The role of changing state. Quarterly Journal of Experimental Psychology, 44A(4), 645–669. https://doi.org/https://doi.org/10.1080/14640749208401304
- Jones, D. M., Marsh, J. E., & Hughes, R. W. (2012). Retrieval from memory: Vulnerable or inviolable? Journal of Experimental Psychology: Learning, Memory, & Cognition, 38(4), 905–922. https://doi.org/https://doi.org/10.1037/a0026781
- Jones, D., Miles, C., & Page, J. (1990). Disruption of proofreading by irrelevant speech: Effects of attention, arousal or memory? Applied Cognitive Psychology, 4(2), 89–108. https://doi.org/https://doi.org/10.1002/acp.2350040203
- Jones, D. M., & Tremblay, S. (2000). Interference by process or context? A reply to Neath (2000). Psychonomic Bulletin & Review, 7(3), 550–558. https://doi.org/https://doi.org/10.3758/BF03214370
- Jung-Beeman, M., Bowden, E. M., Haberman, J., Frymiare, J. L., Arambel-Liu, S., Greenblatt, R., Reber P.J., & Kounios J. (2004). Neural activity when people solve verbal problems with insight. PLoS Biology, 2(4), e97. https://doi.org/https://doi.org/10.1371/journal.pbio.0020097
- Kenett, Y. N., Anaki, D., & Faust, M. (2014). Investigating the structure of semantic networks in low and high creative persons. Frontiers in Human Neuroscience, 8, Article 407, 1–16. https://doi.org/https://doi.org/10.3389/fnhum.2014.00407
- Kenett, Y. N., Levy, O., Kenett, D. Y., Stanley, H. E., Fraust, M., & Havlin, S. (2018). Flexibility of thought in high creative individuals represented by percolation analysis. Proceedings of the National Academy of Sciences, 115(5), 867–872. https://doi.org/https://doi.org/10.1073/pnas.1717362115
- Kizilirmak, J. M., Serger, V., Kehl, J., Öllinger, M., Folta-Schoofs, K., & Richardson-Klavehn, A. (2018). Feelings-of-warmth increase more abruptly for verbal riddles solved with in contrast to without Aha! Experience. Frontiers in Psychology, 9, Article 1404, 1–11. https://doi.org/https://doi.org/10.3389/fpsyg.2018.01404
- Kounios, J., & Beeman, M. (2009). The Aha! moment: The cognitive neuroscience of insight. Current Directions in Psychological Science, 18(4), 210–216. https://doi.org/https://doi.org/10.1111/j.1467-8721.2009.01638.x
- Kuznetsova, A., Brockhoff, P. B., & Christensen, R. H. B. (2017). Lmertest package: Tests in linear mixed effects models. Journal of Statistical Software, 82(13), 1–26. https://doi.org/https://doi.org/10.18637/jss.v082.i13
- Lavric, A., Forstmeier, S., & Rippon, G. (2000). Differences in working memory involvement in analytic and creative tasks and ERP study. NeuroReport, 11(8), 1613–1618. https://doi.org/https://doi.org/10.1097/00001756-200006050-00004
- Lee, C. S., Huggins, A. C., & Therriault, D. J. (2014). A measure of creativity or intelligence? Examining internal and external structure validity evidence of the remote associates test. Psychology of Aesthetics, Creativity, & the Arts, 8(4), 446–460. https://doi.org/https://doi.org/10.1037/a0036773
- Lee, C. S., & Therriault, D. J. (2013). The cognitive underpinnings of creative thought: A latent variable analysis exploring the roles of intelligence and working memory in three creative thinking processes. Intelligence, 41(5), 306–320. https://doi.org/https://doi.org/10.1016/j.intell.2013.04.008
- Lenth, R., Singmann, H., Love, J., Buerkner, P., & Herve, M. (2018). emmeans: Estimated marginal means, aka least-squares means. R package. See https://CRAN.R-project.org/package=emmeans
- Lev-Ari, S., & Keysar, B. (2010). Why don't we believe non-native speakers? The influence of accent on credibility. Journal of Experimental Social Psychology, 46(6), 1093–1096. https://doi.org/https://doi.org/10.1016/j.jesp.2010.05.025
- Marko, M., Michalko, D., & Riečanský, I. (2019). Remote associates test: An empirical proof of concept. Behavior Research Methods, 51(6), 2700–2711. https://doi.org/https://doi.org/10.3758/s13428-018-1131-7
- Marsh, J. E., Beaman, C. P., Hughes, R. W., & Jones, D. M. (2012). Inhibitory control in memory: Evidence for negative priming in free recall. Journal of Experimental Psychology: Learning, Memory, & Cognition, 38(5), 1377–1388. https://doi.org/https://doi.org/10.1037/a0027849
- Marsh, J. E., Crawford, J. C., Pilgrim, L. K., Sörqvist, P., & Hughes, R. W. (2017). Trouble articulating the right words: Evidence for a response-exclusion account of distraction during semantic fluency. Scandinavian Journal of Psychology, 58(5), 367–372. https://doi.org/https://doi.org/10.1111/sjop.12386
- Marsh, J. E., Hughes, R. W., & Jones, D. M. (2008). Auditory distraction in semantic memory: A process-based approach. Journal of Memory & Language, 58(3), 682–700. https://doi.org/https://doi.org/10.1016/j.jml.2007.05.002
- Marsh, J. E., Hughes, R. W., & Jones, D. M. (2009). Interference by process, not content, determines semantic auditory distraction. Cognition, 110(1), 23–38. https://doi.org/https://doi.org/10.1016/j.cognition.2008.08.003
- Marsh, J. E., & Jones, D. M. (2010). Cross-modal distraction by background speech. What role for meaning? Noise & Health, 12(49), 210–216. https://doi.org/https://doi.org/10.4103/1463-1741.70499
- Martin, R. C., Wogalter, M. S., & Forlano, J. G. (1988). Reading comprehension in the presence of unattended speech and music. Journal of Memory & Language, 27(4), 382–398. https://doi.org/https://doi.org/10.1016/0749-596X(88)90063-0
- Martindale, C. (1989). Personality, situation, and creativity. In J. A. Glover, R. R. Ronning, & C. R. Reynolds (Eds.), Handbook of creativity: Perspectives on individual differences. Springer. https://doi.org/10.1007/978-1-4757-5356-1_13.
- Martindale, C. (1995). Creativity and connectionism. In S. M. Smith, T. B. Ward, & R. A. Finke (Eds.), The creative cognition approach (pp. 249–268). MIT Press.
- Marupaka, N., Iyer, L. R., & Minai, A. A. (2012). Connectivity and thought: The influence of semantic network structure in a neurodynamic model of thinking. Neural Networks, 32, 147–158. https://doi.org/https://doi.org/10.1016/j.neunet.2012.02.004
- McCabe, D. P. (2008). The role of covert retrieval in working memory span tasks: Evidence from delayed recall tests. Journal of Memory & Language, 58(2), 480–494. https://doi.org/https://doi.org/10.1016/j.jml.2007.04.004
- Mednick, S. (1962). The associative basis of the creative process. Psychological Review, 69(3), 220–232. https://doi.org/https://doi.org/10.1037/h0048850
- Meng, Z., Lan, Z., Yan, G., Marsh, J. E., & Liversedge, S. P. (2020). Task demands modulate the effects of speech on text processing. Journal of Experimental Psychology: Learning, Memory, & Cognition, 46(10), 1892–1905. https://doi.org/https://doi.org/10.1037/xlm0000861
- Merten, T., & Fischer, I. (1999). Creativity, personality and word association responses: Associative behaviour in forty supposedly creative persons. Personality & Individual Differences, 27(5), 933–942. https://doi.org/https://doi.org/10.1016/S0191-8869(99)00042-2
- Miyake, A., Emerson, M. J., Padilla, F., & Ahn, J.-C. (2004). Inner speech as retrieval aid for task goals: The effects of cue type and articulatory suppression in the random task cuing paradigm. Acta Psychologica, 115(2-3), 123–142. https://doi.org/https://doi.org/10.1016/j.actpsy.2003.12.004
- Miyake, A., Friedman, N. P., Emerson, M. J., Witzki, A. H., Howerter, A., & Wager, T. D. (2000). The unity and diversity of executive functions and their contributions to complex “frontal lobe” tasks: A latent variable analysis. Cognitive Psychology, 41(1), 49–100. https://doi.org/https://doi.org/10.1006/cogp.1999.0734
- Mok, L. W. (2014). The interplay between spontaneous and controlled processing in creative cognition. Frontier in Human Neuroscience, 8, Article 663, 1–5. https://doi.org/https://doi.org/10.3389/fnhum.2014.00663
- Morris, N., & Jones, D. M. (1990). Habituation to irrelevant speech: Effects on a visual short-term memory task. Perception & Psychophysics, 47(3), 291–297. https://doi.org/https://doi.org/10.3758/BF03205003
- Murray, D. J. (1968). Articulation and acoustic confusability in short-term memory. Journal of Experimental Psychology, 78(4, Pt.1), 679–684. https://doi.org/https://doi.org/10.1037/h0026641
- Neath, I. (2000). Modeling the effects of irrelevant speech on memory. Psychonomic Bulletin & Review, 7(3), 403–423. https://doi.org/https://doi.org/10.3758/BF03214356
- Neely, C. B., & LeCompte, D. C. (1999). The importance of semantic similarity to the irrelevant speech effect. Memory & Cognition, 27(1), 37–44. https://doi.org/https://doi.org/10.3758/BF03201211
- Newell, A., & Simon, H. A. (1972). Human problem solving. Prentice-Hall.
- Nijstad, B. A., De Dreu, C. K., Rietzschel, E. F., & Baas, M. (2010). The dual pathway to creativity model: Creative ideation as a function of flexibility and persistence. European Review of Social Psychology, 21(1), 34–77. https://doi.org/https://doi.org/10.1080/10463281003765323
- Nishiyama, R. (2013). Dissociative contributions of semantic and lexical-phonological information to immediate recognition. Journal of Experimental Psychology: Learning, Memory, & Cognition, 39(2), 642–648. https://doi.org/https://doi.org/10.1037/a0029160
- Nishiyama, R. (2018). Separability of active semantic phonological maintenance in verbal working memory. PLoS One, 13(3), e0193808, 1–16. https://doi.org/https://doi.org/10.1371/journal.pone.0193808
- Nusbaum, E. C., & Silvia, P. J. (2011). Are intelligence and creativity really so different?: Fluid intelligence, executive processes, and strategy use in divergent thinking. Intelligence, 39(1), 36–45. https://doi.org/https://doi.org/10.1016/j.intell.2010.11.002
- Ohlsson, S. (1992). Information-processing explanations of insight and related phenomena. In M. T. Keane, & K. J. Gilhooly (Eds.), Advances in the psychology of thinking (Vol. 1, pp. 1–44). Harvester-Wheatsheaf.
- Ohlsson, S. (2011). Deep learning: How the mind overrides experience. Cambridge University Press.
- Olteţeanu, A. M., Schultheis, H., & Dyer, J. B. (2018). Computationally constructing a repository of compound remote associates test items in American English with comRAT-G. Behavior Research Methods, 50(5), 1971–1980. https://doi.org/https://doi.org/10.3758/s13428-017-0965-8
- Oswald, C. J. P., Tremblay, S., & Jones, D. M. (2000). Disruption of comprehension by the meaning of irrelevant sound. Memory, 8(5), 345–350. https://doi.org/https://doi.org/10.1080/09658210050117762
- Perkins, D. (2000). The Eureka effect: The art and logic of breakthrough thinking. Norton.
- Richardson, J. T. (1984). Developing the theory of working memory. Memory & Cognition, 12(1), 71–83. https://doi.org/https://doi.org/10.3758/BF03196999
- Röer, J. P., Körner, U., Buchner, A., & Bell, R. (2017). Semantic priming by irrelevant speech. Psychological Bulletin & Review, 24(4), 1205–1210. https://doi.org/https://doi.org/10.3758/s13423-016-1186-3
- Rosen, V. M., & Engle, R. W. (1997). The role of working memory capacity in retrieval. Journal of Experimental Psychology: General, 126(3), 211–227. https://doi.org/https://doi.org/10.1037/0096-3445.126.3.211
- Rossmann, E., & Fink, A. (2010). Do creative people use shorter association pathways? Personality & Individual Differences, 49(8), 891–895. https://doi.org/https://doi.org/10.1016/j.paid.2010.07.025
- Saint-Aubin, J., & Poirier, M. (1999). Semantic similarity and immediate serial recall: Is there a detrimental effect on order information? Quarterly Journal of Experimental Psychology, 52A(2), 367–394. https://doi.org/https://doi.org/10.1080/713755814
- Salamé, P., & Baddeley, A. (1990). The effects of irrelevant speech on immediate free recall. Bulletin of the Psychonomic Society, 28(6), 540–542. https://doi.org/https://doi.org/10.3758/BF03334073
- Salvi, C., Bricolo, E., Kounios, J., Bowden, E., & Beeman, M. (2016). Insight solutions are correct more often than analytic solutions. Thinking & Reasoning, 22(4), 443–460. https://doi.org/https://doi.org/10.1080/13546783.2016.1141798
- Sandkühler, S., & Bhattacharya, J. (2008). Deconstructing insight: EEG correlates of insightful problem solving. PLoS One, 3(1), e1459, 1–12. https://doi.org/https://doi.org/10.1371/journal.pone.0001459
- Schooler, J. W., & Melcher, J. (1995). The ineffability of insight. In S. M. Smith, T. B. Ward, & R. A. Finke (Eds.), The creative cognition approach (pp. 97–133). MIT Press.
- Schooler, J. W., Ohlsson, S., & Brooks, K. (1993). Thoughts beyond words: When language overshadows insight. Journal of Experimental Psychology: General, 122(2), 166–183. https://doi.org/https://doi.org/10.1037/0096-3445.122.2.166
- Scott, S. K., Rosen, S., Beaman, C. P., Davis, J. P., & Wise, R. J. S. (2009). The neural processing of masked speech: Evidence for different mechanisms in the left and right temporal lobes. The Journal of the Acoustical Society of America, 125(3), 1737–1743. https://doi.org/https://doi.org/10.1121/1.3050255
- Shivde, G., & Thompson-Schill, S. L. (2004). Dissociating semantic and phonological maintenance using fMRI. Cognitive, Affective, & Behavioral Neuroscience, 4(1), 10–19. https://doi.org/https://doi.org/10.3758/CABN.4.1.10
- Silvia, P. J., Beaty, R. E., & Nusbaum, E. C. (2013). Verbal fluency and creativity: General and specific contributions of broad retrieval ability (Gr) factors to divergent thinking. Intelligence, 41(5), 328–340. https://doi.org/https://doi.org/10.1016/j.intell.2013.05.004
- Smith, S. M., & Blankenship, S. E. (1989). Incubation effects. Bulletin of the Psychonomic Society, 27(4), 311–314. https://doi.org/https://doi.org/10.3758/BF03334612
- Smith, K. A., Huber, D. E., & Vul, E. (2013). Multiply-constrained semantic search in the remote associates test. Cognition, 128(1), 64–75. https://doi.org/https://doi.org/10.1016/j.cognition.2013.03.001
- Smith, S. M., Sifonis, C. M., & Angello, G. (2012). Clue insensitivity in remote associates test problem solving. The Journal of Problem Solving, 4(2), 28–49. https://doi.org/https://doi.org/10.7771/1932-6246.1124
- Smith, J. D., Wilson, M., & Reisberg, D. (1995). The role of subvocalization in auditory imagery. Neuropsychologia, 33(11), 1433–1454. https://doi.org/https://doi.org/10.1016/0028-3932(95)00074-D
- Sörqvist, P., Nöstl, A., & Halin, N. (2012). Disruption of writing processes by the semanticity of background speech. Scandinavian Journal of Psychology, 53(2), 97–102. https://doi.org/https://doi.org/10.1111/j.1467-9450.2011.00936.x
- Sowden, P. T., Pringle, A., & Gabora, L. (2015). The shifting sands of creative thinking: Connections to dual-process theory. Thinking & Reasoning, 21(1), 40–60. https://doi.org/https://doi.org/10.1080/13546783.2014.885464
- Stokes, K. A., & Arnell, K. M. (2012). New considerations for the cognitive locus of impairment in the irrelevant-sound effect. Memory & Cognition, 40(6), 918–931. https://doi.org/https://doi.org/10.3758/s13421-012-0194-4
- Storm, B. C. (2011). The benefit of forgetting in thinking and remembering. Current Directions in Psychological Science, 20(5), 291–295. https://doi.org/https://doi.org/10.1177/0963721411418469
- Storm, B. C., & Angello, G. (2010). Overcoming fixation: Creative problem solving and retrieval-induced forgetting. Psychological Science, 21(9), 1263–1265. https://doi.org/https://doi.org/10.1177/0956797610379864
- Storm, B. C., Angello, G., & Bjork, E. L. (2011). Thinking can cause forgetting: Memory dynamics in creative problem solving. Journal of Experimental Psychology: Learning, Memory & Cognition, 37(5), 1287–1293. https://doi.org/https://doi.org/10.1037/a0023921
- Storm, B. C., & Koppel, R. H. (2012). Testing the cue dependence of problem-solving-induced forgetting. The Journal of Problem Solving, 4(2), Article 4, 1–16. https://doi.org/https://doi.org/10.7771/1932-6246.1125
- Thomas, R. P., Dougherty, M. R., Sprenger, A. M., & Harbison, J. I. (2008). Diagnostic hypothesis generation and human judgment. Psychological Review, 115(1), 155–185. https://doi.org/https://doi.org/10.1037/0033-295X.115.1.155
- Threadgold, E., Marsh, J. E., McLatchie, N., & Ball, L. J. (2019). Background music stints creativity: Evidence from compound remote associate tasks. Applied Cognitive Psychology, 33(5), 873–888. https://doi.org/https://doi.org/10.1002/acp.3532
- Troyer, A. K., Moscovitch, M., Winocur, G., Alexander, M., & Stuss, D. (1998). Clustering and switching on verbal fluency: The effects of focal frontal- and temporal-lobe lesions. Neuropsychologia, 36(6), 499–504. https://doi.org/https://doi.org/10.1016/S0028-3932(97)00152-8
- Turner, M. L., & Engle, R. W. (1989). Is working memory capacity task dependent? Journal of Memory & Language, 28(2), 127–154. https://doi.org/https://doi.org/10.1016/0749-596X(89)90040-5
- Vachon, F., Marsh, J. E., & Labonté, K. (2020). The automaticity of semantic processing revisited: Auditory distraction by a categorical deviation. Journal of Experimental Psychology: General, 149(7), 1360–1397. https://doi.org/https://doi.org/10.1037/xge0000714
- Van Casteren, M., & Davis, M. H. (2007). Match: A program to assist in matching the conditions of factorial experiments. Behavior Research Methods, 39(4), 973–978. https://doi.org/https://doi.org/10.3758/BF03192992
- Venables, W. N., & Ripley, B. D. (2002). Modern applied statistics with S (4th ed.). Springer. http://www.stats.ox.ac.uk/pub/MASS4/
- Wallace, G. L., Peng, C. S., & Williams, D. (2017). Interfering with inner speech selectively disrupts problem solving and is linked with real-word executive functioning. Journal of Speech, Language & Hearing Research, 60(12), 3456–3460. https://doi.org/https://doi.org/10.1044/2017_JSLHR-S-16-0376
- Webb, M. E., Little, D. R., & Cropper, S. J. (2016). Insight is not in the problem: Investigating insight in problem solving across task types. Frontiers in Psychology, 7, Article 1424, 1–13. https://doi.org/https://doi.org/10.3389/fpsyg.2016.01424
- Weiland, H., Bambini, V., & Schumacher, P. B. (2014). The role of literal meaning in figurative language comprehension: Evidence from masked priming ERP. Frontiers in Human Neuroscience, 8, Article 583, 1–17. https://doi.org/https://doi.org/10.3389/fnhum.2014.00583
- Weisberg, R. W. (2015). Toward an integrated theory of insight in problem solving. Thinking & Reasoning, 21(1), 5–39. https://doi.org/https://doi.org/10.1080/13546783.2014.886625
- Weisberg, R. W. (2018). Problem solving. In L. J. Ball, & V. A. Thompson (Eds.), The Routledge international handbook of thinking and reasoning (pp. 607–623). Routledge.
- Wiley, J., & Jarosz, A. F. (2012). Working memory capacity, attentional focus and problem solving. Current Directions in Psychological Science, 21(4), 258–262. https://doi.org/https://doi.org/10.1177/0963721412447622
- Winsler, A., Fernyhough, C., & Montero, I. (2009). Private speech, executive functioning, and the development of verbal self-regulation. Cambridge University Press. doi:10.1017/CBO9780511581533.
- Wu, C. L., & Chen, H. C. (2017). Normative data for Chinese compound remote associate problems. Behaviour Research Methods, 49(6), 2163–2172. doi:https://doi.org/10.3758/s13428-016-0849-3