ABSTRACT
The emergence of SARS-CoV-2, a coronavirus with suspected bat origins, highlights a critical need for heightened understanding of the mechanisms by which bats maintain potentially zoonotic viruses at the population level and transmit these pathogens across species. We review mechanistic models, which test hypotheses of the transmission dynamics that underpin viral maintenance in bat systems. A search of the literature identified only twenty-five mechanistic models of bat-virus systems published to date, derived from twenty-three original studies. Most models focused on rabies and related lyssaviruses (eleven), followed by Ebola-like filoviruses (seven), Hendra and Nipah-like henipaviruses (five), and coronaviruses (two). The vast majority of studies has modelled bat virus transmission dynamics at the population level, though a few nested within-host models of viral pathogenesis in population-level frameworks, and one study focused on purely within-host dynamics. Population-level studies described bat virus systems from every continent but Antarctica, though most were concentrated in North America and Africa; indeed, only one simulation model with no associated data was derived from an Asian bat-virus system. In fact, of the twenty-five models identified, only ten population-level models were fitted to data – emphasizing an overall dearth of empirically derived epidemiological inference in bat virus systems. Within the data fitted subset, the vast majority of models were fitted to serological data only, highlighting extensive uncertainty in our understanding of the transmission status of a wild bat. Here, we discuss similarities and differences in the approach and findings of previously published bat virus models and make recommendations for improvement in future work.
Introduction
Bats have received much attention in recent years for their roles as reservoir hosts for several, highly virulent, emerging infectious diseases of humans, including rabies and related lyssaviruses, Ebola and Marburg filoviruses, Hendra and Nipah henipaviruses, and SARS, MERS, and now SARS-CoV-2 coronaviruses [Citation1,Citation2]. At the time of this writing, the SARS-CoV-2 virus, which causes the disease COVID-19, has infected more than 27 million people [Citation3]. Though the exact phylogenetic ancestry of SARS-CoV-2 is still unknown [Citation4], the virus is believed to have originated from a common ancestor of several closely related coronaviruses, circulating in wild populations of Rhinolophus spp. horseshoe bats, in south-central China [Citation5,Citation7–9].
Predicting and preventing zoonotic emergence – or the cross-species spillover of a pathogen from a wildlife reservoir to a human host – first requires understanding transmission and infection dynamics in the reservoir population. Despite the public health impact of bat-borne zoonoses, the mechanisms by which bats maintain pathogens at the population level, including the extent to which they experience within-host infection-induced morbidity or mortality, remain poorly characterized [Citation10–12]. Understanding these mechanisms will be critical for predicting future cross-species spillover events, as well as informing strategies of possible public health intervention. Compartmental models offer an essential tool for elucidating the mechanisms underpinning reservoir bat transmission, as they can be applied to longitudinally collected field data from bat systems to test transmission hypotheses.
Mechanistic compartmental models of pathogen transmission originated in 1915 when Sir Ronald Ross first described the mathematical relationship between mosquito biting and human malaria incidence [Citation13]. This early Ross model classed humans into infected or uninfected ‘compartments’, or categories, using a system of differential equations to track movements between them [Citation14]. Later adapted this malaria model to construct a generalized framework of pathogen transmission in a population, known as the ‘SIR Model’, in which individuals move between susceptible (‘S’), infected (‘I’), and recovered (‘R’) compartments. Distinct from statistical models – which demonstrate associations between variables of interest – mechanistic models describe processes underlying observations through time, as defined by the mathematical relationships between compartments. The compartmental model framework has allowed researchers to move beyond statistical correlations to generate and compare hypotheses regarding the infection and transmission dynamics underlying observed patterns in pathogen incidence within a population [Citation15].
The classic SIR model has been adapted to describe pathogen transmission in many different human and wildlife systems. For instance, stochastic compartmental models once used to predict yearly influenza epidemics are now being used to capture the transmission patterns of SARS-CoV-2 in human populations across the globe [Citation16,Citation17]. Mechanistic elucidation of viral transmission in bats has not been pursued with the same energy. Given that bats represent one of the most important source taxa for emerging zoonotic infections [Citation18–20], mechanistic models of viral dynamics in bat reservoirs will play a vital role in efforts to predict or prevent the next spillover [Citation21]. In this review, we identified and analyzed twenty-five mechanistic models elucidating the dynamics of viruses circulating in bat reservoir systems. We catalog model characteristics to compare the advantages and disadvantages of various modeling approaches, as well as identify gaps in the existing research on potentially zoonotic infections in bat hosts. We collate previous findings and highlight priorities for future research with the goal of advancing modeling efforts to elucidate within- and between-host dynamics of potentially zoonotic viruses in bat reservoirs – a critical step toward developing frameworks for predicting and preventing spillover to humans.
Materials and methods
Surveying the literature
We surveyed the literature for previous modeling studies of virus dynamics in bat reservoir hosts, querying both Web of Science and Google Scholar with the following search terms: ‘bat virus model’, ‘bat virus transmission’, ‘bat virus dynamics’, and ‘bat virus mechanism’. We examined all results returned by Web of Science and the first fifty pages of results returned by Google Scholar and collected information on any study which reported a mechanistic model of virus transmission or infection dynamics in a bat reservoir host. We list all models of unique virus-host associations recovered from this search in , along with information on author, journal, publication year, broad virus taxonomic group (family or genus), virus species, bat host, study site, scale of model, and approach to data.
Table 1. All published mechanistic models of virus dynamics in bat systems (2007–2020)
From these studies, we further identified a subset of eight publications that fitted compartmental models to field data from ten distinct host-virus systems with the aim of elucidating mechanisms of virus persistence in bat reservoir hosts at the population level (). From this data subset, we further collected information on the compartmental model structures tested, the modeling approach (continuous vs. discrete, deterministic vs. stochastic, frequency- vs. density-dependent transmission), the study design of the associated data, the method of model fitting, and the resulting conclusions – including estimation of the basic pathogen reproduction number (R0) for the virus. R0 describes the number of new infections generated by one existing infection in a completely naïve host population and is a key parameter in understanding a pathogen’s intrinsic probability of persistence and transmissibility – including transmissibility across species [Citation21]. For two continuous time fitted models of host-virus associations from our subset which did not report R0, we calculated R0 from the system of equations and best-fit parameters reported in the corresponding article using a Next Generation Matrix (NGM) approach [Citation22–24] (Supplementary Appendix 1 and 2). For two discrete time fitted models from our subset that did not report R0 [both from Citation25], we calculated R0 following a discrete time approximation of the NGM approach, again using best-fit parameters reported in the corresponding article [Citation24,Citation26] (Supplementary Appendix 3). All maps and summary figures were generated using R v. 4.0.0 for Macintosh.
Table 2. Summary of data-fitted models investigating virus transmission dynamics
Results
Broad patterns across the literature
Our literature search identified twenty-three publications (published between 2007 and 2020) which presented results from mechanistic models applied to twenty-five distinct bat-virus systems [two of the studies – [Citation25,Citation27] – applied the same modeling framework to two different bat-virus associations, using data from the same field study] (). All models were focused in one discrete study system, the geographic locality of which ranged across every continent except Antarctica, and centered on a few particular species of bat host, which spanned both the major Yangochiropteran and Yinpteropchiropteran suborders of the bat clade (). Each model was applied to one of the four major bat virus families/genera of interest, with the majority (eleven) focused on lyssaviruses (particularly rabies), followed by filoviruses (seven), henipaviruses (five), and, finally, coronaviruses (two).
Figure 1. Distribution of bat virus modeling studies to date.
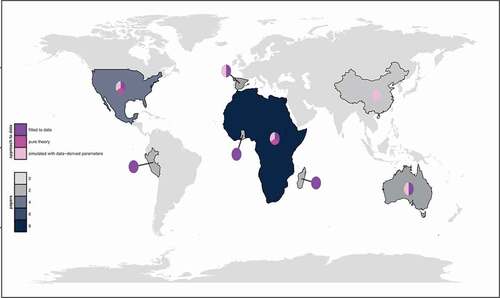
The frequency of these studies increased across the timespan captured in our dataset, suggesting that research into the mechanisms underpinning bat virus dynamics is on the rise (). While early studies tended to focus on the dynamics of lyssaviruses in North and South American systems, more recent work shows an emphasis on studies conducted in Africa, often with a focus on filoviruses (,)). Of the twenty-five systems modeled, twenty-one were focused at the population-level, while four described the within-host dynamics of viral pathogenesis in a bat immune system (). Among the within-host studies, three of the four embedded the within-host model into a population-level framework to explore how within-host metrics, such as viral load or antibody titer, translated to population-level processes, such as transmission and mortality [Citation28–30]. The fourth model kept the analysis focused at the level of within-host viral dynamics [Citation31].
Figure 2. Trends in mechanistic modeling of bat-virus systems.
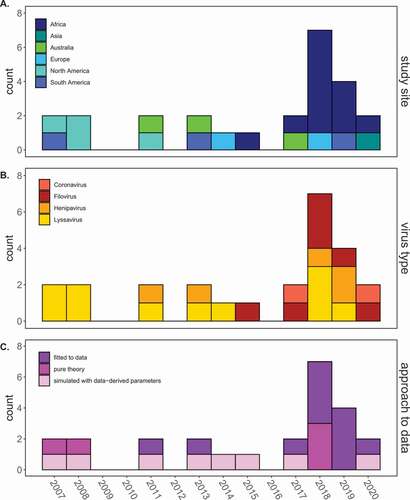
Across the entire dataset, thirteen of twenty-five models were purely theoretical or based on simulations using data-derived parameters from previous publications or analysis of field data external from the model construction [Citation32,Citation33]. The remaining twelve models were explicitly fitted to data, using a variety of statistical techniques. Notably, we observed that data-fitted models have increased in frequency in recent years (), suggesting that these studies represent a new research focus. Of these fitted models, one [Citation31] was a within-host model fitted to in vitro data in bat cell tissue culture, while the other eleven were fitted to field data. One of the field-based models [Citation34] was used to evaluate the efficacy of a proposed intervention (a transferable, orotopical rabies vaccine), while the remaining ten all aimed to identify the transmission dynamics underlying pathogen persistence in various bat systems – with a particular focus on four zoonotic bat virus taxa of interest: lyssaviruses, filoviruses, henipaviruses, or coronaviruses (). We summarize modeling insights across this taxa in the following sections.
Lyssaviruses
Lyssaviruses are non-segmented, single-stranded, negative-sense RNA viruses in the family Rhabdoviridae, order Mononegavirales [Citation35]. Lyssaviruses can be classed into three distinct phylogroups, which all show a strong association with bats [Citation36]. Lyssaviruses in phylogroup I include rabies virus (RABV), one of the oldest known viral zoonoses that accounts for over 60,000 human deaths per year [Citation37]. Though most RABV infections in humans are sourced from domestic dog populations [Citation37], the virus also persists in wild, Yangochiropteran bats across the New World, and bat-sourced infections account for the majority of RABV cases annually in the United States [Citation38]. Outside of the Americas, both Yinpterochiropteran and Yangochiropteran bats maintain a suite of diverse lyssaviruses; besides RABV, several other phylogroup 1 lyssaviruses with bat origins are known to infect humans, including Australian bat lyssavirus (ABLV), European bat lyssavirus (EBLV), and Duvenhage lyssavirus (DDUV) [Citation36]. Though all lyssaviruses are presumed to have some zoonotic capacity [Citation39], bat-borne lyssaviruses in phylogroup 2 (i.e. Lagos Bat Virus, LBV) or phylogroup 3 (i.e. Leida bat lyssavirus, LLBV) have not yet been identified infecting humans.
In our review, we identified eleven models describing the dynamics of bat-borne lyssaviruses, the majority of which (six) were focused on RABV infections in Yangochiropteran bats in the New World, while three others explored EBLV-1 dynamics in European Yangochiropterans, and two others investigated LBV dynamics in Yinpterochiropterans in Africa (). Of the RABV studies, three hailed from the same research group [Citation28–30], which used theoretical, within-host models to explain how heterogeneity in individual-level immune responses could promote population-level maintenance of rabies virus in New World Yangochiropteran bats. The authors found that models allowing for disparate dynamical outcomes based on the viral load of an initial infection were critical to obtaining population-level viral persistence; in particular, models allowing low-dosed bats to clear infections and progress to a state of temporary immunity prior to a return to susceptible status best supported viral maintenance within the population.
In addition to these within-host theoretical simulations, we identified three compartmental models of RABV infections in bat populations, all of which were fitted to field data to explore the dynamics underpinning RABV persistence in North American Eptesicus fuscus [Citation40] or Peruvian Desmodus rotundus [Citation41], or to assess the efficacy of communicable vaccines for RABV elimination in Peruvian D. rotundus [Citation34]. Like the within-host models, the population-level models demonstrated an important role for heterogeneous immune responses in rabies virus maintenance: [Citation40] fit season-specific susceptible-exposed-infectious-recovered SE(I)R models – in which exposed bats can either become infectious and die or recover and acquire lifelong immunity – to a five-year mark-recapture time-series tracking serological status of wild, Eptesicus fuscus in Colorado and demonstrated support for elevated mortality rates in infected individuals. Importantly, the authors determined that viral persistence was only possible in this population when models allowed for reduced mortality rates, and long viral incubation times across the winter hibernation period that ‘preserved’ infections until the summer birth pulse replenished the pool of susceptibles. Our R0 calculations from this study highlight the intense seasonal variation in the probability of viral persistence: we estimated R0 at 64.8 during the summer transmission period immediately following the peak birth pulse and at zero during winter hibernation and early summer transmission seasons (; Supplementary Appendix 1).
Like [Citation40, Citation41] modeled two outcomes of viral exposure when fitting models to field data on vampire bat rabies in Peru – one resulting in seroconversion without progression to an infectious state and a second by which exposure resulted in infectious rabies disease and eventual virus-induced mortality. However, since D. rotundus do not hibernate, they were unable to adopt the same seasonal variation in mortality rates and the long incubation periods that supported rabies persistence in the Colorado study. Instead, bats that seroconverted without progression to a diseased state acquired temporary – as opposed to lifelong – immunity with eventual return to a susceptible state (). Even still, [Citation41 reported a range of site-specific R0 values <1 for their system (), suggesting that the virus cannot be maintained at the population level in the absence of immigration within a spatially-structured metapopulation. Finally, in the last mechanistic model of RABV dynamics identified in our system, [Citation34], built on the model published by [Citation41], to investigate the efficacy of communicable, orotopical rabies vaccines for reducing rabies virus outbreaks in Peruvian D. rotundus bats. The authors found that realistic levels of vaccine uptake and transfer would be likely to reduce the probability, size, and duration of an outbreak. In particular, they highlighted advantages of immunization over bat culling as a strategy for reducing transmission – unlike vaccination, culling may increase recruitment of susceptible juveniles into the system, making the intervention ineffective or counterproductive [Citation34,Citation42].
Figure 3. Universal compartmental model structure for models fitted to data.
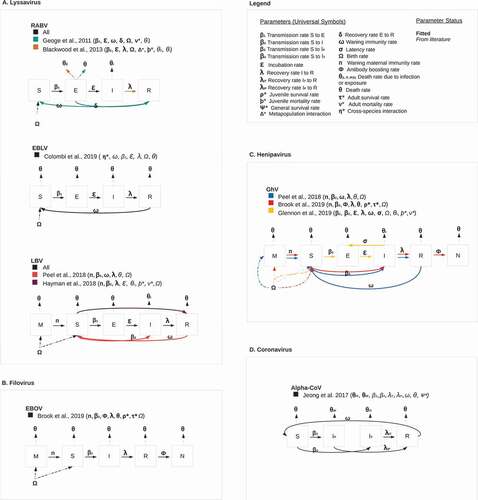
In addition to RABV, our review of the literature recovered three mechanistic models of the dynamics of EBLV-1 infection in European Yangochiropterans. [Citation32] estimated R0 (reported at 1.706) and the average duration of infection from a twelve-year longitudinal serological study of EBLV-1 dynamics in Myotis myotis bats in Spain, and then, using these estimates combined with parameters gleaned from the literature, simulated viral dynamics in a standard susceptible-infectious-recovered (SIR) framework (). Though data-inspired, the authors did not explicitly fit their transmission model to their data, making it impossible to assess the efficacy of this transmission structure in maintaining the virus in the Spain field system. [Citation33] took a similar approach in modeling the dynamics of EBLV-1 infection and cross-species transmission among three Yangochiropteran (Myotis capaccinii, Myotis myotis, Miniopterus schreibersii) and one Yinpterochiropteran [Rhinolophus ferrumequinum) bat species in a three-site metapopulation in Mallorca, Spain. The authors simulated viral persistence and extinction under a suite of different R0 values in a multi-species, metapopulation SEIRS framework and reported a heightened role for M. schreibersii in sourcing cross-species transmissions in the system – largely as a result of the high population density and rapid migration rates used for this species in the simulations. As with [Citation32], the lack of validation of the [Citation33] model against field-derived data made it impossible to assess its validity. [Citation43] compared simulations of spatially explicit, multi-species metapopulation models of EBLV-1 transmission in Miniopterus schreibersii and Myotis myotis with and without virus-induced mortality. The authors found support for an SEIRS model structure which assumed – as previously modeled by [Citation32,Citation33] – no virus-induced mortality in bats infected with EBLV-1 (). However, in contrast to the purely simulation-focused studies discussed above, [Citation43] then fit this SEIRS framework to data from a four-year field survey tracking EBLV-1 seroprevalence in Miniopterus schreibersii and Myotis myotis across two sites in Catalonia. They reported a range of R0 estimates across their field system (from 0.53 to 1.6) and emphasized the need for cross-species mixing and spatial metapopulation structure in maintaining the virus in their system – with a pronounced role for M. schreibersii in sourcing infections across the metapopulation due to its migratory behavior and high population density in the system. Despite fitting their SEIRS model to data, however, [Citation43] did not explicitly compare fits of alternative transmission models allowing for infection-induced mortality. Thus, due to the absence of comparative hypotheses, we cannot be confident that EBLV-1 does not result in infection-induced mortality in bat hosts. Indeed, experimental infection with EBLV-1 in bats can cause mortality [Citation44, Citation45], and pathological effects of infection have been posited in wild bat systems, as well [Citation46].
Finally, the last two lyssavirus models uncovered in our search aimed to decipher the mechanisms underlying LBV persistence in Yinpterochiropteran bats, specifically Eidolon helvum bats on the African continent. Both [Citation27,Citation47] fit their models to field-derived data for E. helvum, the former using a cross-sectional serological dataset spanning six African countries and the latter using a longitudinal serological time series spanning four years of ~quarterly sampling (). [Citation27] fit a maternally immune-susceptible-immune-recovered-susceptible (MSIRS) model to age-seroprevalence data, assuming the I class to correspond to seropositive status with no virus-induced mortality. They estimated an R0 value of 1.6 for LBV in the pan-African E. helvum population and a duration of immunity of twelve years, which they posited to be lifelong. Deviating from [Citation27,Citation47] found support for LBV-induced mortality and lifelong immunity, with long incubation periods and low pathogen-induced mortality promoting low population-level persistence. Specifically, the authors fit an age-structured MSE[I]R model to longitudinal serological data grouped into broad (juvenile-adult) age classes. Like [Citation40,Citation47] model allowed for exposed bats to either seroconvert immediately and acquire lifelong immunity or progress to an infectious state that resulted in virus-induced mortality. [Citation47] did not report a value for R0 in their system, but we estimated it here to be between 0 and 1.90, depending on the seasonality of the birth rate (; Supplementary Appendix 2). In contrast to studies of RABV and EBLV-1 in wild bat hosts, LBV appears to be easily maintained – albeit at low levels – by the cross-Africa, panmictic E. helvum system [Citation47]. Neither [Citation27], nor [Citation47] undertook any model comparison of their respective transmission structures, however, making it difficult to ascertain whether LBV more closes mimics the dynamics of RABV, with clear evidence of infection-induced host mortality, or EBLV-1, for which virus-induced mortality may be less widely reported.
Filoviruses
Like lyssaviruses, viruses of the family Filoviridae (filoviruses) are non-segmented, single-stranded, negative-sense RNA viruses in the order Mononegavirales [Citation35]. Filoviruses include the infamous genera Marburgvirus and Ebolavirus, as well as the lesser known Cuevavirus and Dianlovirus (currently represented by only one species each hosted in wild bats) and Striavirus and Thamnovirus (thus far only described in fish) [Citation79, Citation80]. To date, of the more prominent genera, two species of Marburgvirus and six species of Ebolavirus have been described globally [Citation48], including several which cause case fatality rates ranging from 50% to 90% upon zoonotic emergence into human hosts [Citation49]. Both Marburgviruses and Ebolaviruses are believed to be maintained in bat reservoirs, though to date, only the Marburgviruses have been isolated from wild bat hosts [Citation10,Citation50,Citation51]; nonetheless, compelling serological and PCR-based evidence suggests that bats are also reservoirs for the Ebolaviruses [Citation52]. Since 1967, Marburg virus and Ebola virus have caused over a dozen human outbreaks resulting from independent spillover events, most of which have been concentrated in west or central Africa [Citation10,Citation53].
In our review, we identified seven mechanistic, compartmental models focused on elucidating the dynamics of filoviruses in bat hosts (). One of these studies [Citation31] presented a within-host model that explored the consequences of unique bat immune responses on within-host viral dynamics, using models fit to in vitro data derived from pseudotype filovirus infections in bat cell tissue culture. The remaining six were focused at the population-level (). Of these six, four presented theoretical simulations of the reservoir dynamics underpinning the cross-species emergence of an unspecified Ebolavirus in a hypothetical African bat system [Citation54–57]; results were largely too abstract to effectively advance efforts to understand the dynamics or persistence of filoviruses in wild bat hosts. By contrast, one other theoretical model, [Citation58], used literature-derived parameter estimates for Zaire ebolavirus and Marburg marburgvirus infections in African fruit bats to investigate the probability of pathogen persistence in a panmictic host population, assuming a simple, age-structured SEIR framework. [Citation58] concluded that, given published estimates from the literature for filovirus incubation and infectious periods, these viruses would be unlikely to persist in large, well-mixed bat host populations if host births were synchronous and occurred only once a year. Results suggested that biannual birth pulses in wild, fruit bat hosts might be needed to explain filovirus persistence at the population level. However, conclusions of this research effort rested completely on the assumption that SEIR dynamics were applicable to bat filovirus systems – an assumption with little empirical basis. As such, it is impossible to evaluate the accuracy of the study’s conclusions.
In contrast to the lyssavirus studies, only one of the filovirus models identified in our review [Citation25] fit a compartmental model to field data (). The authors fit a suite of discrete time matrix models to an eighteen-month time series of age-structured filovirus serology data in Pteropus rufus fruit bats in Madagascar, aiming to elucidate the mechanistic underpinnings of population-level filovirus persistence in this field system. They identified a best fit ‘MSIRN’ model structure, whereby bats progressed from maternally immune to susceptible, infectious, recovered, and finally, a non-antibody-mediated immune class. Since these authors represented seropositive individuals in the recovered class, this ‘non-antibody mediated immune’ class allowed them to model individuals who waned in antibody seropositivity but maintained lifelong immunity via some other immunological means, as has been suggested in the experimental literature for bat-borne filoviruses [Citation59,Citation60]. The authors reported a low force of infection and infectious class prevalence which, consistent with the findings of [Citation58], suggested filovirus persistence to be unlikely in the population without some role for metapopulation structure or alternative pathway of viral pathogenesis, such as those explored for lyssavirus infections above. To elaborate on this finding, we refit a simplified, three age class version of the authors’ twenty age class matrix model to the original data, from which we estimated a range of R0 values (depending on the seasonality of the birth pulse) between 0 and 0.742 in this system – thus further supporting the need for spatial structure and/or unique within-host dynamics to explain bat filovirus maintenance. Notably, all models compared in [Citation25] were fit to serological data for a bat-borne filovirus that has yet to be described genetically.
Henipaviruses
Henipavirus is a genus within the family Paramyxoviridae, which includes (yet again) non-segmented, single-stranded, negative-sense RNA viruses [Citation61]. To date, only five species of henipavirus have been described globally, four of which find their reservoir hosts in Yinpterochiropteran fruit bats of the Pteropodidae family: Hendra (HeV) [Citation62], Nipah (NiV) [Citation63], Cedar (CedV) [Citation64], and Ghanaian henipavirus (GhV) [Citation65]. By contrast, the fifth described henipavirus, Mojiang (MojV), is derived from rats in China [Citation66]. HeV and NiV are known zoonoses that cause severe respiratory disease in humans [Citation67], CedV is thought to infect only bats [Citation64], and the host ranges for GhV and MojV have yet to be well-elucidated – though serological evidence suggestive of GhV spillover to humans has been demonstrated in Cameroon [Citation68], and MojV was first isolated in concert with suspected human fatalities to pneumonia [Citation66].
In our review, we identified five studies focused on modeling the dynamics of henipaviruses in bat reservoirs. Two of these studies, [Citation69,Citation70], presented theoretical, simulation-based models of the dynamics of Hendra virus in Australian fruit bat populations, using parameters derived from the literature. [Citation69] developed a simple SEIR model within a metapopulation framework to explore the impact of population-level connectivity on infection dynamics, with the aim of explaining the spatial-temporal dynamics of Hendra virus spillover events in eastern Australia. The authors concluded that anthropogenic fragmentation of habitat and urban habituation reduced fruit bat migration, disaggregating bat metapopulations and decreasing population-level immunity to promote less frequent but more intense viral epidemics. Since the model was not fit to data, it is not possible to evaluate its effectiveness in recovering patterns observed in the field. Using a similar approach, [Citation70] simulated an individual-based MSEIR model of Hendra virus dynamics to explore the impacts of viral latency and recrudescent infection in ‘recovered’ individuals on virus persistence at the population level. Unsurprisingly, the authors found that faster rates of recrudescence corresponded to an increased probability of virus persistence. As with the [Citation69] model, however, the [Citation70] model was purely simulation-based, making it impossible to assess the validity of viral recrudescence as a mechanism of virus persistence.
The remaining three henipavirus models all fit mechanistic, compartmental transmission models to population-level henipavirus data in an effort to explain virus persistence (). Two of the studies, [Citation27,Citation71], investigated the persistence of Ghanaian henipavirus (GhV) in Eidolon helvum bats, using, respectively, pan-African cross-sectional, age-structured serological data and a nine-year serological time series from a captive colony in Ghana. The third study, [Citation25], fits a suite of discrete time compartmental matrix models to an eighteen-month age-structured serological time series tracking infection of an undescribed African henipavirus species in Eidolon dupreanum bats in Madagascar. Both [Citation25,Citation27] fit their henipavirus models to data from the same field system that they used to model the dynamics of other bat infections – respectively, LBV for [Citation27], and an undescribed filovirus for [Citation25] (though the latter found henipaviruses and filoviruses to be hosted by different species within their dataset]. Both author groups reported that best fit model structures were consistent across pathogen systems – the same modeling frameworks used to explain persistence for LBV and filoviruses also effectively recapitulated the dynamics of henipaviruses in these systems ( and ). In Australia, the dynamics of several disparate bat paramyxoviruses have been shown to exhibit synchronicity in space and time [Citation72], suggesting that mechanistic models of bat-virus interactions may be applicable across multiple bat hosts and viruses – though more pronounced differences in bat and virus systems may be more difficult to generalize. Nonetheless, both [Citation25,Citation27] demonstrated support for similar mechanisms of waning antibody-mediated immunity post-initial seroconversion as explanations for patterns observed in their henipavirus data. [Citation27] fit data with an MSIRS model, identifying an important role for return to susceptible status and eventual reinfection in promoting viral persistence in a stochastic framework. [Citation25] did not explicitly investigate viral persistence in their system but rather focused on recovering the mechanisms driving age-seroprevalence patterns in the data. The authors found the strongest support for a model form [MSIRN) which allowed bats to wane from seropositive to seronegative status but nonetheless maintain lifelong non-antibody-mediated immunity to reinfection. The authors noted, however, that troughs in the proportion infected in between annual birth pulses would be difficult to maintain if stochastic fadeout had been permitted in their model. Thus, both [Citation25,Citation27] concluded that henipavirus dynamics would be difficult to explain without some role for viral recrudescence or loss of immunity and reinfection to preserve transmission in the absence of an influx of susceptible births across the year. Notably, however, [Citation27] assumed seropositivity to correspond to infectious epidemic status [the I class], reporting an R0 value of 2.13 for E. helvum henipavirus across Africa, while [Citation25] modeled seropositivity as an indicator of antibody-mediated immunity [the R class]. As with the Madagascar filovirus, we here refit a simplified three age class version of the [Citation25] model to the original data to allow for estimation of R0 in this system [Supplementary Appendix 3]. We calculated a seasonal R0 that ranged between 0 and 0.585 for this system and was unable to support viral persistence.
Finally, [Citation71] recovered similar results to [Citation25,Citation27], through fitting models to captive colony data for E. helvum. The authors compared all biologically possible combinations of compartments within an SEIR framework and ultimately, found the strongest support for models that permitted waning immunity post-seroconversion. As commonly demonstrated by lyssavirus models, the authors recovered considerable support for multiple pathways of viral pathogenesis in different individuals, with some bats permitted to return immediately to susceptibility following initial exposure (SES) while others oscillated between infectious (I) and latent (E) stages in cycles of viral recrudescence. The use of captive colony data makes this system somewhat less comparable to the population-level studies discussed above, though the authors benefitted from the opportunity to fit their model to repeatedly resampled individuals across the time series. Notably, [Citation71] fit models to captive colony data under varied assumptions of the epidemic phenotype indicated by seropositivity – recomputing analyses under assumptions of seropositivity corresponding to exposed, infectious, and recovered states (EIR+), as well as under assumptions of seropositivity corresponding to a recovered state only (R+). The resulting dynamics were largely comparable under both EIR+ and R+ assumptions, though modeling of seropositivity corresponding to only recovered status required an extremely high estimate for R0 (66.7 under R+ assumptions vs. 2 under EIR+ assumptions) to recover the high population-level seroprevalence witnessed in the data. Across all fitted models, authors universally modeled a standard mortality rate across all epidemic classes, though [Citation25] hypothesized in their discussion that elevated henipavirus-induced mortality could drive the observed decline in seroprevalence at late age classes witnessed in their dataset.
Coronaviruses
Only two of the twenty-five bat virus models identified in our review concerned bat infections with coronaviruses, the viral family that includes SARS-CoV-2, the causative agent in COVID-19 (). Distinct from the three bat virus taxa highlighted above, coronaviruses are positive sense, single-stranded RNA viruses, which can be classed into four genera – Alphacoronavirus and Betacoronavirus, which originate in bats, as well as Gammacoronavirus and Deltacoronavirus, which originate in birds [Citation73]. A combination of factors, including large genomes, positive sense genetic material, and a high propensity for viral recombination makes coronaviruses particularly prone to cross-species emergence [Citation74] – including zoonotic emergence into human hosts. Prior to the emergence of SARS-CoV-2, six zoonotic coronaviruses have been previously described, four of which (HCoV-299E, HCoV-NL63, SARS-CoV, and MERS-CoV) are believed to be originally derived from bats [Citation75]. Bats host a staggering diversity of Alphacoronavirus and Betacoronavirus lineages [Citation76]; however, there is a notable lack of modeling and field studies targeted toward understanding the circulation of these viruses in their wild reservoirs.
Of the two coronavirus studies recovered in our review, one [Citation77] was primarily a human model aimed at simulating conditions favoring SARS-CoV-2 spillover from bats to intermediate hosts to humans, in which the bat dynamics were delineated but not explicitly modeled. The other study, [Citation78], fit compartmental models to a ten-week mark-recapture field dataset of PCR-detections of a novel Alphacoronavirus in Australian Myotis macropus bats, comparing the classic SIRS framework with a derivative that allowed bats to become either persistently or transiently infected (). The authors found support for the model with two infectious classes that permitted some individuals to remain infectious for a more protracted (‘persistent’) period, and estimated a best fit R0 value of 1.5903 for the system. Consistent with models of filovirus and henipavirus infections in bats, the authors found no support for elevated mortality in infectious individuals, though the dataset from which inferences were made was notably small.
Discussion
Interest in understanding the dynamics of potentially zoonotic viruses in their wild bat reservoirs has increased across the past decade, and the trend in research effort over time reflects this (). In this review, we identified twenty-five mechanistic, compartmental models published in twenty-three peer-reviewed publications that investigated the dynamics of viruses in bat hosts (). Models were focused on four key bat virus taxa of known zoonotic potential [Citation20] – lyssaviruses, filoviruses, henipaviruses, and coronaviruses – with lyssaviruses (in particular rabies, RABV) most represented, followed by filoviruses and henipaviruses, and very little research effort devoted to coronaviruses. A particularly informative subset of ten population-level models were explicitly fitted to field-derived data from different bat virus systems – with the aim of elucidating the viral dynamics underpinning persistence in each system (). We here summarize findings regarding the persistence mechanisms inferred for each of these four viral clades.
In general, the bat virus modeling literature largely agrees that rabies is maintained in wild bat hosts by heterogeneous outcomes following virus exposure, where some bats die while others seroconvert – though the extent to which bats are able to recover from a clinically infectious state is debated [Citation40,Citation41]. Consistent with these findings, protective immunity from repeated RABV exposure has also been demonstrated experimentally [Citation81]. In addition to immunity, host population dynamics driven by hibernation in temperate regions and spatial structuring in the tropics also appear to play a critical role in RABV maintenance at the population level [Citation40,Citation41]. Given that these results vary across systems, however, the lack of rabies modeling in migratory bats from temperate regions or more solitary species globally, points to gaps in our complete understanding of the generalizable dynamics of the viral genus as a whole. Broadly, the dynamics of EBLV-1, another phylogroup 1 lyssavirus [Citation43], and LBV, a phylogroup-2 lyssavirus [Citation27,Citation47], appear to largely mimic those of RABV, though consensus on the mortality effects of infection with non-RABV lyssaviruses has yet to be achieved. Previous studies supported mechanisms by which at least a subset of bats recover from non-RABV lyssavirus infection to obtain immunity – especially in the case of LBV, for which protective antibodies may persist for life [Citation27,Citation47].
In contrast with lyssaviruses, data-fitted models of filovirus dynamics in bats remain rare. Our review recovered only one such example (), likely reflecting the known difficulty in identifying infections with these viruses in wild bat hosts [Citation25]. The one data-fitted filovirus model identified in our review demonstrated support for filovirus-recovered bats waning in antibody signature but maintaining lifelong immunity via some other immunological means, consistent with results from the experimental laboratory infection literature [Citation25,Citation59,Citation60]. The authors of this study suggested a declining seroprevalence in older age bats, which could reflect heightened mortality in infected individuals, though further research will be needed to elaborate these trends in other systems.
Following lyssaviruses, understanding of henipavirus dynamics in bat reservoirs shows the most promise to date – in part a reflection of the feasibility of noninvasive viral surveillance through under-roost urine collection in these systems [Citation82]. Our analysis identified only five studies focused on bat-henipaviruses, but three of these five studies presented mechanistic models fitted to field-derived data [Citation25,Citation27,Citation71]. All three studies reported that waning antibodies post-seroconversion contributes to observed henipavirus dynamics, consistent with findings for bat filoviruses. Collectively, these studies also suggested a possible role for recrudescent infection or loss of immunity and reinfection in recovering henipavirus persistence. Broadly, data-fitted henipavirus models assumed no elevated mortality in infectious individuals; however, [Citation25,suggested this as a possible mechanism for observed declines in seroprevalence in older bats, as also posited for filoviruses. Generalizable trends for bat henipaviruses remain somewhat muddled largely due to the idiosyncratic nature of the datasets modeled – including one purely cross-sectional study [Citation27], one eighteen-month time series [Citation25], and one time series derived from a captive colony [Citation71]. Notably, no existing study has yet to fit a compartmental transmission model to the Australian bat reservoirs for Hendra virus, despite claims that this system is a model system for understanding bat virus spillover [Citation83].
Finally, our review identified a notable dearth of studies investigating coronavirus dynamics in wild bat hosts, highlighting a critical future research priority, particularly given the COVID-19 pandemic. Notably, the one fitted coronavirus model recovered in our analyses was the only mechanistic model identified which was fitted to PCR-based data, instead of serology, allowing for effective testing of a hypothesis of persistent infection and periodic shedding – as has been posited to drive bat virus dynamics more broadly [Citation11]. Since bat-borne coronaviruses are typically localized in the gastrointestinal tract and shed in feces, these viruses may be amenable to under-roost noninvasive surveillance in a manner similar to that previously adopted for henipaviruses [Citation82], offering one potential future avenue to make rapid gains in our understanding of the ecology of these pathogens in their bat reservoir systems.
Collectively, the vast majority of fitted bat virus models were targeted toward deciphering the dynamics of lyssaviruses in New World or European Yangochiropterans or henipaviruses in African Yinpteropchiropterans (). One notable research gap identified was the absence of any data-fitted models from Asia – the site of emergence for both the SARS-CoV and SARS-CoV-2 pandemic viruses [Citation2] – and the presence of only one data-fitted model from Australia (, ). To date, mechanistic models in bat virus systems have been largely limited to attempts to decipher the transmission mechanisms governing persistence of potentially zoonotic bat lyssaviruses, filoviruses, henipaviruses, and coronaviruses, though ultimately, the field aims to use these tools to do much more: mechanistic models offer a powerful means of describing causal relationships, predicting future trends, and exploring the efficacy of potential interventions. These models’ capacity for meeting more complex challenges rests, however, on the accuracy of the parameter choices and assumptions upon which they are based. The field of bat virus modeling has been limited by a lack of basic understanding of the pathogenesis of these four key viral taxa within an individual bat host, forcing researchers to adopt expansive model comparison approaches to deciphering transmission dynamics that likely only approximate a still-undiscovered reality [e.g. Citation25, Citation41, Citation71]. To date, the most progress has been made in deciphering the dynamics of rabies virus, largely because this system stems from a long history predicated on within-host experimental infections in live bats [Citation84]. Challenges in cost and containment have limited the progress of similar approaches for filo- or henipaviruses of known zoonotic capacity [e.g. Citation59,Citation60,Citation85–87] and been largely unexplored for coronaviruses. Isolation and experimental infections of bat-derived henipaviruses, filoviruses, and coronaviruses without known capacity for human spillover may offer an opportunity for within-host insights to be gleaned with fewer restrictions. Ultimately, studies linking pathogenesis of a particular virus in a particular bat host with data collected for that same host and virus in the field are greatly needed to expand mechanistic inference.
In the face of current limitations surrounding our understanding of the within-host pathogenesis of several clades of bat-borne virus, we propose a few key opportunities for research advances. Firstly, mechanistic modeling of heterogenous field data need not rely so exclusively on classic compartmental approaches; most of the studies highlighted in our subset fit models to pathogens with transmission dynamics that operate on timescales far more rapid than the interval of data collection – such that model fitting attempts to infer mechanism at a scale finer than the data can offer. The vast range of R0 estimates recovered across similar bat-virus systems suggests that research to date has failed to account for critical features of many bat virus infections; for example, [Citation71] estimate a 30-fold higher value for R0 when assuming seropositivity to represent E. helvum bats recovered from vs. actively infected with Ghanaian henipavirus. Such massively different estimates for equally plausible transmission scenarios suggest that the field is reaching for mechanism well beyond that which the data can currently supply. Added insight could instead be derived from more statistically based approaches, such as Bayesian state-space modeling, which can be used to back-infer exposure timing from serological data or discriminate source hosts from recipient hosts in multi-species settings [Citation88,Citation89] – rather than attempting to reconstruct entire time-series. Similarly, more relaxed approaches to dynamical modeling, which allow for time-varying transmission rates without specifying a mechanism [e.g. Citation90], could help overcome uncertainties and gaps in existing field data.
Ultimately, however, if we are serious about preventing the next major pandemic of a bat-borne zoonotic virus, we will need to greatly expand model-guided field studies to reflect the global extent of bat hosts and their pathogens [Citation91]. We recognize that there are considerable barriers to data collection that may slow progress in this field, but advances are needed in order to reduce future public health threats. In particular, more studies incorporating PCR-based viral shedding data, in concert with serology, would greatly enhance our capacity for mechanistic inference from field data by allowing for both a snapshot of immediate infections paired with infection history derived from serology. Nonetheless, active bat virus infections can be notoriously difficult to detect: though a number of bat species have been found to be positive for filovirus antibodies, PCR-positivity for these pathogens is rare and more often detected invasively in organ tissue samples rather than excreta [Citation10]. Additionally, age-structured serological data (documentation of numerical age information paired with serological status) offer another means by which to heighten the mechanistic insight that can be gleaned from field data, by allowing for the fitting of catalytic models to age-seroprevalence curves [Citation92]. Historically, age data have been difficult to obtain from wild bats, requiring invasive chemical and morphological analysis of bat bones or dentition, or long-term tracking of tagged individuals [Citation93]. Recent progress in the bat aging field – in particular the development of epigenetic clocks for bats [Citation94,Citation95] – offers an exciting opportunity to make collection of age-structured serological information more widespread.
Inference from fitted mechanistic models can also be strengthened via longitudinal data collection in repeatedly resampled populations, in particular those which track individuals through capture-mark-recapture processes. The recapture of longitudinally tracked individuals, as modeled in [Citation71] for example, allows for investigation of changes in within-host serological status and its influence on population-level fluctuations in transmission. Captive colony studies like those used in [Citation71] offer the advantage of facilitating access to individuals for recapture, as well as safeguarding the boundaries of closed population assumptions for modeling. Nonetheless, captive environments lack the ecological complexities of metapopulation and interspecies mixing that likely play critical roles in driving bat virus dynamics in the wild [Citation41,Citation43]. Longitudinal field data collection may, however, be infeasible, given difficult-to-access bat roosting sites, which bats sometimes abandon in response to researcher visitation [Citation96].
In this review, we summarize our current understanding of the mechanisms underpinning viral persistence in bat-virus systems and highlight several areas in which future approaches could be prioritized and improved. Both techniques and insights have diversified and developed in the thirteen years since the first dynamical model of a bat-virus system was published; nonetheless, there remains much to still discover.
Supplemental Material
Download Zip (297.8 KB)Acknowledgments
The authors thank Fidisoa Rasambainarivo, Tim Treuer, Fifi Ravelomanantsoa, Hafaliana Ranaivoson, Santino Andry, Angelo Andrianiaina, and Kimberly Rivera for helpful comments on the manuscript.
Disclosure statement
The authors declare no conflict of interest.
Supplementary material
Supplemental data for this article can be accessed here.
Additional information
Funding
References
- Calisher CH, Childs JE, Field HE, et al. Bats: important reservoir hosts of emerging viruses. Clin Microbiol Rev. 2006;19(3):531–545.
- Wang L, Anderson DE, Mackenzie JS, Merson MH. From Hendra to Wuhan: what has been learned in responding to emerging zoonotic viruses. Lancet. 2020;6736(20):2019–2020. Elsevier Ltd.
- WHO. Coronavirus disease (COVID-2019) situation reports. 2020 [cited 2020 Jun 30]. Available from: https://www.who.int/emergencies/diseases/novel-coronavirus-2019/situation-reports
- Andersen KG, Rambaut A, Lipkin WI, Holmes EC, Garry RF. The proximal origin of SARS-CoV-2. Nature. 2020;26:450–455.
- Boni MF, Lemey P, Jiang X, Lam TT, Perry BW, Castoe TA, et al. Evolutionary origins of the SARS-CoV-2 sarbecovirus lineage responsible for the COVID-19 pandemic. Nat Microbiol. 2020. Springer US. DOI:https://doi.org/10.1038/s41564-020-0771-4.
- Zhou H, Chen X, Hu T, et al. A novel bat coronavirus closely related to SARS-CoV-2 contains natural insertions at the S1/S2 cleavage site of the spike protein. Curr Biol. 2020;30(11):2196–2203.e3. Elsevier Ltd.
- Zhou P, et al. A pneumonia outbreak associated with a new coronavirus of probable bat origin. Nature. 2020. DOI:https://doi.org/10.1038/s41586-020-2012-7.
- Liao Y, Wei W, Cheung WY, Li W, Li L, Leung GM, et al. Identifying SARS-CoV-2 related coronaviruses in Malayan pangolins. Nature. 2020;1–6.
- Wu F, Zhao S, Yu B, Chen YM, Wang W, Song ZG, et al. A new coronavirus associated with human respiratory disease in China. Nature. 2020;579(7798):265–269.
- Olival K, Hayman D. Filoviruses in bats: current knowledge and future directions. Viruses. 2014;6(4):1759–1788.
- Plowright RK, Peel AJ, Streicker DG, et al. Transmission or within-host dynamics driving pulses of zoonotic viruses in reservoir-host populations. PLoS Negl Trop Dis. 2016;10(8):e0004796.
- Schountz T, Baker ML, Butler J, et al. Immunological control of viral infections in bats and the emergence of viruses highly pathogenic to humans. Front Immunol. 2017;8(September):Article 1098.
- Ross R. Some a priori pathometric equations. Br Med J. 1915;1(2830):546–547.
- Kermack WO, McKendrick AG. A contribution to the mathematical theory of epidemics. Proc R Soc London A. 1927;115:700–721.
- Lessler J, Azman AS, Grabowski MK, Salje H, Rodriguez-barraquer I. Trends in the mechanistic and dynamic modeling of infectious diseases. Curr Epidemiol Rep. 2016;3:212–222.
- Chinazzi M, et al. The effect of travel restrictions on the spread of the 2019 novel coronavirus (COVID-19) outbreak. Science. 2020;368(6489):395–400.
- Zhang Q Perra N, Perrotta D, Tizzoni M, Paolotti D, Vespignani A. Forecasting seasonal influenza fusing digital indicators and a mechanistic disease model. 26th International World Wide Web Conference, WWW 2017; May 2019; 2017;(May 2019):311–9. DOI: https://doi.org/10.1145/3038912.3052678.
- Brook CE, Dobson AP. Bats as “special” reservoirs for emerging zoonotic pathogens. Trends Microbiol. 2015;23(3):172–180.
- Luis AD, et al. A comparison of bats and rodents as reservoirs of zoonotic viruses: are bats special? Proc R Soc B. 2013;280(20122753):1–9.
- Olival KJ, Hosseini PR, Zambrana-Torrelio C, Ross N, Bogich TL, Daszak P. Host and viral traits predict zoonotic spillover from mammals. Nature. 2017. Publishing Group. DOI:https://doi.org/10.1038/nature22975.
- Lloyd-Smith JO, George D, Pepin KM, et al. Epidemic dynamics at the human-animal interface. Science. 2009;326(5958):1362–1367.
- Allen LJS, Van Den Driessche P. The basic reproduction number in some discrete-time epidemic models. J Differ Equations Appl. 2008;14(10–11):1127–1147.
- Diekmann O, Heesterbeek JAP, Metz JA. On the definition and computation of the basic reproduction ratio R0 in models for infectious diseases in heterogenous populations. J Math Biol. 1990;28:365–382.
- Klepac P, Caswell H. The stage-structured epidemic: linking disease and demography with a multi-state matrix approach model. Theor Ecol. 2011;4(3):301–319.
- Brook CE, Ranaivoson HC, Broder CC, Cunningham AA, Héraud J-M, Peel AJ, et al. Disentangling serology to elucidate henipa- and filovirus transmission in Madagascar fruit bats. J Anim Ecol. 2019;1–16. DOI:https://doi.org/10.1111/1365-2656.12985.
- Oli M, Venkataraman M, Brown MB. Population dynamics of infectious diseases: a discrete time model. Ecol Modell. 2006;198:183–194.
- Peel AJ, Baker KS, Hayman DTS, et al. Support for viral persistence in bats from age-specific serology and models of maternal immunity. Sci Rep. 2018;8(1):3859.
- Dimitrov DT, Hallam TG, Rupprecht CE, et al. Integrative models of bat rabies immunology, epizootiology and disease demography. J Theor Biol. 2007;245:498–509.
- Dimitrov DT, Hallam TG, Rupprecht CE, et al. Adaptive modeling of viral diseases in bats with a focus on rabies. J Theor Biol. 2008;255:69–80.
- Dimitrov DT, King AA. Modeling evolution and persistence of neurological viral diseases in wild populations. Math Biosci Eng. 2008;5(4):729–741.
- Brook CE, Boots M, Chandran K, et al. Accelerated viral dynamics in bat cell lines, with implications for zoonotic emergence. eLife. 2020;9:e48401.
- Amengual B, Bourhy H, López-Roig M, Serra-Cobo J. Temporal dynamics of European bat Lyssavirus type 1 and survival of Myotis myotis bats in natural colonies. PLoS One. 2007;2(6):e566.
- Pons-Salort M, Serra-Cobo J, Jay F, et al. Insights into persistence mechanisms of a zoonotic virus in bat colonies using a multispecies metapopulation model. PloS One. 2014;9(4):e95610.
- Bakker KM, Rocke TE, Osorio JE, et al. Fluorescent biomarkers demonstrate prospects for spreadable vaccines to control disease transmission in wild bats. Nat Ecol Evol. 2019;3:1697–1704. Springer US.
- Afonso CL, Amarasinghe GK, Bányai K, et al. Taxonomy of the order Mononegavirales: update 2016. Arch Virol. 2016;161:2351–2360.
- Markotter W, Coertse J. Bat lyssaviruses. Sci Tech Rev Office Int Des Epizooties. 2018;37(2):385–400.
- Hampson K, Coudeville L, Lembo T, Sambo M, Kieffer A, Attlan M, et al. Estimating the global burden of endemic canine rabies. PLoS Negl Trop Dis. 2015;9(4):1–20.
- Messenger SL, Smith JS, Rupprecht CE. Emerging epidemiology of bat-associated cryptic cases of rabies in humans in the United States. Emerging Infect. 2002;35:738–747.
- Banyard AC, Davis A, Gilbert AT, Markotter W. Bat rabies. In: abies: scientific basis of the disease and its management. 4th ed. London (UK) Elsevier Inc.; 2000. p. 231–276. DOI:https://doi.org/10.1016/b978-0-12-818705-0.00007-8.
- George DB, Webb CT, Farnsworth ML, et al. Host and viral ecology determine bat rabies seasonality and maintenance. Proc Nat Acad Sci. 2011;108(25):10208–10213.
- Blackwood JC, Streicker DG, Altizer S, et al. Resolving the roles of immunity, pathogenesis, and immigration for rabies persistence in vampire bats. Proc Nat Acad Sci. 2013;110(51):20837–20842.
- Streicker DG, Recuenco S, Valderrama W, et al. Ecological and anthropogenic drivers of rabies exposure in vampire bats: implications for transmission and control. Proc R Soc B. 2012;279(1742):3384–3392.
- Colombi D, Serra-Cobo J, Métras R, Apoll A, Poletto C, Lópe M, et al. Mechanisms for lyssavirus persistence in non-synanthropic bats in Europe: insights from a modeling study. Sci Rep. 2019;9:537.
- Franka R, Johnson N, Müller T, et al. Susceptibility of North American big brown bats (Eptesicus fuscus) to infection with European bat lyssavirus type 1. J Gen Virol. 2008;89(8):1998–2010.
- Freuling C, Vos A, Johnson N, et al. Experimental infection of serotine bats (Eptesicus serotinus) with European bat lyssavirus type 1a. J Gen Virol. 2009;90(10):2493–2502.
- Davis PL, Holmes EC, Larrous F, Van der Poel WHM, Tjørnehøj K, Alonso WJ, et al. Phylogeography, population dynamics, and molecular evolution of European bat lyssaviruses. J Virol. 2005;79(16):10487–10497.
- Hayman DTS, Luis AD, Restif O, et al. Maternal antibody and the maintenance of a lyssavirus in populations of seasonally breeding African bats. PloS One. 2018;13(6):e0198563.
- Centers for Disease Control and Prevention. US, Filoviridae. 2018.
- Guth S, Visher E, Boots M, et al. Host phylogenetic distance drives trends in virus virulence and transmissibility across the animal – human interface. Phil Trans R Soc B. 2019;374(1782):20190296.
- Amman BR, Carroll SA, Reed ZD, et al. Seasonal pulses of Marburg virus circulation in juvenile Rousettus aegyptiacus bats coincide with periods of increased risk of human infection. PLoS Pathog. 2012;8(10):e1002877.
- Towner JS, Amman BR, Sealy TK, et al. Isolation of genetically diverse Marburg viruses from Egyptian fruit bats. PLoS Pathog. 2009;5(7):e1000536.
- Leroy EM, Kumulungui B, Pourrut X, et al. Fruit bats as reservoirs of Ebola virus. Nature. 2005;438(7068):575–576.
- Pourrut X, Kumulungui B, Wittmann T, et al. The natural history of Ebola virus in Africa. Microbes Infect. 2005;7(7–8):1005–1014.
- Berge T, Lubuma J, et al. Dynamics of host-reservoir transmission of Ebola with spillover potential to humans. Electron J Qual Theory Differ Equ. 2018;(14):1–32.
- Berge T, Bowong S, Lubuma J, et al. Modeling Ebola Virus Disease transmissions with reservoir in a complex virus life ecology. Math Biosci Eng. 2018;15(1):21–56.
- Buceta J, Johnson K. Modeling the Ebola zoonotic dynamics: interplay between enviroclimatic factors and bat ecology. PLoS One. 2017;12(6):e0179559.
- Rhoubari ZEL, Besbassi H, Hattaf K, Yousfi N. Mathematical modeling of Ebola Virus Disease in bat population. Discrete Dyn Nat Soc. 2018;5104524.
- Hayman DTS. Biannual birth pulses allow filoviruses to persist in bat populations. Proc R Soc B. 2015;282(20142591). DOI:https://doi.org/10.1098/rspb.2014.2591.
- Paweska JT, et al. Lack of Marburg virus transmission from experimentally infected to susceptible in-contact Egyptian fruit bats. J Infect Dis. 2015;1–10. DOI:https://doi.org/10.1093/infdis/jiv132.
- Schuh AJ, Amman BR, Jones MEB, Sealy TK, Uebelhoer LS, Spengler JR, et al. Modelling filovirus maintenance in nature by experimental transmission of Marburg virus between Egyptian rousette bats. Nat Commun. 2017;8:14446. Nature Publishing Group.
- Rima B, Balkema-Buschmann A, Dundon WG, et al. ICTV virus taxonomy profile: Paramyxoviridae. J Gen Virol. 2019;100:1593–1594.
- Halpin K, Young PL, Field HE, et al. Isolation of Hendra virus from pteropid bats: A natural reservoir of Hendra virus. J Gen Virol. 2000;81(Pt8):1927–1932.
- Anderson DE, Islam A, Crameri G, et al. Isolation and full-genome characterization of Nipah Viruses from Bats, Bangladesh. Emerg Infect Dis. 2019;25(1):166–170.
- Marsh GA, de Jong C, Barr JA, et al. Cedar virus: a novel Henipavirus isolated from Australian bats. PLoS Pathog. 2012;8(8):e1002836.
- Drexler JF, Corman VM, Müller MA, et al. Bats host major mammalian paramyxoviruses. Nat Commun. 2012;3:796.
- Wu Z, Yang L, Yang F, et al. Novel Henipa-like virus, Mojiang paramyxovirus, in rats, China. Emerg Infect Dis. 2012;20(6):1064–1066.
- Weatherman S, Feldmann H, De WE. Transmission of henipaviruses. Curr Opin Virol. 2018;28:7–11. Elsevier B.V.
- Pernet O, Schneider BS, Beaty SM, et al. Evidence for henipavirus spillover into human populations in Africa. Nat Commun. 2014;5:5342. Nature Publishing Group.
- Plowright RK, Foley P, Field HE, et al. Urban habituation, ecological connectivity and epidemic dampening: the emergence of Hendra virus from flying foxes (Pteropus spp.). Proc R Soc B. 2011;278(1725):3703–3712.
- Wang -H-H, Kung NY, Grant WE, et al. Recrudescent infection supports Hendra virus persistence in Australian flying-fox populations. PloS One. 2013;8(11):e80430.
- Glennon EE, Becker DJ, Peel AJ, et al. What is stirring in the reservoir? Modelling mechanisms of henipavirus circulation in fruit bat hosts. Phil Trans R Soc B. 2019;374:20190021.
- Peel AJ, Wells K, Giles J, et al. Synchronous shedding of multiple bat paramyxoviruses coincides with peak periods of Hendra virus spillover. Emerg Microbes Infect. 2019;8(1):1314–1323.
- Wong ACP, Li X, Lau S, et al. Global epidemiology of bat coronaviruses. Viruses. 2019;11(174):v11020174.
- Woo PCY, Lau SKP, Huang Y, et al. Coronavirus diversity, phylogeny and interspecies jumping. Exp Biol Med. 2009;234(10):1117–1127.
- Su S, Wong G, Shi W, et al. Epidemiology, genetic recombination, and pathogenesis of coronaviruses. Trends Microbiol. 2016;24(6):490–502. Elsevier Ltd.
- Anthony SJ, Johnson CK, Greig DJ, et al. Global patterns in coronavirus diversity. Virus Evol. 2017;3(1):1–15.
- Chen TM, Rui J, Wang Q-P, et al. A mathematical model for simulating the phase-based transmissibility of a novel coronavirus. Infect Dis Poverty. 2020;9(1):1–8.
- Jeong J, SMITH CS, PEEL AJ, et al. Persistent infections support maintenance of a coronavirus in a population of Australian bats (Myotis macropus). Epidemiol Infect. 2017;145:2053–2061.
- Kuhn JH, Becker S, Ebihara H, Geisbert TW, Johnson KM, Kawaoka Y et al. Proposal for a revised taxonomy of the family Filoviridae: classification, names of taxa, and virus abbreviations. Archives of Virology. 2010; 155(12):2083–103
- Negredo A, Palacios G, Vázquez-Morón S, González F, Dopazo H, Molero F, et al. Discovery of an Ebolavirus-Like Filovirus in Europe. PLoS Pathogens. 2011;7(10)
- Turmelle AS, Jackson FR, Green D, et al. Host immunity to repeated rabies virus infection in big brown bats. J Gen Virol. 2010;91(Pt 9):2360–2366.
- Field H, de Jong C, Melville D, et al. Hendra virus infection dynamics in Australian fruit bats. PLoS ONE. 2011;6(12):8–13.
- Plowright RK, Eby P, Hudson PJ, Smith IL, Westcott D, Bryden WL, et al. Ecological dynamics of emerging bat virus spillover. Proc R Soc B. 2015;282(1798). DOI:https://doi.org/10.1098/rspb.2014.2124.
- Sulkin SE, Allen R. Experimental rabies virus infection in bats: review. In: Baer GM, editor. The natural history of rabies. New York: Academic Press, Inc.; 1975. p. 99–114.
- Jones M, Schuh A, Amman B, et al. Experimental inoculation of Egyptian Rousette bats (Rousettus aegyptiacus) with viruses of the Ebolavirus and Marburgvirus Genera. Viruses. 2015;7(7):3420–3442.
- Paweska JT, Jansen van Vuren P, Masumu J, et al. Virological and serological findings in Rousettus aegyptiacus experimentally inoculated with Vero cells-adapted Hogan strain of Marburg virus. PloS One. 2012;7(9):e45479.
- Schuh AJ, Amman BR, Sealy TK, et al. Egyptian rousette bats maintain long-term protective immunity against Marburg virus infection despite diminished antibody levels. Sci Rep. 2017;7(1):1–7.
- Behdenna A, Lembo T, Calatayud O, et al. Transmission ecology of canine parvovirus in a multi-host, multi-pathogen system. Proc R Soc B. 2019;286(1899):20182772.
- Cross PC, van Manen FT, Viana M, Almberg ES, Bachen D, Brandell EE, et al. Estimating distemper virus dynamics among wolves and grizzly bears using serology and Bayesian state-space models. Ecol Evol. 2018;8(17):8726–8735.
- Funk S, Camacho A, Kucharski AJ, Eggo RM, Edmunds WJ. Real-time forecasting of infectious disease dynamics with a stochastic semi-mechanistic model. Epidemics. 2018;22:56–61. Elsevier B.V.
- Restif O, Hayman DTS, Pulliam JRC, et al. Model-guided fieldwork: practical guidelines for multidisciplinary research on wildlife ecological and epidemiological dynamics. Ecol Lett. 2012;15(10):1083–1094.
- Muench H. Catalytic models in epidemiology. Boston, MA, USA: Harvard University Press; 1959.
- Brunet-Rossinni AK, Wilkinson GS. Methods for age estimation and the study of senescence in bats. Ecological and behavioral methods for the study of bats. 2003 [cited 2009 Dec 11]. p. 315–326. Available from: http://www.life.umd.edu/faculty/wilkinson/Brunet-Rossini_ch15.pdf.
- Wilkinson GS, et al. Genome methylation predicts age and longevity of bats. bioRxiv. 2020.
- Wright PGR, Mathews F, Schofield H, Morris C, Burrage J, Smith A, et al. Application of a novel molecular method to age free-living wild Bechstein’s bats. Mol Ecol Resour. 2018;18(6):1374–1380.
- Kerth G, Ebert C, Schmidtke C. Group decision making in fission-fusion societies: evidence from two-field experiments in Bechstein’s bats. Proc R Soc B. 2006;273(1602):2785–2790.