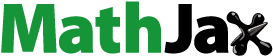
ABSTRACT
The distinctive features of human influenza A phylogeny have inspired many mathematical and computational studies of viral infections spreading in a host population, but our understanding of the mechanisms that shape the coupled evolution of host immunity, disease incidence and viral antigenic properties is far from complete. In this paper we explore the epidemiology and the phylogeny of a rapidly mutating pathogen in a host population with a weak immune response, that allows re-infection by the same strain and provides little cross-immunity. We find that mutation generates explosive diversity and that, as diversity grows, the system is driven to a very high prevalence level. This is in stark contrast with the behavior of similar models where mutation gives rise to a large epidemic followed by disease extinction, under the assumption that infection with a strain provides lifelong immunity. For low mutation rates, the behavior of the system shows the main qualitative features of influenza evolution. Our results highlight the importance of heterogeneity in the human immune response for understanding influenza A phenomenology. They are meant as a first step toward computationally affordable, individual based models including more complex host-pathogen interactions.
Introduction
Many of the outstanding challenges in infectious disease control involve rapidly mutating RNA viruses and other antigenically diverse pathogens that can escape immunity through strain replacement at the population level or within individual hosts.Citation1,2 Understanding this dynamics is of great practical importance, and has been the subject of theoretical efforts since the beginning of this century.Citation3,4 The term ‘phylodynamics’ was coined to describe the study of the coupled epidemiological, immunological, and evolutionary processes that shape the patterns of viral genetic variation.Citation1 These phylodynamic patterns can be grouped in broad classes, associated with characteristic qualitative features of the phylogeny and the epidemiology of the pathogen.Citation1,5 The influenza A virus is the best documented example of one such class, which also includes the foot and mouth disease virus, characterized by short infections, partial cross-immunity between strains, and limited diversity at any given time. Phylogenetic studies of influenza A focus on haemagglutinin (HA) and neuraminidase (NA), the surface glycoproteins that are the main viral antigens and define each subtype, and may combine genetic with antigenic information obtained through hemagglutination inhibition assays.Citation6,7
Among the diseases of its phylodynamic class, the human influenza A virus exhibits within each subtype a distinctive ladder-like phylogeny, with limited diversity in the population at any given time combined with significant variation over time.Citation1,5 This progressive turnover of the amino acid variants of the surface proteins of the circulating strains is called antigenic drift. Vaccines against seasonal influenza are updated every year to keep pace with this variation. It has been further shown that antigenic drift is driven by positive selection,Citation8 and that different strains are organized according to antigenic distance in clusters rather than uniformly distributed in antigenic space,Citation6 so that while genetic change occurs smoothly the corresponding antigenic variation is punctuated, with changes between antigenically similar strains belonging to the same cluster alternating every few years with ‘cluster jumps’. In cluster transitions the cross-immunity between the emerging strain and its predecessors is lower than that between strains of the same cluster, and cluster jumps are associated with the larger seasonal outbreaks observed every few years in the interpandemic epidemic patterns.Citation9
These findings prompted a series of theoretical studies of multi-strain pathogens competing through cross immunity, based on mathematical and computational models, with the common goal of establishing conditions that reproduce the characteristic phylogenetic pattern of influenza A when pathogen mutation is included. Realistic patterns have been obtained under different biological assumptions: short-term temporary strain-transcending immunity,Citation4, 10-12 antigenic strain space structure,Citation3,9,13-15 and lifelong cross-protection in a fixed set of antigenic types.Citation16,17
With so far no experimental confirmation of any of the biological foundations of these competing hypothesis, the problem of understanding the mechanisms that drive the punctuated antigenic evolution of influenza A is still largely open. Several different variants that do without those specific biological assumptions have also been extensively explored, showing that extinction or explosive diversity are the two possible outcomes of simpler models.Citation18-22 Under the assumption that infection with a strain provides lifelong immunity, strong positive selection drives escape mutants generating a large epidemic followed by disease extinction.Citation22 This outcome can be avoided only at the expense of very high mutation rates Citation18 or very high cross immunity between similar strains,Citation21 in which cases explosive diversity ensues.
Despite evidence of variation in the individual immune responses to identical strains Citation23 and of weak immune responses in young children,Citation23,24 models that relax the assumption that infection with a strain provides lifelong immunity have received little attention in studies of influenza. Work in this direction shows that the interplay of two interacting subpopulations with different types of immune response, narrow and weak or broad and strong, generates in a constrained strain space some of the incidence and phylogenetic patterns typical of influenza ACitation25. Further motivation to consider models where infection elicits imperfect protection comes from recent results showing evidence of reinfection by the same strain in an epidemic outbreak.Citation26
In this paper we consider a stochastic, individual-based model of influenza-like infections with mutations. Infection dynamics follows well-mixed Susceptible-Infected-Recovered (SIR) dynamics modified to represent a narrow and weak immune response as follows. We assume a simple setup where each viral strain is characterized by a certain number of epitopes, each of which is in one of a number of possible configurations. All epitopes have equal roles, and the infectious properties of viral strains are all identical; their effectiveness at infecting a host is fully determined by the host's infection history. Upon infection with a strain, the host will produce, independently of the number of epitopes of the strain, only one type of antibody that will be permanently stored in the host’s immune repertoire. Any healthy host with a given infection history can be infected by a virus transmitted through an infectious contact and the protection conferred by its immune repertoire is proportional to the number of matching antibodies for the antigenic sites of the challenging strain. Full protection occurs only when the host has matching antibodies for all antigenic sites. Thus consecutive reinfections by the same strain are possible, although with decreasing probability, except when the number of epitopes is equal to one, in which case the model recovers ordinary SIR dynamics.
Results
On the basis of analytic and computational studies of hemagglutination inhibition assays data, the dimension of the antigenic space of influenza A can be placed between 2 and 5.Citation6,27 In order to highlight the effects of weakening and narrowing the immune response, we have considered 5 epitopes throughout. The number of different configurations that each epitope may assume through mutation is arbitrary and limited only in practice by available memory when running the simulations.
The basic epidemiological parameters were fixed at realistic values for human influenza A. The mortality rate was set to μ = 1/70 years−1, corresponding to an average human lifespan of 70 years, and the birth rate was taken equal to the mortality rate. The average recovery rate was set to γ = 90 years−1, corresponding to an average duration of infection of about four days. The transmission rate β = 136.5 years−1 corresponds to a basic reproduction number of roughly R0 = 1.5 which is consistent with recent literature.Citation28,29 The mutation rate m was left as a free parameter. The population size N is fixed at one million, a realistic value for a well-mixed model. This choice of N is also a good compromise between limiting memory usage and avoiding frequent stochastic extinctions. Simulations generate a record of the total number of infected along time and of all the circulating strains every year.
We have first simulated disease dynamics starting from a small number of infected with a single founding strain in a totally susceptible population. For this type of initial conditions, extinction rates are high. For mutation rate m equal to 0.01 (respectively 0.02 and 0.03), 98,2 % (respectively 97,6%, 72,5%) become extinct after a large, short initial epidemic. Representative results for disease prevalence along the first 20 y of the remaining simulation runs are shown in for mutation rate m equal to 0.01, 0.02 and 0.03 (dashed lines). Also shown (full lines) are the results for prevalence obtained from averaging over 50 other 20 y long simulations performed under the same conditions. The corresponding yearly results for the antigenic distance to the founding strain and for the strain heterogeneity in the population are shown in a) to c) and (dashed lines), respectively. The distance is computed for each circulating strain as the number of single epitope substitutions that it underwent since its emergence from the founding strain. The growth of this metric with time is a measure of the antigenic drift of the pool of circulating strains. The heterogeneity of this pool of strains is monitorized by means of the v-index, a measure of strain diversity. A value v of this metric corresponds to the variability of a viral population where v strains are present with equal frequencies. More details on this quantity are given in the Methods section. As in , the full line curves in show the v-index along time, averaged over 50 simulation runs that persist for 20 years. The curves for the averaged quantities in are of course less noisy but they conserve the main trends of the individual simulations singled out for illustration, which shows that the latter are indeed representative of the behavior of the system.
Figure 1. Prevalence as a function of time for 20 y long simulations starting with 100 infected with a single strain in a population of 106 healthy individuals with no previous exposure. Dashed lines are for single simulation runs, full lines for averages over 50 runs. Three different values for the mutation rate m were considered: 0.01 (brown line), 0.02 (red line) and 0.03 (yellow line). See the main text for the values of the remaining parameters.
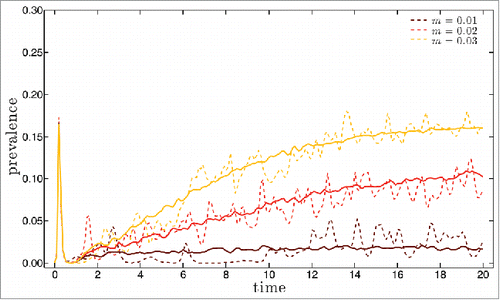
Figure 2. For the set of 3 single runs of , distance to the founding strain measured in number of consecutive mutations as a function of time. All the distances that have representatives in the set of circulating strains are shown each year color coded according to frequency. a) m=0.01; b) m=0.02; c) m=0.03.
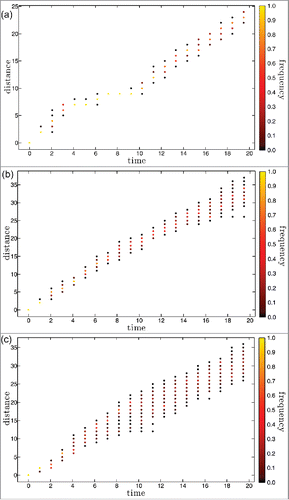
Figure 3. For the set of 3 mutation rate values of and with the same color code, diversity of the set of circulating strains as a function of time measured by the v-index (see main text for a precise definition of this measure). Dashed lines are for single simulation runs, full lines for averages over 50 runs.
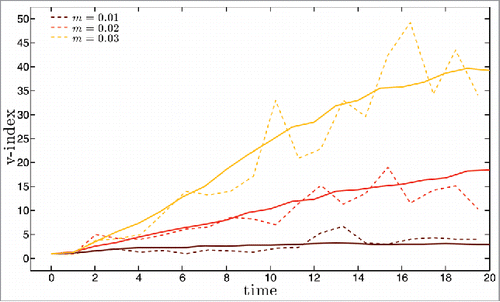
Independently of the mutation rate, all simulations start with a large epidemic of approximately similar size that reflects infections of totally naïve hosts and reinfections by the founding strain. Immunity build up drives the disease close to extinction after this large epidemic, but escape mutations counteract herd immunity and extinction is avoided. For smaller values of m, more than 98% of the simulations become extinct after the first large epidemic.
After the first year, a mutant of the founding strain that becomes the dominant strain initiates a second, much smaller, epidemic wave. After the second year a smoother process starts from a set of escape mutants, further away from the founding strain. This process consists of a sequence of small epidemic bursts over an underlying trend of disease prevalence growth. The rate of prevalence growth increases with mutation rate. It reflects the increase in antigenic distance by which the circulating strains circumvent the immune response of hosts that recovered after the first large epidemic. When at about year 10 antibodies created for the founding strain no longer match most of the epitopes of the circulating strains, prevalence growth goes on at a slower rate, because it also reflects the growing diversity of the pool of circulating strains, by which multiple reinfections become more likely. These three processes feed-back positively on each other, as can be seen in the figures for m=0.01 between year 8 and year 10, where a population bottleneck practically freezes strain evolution.
The burst and bust pattern that can be seen over this background of growing prevalence is due to the appearance of mutants that explore and then exhaust niches of unprotected or poorly protected hosts, in small scale replicas of the first large epidemic. This effect is more apparent for smaller mutation rates and smaller prevalences, when the time scale of the disease dynamics is fast compared with the average time between mutation events.
In brief, during a period of 20 y and for all the mutation rates the system undergoes antigenic drift accompanied by prevalence and diversity growth, at rates that are larger in the first few years when the effects of the immune response to the initial large epidemic are still relevant.
In order to remove the transient due to having initiated the simulations in a scenario of pandemia, and to see whether the system eventually reaches a steady state in a reasonably short time, we have also simulated disease dynamics and evolution starting from a single founding strain close to its equilibrium in the absence of mutations. This has the advantage of allowing direct comparison with results obtained under strong immunity assumptions for similar initial conditions,Citation22,30,31 and together with the results above it covers the two extreme cases as regards the choice of initial conditions. The equilibrium values were computed from a mean-field model of the system for m=0, as detailed in the Methods section.
This type of initial conditions does not generate an initial pandemia and therefore the extinction rates are much lower than in the previous analysis. For m = 0.05 and m = 0.1, 50 out of 50 simulation runs avoid extinctions altogether, while for m = 0.02 (resp. m = 0.01) the extinction rate is 76% for m = 0.01 and 47% for m = 0.02, due to stochastic extinctions during the initial, low prevalence regime. Illustrative results for disease prevalence along the first 20 y are shown in for mutation rate m equal to 0.01, 0.02, 0.05 and 0.1 (dashed lines). The corresponding yearly results for the antigenic distance to the founding strain and for the strain heterogeneity in the population are shown in a) to d) and , respectively. In we also plot with full lines the averages over 50 runs of the prevalence and v-index for each of the 4 mutation rates considered in the individual simulations.
Figure 4. Prevalence as a function of time for simulations up to 20 y long starting with initial conditions close to the single strain equilibrium in the absence of mutations. Four different values for the mutation rate m were considered: 0.01 (brown line), 0.02 (red line) and 0.05 (orange line) and 0.1 (yellow line). Dashed lines are for single simulation runs, full lines for averages over 50 runs. Population size and remaining parameters as in .
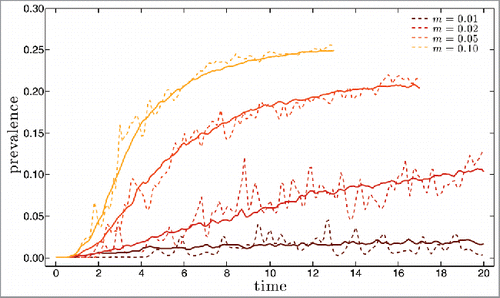
Figure 5. For the set of 4 single runs of , distance to the founding strain measured in number of consecutive mutations as a function of time. All the distances that have representatives in the set of circulating strains are shown each year color coded according to frequency. a) m=0.01; b) m=0.02; c) m=0.05; d) m=0.1.
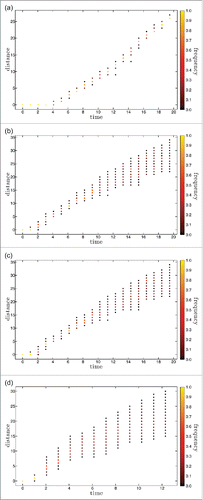
Figure 6. For the set of 4 mutation rate values of and with the same color code, diversity of the set of circulating strains as a function of time measured by the v-index (see main text for a precise definition of this measure). Dashed lines are for single simulation runs, full lines for averages over 50 runs.
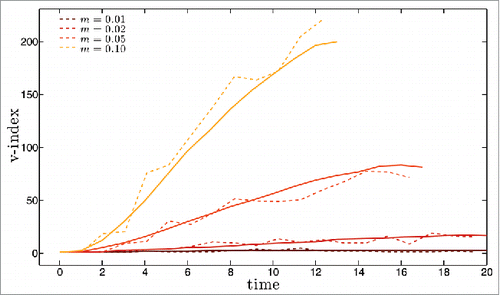
We shall focus only on the features that have not been discussed earlier in this section. Departure from the initial equilibrium situation occurs at the time when the first escape mutant manages to invade, which depends accordingly on the mutation rate. With this smoother initiation the system seems to reach a steady-state, in terms of prevalence, for m = 0.01, m = 0.05 and m = 0.1.
For m = 0.01, the prevalence values are qualitatively compatible with influenza, and the overall behavior shows the main features of influenza phylodynamics, with a pronounced antigenic drift and limited diversity at any given time. This is a surprising result for such a simple system.
The behavior for m = 0.02 interpolates between this scenario and that for larger values of m, and in this case prevalence is still increasing in year 20. Results for m = 0.05 and m = 0.1 are similar in that they both exhibit a very high steady state prevalence, explosive diversity and high rate of antigenic drift, with all these more pronounced for the larger m value. Both simulations end prematurely due to a cut-off on the total number of different epitope configurations actually generated imposed to take into account memory limitations. The most striking feature of these results is how the prevalence shoots up to values that are close to the equilibrium of the Susceptible-Infected-Susceptible (SIS) model, which assumes that infections confer no immunity at all.
This phenomenon can be understood in terms of the concept of reinfection threshold,Citation32 created for traditional compartmental systems with no mutation and reinfection. Systems with reinfection interpolate between SIR and SIS dynamics and, as the reinfection probability σ increases, the equilibrium prevalence value changes from the SIR value to the much higher SIS value. For populations with a low turnover of naïve susceptible such as in the case of influenza where it is given by the birth rate, this interpolation is sigmoid like, with a sharp transition between very low and very high levels of prevalence when σ crosses the value 1/R0, dubbed the reinfection threshold. Our model includes reinfection implicitly through the narrow and weak immunity assumptions, rather than through a fixed reinfection probability. Clearly the probability of a host being reinfected increases as strain diversity increases, crossing the reinfection threshold at about year 2 for m = 0.1, and year 4 for m = 0.05.
We have found no new phenomenology while exploring different population sizes in the range 500.000–2.000.000 and different values of R0 in the range 1.3–2.0 reported in the literature for seasonal influenza in the last decades (results not shown).
Discussion
While this paper was being written, we became aware of two independent major breakthroughs in influenza vaccine developmentCitation33,34 that bring us much closer to a universal influenza vaccine, one that would be effective across different strains within a subtype and even across different subtypes. The key idea to achieve such broad protection is to elicit immune responses directed at a highly conserved region of haemagglutinin, instead of the immunodominant and rapidly evolving H1 domain of HA. If or when this goal is reached, the whole strategy of prevention and control of the disease will change. But even then a mechanistic understanding of influenza evolution will be of great practical and theoretical importance, to inform studies of vaccination efficacy and as a paradigm for rapidly mutating pathogens competing through cross-immunity.
In recent years we witnessed massive data collection from surveillance platforms,Citation35,36 sophisticated data analysis and experimental work that is shedding light on the interplay between disease evolution and host immunity,Citation37-40 and elaborate theoretical studies already mentioned in the introduction. The latter produced several competing models each of which is, when suitably parametrized, capable of generating realistic phylodynamics patterns. However, this ability to reproduce the qualitative features of real data depends crucially on unconfirmed biological assumptions. Without these, the generic outcome of such models, i.e. with no fine tuning of parameters, is disease extinction.
Disease extinction in this context occurs after large epidemics that reflect strong positive selection of escape mutants. Smoothly growing diversity at moderate mutation rates requires a much weaker positive selection, and one way of achieving this is of course to fine tune inter strain cross-immunity to very high values. Another way, in our view much more natural, is to challenge the assumption, shared by all those theoretical models, that infection with a strain confers lifelong immunity to that strain, as we do here.
This study was set so as to highlight the consequences of that alternative rather than as an attempt at realistic influenza modeling. Our results include a nice example of the reinfection threshold phenomenon in a non-equilibrium context as a bonus for this deliberate lack of realism. Even so, the model also yields for a certain mutation rate range phylodynamics patterns that are compatible with influenza A within subtype evolution. From the theoretical point of view, this work should be taken as a proof of principle that heterogeneous host immune response including imperfect protection is an important ingredient for understanding the long term evolution of the disease. This idea was put forward with a different motivation in previous work and illustrated with results obtained for a more complex model that included strain space structure and host population structure as well.Citation25 Strain space constraints are known to influence significantly the behavior of these models,Citation15 but our results indicate that the main features of those results are robust with respect to the removal of strain space structure.
In more general terms, this paper brings support to the view that understanding pathogen evolution requires abandoning the simple SIR paradigm for more complex models of host-pathogen interactionCitation41 and is also a first step toward a comprehensive computational modeling platform where such models can be tested for realistic population sizes on a desktop computer. The modular structure of the code built for the present work allows changing some assumptions, such as the host’s immunity build-up and response and the form of the cross-immunity function, through easy adaptations. It is also possible to include different types of hosts, characterized by their immune performance. Still missing and to be included in future versions are important features such as seasonality, epitope variability and immunodominance, and population structure. A user friendly version of the code will be released publicly when available. In the meantime, interested potential users can contact the authors.
Methods
Traditional compartmental models based on SIR dynamics may be straightforwardly extended to describe multi-strain systems in term of a set of differential equations whose number grows exponentially with the number of strains. Several different approximations have been developed to obtain reduced compartmental models of lower dimension for multi-strain dynamics, but different approximations can lead to qualitatively different results.Citation14,22 Moreover, compartmental models implicitly assume large populations in each compartment, while systems with mutations always have low population sizes in some classes. To circumvent these issues, we have studied the system using a stochastic, individual-based model (IBM) of influenza-like infections with mutations described in detail below. As opposed to traditional compartmental models of infectious disease spread, IBM models are not amenable to analytic treatment and can be computationally demanding, because each individual in the population is explicitly considered, along with its changing immune repertoire. But still they are a popular choice in the literature on long-term evolution of multi-strain systems, because they are free of the methodological difficulties of compartmental models.
In this IBM, strains are characterized by a set of 5 integers, each one representing a different configuration of the antigene at a certain epitope, and 107 different configurations are possible in principle for each epitope, which is large enough to ensure that mutations are limited only by memory availability. The population of N = 106 comprises S healthy and I infected individuals. An individual may be infected only with a single strain (no co-infection). Each individual in the population has an immune repertoire made up of 5 sets of integers, representing antibodies against specific configurations of the antigenes at each of the 5 epitopes.
Immunity builds up upon recovery from infection by addition to the host’s immune repertoire of one antibody corresponding to a randomly selected epitope of the infecting strain (narrow immunity). Attempted infection of a host with a given immune repertoire succeeds with probability f, where f is the fraction of epitopes of the challenging strain that are matched by antibodies of the host (weak immunity).
The dynamics consists of the following stochastic processes:
Birth-death: At rate μ N, an arbitrarily chosen individual becomes healthy with an empty immune repertoire.
Infection and Immunity Acquisition: At rate β S I/N, an arbitrarily chosen infected individual may infect a healthy one, also arbitrarily chosen, which becomes infected with probability f. It then adds to its immune repertoire one antibody corresponding to one of the epitopes of the invading strain. Each possible antibody is produced with the same probability.
Recovery: At rate γ I, an arbitrarily chosen `infected individual becomes healthy.
Mutation: At rate m I, the strain infecting an arbitrarily chosen infected individual changes a random antigene to a random new configuration. If attempted infection with the new strain according to the above rule succeeds, the individual becomes infected with the new strain and undergoes immunity acquisition. Otherwise, the old configuration is retained.
The simulations were implemented using the Gillespie Citation42 event-driven algorithm for the above set of 4 reactions. The computer code was written in the C++ programming language, and will be made publicly available online in the near future. Its main features are the use of associative containers for fast and memory-efficient tracking of immune histories, and use polymorphism- and template-based idioms to allow for different immunity, infection and/or immunity mechanisms, as well as output metrics.
The output of the simulations includes total prevalence along time and, every year, 2 sets of additional data that characterize the viral population. One of them is a histogram of the distances of the circulating strains to the original strain, as measured by the number of mutations undergone by each strain. Notice that there is no one to one correspondence between strain identity, given by the configuration of the 5 epitopes, and strain distance to the founding strain. Clearly, different strains may be at the same distance from the original strain, and 2 copies of the same strain may also be at different distances. The second property registered each year is what we called the v-index or variability index v, a measure of the heterogeneity of the set of circulating strains defined as follows. Let ci (t), i=1,..,n be the copy numbers in decreasing order of the n different strains circulating at time t, and pi(t)=ci/I the corresponding frequencies in decreasing order. The v-index is defined as twice the average of the distribution pi minus one,, and it is easy to check that it has the following nice properties:
n ;
v(t) = 1 if and only if only one strain is present;
v(t) = n if and only if the n strains have equal frequencies.
So the v-index is a measure of heterogeneity that takes into account the number of strains that circulate weighted by the evenness of their frequency distribution.
The equilibrium of the system in the absence of mutations is found from the deterministic model that describes the evolution of the system in that case in the very large population limit. This is a SIR type model modified according to the narrow and weak immune response assumption. Up to 4 consecutive reinfections are possible, and on average the susceptibility to the k-th reinfection is reduced by a factor of (5-k)/5. Denote by s0 the density of totally naïve healthy individuals and by sk, k=1,…,4, that of healthy individuals that have experienced k infections. Denote also by ik, k=1,…,5, the density of infected experiencing their k-th infection and by I the sum of all ik. The equations that govern the evolution of the system are
The unique positive equilibrium of these equations was found numerically.
Disclosure of potential conflicts of interest
No potential conflicts of interest were disclosed.
Funding
Funding by the Portuguese FCT/MCTES/PIDDAC under Grant BioISI UID/MULTI/ 04046/2013 (A.N.) is gratefully acknowledged.
References
- Grenfell BT, Pybus OG, Gog JR, Wood JLN, Daly JM, Mumford JA, Holmes EC, Science 2004; 303(5656):327-32; PMID:14726583; http://dx.doi.org/10.1126/science.1090727
- Heesterbeek H, Anderson RM, Andreasen V, Bansal S, De Angelis D, Dye C, Eames KTD, Edmunds WJ, Frost SDW, Funk S, et al. Science 2015; 347(6227):):aaa4339; PMID:25766240; http://dx.doi.org/10.1126/science.aaa4339
- Gog JR, Grenfell BT, Proc Nat Acad Sci 2002; 99(26):17209-14; PMID:12481034; http://dx.doi.org/10.1073/pnas.252512799
- Ferguson NM, Galvani AP, Bush RM, Nature 2003; 422(6930):428-33; PMID:12660783; http://dx.doi.org/10.1038/nature01509
- Lipsitch M, Hagan JJ, J Roy Soc Interf 2007; 4 (16): 787-802; http://dx.doi.org/10.1098/rsif.2007.0229
- Smith DJ, Lapedes AS, de Jong JC, Bestebroer TM, Rimmelzwaan GF, Osterhaus ADME, Fouchier RAM. Science 2004; 305(5682):371-6; PMID:15218094; http://dx.doi.org/10.1126/science.1097211
- Nelson MI, Holmes EC. Nat Rev Genet 2007; 8(3):196-205; PMID:17262054; http://dx.doi.org/10.1038/nrg2053
- Shih A. C.-C., Hsiao T-C, Ho M-S, Li W-H. Proc Nat Acad Sci 2007; 104 (15):6283-8; PMID:17395716; http://dx.doi.org/10.1073/pnas.0701396104
- Koelle K, Cobey S, Grenfell B, Pascual M. Science 2006; 314(5807):1898-1903; PMID:17185596; http://dx.doi.org/10.1126/science.1132745
- Zinder D, Bedford T, Gupta S, Pascual M. PLoS Pathogens 2013; 9(1):e1003104; PMID:23300455; http://dx.doi.org/10.1371/journal.ppat.1003104
- Minayev P, Ferguson N. J Roy Soc Interf 2009; 6 (40):989-96; PMID: NOT_FOUND; http://dx.doi.org/10.1098/rsif.2008.0467
- Minayev P, Ferguson N. J Roy Soc Interf 2009; 6:509-18; PMID: NOT_FOUND; http://dx.doi.org/10.1098/rsif.2008.0333
- Gomes MGM, Medley GF, Nokes DJ. Proc Royl Soc London B: Biol Sci 2002; 269(1488):227-33; PMID:11839191; http://dx.doi.org/10.1098/rspb.2001.1869
- Kryazhimskiy S, Dieckmann U, Levin SA, Dushoff J. PLoS Comput Biol 2007; 3(8):e159; PMID:17708677; http://dx.doi.org/10.1371/journal.pcbi.0030159
- Bedford T, Rambaut A, Pascual M. BMC Biol 2012; 10(1):38; PMID:22546494; http://dx.doi.org/10.1186/1741-7007-10-38
- Recker M, Pybus OG, Nee S, Gupta S. Proc Nat Acad Sci 2007; 104(18):7711-6; PMID:17460037; http://dx.doi.org/10.1073/pnas.0702154104
- Wikramaratna PS, Sandeman M, Recker M, Gupta S. Philos Trans Roy Soc London B: Biol Sci 2013; 368(1614):20120200; PMID:23382423; http://dx.doi.org/10.1098/rstb.2012.0200
- Tria F, Lässig M, Peliti L, Franz S. J Stat Mech: Theor Exp 2005; 2005(07):P07008; http://dx.doi.org/10.1088/1742-5468/2005/07/P07008
- Nunes A, Telo da Gama MM, Gomes MGM. J Theor Biol 2006; 241(3):477-87; PMID:16427654; http://dx.doi.org/10.1016/j.jtbi.2005.12.010
- Ballesteros S, Vergu E, Cazelles B. PLoS ONE 2009; 4(10):e7426; PMID:19841740; http://dx.doi.org/10.1371/journal.pone.0007426
- Koelle K, Kamradt M, Pascual M. Epidemics 2009; 1(2):129-37; PMID:21352760; http://dx.doi.org/10.1016/j.epidem.2009.05.003
- Aquino T, Bolster D, Nunes A. J Theor Biol 2015; 368:27-36; PMID:25496729; http://dx.doi.org/10.1016/j.jtbi.2014.12.001
- Nakajima S, Nobusawa E, Nakajima K. Virology 2000; 274(1);220-31; PMID:10936103; http://dx.doi.org/10.1006/viro.2000.0453
- Sato K, Morishita T, Nobusawa E, Tonegawa K, Sakae K, Nakajima S, Nakajima K. Epidemiol Infect 2004; 132(3):399-406; PMID:15188708; http://dx.doi.org/10.1017/S0950268803001821
- Parisi A, Lopes JS, Nunes A, Gomes MGM. Ecol Complex 2013; 14:157-65; http://dx.doi.org/10.1016/j.ecocom.2012.12.001
- Camacho A, Ballesteros S, Graham AL, Carrat F, Ratmann O, Cazelles B. Proceedings of the Royal Society of London B: Biological Sciences 2011; 278 (1725): 3635-43.
- Lapedes A, Farber R. J Theor Biol 2001; 212(1): 57-69; PMID:11527445; http://dx.doi.org/10.1006/jtbi.2001.2347
- Medlock J, Galvani AP. Science 2009; 325(5948):1705-8; PMID:19696313; http://dx.doi.org/10.1126/science.1175570
- Fraser C, Donnelly CA, Cauchemez S, Hanage WP, Van Kerkhove MD, Hollingsworth TD, Griffin J, Baggaley RF, Jenkins HE, Lyons EJ, et al. Science 2009; 324(5934):1557-61; PMID:19433588; http://dx.doi.org/10.1126/science.1176062
- Adams B, Sasaki A. Theor Popul Biol 2009; 76(3):157-67; PMID:19501606; http://dx.doi.org/10.1016/j.tpb.2009.06.001
- Adams B, Sasaki A. Math Biosci 2007; 210(2):680-99; PMID:17904167; http://dx.doi.org/10.1016/j.mbs.2007.08.001
- Gomes MGM, White LJ, Medley GF, J Theor Biol 2005; 236(1):111-3; PMID:15967188; http://dx.doi.org/10.1016/j.jtbi.2005.03.001
- Yassine HM, Boyington JC, McTamney PM, Wei C-J, Kanekiyo M, Kong W-P, Gallagher JR, Wang L, Zhang Y, Joyce MG, et al. Nature Medicine 2015; 21: 1065-70.
- Impagliazzo A, Milder F, Kuipers H, Wagner M, Zhu X, Hoffman RMB, van Meersbergen R, Huizingh J, Wanningen P, Verspuij J, et al. Science 2015; 349 (6254): 1301-6.
- Bogner P, Capua I, Lipman DJ, Cox NJ, others. Nature 2006; 442(7106):981-981; http://dx.doi.org/10.1038/442981a
- Neher RA, Bedford T. Bioinformatics 2015 (btv381); PMID:26115986
- Bahl J, Nelson MI, Chan KH, Chen R, Vijaykrishna D, Halpin RA, Stockwell TB, Lin X, Wentworth DE, Ghedin E, et al. Proc Nat Acad Sci 2011; 108 (48):19359-64; PMID:22084096; http://dx.doi.org/10.1073/pnas.1109314108
- Koel BF, Burke DF, Bestebroer TM, van der Vliet S, Zondag GCM, Vervaet G, Skepner E, Lewis NS, Spronken MIJ, Russell CA, et al. Science 2013; 342(6161):976-9; PMID:24264991; http://dx.doi.org/10.1126/science.1244730
- Luksza M, Lassig M. Nature 2014; 507(7490):57-61; PMID:24572367; http://dx.doi.org/10.1038/nature13087
- Fonville JM, Wilks SH, James SL, Fox A, Ventresca M, Aban M, Xue L, Jones TC, L. N. M. H., P. Q. T., et al. Science 2014; 346(6212):996-1000; PMID:25414313; http://dx.doi.org/10.1126/science.1256427
- Cobey S. Ann Ny Acad Sci 2014; 1320(1):1-15; PMID:25040161; http://dx.doi.org/10.1111/nyas.12493
- Gillespie DT. J Phys Chem 1977; 81(25):2340-61; http://dx.doi.org/10.1021/j100540a008