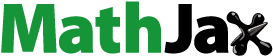
ABSTRACT
This study investigated whether unsuccessful transfer of critical thinking (CT) would be due to recognition, recall, or application problems (cf. three-step model of transfer). In two experiments (laboratory: N = 196; classroom: N = 104), students received a CT-skills pretest (including learning, near transfer, and far transfer items), CT-instructions, practice problems, and a CT-skills posttest. On the posttest transfer items, students either (1) received no support, (2) received recognition support, (3) were prompted to recall acquired knowledge, or (4) received recall support. Results showed that CT could be fostered through instruction and practice: we found learning, near transfer, and (albeit small) far transfer performance gains and reduced test-taking time. There were no significant differences between the four support conditions, however, suggesting that the difficulty of transfer of CT-skills lies in problems with application/mapping acquired knowledge onto new tasks. Additionally, exploratory results on free recall data suggested suboptimal recall can be a problem as well.
Every day, we have to make a multitude of quick but sound judgments and decisions. Since our working-memory capacity and duration are limited and we cannot process all the information around us, we have to resort to heuristics (i.e. mental shortcuts) that ease reasoning processes (Tversky & Kahneman, Citation1974). Usually, heuristic reasoning is very functional and inconsequential—think, for example, of where you decide to sit in a train—but it also makes us prone to illogical and biased decisions (i.e. deviating from ideal normative standards derived from logic and probability theory) that can have a significant impact. To illustrate, a forensic expert who misjudges fingerprint evidence because it verifies his or her preexisting beliefs concerning the likelihood of the guilt of a defendant, displays the so-called confirmation bias, which can result in a misidentification and a wrongful conviction (e.g. the Madrid bomber case; Kassin et al., Citation2013).
To reduce or eliminate biased decisions and to successfully function in today’s society, one should engage in critical thinking (CT: e.g. Dewey, Citation1910; Pellegrino & Hilton, Citation2012). In the field of educational assessment and instruction, CT is generally defined as “purposeful, self-regulatory judgment that results in interpretation, analysis, evaluation, and inference, as well as explanation of the evidential, conceptual, methodological, criteriological, or contextual considerations on which that judgment is based” (APA: Facione, Citation1990, p. 2). According to this widely used definition, “the ideal critical thinker is habitually inquisitive, well-informed, trustful of reason, open-minded, flexible, fair-minded in evaluation, honest in facing personal biases, prudent in making judgments, willing to reconsider, clear about issues, orderly in complex matters, diligent in seeking relevant information, reasonable in the selection of criteria, focused in inquiry, and persistent in seeking results which are as precise as the subject and the circumstances of inquiry permit” (Facione, Citation1990, p. 3). Despite the variety of definitions of CT and the multitude of components CT encompasses (cf. Facione, Citation1990), there appears to be agreement that one key aspect of CT is the ability to avoid bias in reasoning and decision-making (Baron, Citation2008; Duron et al., Citation2006; Facione, Citation1990; West et al., Citation2008), such as overturning belief-biased responses when evaluating the logical validity of arguments. Biases occur when people rely on heuristic reasoning (i.e. Type 1 processing) when that is not appropriate, do not recognize the need for analytical or reflective reasoning (i.e. Type 2 processing), are not willing to switch to Type 2 processing or unable to sustain it, or miss the relevant mindware to come up with a better response (e.g. Evans, Citation2003; Stanovich, Citation2011). Consequently, in order to prevent biased reasoning, it is necessary to stimulate people to switch to Type 2 processing. However, that may not be enough if the lack they lack the relevant mindware, so in many cases, mindware has to be taught as well.
It is not surprising that educational researchers, practitioners, and policymakers agree that CT is one of the most valued and sought-after skills that higher education students are expected to learn (Davies, Citation2013; Facione, Citation1990; Halpern, Citation2014; Van Gelder, Citation2005). Consequently, there is a substantial body of research on teaching CT-skills (Abrami et al., Citation2008, Citation2014) including reducing biases in reasoning (e.g. Van Peppen et al., Citation2018, Citation2021a; Flores et al., Citation2012; Heijltjes et al., Citation2014a, Citation2014b, Citation2015; Janssen et al., Citation2019; Kuhn, Citation2005; Sternberg, Citation2001). It is well established, for instance, that explicit teaching of CT combined with practice improves learning of CT-skills required for unbiased reasoning. However, transfer to similar tasks that were not instructed or practiced is very hard to establish (Van Peppen et al., Citation2018, Citation2021a; Heijltjes et al., Citation2014a, Citation2014b, Citation2015). As it would be unfeasible to train students on each and every type of reasoning bias they will ever encounter, there is increased concern as to how to promote transfer of these skills (and this also applies to CT-skills more generally, see, for example, Halpern, Citation2014; Kenyon & Beaulac, Citation2014; Lai, Citation2011; Ritchhart & Perkins, Citation2005).
The process of transfer
Transfer is the process of applying one’s prior knowledge or skills to some new context or related materials (e.g. Barnett & Ceci, Citation2002; Cormier & Hagman, Citation2014; Druckman & Bjork, Citation1994; McDaniel, Citation2007; Perkins & Salomon, Citation1992). Transfer involves gradients of similarity between the initial and novel situation, so that transfer between situations that have less in common occurs less often than transfer between closely related situations (e.g. Barnett & Ceci, Citation2002; Dinsmore et al., Citation2014). In the educational psychology literature, transfer is usually subdivided into near and far transfer, differentiating in degree of similarity between the initial task or situation and the transfer task or situation (e.g. Perkins & Salomon, Citation1992). Transferring knowledge or skills to a very similar situation, for instance, problems in an exam of the same kind as that have been practiced during the lessons, refers to “near” transfer. By contrast, transferring between situations that share similar structural features but, on appearance, seem remote and alien to one another is considered “far” transfer. It is important to realize, however, that near and far transfer occur on a continuum and do not imply any precise codification of closeness (Salomon & Perkins, Citation1989), for instance, because people differ considerably in their ability to identify similarities between different problem situations. In their attempt to bring clarity to the literature on transfer of knowledge, Barnett and Ceci (Citation2002) developed a taxonomy in which they conceptualized transfer as a three-step process in which learners need to (a) recognize that acquired knowledge is relevant in a new context, (b) recall that knowledge, and (c) apply that knowledge to the new context.
Previous research has shown that to promote successful (far) transfer of learning, instructional strategies should contribute to permanent changes, by creating effortful learning conditions that trigger active and deep processing (i.e. generative processing; e.g. Fiorella & Mayer, Citation2016; Wittrock, Citation2010). More specifically, it is important that learners explore similarities and differences between different problem types to acquire better mental representations of the structural features of the different types of problems (i.e. schemas; Bassok & Holyoak, Citation1989; Fiorella & Mayer, Citation2016; Holland et al., Citation1989; Wittrock, Citation2010). Ways to stimulate this are, for instance, creating variability in practice (e.g. Barreiros et al., Citation2007; Moxley, Citation1979) or encouraging elaboration, questioning, or explanation during practice (e.g. Fiorella & Mayer, Citation2016; Renkl & Eitel, Citation2019). Taken together, transfer of learning can occur when a learner acquires an abstract action schema responsive to the requirements of a problem. If the potential transfer situation presents similar requirements and the learner recognizes them, they may apply (or map) the same or a somewhat adapted action schema to solve the novel problem (e.g. Gentner, Citation1983, Citation1989; Mayer & Wittrock, Citation1996; Reed, Citation1987; Vosniadou & Ortony, Citation1989).
When interventions that encourage generative processing are applied to CT-skills, however, it is often found that they promote learning but not transfer; the effects hardly seem to transfer across tasks or domains (Halpern & Butler, Citation2019; Ritchhart & Perkins, Citation2005; Tiruneh et al., Citation2014, Citation2016). Research that focused on teaching unbiased reasoning has uncovered that a combination of instruction and task practice enhances transfer to isomorphic problems, i.e. same structural features/problem type but different superficial features, meaning other values or story contexts; in this study we refer to the ability to solve such problems after instruction as evidence of learning (e.g. Heijltjes et al., Citation2014b). However, it was shown that CT-skills required for unbiased reasoning consistently failed to transfer to novel problem types that have different structural features yet share underlying principles, i.e. far transfer, even when using instructional methods that proved effective for fostering transfer in various other domains. These methods, administered after initial instruction, were encouraging students to self-explain during practice (Van Peppen et al., Citation2018; Heijltjes et al., Citation2014a, Citation2014b, Citation2015) and offering variable as opposed to blocked practice with examples or problems (i.e. interleaved practice; Van Peppen et al., Citation2021c). Other methods involved comparing correct and erroneous worked out examples (Van Peppen et al., Citation2021a) and repeated retrieval practice (i.e. testing effect; Van Peppen et al., Citation2021b). Additionally, a recent study with teachers who were trained on (teaching) CT in three sessions and engaged in effortful learning activities (i.e. designing a CT-task; Janssen et al., Citation2019), found no evidence of transfer to novel problems.
These findings raise the question of what obstacle(s) underlie(s) the lack of transfer of CT-skills required for unbiased reasoning. According to the three-step process of transfer (Barnett & Ceci, Citation2002), the lack of transfer in previous studies could lie in a recognition, recall, or application problem. As mentioned above, understanding the obstacle(s) underlying (un)successful transfer is crucial to design courses to achieve it and, moreover, is relevant for theories of learning and transfer.
The present study
In the current study, we, therefore, investigated different conditions during the final test procedure that support the recognition, recall, and application steps in the transfer process (cf. Butler et al., Citation2013, Citation2017; for a similar procedure, see Gick & Holyoak, Citation1980, Citation1983). By comparing the effects of support for different steps in the process, we infer where difficulties arise for learners. We simultaneously conducted two experiments: Experiment 1 in a laboratory setting and Experiment 2 in a classroom setting (i.e. replication experiment to assess the robustness of our findings and to increase ecological validity). Participants first completed a pretest and, thereafter, received video-instructions on CT and on specific CT-tasks. Subsequently, they practiced with these tasks on domain-specific problems, followed by correct-answer feedback and a worked example. Finally, participants completed a posttest—including learning (i.e. same problem type but different story contexts), near transfer (i.e. same problem type but offered in a different/less abstract format), and far transfer (i.e. similar principles but different problem types: see method section for more information) items.
The experimental intervention took place during the posttest. Participants were randomly allocated to one of four conditions, in which they completed the near and far transfer posttest items: (1) without receiving support (no support condition), (2) while receiving hints that the information provided in the learning phase is relevant for these items (recognition support condition), (3) while receiving hints that the information provided in the learning phase is relevant and being prompted to recall the acquired knowledge (free recall condition), or (4) while receiving hints that the information provided in the learning phase is relevant and receiving a reminder of the paper-based overview of that information that they received prior to the transfer tasks (recall support condition).
provides a schematic overview of the logic behind the procedure. If the lack of transfer is only due to participants’ ability to recognize that the acquired knowledge is relevant to the new task, then receiving a hint that the knowledge is relevant should be sufficient to establish transfer. Thus, if inadequate recognition underlies the problem, we expected greater performance gains on transfer items in all conditions compared to the no support condition. (Hypothesis 1: no support < recognition support = free recall = recall support). If, however, participants are able to recognize the relevance but have problems recalling the exact rules of logic, then presenting these rules while completing the transfer items would lead to greater performance gains on transfer items than the no support, recognition support, and free recall condition. If participants are not able to recall any of the information, we expected no differences in transfer performance gains between the free recall and recognition support condition (Hypothesis 2a: no support = recognition support = free recall < recall support). But if they can retrieve some of the relevant information, we expected higher transfer performance gains in the free recall condition compared to the recognition support condition (Hypothesis 2b: no support = recognition support < free recall < recall support). If, within the free recall condition, participants’ ability to recall the acquired knowledge positively correlates with their performance on transfer items, that would provide further evidence for the assumption that suboptimal recall underlies the lack of transfer. Finally, if difficulties in applying the relevant knowledge onto the new task underlie the lack of transfer—while participants are able to recognize that the acquired knowledge is relevant and to recall that knowledge—there would be no differences in transfer performance gains between conditions (Hypothesis 3: no support = recognition support = recall support = free recall).
Table 1. The logic behind the procedure used.
Experiment 1
Method
The hypotheses, planned analyses, and method section were preregistered on the Open Science Framework (OSF). Detailed descriptions of the design and procedures and all data/script files and materials (in Dutch) are publicly available on the project page we created for this study (osf.io/ybt5g).
Participants
Participants were 196 first-year and second-year Psychology students attending a Dutch University. Of these, two students were unable to complete the free recall due to an experimenter error and six students did not adhere to instructions (i.e. they copied information from the CT-instructions). They were therefore excluded from the analyses and this resulted in a final sample size of 188 students (Mage = 20.59, SD = 2.53; 69 males). Four students who were originally allocated to the recall support condition did not receive the reminder of the information provided in the learning phase and were therefore automatically assigned to the recognition support condition (i.e. they only received the recognition support).
Based on the sample size of 188 students, a power function for mixed ANOVAs with a single within-subjects factor (two levels) and a single between-subjects factor (four levels) using the G*Power software (Faul et al., Citation2009), shows that the power of our study—under a fixed alpha level of 0.05 and with a correlation between measures of 0.3—is estimated at .47, >.99, and >.99 for detecting a small (), medium (
), and large (
) interaction effect, respectively. Thus, the power of our study should be sufficient to at least pick up medium-sized interaction effects.
Design
The experiment consisted of three phases (see for an overview) and had a 2 (Test Moment: pretest and posttest) × 4 (Condition: no support, recognition support, free recall, recall support) design, with Test Moment as within-subjects factor and Condition as between-subjects factor. Dependent variables were performance on learning, near transfer, and far transfer items. Participants first completed the CT-skills pretest and then received video-based instructions on CT in general and on specific CT-tasks. Subsequently, they practiced with these tasks on domain-specific problems, followed by correct-answer feedback and a worked example that showed the correct line of reasoning. After a short break of four minutes, participants completed a posttest including learning, near transfer, and far transfer items (for more information see materials subsection). They started with the learning items and were thereafter randomly allocated to one of four conditions. Depending on assigned condition, they completed the near and far transfer items: (1) without receiving support (no support condition, n = 47), (2) while receiving hints that the information provided in the learning phase is relevant for these items (recognition support condition, n = 55), (3) while receiving hints that the information provided in the learning phase is relevant and being prompted to recall the acquired knowledge (free recall condition, n = 44), or (4) while receiving hints that the information provided in the learning phase is relevant and receiving a reminder of the paper-based overview of that information that they received prior to the transfer tasks (recall support condition, n = 42). Time-on-task was logged during all phases.
Materials
All materials were administered as an online survey with a forced response-format using Qualtrics Survey Software (Qualtrics, Provo, UT; http://www.qualtrics.com).
CT-skills tests
In line with previous research on avoiding bias in reasoning and decision-making, we used several heuristics-and biases tasks as measures of CT (e.g. Stanovich et al., Citation2016; Tversky & Kahneman, Citation1974; West et al., Citation2008). As mentioned in the introduction, learning/transfer occur on a continuum and represent different gradients of similarity (not necessarily difficulty) with the initial CT-tasks. Learning via isomorphic problems with the same structural features as the initial tasks but different superficial features (i.e. different topic/cover story) is considered as evidence of learning and transferring knowledge or skills to a very similar situation to the initial task or situation is considered “near” transfer. Given that the initial tasks were general syllogistic reasoning tasks, we developed syllogistic reasoning tasks in a slightly different format to assess near transfer. Transferring between situations that share similar structural features but, on appearance, seem remote and alien to one another is considered “far” transfer. Hence, we used Wason selection tasks, that are novel tasks but share similar principles with syllogistic reasoning tasks, to assess far transfer. Thus, students’ performance was measured on general syllogistic reasoning tasks with different story contexts (to assess learning), syllogistic reasoning tasks in a different/less abstract format, i.e. vignettes (to assess near transfer), and Wason selection tasks that are novel tasks but share similar principles with syllogistic reasoning tasks (to assess far transfer) both on a pretest and immediate posttest. The pretest and posttest contained parallel versions of the learning, near transfer, and far transfer items. To illustrate, a posttest item contained the exact same wording as the respective pretest items but, for instance, described a different company.
In all tasks, belief bias played a role. Belief bias occurs when the conclusion aligns with prior beliefs or real-world knowledge (i.e. is believable) but is invalid, or vice versa (Evans et al., Citation1983; Markovits & Nantel, Citation1989; Newstead et al., Citation1992). These tasks require that one recognizes the need for analytical and reflective reasoning (i.e. based on knowledge and rules of logic) and switches to this type of reasoning. This is only possible, however, when heuristic responses are successfully inhibited. Example items of each task category are provided in Appendix B. For the sake of comparability, the content of the surface features (cover stories) of all test items was the same for both experiments and was based on the study domain of participants of Experiment 2 (because that experiment was conducted as part of an existing course), namely “Biology and Medical Laboratory Research” and “Chemistry”. The content of the tasks referred to very general knowledge these students could be expected to hold. In the tasks, the logical validity of the conclusions conflicted strongly with that general knowledge (i.e. the tasks likely evoked belief biases). The content of all materials was evaluated and approved by a teacher working in the domain (who also taught CT as part of her courses), to ensure that the tasks were authentic and fit for the study purpose (e.g. the teacher evaluated the believability of the conclusions, as well as the equivalence of pretest and posttest tasks).
Learning items
Each test contained eight conditional syllogistic reasoning items that measured learning (hence, hereafter referred to as learning items), as these were instructed and practiced during the learning phase. All items included a belief bias and examined the tendency to be influenced by the believability of a conclusion when evaluating the logical validity of arguments (Evans, Citation2003; Evans et al., Citation1983). Conditional syllogisms consist of a premise including a conditional statement and a premise that either affirms or denies either the antecedent or the consequent. Our tests contained 2 × affirming the consequent of a conditional (if p then q, q therefore p; conclusion invalid but believable); 2 × denying the consequent of a conditional (if p then q, not q therefore not p; conclusion valid but unbelievable); 2 × affirming the antecedent of a conditional (if p then q, p therefore q; conclusion valid but unbelievable); and 2 × denying the antecedent of a conditional (if p then q, not p therefore not q; conclusion invalid but believable). Participants had to indicate for each item whether the conclusion is valid or invalid. Thereafter, they were asked to explain their multiple-choice answer. The forced response-format of these items required them to guess if they did not know the answer.
Near transfer items
For each test, we constructed six short vignettes (about 100 words) to assess whether students are able to evaluate the logical validity of arguments in a written news item or article on a topic that participants might encounter in their working life. Each vignette contained a logically invalid but believable conclusion or a logically valid but unbelievable conclusion from two given premises (i.e. conditional syllogisms). These items reflected near transfer items as they were offered in a different format/situation compared to the learning phase. Participants were instructed to read the text thoroughly, to indicate whether the conclusion in the text is valid or invalid, and to provide an explanation. To illustrate, students read a short text from an article about a novel vaccine against HIV/AIDS developed in the Netherlands, stating that if a country develops a particular vaccine against a virus, the risk of that virus is higher in that country than elsewhere. Students were asked to indicate whether the conclusion that there is a higher risk of HIV in the Netherlands than elsewhere, is valid or invalid based on the information given in the text (correct answer is “valid”, for more information see Appendix B).
Far transfer items
Each test contained six Wason selection items that measured the tendency to confirm a hypothesis rather than to falsify it (adapted from Evans, Citation2002; Gigerenzer & Hug, Citation1992). These items reflected far transfer items as they were not explicitly instructed and practiced during the learning phase but shared similar features with the four forms of conditional syllogistic reasoning (i.e. each item required recall and application of all four conditional syllogism principles to solve it correctly). For each of the two forms of Wason selection items (abstract or concrete, with the latter being study-related), there were three test items. A multiple-choice forced-response format with four answer options was used (cf. four forms of conditional syllogistic reasoning) in which only a specific combination of two selected answers was the correct answer. Thereafter, participants were asked to explain their multiple-choice answer. Again, all correct answers were related to reasoning strategies and incorrect answers were related to biased reasoning. For example, students were presented with four medical files, with information about the cause of death on the one hand (unnatural or natural) and whether or not autopsy has been conducted. They were provided with the rule that “if there are indications of an unnatural death, autopsy will be conducted” and asked which medical files they should read to check if the rule is correct (correct answer is “unnatural death file” + ”no autopsy file”, for more information see Appendix B).
Supporting prompts
Depending on assigned condition, participants received different levels of support while completing the near and far transfer items of the posttest. Participants in the no support condition completed the near and far transfer items without receiving additional support. In the recognition support condition, participants received a prompt that emphasized the relevance of the information provided in the learning phase: “To solve this task, you can use the rules of logic explained in the instructions”. In the free recall condition, participants were first asked to recall the rules of logic explained in the instruction and to write them down on the blank paper they received. Then participants completed each near and far transfer item while receiving the following prompt: “To solve this task, you can use the rules of logic explained in the instructions that you tried to recall beforehand. Take that paper to solve the task”.
In the recall support condition, participants were requested to pick up a paper from the experiment leader and they received a prompt that emphasized the relevance of the information provided in the learning phase and that indicated where they could find this information: “To solve this task, you can use the rules of logic explained in the instructions. You can find these rules in the overview on the paper that you have received. Take that paper to solve the task”. For the detailed description of the supporting prompts and the rules of logic that participants in the recall support condition receive, see Appendix A.
CT-instructions
The video-based CT-instructions (15 min) consisted of a general instruction on CT and explicit instructions on avoiding belief-bias in syllogistic reasoning. In the general instruction, the features of CT and the attitudes and skills that are needed to think critically were described. These were followed by the explicit instructions on rules of logic and avoiding belief-bias in syllogistic reasoning, which consisted of a worked example of each form of syllogistic reasoning included in the pretest. The worked examples not only showed the rationale behind the solution steps but also included possible problem-solving strategies which allowed participants to mentally correct initially erroneous responses. The explicit instructions served to stimulate students to inhibit heuristic responses when needed, but, given that that may not be enough to prevent bias in reasoning if they lack the necessary mindware, the mindware (i.e. knowledge and rules of logic) was taught as well. At the end of the video-based instruction, participants received a hint stating that the principles used in these examples can be applied to several other reasoning tasks.
CT-practice
After the video-based instruction, participants practiced with the four types of syllogistic reasoning problems of the pretest and explicit instructions, on topics that they might encounter in their working-life. Participants were instructed to read the problems thoroughly, to choose the best multiple-choice answer option, and to give a written explanation of how the answer was obtained in a text entry box below the multiple-choice question. After each practice-task, participants received correct-answer feedback (e.g. “You gave the following answer: conclusion follows logically from the two premises. This answer is incorrect”.) and were given a worked example that consisted of the problem statement and a correct solution to this problem. The line of reasoning and the underlying principles were explained in steps and clarified with a visual representation. Again, participants were asked to read the worked examples thoroughly before they continued to the next problem. The content of the surface features (cover stories) of all practice items was adapted to the study domain of participants of Experiment 2 (i.e. Biology and Medical Laboratory Research/Chemistry), because that experiment was conducted in a classroom setting as part of an existing course.
Procedure
Experiment 1 was run in the computer lab of the university and lasted circa 90 min. One experiment leader (first author of this paper or research assistant) was present during all phases of the experiment. Participants were seated in individual cubicles, where A4-papers were distributed before they arrived. These papers contained some general rules, a link to the Qualtrics environment where all materials were delivered, and a blank page that was only needed for participants in the free recall condition. The experiment leader first introduced herself and provided some basic information about the experiment. Afterwards, she instructed participants to read the A4-paper containing some general instructions and a link to the Qualtrics environment where they first signed an informed consent form.
Next, participants filled out a short demographic questionnaire and completed the pretest. Thereafter, participants entered the learning phase in which they viewed the video (15 min.) Including the general CT-instruction and the explicit instructions, followed by the four practice problems. Immediately after the learning phase, they took a short break of four minutes in which they could relax or move about. Next, participants completed the learning items of the posttest. Subsequently, the Qualtrics program randomly assigned the participants to one of the four conditions. Depending on assigned condition, participants received different levels of support while completing the near and far transfer items of the posttest (see supporting prompts subsection). Participants could work at their own pace and time-on-task was logged during all phases. Furthermore, participants could use scrap paper during the practice phase and the CT-tests.
Data analysis
Unbiased reasoning items were scored for accuracy based on multiple-choice responses and explanations, using a coding scheme that can be found in the Appendices (see Appendix C). Specifically, each correct multiple-choice answer was worth 0.5 point and a correct explanation was worth 1 point, a partially correct explanation received 0.25–0.5 point, and an incorrect explanation was awarded 0 points. The scores were summed, resulting in a maximum score of 12 points on the learning items, 9 points on the near transfer items, and 9 points on the far transfer items. Unfortunately, one near transfer item had to be removed because it was inconsistent in difficulty between test moments, as the belief bias was less effective in the pretest compared to the posttest, making it relatively easier on the pretest.Footnote† As a result, a total score of 7.5 points could be gained on near transfer items. Two raters independently scored 25% of the posttest. Intra-class correlation coefficients were 0.985 for the learning test items, 0.989 for the near transfer test items, and 0.977 for the far transfer items. After the discrepancies were resolved by discussion, the remainder of the tests was scored by one rater.
To explore whether participants’ ability to recall the acquired knowledge underlies difficulties with transfer, free recall was scored, using another coding scheme (see Appendix D). Participants in the free recall condition could earn a maximum of 1 point per rule of logic correctly retrieved (in steps of 0.5), resulting in a maximum total score of 4 points on retrieved information. The two raters independently scored all free recall data. Intra-class correlation coefficients were .963 (nothing written down coded as no recall) and .998 (nothing written down coded as missing value).
Reliability (Cronbach’s alpha) of the learning items was .56 on the pretest and .75 on the posttest, reliability of the near transfer items was .51 on the pretest and .71 on the posttest, and reliability of the far transfer items was .74 on the pretest and .92 on the posttest. It was expected that participants would have very limited knowledge relative to these tasks at the outset, and therefore were unable to generate coherent explanations (and may even have had to guess), leading to low variability and low alphas at pretest. Posttest alphas are thus more indicative of the reliability of these tasks when respondents are presumed to have some knowledge or exposure to the content being assessed.
Results
In all analyses reported below, a p-value of .05 was used as a threshold for statistical significance. Partial eta-squared () is reported as a measure of effect size for all ANOVAs with
,
, and
denoting small, medium, and large effects, respectively (Cohen, Citation1988). Cohen’s d is reported as a measure of effect size for all t-tests, with values of 0.20, 0.50, and 0.80 representing small, medium, and large effects, respectively (Cohen, Citation1988). Furthermore, Cramer’s V is reported as an effect size for chi-square tests with (having 2 degrees of freedom) V = .07, V = .21, and V = .35 denoting small, medium, and large effects, respectively.
We created boxplots to identify outliers (i.e. values that fall more than 1.5 times the interquartile range above the third quartile or below the first quartile) in the data. If there were any, we first conducted the analyses on the data of all participants who completed the experiment (i.e. including outliers) and reran the analyses on the data without outliers. If outliers influenced on the results, we reported the results of both analyses. If the results were the same, we only reported the results on the full data.
Before addressing our hypotheses, preliminary analyses were conducted to assess whether the four conditions were comparable before the start of the manipulation. Results confirmed that there were no a-priori differences between the conditions in educational background, χ²(12) = 16.50, p = .17, V = .17; gender, χ²(3) = 0.41, p = .938, V = .05; age, F(3, 184) = 0.98, p = .406, ; performance on near transfer items of the pretest, F(3, 184) = 0.60, p = .616,
; time-on-task on near transfer items of the pretest, F(3, 184) = 0.33, p = .804,
; performance on far transfer items of the pretest, F(3, 184) = 0.20, p = .895,
; time-on-task on far transfer items of the pretest, F(3, 184) = 0.36, p = .782,
; performance on practice tasks, F(3, 184) = 2.30, p = .079,
; and time-on-task on practice tasks, F(3, 184) = 0.41, p = .746,
. provide Violin plots in which the full distribution per condition and test moment is visualized for each dependent variable.
Figure 2. Violin plots with the full distribution per condition and test moment (i.e. pretest and posttest) on performance on learning items (maximum total score of 12) in Experiment 1.
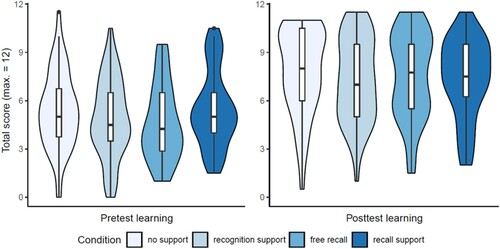
Figure 3. Violin plots with the full distribution per condition and test moment (i.e. pretest and posttest) on performance on near transfer items (maximum total score of 7.5) in Experiment 1.
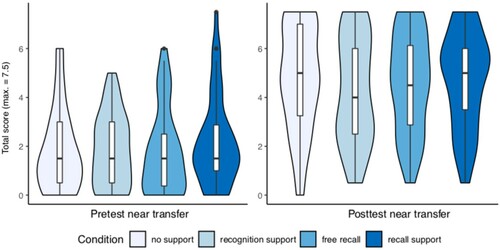
Figure 4. Violin plots with the full distribution per condition and test moment (i.e. pretest and posttest) on performance on far transfer items (maximum total score of 9) in Experiment 1.
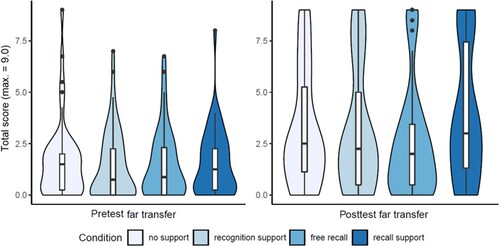
Performance on learning items
Performance scores on the pretest and posttest per condition are presented in . Correlations between performance measures are presented in . Caution is warranted in interpreting these correlations, however, because of the exploratory nature of these correlational analyses, which makes it impossible to control for the probability of type 1 errors. To test if we could replicate the finding from prior research that providing students with explicit instructions and practice activities is effective for learning to avoid biased reasoning, we conducted a paired samples t-test with Test Moment (pretest and posttest) as within-subjects factor on performance on learning items.Footnote‡ In line with previous findings, the results revealed an overall pretest (M = 5.04, SD = 2.38) to posttest (M = 7.83, SD = 2.76) performance gain on learning items, t(188) = −13.53, p < .001, d = 1.07.
Table 2. Experiment 1: mean (SD) of test performance (number of items correct) on learning (0–12), near transfer (0–7.5), and far transfer items (0–9) and mean (SD) of time-on-task (in seconds) on learning, near transfer, and far transfer items per condition.
Table 3. Experiment 1: Pearson correlation matrix (p-value) for the learning and transfer measures.
Performance on near and far transfer items
Again, performance scores on the pretest and posttest per condition are presented in . To test our main question what obstacle(s) underlie(s) the lack of transfer what has been learned to new—but related—tasks requiring CT-skills, we conducted a 2 × 4 mixed ANOVA with Test Moment (pretest and posttest) as within-subjects factor and Level of Support (no support, recognition support, free recall, and recall support) as between-subjects factor. On performance on near transfer items, this revealed a main effect of Test Moment, F(1, 184) = 261.75, p < .001, : mean performance was higher on the posttest (M = 4.56, SD = 2.07) compared to the pretest (M = 1.90, SD = 1.66). However, there was no significant main effect of Level of Support, F(3, 184) = 0.61, p = .613,
, nor an interaction between Test Moment and Level of Support, F(3, 184) = 0.66, p = .576,
.
On performance on far transfer items, results revealed a main effect of Test Moment, F(1, 184) = 77.31, p < .001, : mean performance was higher on the posttest (M = 3.18, SD = 2.97) compared to the pretest (M = 1.52, SD = 1.71). However, there was no significant main effect of Level of Support, F(3, 184) = 0.85, p = .469,
, nor an interaction between Test Moment and Level of Support, F(3, 184) = 1.74, p = .161,
.
Finally, to explore whether participants’ ability to recall the acquired knowledge underlies difficulties with transfer, we computed Pearson correlations on the data of participants within the free recall condition, between retrieved information and posttest performance on near transfer items and between retrieved information and performance on far transfer items (see and for a graphical representation of the relationship between the variables). Retrieved information was positively related to posttest performance on near transfer items, r(44) = .41, p = .005, as well as to posttest performance on far transfer items, r(44) = .34, p = .023. When nothing written down during free recall was coded as missing value instead of no recall, retrieved information was still positively related to posttest performance on near transfer performance, r(27) = .41, p = .033, but not with posttest performance on far transfer items, r(27) = .29, p = .139.
Time-on-test
We also explored differences over time and among conditions in the time spent on test items (in seconds). Descriptive statistics are provided in . A paired samples t-test with Test Moment (pretest and posttest) as within-subjects factor on time spent on learning items revealed that the mean time was lower for the posttest items (M = 52.76, SD = 21.77) than the pretest items (M = 80.76, SD = 37.43), t(187) = 11.98, p < .001, d = 0.91.Footnote§
We conducted 2 × 4 mixed ANOVAs on the time spent on transfer items with Test Moment (pretest and posttest) as within-subjects factor and Level of Support (no support, recognition support, free recall, and recall support) as between-subjects factor. On time spent on near transfer items, this revealed a main effect of Test Moment, F(1, 184) = 30.20, p < .001, : participants spent less time on average on the posttest items (M = 84.57, SD = 31.58) compared to the pretest items (M = 104.75, SD = 49.40). There was no significant main effect of Level of Support, F(3, 184) = 1.47, p = .225,
, nor an interaction between Test Moment and Level of Support, F(3, 184) = 1.64, p = .181,
.
On time spent on far transfer items, results revealed a main effect of Test Moment, F(1, 184) = 173.78, p < .001, : again, participants spent less time on average on the posttest items (M = 53.45, SD = 29.11) compared to the pretest items (M = 86.84, SD = 40.73). There was no significant main effect of Level of Support, F(3, 184) = 1.35, p = .260,
, nor an interaction between Test Moment and Level of Support, F(3, 184) = 0.49, p = .684,
.
Experiment 2
We simultaneously conducted a replication experiment in a classroom setting to assess the robustness of our findings and to increase ecological validity. The educational committee of the university approved on conducting this study within the curriculum. The design and materials were the same as that of Experiment 1.
Methods
Participants
Participants were 104 third-year “Biology and Medical Laboratory Research” and “Chemistry” students of a University of Applied Sciences. Of these, three students did not complete the complete study due to technical problems and four students did not adhere to instructions (i.e. they copied information from the CT-instructions). They were therefore excluded from the analyses and this resulted in a final sample size of 97 students (Mage = 20.39, SD = 1.67; 23 males).
Because the experiment took place in classroom setting as part of an existing course, our sample size was limited to the total number of students in this cohort. The power of our Mixed ANOVAs—under a fixed alpha level of .05, with a correlation between measures of 0.3, and with a sample size of 97—is estimated at .25, .95, and <.99 for picking up a small (), medium (
), and large (
) interaction effect, respectively. Therefore, our sample size should be sufficient to pick up medium-to-large interaction effects.
Procedure
The main difference with Experiment 1 was that Experiment 2 was run in a real education setting, namely during the first lesson of a CT-course. In the following lessons, the origins of the concept of CT, inductive and deductive reasoning, and the occurrence of biases in participants’ own work, for example, were discussed, among others. The experiment was conducted in a computer classroom at the participants’ university with an entire class of students present. Participants came from five different classes (of 17–23 participants) and were randomly distributed among the four conditions within each class. In advance of the experiment, students were informed about the experiment by their teacher. The experiment leader (first author) and the teacher of the CT-course were present during the experiment. When entering the classroom, participants were instructed to sit down at one of the desks. The experiment leader first introduced herself and provided some basic information about the experiment. Afterwards, she instructed participants to read a sheet of paper containing some general instructions and a link to the Qualtrics environment where they first signed an informed consent form. Again, participants could work at their own pace and time-on-task was logged during all phases. Furthermore, participants could use scrap paper during the practice phase and the CT-tests. Participants had to wait (in silence) until the last participant had finished the posttest before they could leave the classroom.
Data analysis
The same coding schemes were used as in Experiment 1. Again, a total score of 12 points could be earned on learning items, of 7.5 points on near transfer items, and of 9 points on far transfer items. Again, two raters independently scored all free recall data. Intra-class correlation coefficients were .987 (nothing written down coded as no recall) and .971 (nothing written down coded as missing value).
Reliability (Cronbach’s alpha) on the pretest and posttest, respectively, of the learning items were .45 and .68; of the near transfer items were .32 and .67; and of the far transfer items .77 and .89. While these low reliabilities on the pretest might again be explained by lack of prior knowledge, they are substantially lower in experiment 2 than in experiment 1, and under these circumstances, the probability of detecting a significant effect (given one exists) is low (e.g. Cleary et al., Citation1970; Rogers & Hopkins, Citation1988), and therefore, the chance that Type 2 errors may have occurred in the current study is relatively high. Therefore, we conducted alternative analyses (see Results section), as preregistered.
Two participants had two missing near transfer answers on the posttest, which were replaced by their series mean. One participant did not fill in the far transfer items of the posttest, so data for this participant were not included in the analyses involving the respective measure.
Results
Again, a p-value of .05 was used as a measure of statistical significance in all analyses reported below. Partial eta-squared () is reported as a measure of effect size for the ANOVAs for which 0.01 is considered small, 0.06 medium, and 0.14 large (Cohen, Citation1988). If outliers influenced the results, we reported the results of the analysis on the data of all participants who completed the experiment (i.e. including outliers) and the analysis on the data without outliers. If the results were the same, we only reported the results on the full data.
Preliminary analyses confirmed that there were no a-priori differences between the conditions in educational background, χ²(12) = 8.90, p = .712, V = .18; gender, χ²(6) = 3.97, p = .681, V = .14; age, F(3, 97) = 1.08, p = .361, ; performance on near transfer items of the pretest, F(3, 93) = 1.76, p = .159,
; time-on-task on near transfer items of the pretest, F(3, 93) = 0.70, p = .552,
; time-on-task on far transfer items of the pretest, F(3, 93) = 0.21, p = .888,
; performance on practice tasks, F(3, 96) = 0.39, p = .762,
; and time-on-task on practice tasks, F(3, 96) = 1.59, p = .196,
. However, the conditions differed in performance on far transfer items of the pretest, F(3, 93) = 4.17, p = .008,
. If it turns out that the conditions would differ significantly in performance gains on far transfer items, this finding should be taken into account. provide Violin plots in which the full distribution per condition and test moment is visualized for each dependent variable.Footnote**
Figure 7. Violin plots with the full distribution per condition and test moment (i.e. pretest and posttest) on performance on learning items (maximum total score of 12) in Experiment 2.
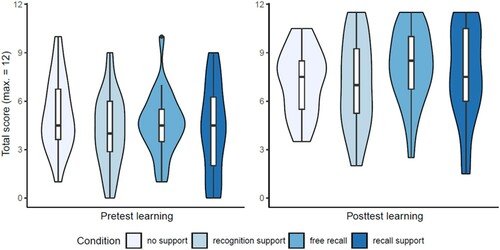
Figure 8. Violin plots with the full distribution per condition and test moment (i.e. pretest and posttest) on performance on near transfer items (maximum total score of 7.5) in Experiment 2.
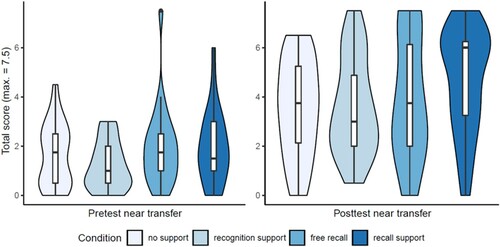
Figure 9. Violin plots with the full distribution per condition and test moment (i.e. pretest and posttest) on performance on far transfer items (maximum total score of 9) in Experiment 2.
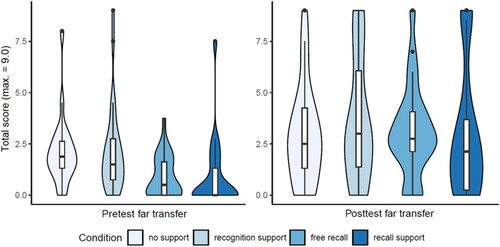
Performance on learning items
Performance scores on the pretest and posttest per condition are presented in . Correlations between performance measures are presented in . Caution is warranted in interpreting these correlations, however, because of the exploratory nature of these correlational analyses, which makes it impossible to control for the probability of type 1 errors. Because Cronbach’s Alpha on the pretest was very low, we conducted a one-sample t-test on posttest performance on learning items, in which we compared the average on the posttest of the entire sample against the reference value of the average on the pretest (M = 4.59, SD = 2.43). In line with Experiment 1, the results revealed an overall pretest to posttest (M = 7.79, SD = 2.69) performance gain on learning items, t(97) = −11.73, p < .001, d = 1.25.
Table 4. Experiment 2: mean (SD) of test performance (number of items correct) on learning (0–12), near transfer (0–7.5), and far transfer items (0–9) and mean (SD) of time-on-task (in seconds) on learning, near transfer, and far transfer items per condition.
Table 5. Experiment 2: Pearson correlation matrix (p-value) for the learning and transfer measures.
Performance on near and far transfer items
Performance scores on the pretest and posttest per condition are presented in . To test our main question what obstacle(s) underlie(s) the lack of transfer what has been learned to novel tasks requiring CT-skills, we conducted a one-way ANOVA (due to low reliability on the pretest, see preregistration where we reported what analyses would be performed if Cronbach’s Alpha on the pretest turned out to be low) with Level of Support (no support, recognition support, free recall, and recall support) as between-subjects factor on performance on near transfer items. The results revealed no significant main effect of Level of Support, F(3, 93) = 1.36, p = .259, . In addition to planned analysis, we decided to conduct a one-sample t-test on posttest performance on near transfer items, compared to the reference value of the average on the pretest (M = 1.66, SD = 1.35). The results revealed an overall pretest to posttest (M = 3.91, SD = 2.16) performance gain on near transfer items, t(96) = 10.21, p < .001, d = 1.25.
Additionally, we conducted a 2×4 Mixed ANOVA on performance on far transfer items with Test Moment (pretest and posttest) as within-subjects factor and Level of Support (no support, recognition support, free recall, and recall support) as between subjects factor.Footnote†† The results revealed a main effect of Test Moment F(1, 92) = 43.91, p < .001, : mean performance was higher on the posttest (M = 3.20, SD = 2.74) compared to the pretest (M = 1.55, SD = 1.80). However, there was no significant main effect of Level of Support, F(3, 92) = 1.39, p = .250,
, nor an interaction between Test Moment and Level of Support, F(3, 92) = 1.48, p = .226,
.
Finally, to explore whether participants’ ability to recall the acquired knowledge underlies difficulties with transfer, we computed Pearson correlations on the data of participants within the free recall condition, between retrieved information and posttest performance on far transfer items and between retrieved information and performance on near transfer items (see and for a graphical representation of the relationship between the variables). Retrieved information was not positively related to posttest performance on near transfer items, r(24) = .33, p = .114, nor with posttest performance on far transfer items, r(24) = .06, p = .787. When nothing written down during free recall was coded as missing value instead of no recall, however, retrieved information was positively related to posttest performance on near transfer performance, r(11) = .87, p = .001, but not with posttest performance on far transfer items, r(11) = .26, p = .443.
Time-on-test
We exploratory analyzed the time spent on test items (in seconds). Descriptive statistics are provided in . A Paired samples t-test with Test Moment (pretest and posttest) as within-subjects factor on time spent on learning items revealed that the mean time spent on posttest items (M = 52.76, SD = 21.77) was lower than on pretest items (M = 80.76, SD = 37.43), t(97) = 9.88, p < .001, d = 1.11.
We conducted 2 × 4 mixed ANOVAs on the time spent on transfer items with Test Moment (pretest and posttest) as within-subjects factor and Level of Support (no support, recognition support, free recall, and recall support) as between-subjects factor. On time spent on near transfer items, that revealed a main effect of Test Moment, F(1, 93) = 37.59, p < .001, : participants spent less time on the posttest items (M = 84.32, SD = 26.87) compared to the pretest items (M = 108.78, SD = 40.27). There was no significant main effect of Level of Support, F(3, 93) = 1.85, p = .143,
, nor an interaction between Test Moment and Level of Support, F(3, 93) = 2.18, p = .096,
.
On time spent on far transfer items, results revealed a main effect of Test Moment, F(1, 92) = 151.39, p < .001, : again, participants spent less time on the posttest items (M = 62.84, SD = 25.23) compared to the pretest items (M = 104.41, SD = 35.49). There was no significant main effect of Level of Support, F(3, 92) = 0.63, p = .595,
, nor an interaction between Test Moment and Level of Support, F(3, 92) = 1.21, p = .309,
.
Performance differences across study domains
As requested by a reviewer, we exploratorily analyzed whether there were differences in performance gains across the study domains. We conducted three 2×4 mixed ANOVAs on the performance measures with Test Moment (pretest and posttest) as within-subjects factor and Study Domain (Biology and Medical Laboratory research, major biomedical research; Biology and Medical Laboratory research, major forensic laboratory research; Chemistry, major forensic laboratory research) as between-subjects factor. Regarding performance on learning items, results did not reveal a main effect of Study Domain, F(2, 94) = 2.22, p = .115, , nor an interaction between Test Moment and Study Domain, F(2, 94) = 0.04, p = .964,
. Similarly, regarding performance on near transfer items, results did not reveal a main effect of Study Domain, F(2, 94) = 2.66, p = .076,
, nor an interaction between Test Moment and Study Domain, F(2, 94) = 2.71, p = .072,
. Also, regarding performance on far transfer items, results did not reveal a main effect of Study Domain, F(2, 93) = 0.44, p = .644,
, nor an interaction between Test Moment and Study Domain, F(2, 93) = 0.31, p = .735,
.
General discussion
The present study aimed to identify obstacles to transfer of CT-skills required for unbiased reasoning. Prior studies observed a lack of transfer of these CT-skills (e.g. Van Peppen et al., Citation2018, Citation2021a, Citation2021b, Citation2021c; Heijltjes et al., Citation2014a, Citation2014b, Citation2015), and we examined whether this would be due to (a) failure to recognize that the acquired knowledge is relevant to the new task, (b) inability to recall the acquired knowledge, or (c) difficulties in actually mapping that knowledge onto the new task (cf. the three-step model of transfer: Barnet & Ceci, Citation2002).
Benefits of instruction and practice
In line with our expectations and consistent with earlier research (e.g. Abrami et al., Citation2014; Heijltjes et al., Citation2014b), we found that providing students with explicit instructions and practice (during the pretest and practice phase) is associated with a performance gain in unbiased reasoning and a reduction in test-taking time in two experiments. These results further support the idea of Stanovich (Citation2011) that acquisition of relevant knowledge structures and stimulating students to engage in CT, is useful to prevent biased reasoning. As people gain expertise, they can often attain an equal/higher level of performance with less/equal time investment. As such, these findings appear to be consistent with the notion that a relatively brief instructional intervention including explicit instructions and practice opportunities is both effective and efficient for learning and transfer of CT-skills, which is promising for educational practice. However, we should stress that our research design does not permit us to draw causal conclusions about the effectiveness of the instructions-plus-practice intervention from our experiments. This is because our manipulation occurred in the test-phase. We did not include a control group with a different intervention or a no-intervention –this was not required given our central research question and the beneficial effects of this type of training have already been well-established in comparison to several control conditions (e.g. Heijltjes et al., Citation2014b).
Interestingly, our experiments suggest that these instructions and practice activities may also enhance transfer (both to similar tasks in a different format and to novel task types) to some extent: students showed better performance on posttest transfer tasks, and, again, with reduced test-taking time. As one would expect (Barnett & Ceci, Citation2002; Bray, Citation1928; Dinsmore et al., Citation2014), transfer between closely related situations occurred more often than transfer between situations that had less in common: performance gains were highest on learning items (i.e. same problem type but different story contexts), followed by near transfer items (i.e. same problem type but offered in a different/less abstract format), and thereafter far transfer items (i.e. similar principles but applied to novel problem types).
It is particularly promising that participants improved noticeably on near transfer items after a relatively short instruction and practice phase. These items consisted of belief biases in written news items or articles on topics that participants might encounter in other courses and their working life. The few studies that investigated effects of instruction/practice on transfer of CT-skills, and failed to find evidence of transfer, only examined tasks reflecting far transfer (Van Peppen et al., Citation2018; Heijltjes et al., Citation2014a, Citation2015). We even observed some increase in performance on far transfer items in the present study. Other studies did not include these items on the pretest (Van Peppen et al., Citation2021a, Citation2021b, Citation2021c) and were, therefore, not able to detect transfer gains. It could also be argued that pre-testing had some effect on the posttest scores and, moreover, masked the effect of the experimental manipulation, although this seems unlikely given that participants did not receive feedback on their performance and the posttest scores were still rather low. Thus, our findings are promising as they seem to support the idea that instruction/practice can be beneficial for near and far transfer of CT-skills. However, there was a lot of room for improvement, yet students did not seem to benefit from the support conditions, as we will discuss in the next section.
Obstacles to successful transfer of CT-skills
As for our main question regarding the obstacles to successful transfer of CT-skills, our findings suggest that participants were able to recognize that the acquired knowledge was relevant to the new task and to recall that knowledge: they did not benefit from recognition and recall support (i.e. there were no significant differences among conditions). Thus, our findings suggest that students may have had difficulties in applying the relevant knowledge on the new tasks (Hypothesis 3).
However, findings from the free recall condition do not fully support the idea that it is only an application/mapping problem. Most participants did not retrieve all relevant information and exploratory results pointed to moderate-to-large positive correlations between participants’ retrieved knowledge and their performance on near transfer (in both experiments) and far transfer (only in Experiment 1 when nothing written down was coded as no recall) items. Although exploratory analyses might lack statistical rigor, these results provide insight into further avenues to explore the relation between knowledge retrieval and transfer: this finding may suggest that suboptimal recall could also have played a role in unsuccessful transfer (Hypothesis 2b). Descriptive statistics support this idea: participants who received recall support numerically outperformed the other conditions on far transfer items at posttest in Experiment 1 and on near transfer items at posttest in Experiment 2. Because the power of our study was only sufficient to pick up medium-to-large interaction effects and it may be that providing recall support had a small effect on transfer, a further study with a more powerful design (e.g. a larger sample size) is suggested.
Interestingly, previous studies on analogical transfer (Gick & Holyoak, Citation1980, Citation1983) showed that recognition is often the barrier to transfer. Contrary to these studies, participants in the current study were aware that they received instructions on CT (in Experiment 2 even during an CT-course), which could have helped them recognize that the knowledge learned had to be transferred to the new task. Various other studies, however, revealed that students often have application problems in novel situations (i.e. inert knowledge problem, see Renkl et al., Citation1996). It seems possible that students in the current study did not know how to use the acquired knowledge in a novel situation because the knowledge was not available in a form that allows for direct application (i.e. structure deficit). Future research on instructional interventions that focuses more on the recall and application steps in the transfer process, for instance by having students repeatedly retrieving and applying knowledge to different examples (Butler et al., Citation2017; Carpenter, Citation2012) while providing explanation feedback (Butler et al., Citation2013; Van Eersel et al., Citation2016), would be of great help in establishing how to successfully promote transfer of CT-skills.
Limitations and future directions
Fruitful next steps would be to replicate our finding that the difficulty of transfer of CT-skills lies in inadequate application/mapping and to support this finding by (conceptual) replications (with other types of CT-tasks). A further study could, for instance, teach students about certain subject matter and let them consult a full solution procedure to tasks related to that subject matter (thus eliminating the need to recognize and retain knowledge) while completing tasks that vary in overlap with the subject-matter knowledge. In one condition, students complete isomorphic tasks, in another condition near transfer tasks, and in a third condition far transfer tasks. If performance decreases over these conditions, that would provide further evidence for the assumption that the difficulty of transfer lies in inadequate application/mapping. Another research question that could be addressed in qualitative studies is why students have application problems in novel situations. Do they have difficulties adapting the acquired mindware (i.e. inert knowledge problem: e.g. Renkl et al., Citation1996) or with suppressing heuristic responses to novel problems, or both?
One potential limitation of this study concerns the short training duration. While it is interesting to see that this relatively brief training already had beneficial effects on learning and near transfer, gaining deep understanding of the underlying principles of the subject matter (i.e. meaningful knowledge structures), required for far transfer, might need more extensive or longer training. Even though our results indicate that participants learned to solve abstract CT-tasks (i.e. syllogisms), their subject-matter knowledge may have been insufficient for identifying structural overlap between problems and, consequently, for solving more complex or novel CT-tasks. The challenge for researchers and educational practitioners (e.g. consultants, teachers) in the CT-domain is to develop instructional designs that contribute to actively constructing meaning from the to-be-learned information (i.e. generative processing; e.g. Fiorella & Mayer, Citation2016; Wittrock, Citation2010), which is conditional for recall and application. Ways to stimulate generative processing are, for instance, encouraging elaboration, questioning, or explanation during practice or having students repeatedly retrieve to-be learned information from memory. Although prior studies did not show beneficial effects of such instructional strategies with regard to improving transfer of CT, these were also studies with relatively short training session. Another possible direction could be to provide exemplars of knowledge application while gradually remove scaffolding (cf. four-component instructional design model; Van Merriënboer et al., Citation1992) or while fading from concrete-to-abstract situations (i.e. concreteness fading; McNeil & Fyfe, Citation2012).
Another potential limitation of the study is that one might ask if the hint provided at the end of the CT-instructions could have “washed out” condition effects. However, note that this was a very generic statement, so no replacement for the specific recognition support given during the transfer phase. Moreover, we should note that there was a practice phase in between the CT-instructions and the transfer phase.
Given that multiple studies reported rather low levels of reliability of tests consisting of heuristics-and-biases tasks (Aczel et al., Citation2015a; West et al., Citation2008) and revealed concerns with the reliability of widely used standardized CT tests, particularly with regard to subscales (Bernard et al., Citation2008; Bondy et al., Citation2001; Ku, Citation2009; Leppa, Citation1997; Liu et al., Citation2014; Loo & Thorpe, Citation1999), we aimed to increase reliability of our measures. Therefore, we included multiple items of one CT-task category to narrow down the test into a single measurable construct and, thereby, to decrease measurement error (LeBel & Paunonen, Citation2011), which resulted—except on the pretest—in quite reliable measures. However, because of this, we focused on only one, albeit highly important, aspect of CT, namely overturning belief-biased responses when evaluating the logical validity of arguments (De Chantal et al., Citation2019; Evans, Citation2003). Although this is just one aspect of CT, it should be noted that heuristics and biases tasks represent how people make judgements under uncertain or varied contexts (e.g. heuristics and biases appear in newspapers, books, courses, and applications of many kinds) and the current study thus provides valuable insight into how people think and reason. Especially since (un)biased reasoning was assessed in the context of the level of individual study domain—contrary to standardized CT-tests and most research on heuristics and biases—and could, therefore, be evaluated within authentic contexts. Hence, participants’ performance on these heuristics and biases tasks presumably offers a realistic view of everyday reasoning (see, for example, Gilovich et al., Citation2002). Relevant next steps would be to investigate effects of instruction/practice on other types of reasoning biases, for instance those involving probabilistic reasoning. In particular, since it has been shown that effective “debiasing” training methods are not always effective for avoiding all types of biases (see, for example, Aczel et al., Citation2015b); these methods may be less helpful for overcoming biases related to less abstract principles for which there is no concrete alternative strategy.
A noteworthy strength of this study was that we simultaneously conducted a replication experiment in a classroom setting to assess the robustness of our findings and to increase ecological validity. As promising interventions sometimes fail in more realistic settings (e.g. Hulleman & Cordray, Citation2009) and classroom studies aimed at fostering transfer of CT-skills are relatively rare, this study provides valuable new insights for educational practice. To wit, that transfer of CT-skills from abstract tasks to domain relevant texts and to novel task types can be established with a relatively short instruction and practice phase. However, there is still a lot of room for improvement in bringing about far transfer, and for that, obstacles such as suboptimal recall and application should be countered. Considerably more studies, preferably including direct or conceptual replications to increase robustness of findings, are needed to develop a full picture of effective ways to teach (far) transfer of CT-skills.
Conclusion
To conclude, the present study established that it is possible to foster students’ learning and transfer of CT-skills to different formats/situations and novel task types through a relatively simple intervention. Our findings suggest that difficulties in (far) transfer are mainly due to an inability to apply relevant knowledge onto novel problems and exploratory analyses point to the possibility that suboptimal recall may play a role as well. Students seemed to have no problems recognizing that the acquired knowledge was relevant to the new problem. Hence, this study suggests that instructional interventions aimed at transfer of CT-skills should focus particularly on the application and possibly also on the recall steps in the transfer process. Nevertheless, more research is needed to corroborate this conclusion and to find out why students have application problems in novel situations. As far as we know, our study was the first to systematically vary gradients of similarity between the initial CT-task and the transfer task (i.e. learning, near transfer, and far transfer) and, thus, adds to the small body of literature on whether instruction/practice can foster students’ CT. Understanding the obstacle(s) underlying (un)successful transfer is crucial to design courses to achieve it and, moreover, is relevant for theories of learning and transfer.
Acknowledgements
The authors would like to thank Ilse Hartel – Slager and Marjolein Looijen for their help with running this study, Anita Heijltjes and Eva Janssen for their input on the materials, and the members of the Disciplined Reading & Learning Research Laboratory of the University of Maryland for their input on the discussion.
Disclosure statement
No potential conflict of interest was reported by the author(s).
Data availability statement
The datasets and script files are stored on an Open Science Framework (OSF) page for this project, see osf.io/ybt5g.
Additional information
Funding
Notes
† More specifically, students’ explanations accompanying this pretest item revealed that the first premise was generally considered believable, while it was developed to seem unbelievable. Consequently, the conclusion was presumably believable to them (i.e., Valid and believable). Their explanations accompanying the equivalent posttest item revealed that they generally considered the first premise there to be unbelievable (as intended; false and unbelievable). The chance of a correct answer was, therefore, lower on the posttest than the pretest due to a belief bias. To avoid falsely showing decrease or no progress after instruction/practice on this item, we decided to exclude it from the analyses.
‡ For clarification, we did not compare the four support conditions on performance on learning items data because the manipulation took place after all learning items were completed.
§ For clarification, we did not compare the four support conditions on time spent on learning items data because the manipulation took place after all learning items were completed.
** We also conducted some exploratory analyses regarding students’ study background and the time participants spent on the CT-instructions. However, these analyses did not have much added value for this paper, and, therefore, are not reported here but provided on our OSF-page.
†† Because of severe violations of the normality assumption, we additionally conducted a Kruskal-Wallis H Test (nonparametric alternative of ANOVA); however, the results did not differ from the parametric analyses and, therefore, are not reported in this paper but provided on our OSF-page.
References
- Abrami, P. C., Bernard, R. M., Borokhovski, E., Waddington, D. I., Wade, C. A., & Persson, T. (2014). Strategies for teaching students to think critically: A meta-analysis. Review of Educational Research, 85(2), 275–314. https://doi.org/https://doi.org/10.3102/0034654314551063
- Abrami, P. C., Bernard, R. M., Borokhovski, E., Wade, A., Surkes, M. A., Tamim, R., & Zhang, D. (2008). Instructional interventions affecting critical thinking skills and dispositions: A stage 1 meta-analysis. Review of Educational Research, 78(4), 1102–1134. https://doi.org/https://doi.org/10.3102/0034654308326084
- Aczel, B., Bago, B., Szollosi, A., Foldes, A., & Lukacs, B. (2015a). Is it time for studying real-life debiasing? Evaluation of the effectiveness of an analogical intervention technique. Frontiers in Psychology, 6, 1120. https://doi.org/https://doi.org/10.3389/fpsyg.2015.01120
- Aczel, B., Bago, B., Szollosi, A., Foldes, A., & Lukacs, B. (2015b). Measuring individual differences in decision biases: Methodological considerations. Frontiers in Psychology, 6, 1770. https://doi.org/https://doi.org/10.3389/fpsyg.2015.01770
- Barnett, S. M., & Ceci, S. J. (2002). When and where do we apply what we learn?: A taxonomy for far transfer. Psychological Bulletin, 128(4), 612–637. https://doi.org/https://doi.org/10.1037/0033-2909.128.4.612
- Baron, J. (2008). Thinking and deciding (4th ed.). Cambridge University Press.
- Barreiros, J., Figueiredo, T., & Godinho, M. (2007). The contextual interference effect in applied settings. European Physical Education Review, 13(2), 195–208. https://doi.org/https://doi.org/10.1177/1356336X07076876
- Bassok, M., & Holyoak, K. J. (1989). Interdomain transfer between isomorphic topics in algebra and physics. Journal of Experimental Psychology: Learning, Memory, and Cognition, 15(1), 153. https://doi.org/https://doi.org/10.1037/0278-7393.15.1.153
- Bernard, R. M., Zhang, D., Abrami, P. C., Sicoly, F., Borokhovski, E., & Surkes, M. A. (2008). Exploring the structure of the watson–glaser critical thinking appraisal: One scale or many subscales? Thinking Skills and Creativity, 3(1), 15–22. https://doi.org/https://doi.org/10.1016/j.tsc.2007.11.001
- Bondy, K. N., Koenigseder, L. A., Ishee, J. H., & Williams, B. G. (2001). Psychometric properties of the California critical thinking tests. Journal of Nursing Measurement, 9(3), 309–328. https://doi.org/https://doi.org/10.1891/1061-3749.9.3.309
- Bray, C. W. (1928). Transfer of learning. Journal of Experimental Psychology, 11(6), 443. https://doi.org/https://doi.org/10.1037/h0071273
- Butler, A. C., Black-Maier, A. C., Raley, N. D., & Marsh, E. J. (2017). Retrieving and applying knowledge to different examples promotes transfer of learning. Journal of Experimental Psychology: Applied, 23(4), 433–446. https://doi.org/https://doi.org/10.1037/xap0000142
- Butler, A. C., Godbole, N., & Marsh, E. J. (2013). Explanation feedback is better than correct answer feedback for promoting transfer of learning. Journal of Educational Psychology, 105(2), 290–298. https://doi.org/https://doi.org/10.1037/a0031026
- Carpenter, S. K. (2012). Testing enhances the transfer of learning. Current Directions in Psychological Science, 21(5), 279–283. https://doi.org/https://doi.org/10.1177/0963721412452728
- Cleary, T. A., Linn, R. L., & Walster, G. W. (1970). Effect of reliability and validity on power of statistical tests. Sociological Methodology, 2, 130–138. https://doi.org/https://doi.org/10.1037/a0031026
- Cohen, J. (1988). Statistical power analysis for the behavioral sciences (2nd. ed., reprint). Psychology Press.
- Cormier, S. M., & Hagman, J. D. (2014). Transfer of learning: Contemporary research and applications. Academic Press.
- Davies, M. (2013). Critical thinking and the disciplines reconsidered. Higher Education Research & Development, 32(4), 529–544. https://doi.org/https://doi.org/10.1080/07294360.2012.697878
- De Chantal, P. L., Newman, I. R., Thompson, V., & Markovits, H. (2019). Who resists belief-biased inferences? The role of individual differences in reasoning strategies, working memory, and attentional focus. Memory & Cognition, 48, 655–671. https://doi.org/https://doi.org/10.3758/s13421-019-00998-2
- Dewey, J. (1910). How we think. D C Heath.
- Dinsmore, D. L., Baggetta, P., Doyle, S., & Loughlin, S. M. (2014). The role of initial learning, problem features, prior knowledge, and pattern recognition on transfer success. The Journal of Experimental Education, 82(1), 121–141. https://doi.org/https://doi.org/10.1080/00220973.2013.835299
- Druckman, D., & Bjork, R. A. (1994). Learning, remembering, believing: Enhancing human performance. National Academy Press.
- Duron, R., Limbach, B., & Waugh, W. (2006). Critical thinking framework for any discipline. International Journal of Teaching and Learning in Higher Education, 17, 160–166.
- Evans, J. S. B. (2002). Logic and human reasoning: An assessment of the deduction paradigm. Psychological Bulletin, 128(6), 978–996. https://doi.org/https://doi.org/10.1037/0033-2909.128.6.978
- Evans, J. S. B. (2003). In two minds: Dual-process accounts of reasoning. Trends in Cognitive Sciences, 7(10), 454–459. https://doi.org/https://doi.org/10.1016/j.tics.2003.08.012
- Evans, J. S. B., Barston, J. L., & Pollard, P. (1983). On the conflict between logic and belief in syllogistic reasoning. Memory & Cognition, 11(3), 295–306. https://doi.org/https://doi.org/10.3758/BF03196976
- Facione, P. A. (1990). Critical thinking: A statement of expert consensus for purposes of Educational assessment and instruction. The California Academic Press.
- Faul, F., Erdfelder, E., Buchner, A., & Lang, A. G. (2009). Statistical power analyses using G* Power 3.1: Tests for correlation and regression analyses. Behavior Research Methods, 41(4), 1149–1160. https://doi.org/https://doi.org/10.3758/BRM.41.4.1149
- Fiorella, L., & Mayer, R. E. (2016). Eight ways to promote generative learning. Educational Psychology Review, 28(4), 717–741. https://doi.org/https://doi.org/10.1007/s10648-015-9348-
- Flores, K. L., Matkin, G. S., Burbach, M. E., Quinn, C. E., & Harding, H. (2012). Deficient critical thinking skills among college graduates: Implications for leadership. Educational Philosophy and Theory, 44(2), 212–230. https://doi.org/https://doi.org/10.1111/j.1469-5812.2010.00672.x
- Gentner, D. (1983). Structure-mapping: A theoretical framework for analogy. Cognitive Science, 7(2), 155–170. https://doi.org/https://doi.org/10.1016/S0364-0213(83)80009-3
- Gentner, D. (1989). The mechanism of analogical learning. In S. Vosniadou & A. Ortony (Eds.), Similarity and analogical reasoning (pp. 199–241). Cambridge University Press. https://doi.org/https://doi.org/10.1017/CBO9780511529863
- Gick, M. L., & Holyoak, K. J. (1980). Analogical problem solving. Cognitive Psychology, 12(3), 306–355. https://doi.org/https://doi.org/10.1016/0010-0285(80)90013-4
- Gick, M. L., & Holyoak, K. J. (1983). Schema induction and analogical transfer. Cognitive Psychology, 15(1), 1–38. https://doi.org/https://doi.org/10.1016/0010-0285(83)90002-6
- Gigerenzer, G., & Hug, K. (1992). Domain-specific reasoning: Social contracts, cheating, and perspective change. Cognition, 43(2), 127–171. https://doi.org/https://doi.org/10.1016/0010-0277(92)90060-U
- Gilovich, T., Griffin, D., & Kahneman, D. (2002). Heuristics and biases: The psychology of intuitive judgment. Cambridge University Press.
- Halpern, D. F. (2014). Critical thinking across the curriculum: A brief edition of thought & knowledge. Routledge.
- Halpern, D. F., & Butler, H. A. (2019). Teaching critical thinking as if our future depends on it, because it does. In J. Dunlosky, & K. Rawson (Eds.), The Cambridge handbook of cognition and education (pp. 51–66). Cambridge University Press.
- Heijltjes, A., Van Gog, T., Leppink, J., & Paas, F. (2014). Improving critical thinking: Effects of dispositions and instructions on economics students’ reasoning skills. Learning and Instruction, 29, 31–42. https://doi.org/https://doi.org/10.1016/j.learninstruc.2013.07.003
- Heijltjes, A., Van Gog, T., Leppink, J., & Paas, F. (2015). Unraveling the effects of critical thinking instructions, practice, and self-explanation on students’ reasoning performance. Instructional Science, 43(4), 487–506. https://doi.org/https://doi.org/10.1002/acp.3025
- Heijltjes, A., Van Gog, T., & Paas, F. (2014). Improving students’ critical thinking: Empirical support for explicit instructions combined with practice. Applied Cognitive Psychology, 28(4), 518–530. https://doi.org/https://doi.org/10.1002/acp.3025
- Holland, J. H., Holyoak, K. J., Nisbett, R. E., & Thagard, P. R. (1989). Induction: Processes of inference, learning, and discovery. MIT press.
- Hulleman, C. S., & Cordray, D. S. (2009). Moving from the lab to the field: The role of fidelity and achieved relative intervention strength. Journal of Research on Educational Effectiveness, 2(1), 88–110. https://doi.org/https://doi.org/10.1080/19345740802539325
- Janssen, E. M., Mainhard, T., Buisman, R. S. M., Verkoeijen, P. P. J. L., Heijltjes, A. E. G., Van Peppen, L. M., & Van Gog, T. (2019). Training higher education teachers’ critical thinking and attitudes towards teaching It. Contemporary Educational Psychology, 58, 310–322. https://doi.org/https://doi.org/10.1016/j.cedpsych.2019.03.007
- Kassin, S. M., Dror, I. E., & Kukucka, J. (2013). The forensic confirmation bias: Problems, perspectives, and proposed solutions. Journal of Applied Research in Memory and Cognition, 2(1), 42–52. https://doi.org/https://doi.org/10.1016/j.jarmac.2013.01.001
- Kenyon, T., & Beaulac, G. (2014). Critical thinking education and debiasing. Informal Logic, 34(4), 341. https://doi.org/https://doi.org/10.22329/il.v34i4.4203
- Ku, K. Y. L. (2009). Assessing students’ critical thinking performance: Urging for measurements using multi-response format. Thinking Skills and Creativity, 4(1), 70–76. https://doi.org/https://doi.org/10.1016/j.tsc.2009.02.001
- Kuhn, D. (2005). Education for thinking. Harvard University Press.
- Lai, E. R. (2011). Critical thinking: A literature review. Pearson’s Research Reports, 6(1), 40–41.
- LeBel, E. P., & Paunonen, S. V. (2011). Sexy but often unreliable: The impact of unreliability on the replicability of experimental findings with implicit measures. Personality and Social Psycholy Bulletin, 37(4), 570–583. https://doi.org/https://doi.org/10.1177/0146167211400619
- Leppa, C. J. (1997). Standardized measures of critical thinking: Experience with the California critical thinking tests. Nurse Educator, 22(5), 29–33. https://doi.org/https://doi.org/10.1097/00006223-199709000-00012
- Liu, O. L., Frankel, L., & Roohr, K. C. (2014). Assessing critical thinking in higher education: Current state and directions for next-generation assessment. ETS Research Report Series, 2014(1), 1–23. https://doi.org/https://doi.org/10.1002/ets2.12009
- Loo, R., & Thorpe, K. (1999). A psychometric investigation of scores on the Watson-glaser critical thinking appraisal new forms. Educational and Psychological Measurement, 59(6), 995–1003. https://doi.org/https://doi.org/10.1177/00131649921970305
- Markovits, H., & Nantel, G. (1989). The belief-bias effect in the production and evaluation of logical conclusions. Memory & Cognition, 17(1), 11–17. https://doi.org/https://doi.org/10.3758/BF03199552
- Mayer, R. E., & Wittrock, M. C. (1996). Problem-solving transfer. In D. C. Berlinert, & R. C. Calfee (Eds.), Handbook of educational psychology (pp. 47–62). Macmillan.
- McDaniel, M. A. (2007). Transfer: Rediscovering a central concept. In H. L. Roediger, Y. Dudai, & S. M. Fitzpatrick (Eds.), Science of memory: Concepts (pp. 267–270). Oxford University Press.
- McNeil, N. M., & Fyfe, E. R. (2012). “Concreteness fading” promotes transfer of mathematical knowledge. Learning and Instruction, 22(6), 440–448. https://doi.org/https://doi.org/10.1016/j.learninstruc.2012.05.001
- Moxley, S. E. (1979). Schema: The variability of practice hypothesis. Journal of Motor Behavior, 11(1), 65–70. https://doi.org/https://doi.org/10.1080/00222895.1979.10735173
- Newstead, S. E., Pollard, P., Evans, J. S. B. T., & Allen, J. L. (1992). The source of belief bias effects in syllogistic reasoning. Cognition, 45(3), 257–284. https://doi.org/https://doi.org/10.1016/0010-0277(92)90019-E
- Pellegrino, J. W., & Hilton, M. L. (2012). Education for life and work: Transferable knowledge and skills for the 21st century. National Academies Press.
- Perkins, D. N., & Salomon, G. (1992). Transfer of learning. In T. Husen, & T. N. Postelwhite (Eds.), The international encyclopedia of educational (Vol. 11, 2nd ed., pp. 6452–6457). Pergamon Press.
- Reed, S. K. (1987). A structure-mapping model for word problems. Journal of Experimental Psychology: Learning, Memory, and Cognition, 13(1), 124. https://doi.org/https://doi.org/10.1037/0278-7393.13.1.124
- Renkl, A., & Eitel, A. (2019). Self-explaining: Learning about principles and their application. In J. Dunlosky, & K. Rawson (Eds.), The Cambridge handbook of cognition and education (pp. 528–549). Cambridge University Press.
- Renkl, A., Mandl, H., & Gruber, H. (1996). Inert knowledge: Analyses and remedies. Educational Psychologist, 31(2), 115–121. https://doi.org/https://doi.org/10.1207/s15326985ep3102_3
- Ritchhart, R., & Perkins, D. N. (2005). Learning to think: The challenges of teaching thinking. In K. J. Holyoak, & R. G. Morrison (Eds.), The Cambridge handbook of thinking and reasoning (pp. 775–802). Cambridge University Press.
- Rogers, W. T., & Hopkins, K. D. (1988). Power estimates in the presence of a covariate and measurement error. Educational and Psychological Measurement, 48(3), 647–656. https://doi.org/https://doi.org/10.1177/0013164488483008
- Salomon, G., & Perkins, D. N. (1989). Rocky roads to transfer: Rethinking mechanism of a neglected phenomenon. Educational Psychologist, 24(2), 113–142. https://doi.org/https://doi.org/10.1207/s15326985ep2402_1
- Stanovich, K. E. (2011). Rationality and the reflective mind. Oxford University Press.
- Stanovich, K. E., West, R. F., & Toplak, M. E. (2016). The rationality quotient: Toward a test of rational thinking. MIT Press.
- Sternberg, R. J. (2001). Why schools should teach for wisdom: The balance theory of wisdom in educational settings. Educational Psychologist, 36(4), 227–245. https://doi.org/https://doi.org/10.1207/S15326985EP3604_2
- Tiruneh, D. T., Verburgh, A., & Elen, J. (2014). Effectiveness of critical thinking instruction in higher education: A systematic review of intervention studies. Higher Education Studies, 4(1), 1–17. https://doi.org/https://doi.org/10.5539/hes.v4n1p1
- Tiruneh, D. T., Weldeslassie, A. G., Kassa, A., Tefera, Z., De Cock, M., & Elen, J. (2016). Systematic design of a learning environment for domain-specific and domain-general critical thinking skills. Educational Technology Research and Development, 64(3), 481–505. https://doi.org/https://doi.org/10.1007/s11423-015-9417-2
- Tversky, A., & Kahneman, D. (1974). Judgment under uncertainty: Heuristics and biases. Science, 185(4157), 1124–1131. https://doi.org/https://doi.org/10.1126/science.185.4157.1124
- Van Eersel, G. G., Verkoeijen, P. P., Povilenaite, M., & Rikers, R. (2016). The testing effect and far transfer: The role of exposure to key information. Frontiers in Psychology, 7, 1977. https://doi.org/https://doi.org/10.3389/fpsyg.2016.01977
- Van Gelder, T. V. (2005). Teaching critical thinking: Some lessons from cognitive science. College Teaching, 53(1), 41–48. https://doi.org/https://doi.org/10.3200/CTCH.53.1.41-48
- Van Merriënboer, J. J. G., Jelsma, O., & Paas, F. G. W. C. (1992). Training for reflective expertise: A four component instructional design model for complex cognitive skills. Educational Technology Research and Development, 40(2), 23–43. https://doi.org/https://doi.org/10.1007/bf0229704
- Van Peppen, L. M., Verkoeijen, P. P. J. L, Heijltjes, A., Janssen, E., & van Gog, T. (2021b). Repeated Retrieval Practice to Foster Students’ Critical Thinking Skills. Collabra: Psychology, 7(1). https://doi.org/http://doi.org/10.1525/collabra.28881
- Van Peppen, L. M., Verkoeijen, P. P. J. L., Heijltjes, A. E. G., Janssen, E. M., Koopmans, D., & van Gog, T. (2018). Effects of self-explaining on learning and transfer of critical thinking skills. Frontiers in Education, 3, 275. https://doi.org/https://doi.org/10.3389/feduc.2018.00100
- Van Peppen, L. M., Verkoeijen, P. P. J. L., Heijltjes, A. E. G., Janssen, E. M., & van Gog, T. (2021a). Enhancing students’ critical thinking skills: Is comparing correct and erroneous examples beneficial?Instructional Science, 78, 1102. https://doi.org/https://doi.org/10.1007/s11251-021-09559-0
- Van Peppen, L. M., Verkoeijen, P. P. J. L., Kolenbrander, S. V., Heijltjes, A. E. G., Janssen, E. M., & van Gog, T. (2021c). Learning to avoid biased reasoning: Effects of interleaved practice and worked examples. Journal of Cognitive Psychology, 33(3), 304–326. https://doi.org/https://doi.org/10.1080/20445911.2021.1890092
- Vosniadou, S., & Ortony, A. (1989). Similarity and analogical reasoning. Cambridge University Press.
- West, R. F., Toplak, M. E., & Stanovich, K. E. (2008). Heuristics and biases as measures of critical thinking: Associations with cognitive ability and thinking dispositions. Journal of Educational Psychology, 100(4), 930–941. https://doi.org/https://doi.org/10.1037/a0012842
- Wittrock, M. C. (2010). Learning as a generative process. Educational Psychologist, 45(1), 40–45. https://doi.org/https://doi.org/10.1080/00461520903433554
A
Overview of the supporting prompts.
Below, we provided an overview per condition of the supporting prompts (translated from Dutch) that participants received at the start of the posttest transfer items and with each posttest transfer item.
No support condition
–
Recognition support condition
To solve the following problems, you can use the rules of logic explained in the instructions.
Hint: To solve this task, you can use the rules of logic explained in the instructions.
Free recall condition
To solve the following problems, you can use the rules of logic explained in the instructions. Try to recall these rules and write them on the paper that you have received (Paper 2).______________________________________________________________________________________________
Hint: To solve this task, you can use the rules of logic explained in the instructions that you tried to recall beforehand. Take that paper to solve the task.
Recall support condition
To solve the following problems, you can use the rules of logic explained in the instructions. You can find these rules in the overview on the paper that you just have received.______________________________________________________________________________________________
Hint: To solve this task, you can use the rules of logic explained in the instructions. You can find these rules in the overview on the paper that you have received. Take that paper to solve the task.
B
Example items critical thinking tests.
Below, we translated an example item of each task category administered in the critical thinking tests and the correct answer with an explanation.
Learning task (syllogistic reasoning)
Malaria
Below, you will find two premises that you must assume are true. Indicate whether the conclusion follows logically from the given premises.
Premise 1. If a disease is caused by parasites, then it is an infectious disease.
Premise 2. Malaria is an infectious disease.
Conclusion. Malaria is caused by parasites.
□ Conclusion follows logically from the premises.
□ Conclusion does not follow logically from the premises.
Explain briefly why you chose this answer:
Correct answer: conclusion does not follow logically from the two premises.
Explanation: This assignment requires to not confuse logical validity of the conclusion with the believability of the conclusion, which presumably seems believable to participants due to their prior beliefs or real-world knowledge. If the first part of premise 1 (if a disease is caused by parasites) is met, the second part (then it is an infectious disease) automatically follows. The second premise states that Malaria is an infectious disease. But this does not necessarily mean that it is caused by parasites. There might be another cause.
Near transfer task (syllogistic reasoning in a vignette)
An article by the Netherlands Forensic Institute (NFI) about the essence of forensic hair analyses states:
Forensic hair analyses can provide important information in solving crimes. If the aim of forensic hair analyses is to identify the donor of the hair sample, then hair comparisons are performed. The investigator compares the hair sample that is found at the crime scene with reference samples of a suspect, victim, or person involved. In a recent investigation including forensic hair analyses, no hair comparisons are performed and, thus, the aim was not to identify the donor of the hair sample.
□ Conclusion follows logically from the premises.
□ Conclusion does not follow logically from the premises.
Explain briefly why you chose this answer:
Correct answer: conclusion follows logically from the premises.
Explanation: This assignment requires to not confuse logical validity of the conclusion with the believability of the conclusion, which presumably seems unbelievable to participants due to their prior beliefs or real-world knowledge. According to the statement in the second sentence “if the aim of forensic hair analyses is to identify the donor of the hair sample” (P) is met, then “hair comparisons are performed” (Q) automatically follows. In the last sentence it can be read that hair comparisons are not performed in a recent investigation, so Q is denied. Therefore, P is not present. Because if P had been present, Q would have always followed.
Far transfer task (Wason selection)
Bacterial strains
Below, you can see four bacterial strains. Each bacterial strain has two characteristics: (1) it contains gene X or gene Y and (2) it is resistant to antibiotics or not. Of the four bacterial strains, you only see one of the two characteristics. You will have to test the bacterial strain to find out the second characteristic.
The rule is “if the bacterial strain contains gene X, then it is resistant to antibiotics (AB)”.
Which bacterial strains do you need to test to check if the rule is correct? Choose one or more from the options, but only choose the option(s) that is/are necessary to check whether the rule is correct:
Explain briefly why you chose this answer:
Correct answer: bacterial strain gene X + bacterial strain not AB-resistant.
Explanation: This assignment requires to not only confirm the rule but also look for falsification of the rule. By testing the bacterial strain with Gene X, you can test whether the rule is violated: if it is not AB-resistant, the rule is violated. The same for testing the bacterial strain that is not AB-resistant: if it contains gene X, the rule is violated. Because if it contained gene X, then it should have been resistant to antibiotics. People who choose other options than the combination of bacterial strain gene X + bacterial strain not AB-resistant probably fail to apply logical principles, verify rules rather than to falsify them, or demonstrate matching bias by selecting options explicitly mentioned in the conditional statement.
C
Coding scheme critical thinking tests.
Below, we provided the coding scheme used to score participants’ performance on the critical thinking tests, translated from Dutch.
Multiple-choice score
Participants can earn 0.5 point for the correct multiple-choice answer.
Explanation score
Participants can earn 1 point for the correct explanation, 0.5 point for a partially correct explanation, and 0 points for an incorrect explanation.
D
Coding scheme free recall.
Below, we provided the coding scheme used to score participants’ free recall data (i.e. participants in the free recall condition only) translated from Dutch.
Participants can earn 1 point for a correct explanation per rule of logic and 0.5 point for a partially correct explanation per rule of logic. The maximum total score is 4 points.
Remarks
– If mentioned (apart from explaining the rules of logic): “if P, then Q” or “if-then statements” → 0.5 point.
– If mentioned (apart from explaining the rules of logic): “you are not allowed to turn the rule” → 0.5 point.
– No points for one of these two comments if the maximum total score of 4 points is already achieved.
– If one describes the four correct conclusions (instead of validity for a given conclusion), then only mentioning “If P, then Q” is worth 1 point instead of 0.5 as in the coding scheme. Such as: “If P, then Q”; “If not P, then Q may be present”; “If Q, then P does not have to be present”; or “If not Q, then P is also not present”.