ABSTRACT
Globally, malaria is a public health concern, with severe malaria (SM) contributing a major share of the disease burden in malaria endemic countries. In this context, identification and validation of SM biomarkers are essential in clinical practice. Some biomarkers (C-reactive protein, angiopoietin 2, angiopoietin-2/1 ratio, platelet count, histidine-rich protein 2) have yielded interesting results in the prognosis of Plasmodium falciparum severe malaria, but for severe P. vivax and P. knowlesi malaria, similar evidence is missing. The validation of these biomarkers is hindered by several factors such as low sample size, paucity of evidence-evaluating studies, suboptimal values of sensitivity/specificity, poor clinical practicality of measurement methods, mixed Plasmodium infections, and good clinical value of the biomarkers for concurrent infections (pneumonia and current COVID-19 pandemic). Most of these biomarkers are non-specific to pathogens as they are related to host response and hence should be regarded as prognostic/predictive biomarkers that complement but do not replace pathogen biomarkers for clinical evaluation of SM patients. This review highlights the importance of research on diagnostic/predictive/therapeutic biomarkers, neglected malaria species, and clinical practicality of measurement methods in future studies. Finally, the importance of omics technologies for faster identification/validation of SM biomarkers is also included.
Introduction
Tremendous efforts are being made worldwide to fight against malaria, but the disease remains an important public health concern, with 241 million cases and 627,000 deaths estimated in 2020 [Citation1]. Malaria is caused by a Plasmodium protozoan parasite transmitted to humans through the infectious bite of female Anopheline mosquitoes during a blood meal. Five Plasmodium species can elicit human malaria viz. P. falciparum (Pf), P. vivax (Pv), P. malariae (Pm), P. ovale spp (Po), and P. knowlesi (Pk), with Pf and Pv responsible for the major bulk of malaria burden worldwide [Citation1,Citation2].
The clinical spectrum of malaria is diverse, going from asymptomatic carriage of parasites to clinical malaria with varying degrees of signs/symptoms that define non-severe and severe malaria [Citation3]. A large number of factors (e.g. host genetics, immune status, drug resistance phenotype, host behavior, and concurrent infections) in the complex parasite—host—environment interaction modulate the clinical symptomatology in severe malarious patients [Citation3]. Severe malaria (SM) is more frequently seen in patients infected with Pf and Pv [Citation3], but recent reports have shown the ability of Pm, Po, and Pk to also elicit SM [Citation4–6].
In clinical practice, a good management of SM is achievable in endemic areas. However, SM management is very tricky in some areas, especially those from sub-Saharan Africa (sSA) that have a proportionally high share (>90%) of world malaria morbidity and mortality [Citation1], with a large number of remote areas and hard-to-reach populations. Skilled care providers, efficient good quality diagnostic tools, and antimalarial drugs are often missing in these areas, and thus, the management of SM is very challenging in such areas. In Sudan and Zimbabwe – two sSA countries – it was seen that the management of SM was suboptimal in the hospitals especially due to shortage of i) care providers, ii) availability of supplies (diagnostic test and treatment), and iii) the quality of care provided [Citation7,Citation8].
SM mainly due to Pf accounts for ~500,000 deaths annually, where children aged below five years old, pregnant women, and nonimmune travelers are major victims [Citation9]. However, there is paucity of data on the real extent of SM in endemic areas mostly [Citation10]. Using a systematic review and meta-analysis, we have shown that the overall proportion of Pv mono-infection-related SM is 22.9% in India [Citation11]. A recent modeling study showed the cost-effectiveness of implementing interventions aiming at reducing SM in conjunction with standard measures for reducing costs and health burden due to malaria [Citation12].
In the present review, we evaluated clinical biomarkers for their potential in the diagnosis, prognosis, prediction, and therapy of SM, identified the potential gaps, and proposed areas for future investigations/solutions, with a focus on omics technologies and their possible utility in the current research era.
Severe malaria
Since the 1990s, the World Health Organization (WHO) defined guidelines to diagnose SM based on findings from field and clinical studies. These guidelines have been regularly revised from 2000 to 2015 [Citation13–18]. For SM diagnosis, the clinical symptoms mainly include severe malarial anemia (SMA), cerebral malaria (CM), hypoglycemia, multiple convulsions, prostration, acute respiratory distress syndrome (ARDS), pulmonary edema, acute renal failure, multiorgan dysfunction, jaundice, shock, bleeding, and hyperparasitemia ( and ). In malarious patients, several of these symptoms can exist together, leading to death within hours or days [Citation19], and can be strong predictors in SM patients' recovery/survival [Citation20]. Few studies that addressed the risk factors of SM listed some frequently identified factors as distance to the nearest health facility, concurrent comorbidities, nonuse of preventive methods, immune status, pregnancy, duration of illness before receiving antimalarial drug, delayed care, seeking self-medication, and patients' age [Citation21–28]. For instance, Xia and colleagues have recently found that the risk of SM was higher in females and patients' aged ≥50 years old in the Hubei Province, a low malaria endemic area in China [Citation28]. Few unusually addressed risk factors such as ethnicity have also been identified among Pf-infected adults [Citation29].
Figure 1. Latest WHO guidelines on the clinical presentation of severe malaria [Citation18].
![Figure 1. Latest WHO guidelines on the clinical presentation of severe malaria [Citation18].](/cms/asset/b5cc3603-4219-409a-bb12-19bd3a12df36/kvir_a_2056966_f0001_oc.jpg)
Figure 2A. Worldwide burden of severe malaria.
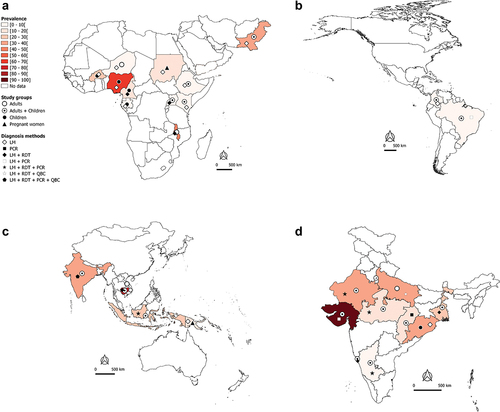
Worldwide burden of severe malaria in hospital-admitted patients
In the African and Eastern Mediterranean regions, SM may account for 40–50% of hospital-admitted malaria cases in countries such as Burkina Faso, Malawi, and Pakistan (). In Nigeria and Cambodia, the prevalence of hospital admitted SM due to Plasmodium regardless of species is very high (>70%) in clinical malaria cases with majority being Pf cases (). Data available and presented in suggest that Pf is mainly responsible for hospital admitted SM cases in sSA regions. In the Americas, the SM prevalence is relatively low (<10%), with Pf and Pv both involved in hospital admitted SM cases, but with a clear predominance of Pv in Brazil and Colombia (). In the South East Asia (SEA) region, the bulk of SM data comes from India. The extent of involvement of Pf and Pv in SM cases varies between the different states of the country. In Orissa, Pf-SM burden is the highest (40–50% of the malaria cases) as compared to other states (), whereas Pv species is predominantly responsible for hospital admitted SM cases in several states, including Delhi, Gujarat, Maharashtra, Madhya Pradesh, Uttar Pradesh, Rajasthan, and West Bengal ().
The need for clinical biomarkers of severe malaria
A biomarker is a characteristic presentation (e.g. clinical, genetic, and hematological) or a substrate (e.g. proteins and metabolites) that can objectively be measured and evaluated as an indicator of normal biological/pathogenic processes or response to a therapeutic intervention [Citation30]. In malariology, it can be particularly helpful in clinical practice and public health from disease diagnosis to identification of patients at risk for long-term complications ().
Biomarkers can be classified into four types viz diagnostic, prognostic, predictive, and therapeutic. Generally, a diagnostic biomarker allows the early detection of a disease in a noninvasive way, leading to its prevention. A prognostic biomarker is a clinical or biological characteristic that is objectively measurable and that provides information on the likely outcome of the disease in an untreated individual [Citation31–33]. A predictive biomarker is a clinical or biological characteristic that reveals information, allowing one to forecast the response of patients to a given treatment and thus identify individuals who will benefit from the treatment [Citation31–33]. A therapeutic biomarker is most commonly a protein that can be used as a target for disease therapy ().
In clinical practice, prognostic markers can allow one i) to discriminate untreated asymptomatic malaria patients susceptible to manifest clinical signs/symptoms, ii) identify untreated clinical patients whose malaria infection could lead to a severe form, and iii) identify untreated SM patients (). Thus, the identification of such markers for SM is of utmost importance as they will facilitate in the reduction of SM burden.
Evaluation and validation of clinical biomarkers
The characteristics of an ideal biomarker have been described previously with the following attributes: i) clinical relevance, ii) good sensitivity, specificity, and predictive values, iii) reliability, iv) simplicity, and v) practicality [Citation33,Citation34].
The clinical relevance of a biomarker refers to the evidence that supports a coherent basis of its utility (e.g. the ability of a biomarker to reflect any aspect of the pathological process, in this context, SM). Sensitivity refers to the ability of a biomarker to correctly identify patients with the disease-related outcome (e.g. mortality risk and severity outcome), while specificity refers to the capacity of a biomarker to correctly identify patients exempted from the disease-related outcome. The determination of the area under curve (AUC) of the biomarker by plotting a receiver operating characteristic curve (ROC curve) can also be used as an alternative to sensitivity and specificity. In practice, a biomarker with sensitivity (Se) ≥85%/specificity (Sp) ≥75% or an AUC ≥0.75 is considered to be of good clinical utility [Citation35–37]. A reliable biomarker that can be quantifiable should have acceptable precision, accuracy, reproducibility, and robustness. The quantitative evaluation of the biomarker should be simple to perform (i.e. without the need for a skilled operator and costly equipment) and as least invasive as possible in order to obtain approval of patients and apparently healthy individuals. According to the nature of the biological sample used for quantifying the biomarker, The Ronald and Nancy Reagan Research Institute of the Alzheimer’s Association and the National Institute on Aging Working Group have categorized the invasive methods into three categories such as noninvasive (blood, urine, saliva, or buccal scrapings), moderately invasive (skin or rectal biopsies, cerebrospinal fluid-CSF, or bone marrow), and highly invasive (brain tissue) [Citation35]. These different criteria are used to evaluate the performances and clinical utility of biomarkers.
The validation of biomarkers is the guarantee underlying the subsequent production of high-quality research data on diagnosis, prognosis, and prediction of the SM outcome. The procedure for the validation of biomarkers is multifaceted and more complex than their evaluation where validation takes into account the above-mentioned criteria on evaluation along with additional aspects such as the study design (sample size, characteristics of cases/controls), reproducibility in varied settings, pharmacokinetics—pharmacodynamics data, establishment of the biomarker normal range, and biological plausibility [Citation33,Citation34].
Biomarkers for severe malaria human Plasmodium species
The literature analysis of the related topic showed very few studies that evaluated the prognostic potential of few biomarkers for SM. One study addressed the evaluation of the platelet count and plateletcrit as SM prognostic markers without any distinction of the involved malaria species [Citation38]. A cross-sectional study conducted on Indians aged ≥18 years evaluated the prognostic value of the platelet (PLT) count and plateletcrit to discriminate SM patients from non-SM patients. The authors found that a PLT of 50,000/µL had a sensitivity of 65.6%, a specificity of 70.6%, and an AUC of 0.713. The values of sensitivity and specificity were similar for a plateletcrit of 0.05% but had an AUC of 0.718 [Citation38].
Biomarkers for severe Pf malaria
A large number of biomarker-related aspects have been extensively studied for this malarial species for the identification of i) patients at SM risk, ii) markers of SM outcomes, iii) patients at risk of CM, SMA, shock, ARDS/pulmonary edema, and renal failure, iv) patients with chances to recover from an SM episode, and v) CM patients at fatal risk or survival with the development of neurological sequelae (Supplemental material 1 and ). Most of the Pf biomarkers evaluated were host proteins ().
Figure 4. Clinical biomarkers and severe malaria.
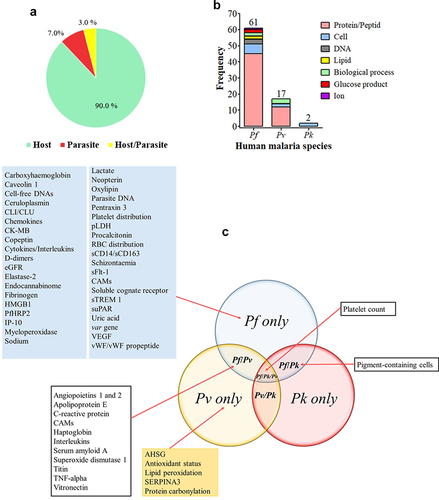
Figure 5. Biomarkers evaluated for severe Pf-SM.
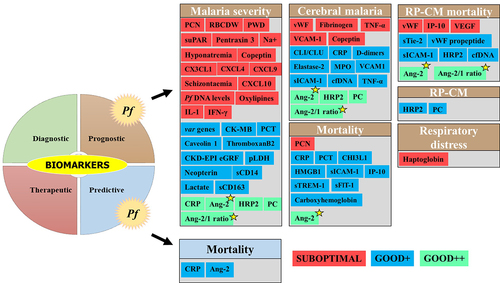
Among the Pf-SM biomarkers, five prognostic biomarkers viz CRP, Ang-2, Ang-2/1 ratio, PfHRP2, and platelet count showed good clinical values in malaria severity and CM. For example, CRP and platelet count exhibited interesting performances of malaria severity in nonimmune European patients as well as in individuals from malaria endemic areas (India, Sudan, Nigeria, Senegal, and Malawi) (Supplemental material 1 & ) [Citation39–44]. As reported for malaria severity and CM, the Ang-2 and Ang-2/1 ratio showed a good clinical value for the prognosis of mortality among SM cases and mortality among CM patients diagnosed with retinopathy [Citation45–47] (Supplementary file 1 and ). On analysis of these five prognostic biomarkers, PfHRP2 seems to be the most discriminant for CM, while Ang-2 and Ang-2/Ang-1 seem to be the best indicator for malaria severity. In addition, the Ang-2 and Ang-2/1 ratios seem to be the most promising prognostic biomarkers as they showed good performances for most of the SM-related outcomes (i.e. malaria severity, CM, mortality, and RP-CP mortality) ().
Other biomarkers that were included in the list have shown preliminary good performances, but the evidence of their clinical utility is still limited by the low number of studies, evaluation of specific populations only (i.e. nonimmune travelers), and the absence of statistical significance. Such biomarkers are procalcitonin (PCT), var genes, haptoglobin, neopterin, circulatory complement‑lysis inhibitor or clusterin (CLI/CLU), cardiac disease creatine kinase muscle-brain type (CK-MB), uric acid, chronic kidney disease-epidemiology estimated glomerular filtration rate (CKD-EPI eGFR), total bilirubin, caveolin 1, parasite lactate dehydrogenase (pLDH), high mobility group box protein 1 (HMGB1), oxylipin and endocannabidome metabolites, D-dimers, cell-free DNA (cfDNA), and carboxyhemoglobin (Supplemental material 1 and ). The prognostic value of CM biomarkers was also greatly influenced by the host immunity as good performances of cell adhesion molecules (VCAM-1, sICAM-1) were reported in European travelers, but in contrast, poor performances were found in Ugandan children [Citation48,Citation49].
Finally, biomarkers such as, for instance, pigment-contained neutrophils (PCNs), fibrinogen, copeptin, some chemokines (CX3CL1, CXCL4, CXCL9, and CXCL10), IP-10, and schizontaemia showed poor clinical performances for their ability to discriminate the above-mentioned SM outcomes ().
Biomarkers for severe Pv malaria
Several studies addressed biomarkers in Pv-SM, although the number of biomarkers evaluated is far lower than that seen in Pf-SM. To date, no strong evidence of the clinical utility of biomarkers is available for Pv-SM even though some of them such as superoxide dismutase 1 (SOD), titin, vitronectin, TNF-α, and the Ang-2/1 ratio have shown interesting performances for the prognosis of malaria severity (). Other CM biomarkers (IL-10, VCAM-1, TNF-α, and Ang-2) for potential prognosis were seen in studies from Brazil, India, and Pakistan [Citation50–52]. In contrast, poor performances, especially for malaria severity prognosis, were reported for other biomarkers, including IL-6, haptoglobin, apolipoprotein E, serum amyloid A, Ang-1, ICAM-1, and platelet count (Supplemental material 2 and ).
Figure 6. Biomarkers evaluated for severe Pv and Pk malaria.
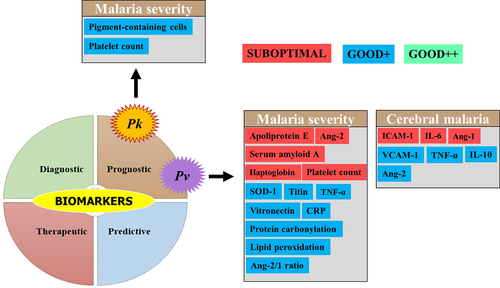
Biomarkers for severe Pk malaria
Plasmodium knowlesi is mostly found in SEA especially in Malaysia where its prevalence and burden often outdo those of Pf species [Citation5]. Platelet count and PCN were evaluated for their prognostic value toward SM in Malaysian adults and were found to show similar performances between the platelet count (AUC = 0.77) and PCN (AUC = 0.85) compared to the parasite density (AUC >0.80) (Supplemental material 2) [Citation53]. Another study found that plasma Ang-2 and osteoprotegerin levels were significantly higher in the plasma levels of Malaysian SM patients aged below 18 years old, and these two proteins independently were risk factors for renal impairment, despite the fact that the authors did not evaluate their malaria severity-related sensitivity and specificity [Citation54]. Despite these encouraging results, evidence of their clinical utility for Pk-SM is still insufficient ().
Biomarkers proposed for severe Pm and Po malaria
There are no studies for the evaluation of SM biomarkers for these two species, and this is likely due to a very low number of Po- and Pm-related morbidity and mortality cases, worldwide [Citation4,Citation6]. Based on a systematic review and meta-analysis, our research group has recently shown that the global prevalence of these two species was 2.01% for Pm and 0.77% for Po spp, with the highest values recorded in sSA (3.16% for Pm and 1.69% for Po) [Citation55].
SM biomarkers' critical evaluation, challenges, and future research
The evaluation and validation of reliable biomarkers for SM are crucial to develop efficient strategies to manage this important public concern worldwide. In the present review, it is obvious that this research era is still in its infancy, given the paucity of data on potential biomarkers. The studies were mainly focused on the prognostic aspect of the evaluated biomarkers rather than on their predictive and therapeutic aspects. The forecasting of the probable occurrence of SM is important, but the identification of patients with less chances of recovery from an antimalarial therapy (i.e. predictive biomarkers) and identification of biomolecules susceptible to be targeted by this therapy (i.e. therapeutic biomarkers) are also important aspects of management of SM by practitioners. Some studies outlined an association of elevated levels of biomarkers such as Ang-1, sTREM-1, CXCL10, and sICAM-1, with prolonged clinical recovery times in patients having survived from Pf-SM disease [Citation56], while other putative biomarkers were not found to be associated with Pf-SM-related clinical recovery and time [Citation57]. Despite the fact that such findings were reported in some studies, it would be premature to conclude a possible use of these biomolecules as potential predictive and/or therapeutic biomarkers as the studies were not properly designed to address these aspects. We noted that some studies concluded on a possible value of some biomarkers based on the statistical evidence of a difference in body fluid levels or levels of genomic expression between their different groups (e.g. healthy control – HC, uncomplicated malaria – UM, mild malaria – MM, severe malaria – SM, and cerebral malaria – CM) or an OR-based quantification of SM risk or mortality and have not given any estimates of sensitivity, specificity, predictive values, and AUC. These parameters should be given impetus by research community and practitioners for effective clinical impact of the putative biomarkers.
Some biomarkers have been found to produce relatively good and statistically significant prognostic performances in terms of AUC, sensitivity, and specificity, which include i) CRP, circulatory complement‑lysis inhibitor, Ang-2, HRP2, thromboxane 2, cfDNA, platelet count, TNF-α, and 10-kDa INF gamma-induced protein for Pf and ii) SOD-1, TNF-α, protein carbonylation, lipid peroxidation, IL-10, and cell adhesion molecules for Pv (Supplementary files 1 2, ). Unfortunately, the findings presented come either from only one study or from several studies of the same research group or from the same geographical setting, thereby limiting the evidence. In addition, the design of studies was not comparable and some studies addressed different aspects of SM (i.e. malaria severity, CM only, mortality, etc.). The demonstration of the usefulness of a given biomarker requires the implementation of more evidence-providing studies in different contexts and by different research teams. Case—control designed studies are better than retrospective and cross-sectional studies to evaluate any potential clinical biomarker. The evaluation of the behavior of biomarkers in other malaria vulnerable groups such as pregnant women and immunocompromised people other than children is also encouraged as they are majorly affected by malaria disease, especially SM.
On studying individually, we noted that a large proportion of biomarkers did not qualify as prognostic markers for SM, as no evidence of statistical significance or performance criteria values below the acceptable thresholds was available. One way to improve the potential of biomarkers could be to use them in combination as seen in previous studies [Citation45,Citation58–63]. Many reasons can explain poor individual performances of these biomarkers:
small sample size of cases and controls impacting greatly on the data analysis, statistical test findings, and finally the interpretation of data. Thus, the implementation of adequately powered studies is a prerequisite to support the findings;
findings on the accuracy of methods used to determine the levels of biomarkers in biological fluids are rarely given in the studies and absence of taking into account this aspect may give prejudices to results;
the presence of latent concurrent infections such as viral, parasitic, and bacterial infections or conditions like malnutrition, which also may modulate the levels of biomarkers investigated and severity of malaria disease. Few studies used concurrent infections such as dengue to identify and evaluate the potential of some biomarkers for severe Pv malaria [Citation64,Citation65]. Other studies showed a good prognostic value of Hp and Lpc-2 to distinguish severe pneumonia due to malaria from those of bacterial and viral origin [Citation58,Citation66], while few studies found CRP to be a good biomarker in the current coronavirus pandemic (COVID-19) [Citation67,Citation68].
The validation of SM biomarkers should also overcome the problem of mixed infections with plasmodial species. In some settings, the proportion of co-infections is often surprisingly high as reported for Pf-Pm co-infection from North-Western part of Cameroon [Citation69]. The proportion of mixed Plasmodium infections is also increasing in sSA as reported recently in a meta-analysis where 9% of mixed infections led to SM and that the proportion of pulmonary complications and severe anemia were higher in patients with mixed infections [Citation70]. Even though low proportions of mixed infections are reported from malaria endemic settings, whether these mixed infections influence the biological dynamics and usefulness of biomarkers remains an elusive question still.
The PfHRP2 protein has shown good performances for CM prognosis and distinction between CM malaria patients with positive and negative diagnosis for retinopathy (CM-RP and CM-RN) (Supplementary file 1 and ). The recent reports on the appearance of Pf parasites with pfhrp2 deletions could be an important concern on the long-term utilization of PfHRP2 as a diagnostic biomarker for Pf infections where it is highly predominant in settings as in sSA and some areas of India [Citation71–73] and could also be an obstacle to its utilizationin the prognosis of CM and distinction between CM-RP and CM-RN cohorts.
The Pk species is majorly present in SEA, especially in Malaysia where its prevalence is very high in some areas of the country [Citation74–76]. For severe Pk malaria, no promising biomarkers have been reported till now. Some putative biomarkers of Pk malaria infection such as Hpx, haptoglobin, and serotransferrin were proposed [Citation77], and it would be interesting to evaluate their potential in severe Pk malaria.
Even though a good biomarker needs to be validated for SM, the clinical practicality of the methods used to measure this biomarker is also a crucial parameter. ELISA is commonly used to measure the putative biomarkers along with other methods including molecular methods (i.e. real-time PCR, genotyping/cloning), flow cytometry, light microscopy, immunocolorimetric assays, co-oximetry, and indirect potentiometry (Supplemental materials 1 and 2). Routine implementation of these tests at clinical sites is greatly jeopardized by drawbacks such as high cost, time consumption, extensive training/expertise/workload for clinical staff, sensitivity/specificity, and reproducibility (). It would be interesting to address the cost-effectiveness of these different methods and develop point-of-care technologies in the context of biomarkers for SM, especially in resource-constrained and remote areas.
Table 1. Comparison of quantitative methods of SM biomarkers.
The few potentially helpful biomarkers identified here are mainly related to host response and they are therefore more likely suitable as prognostic and/or predictive biomarkers that complement but do not replace or substitute for diagnostics in the clinical evaluation and management of malaria patients. Biomarkers of host response are pathogen non-specific as mentioned in bacterial and COVID-19 infections and do not indicate the pathogen responsible for the clinical syndrome of “sepsis,” as represented by SM. As such, the biomarkers of host response provide insight into the potential risk for end-organ injury and adverse clinical outcomes. For example, biomarkers can potentially provide risk of progression to SM upon presentation of a patient with malaria (e.g. Ang-2).
The investigation of new potentially promising candidate biomarkers is also crucial, and with the advent of transcriptomic, peptidomic, and proteomic technologies, the process of identification, evaluation, and validation of biomarkers for different aspects of malaria severity as seen in previous studies can be accelerated [Citation64,Citation65,Citation78–80] (). In , some candidate biomarkers such as apolipoprotein A-I, hemopexin (Hpx), apolipoprotein E, retinol-binding protein 4 (RBP4), ceruloplasmin, and plasminogen were identified using these technologies and further validated through estimates of their performances (i.e. sensitivity, specificity, predictive values, and AUC) using adequately designed studies. The cost, complexity, and time-consuming nature of omics technologies are major obstacles to their real-time clinical application in malaria settings, which are predominantly resource-limited areas. Another crucial challenge in the usage of such advanced technologies is to be able to translate “omics” technology-identified potential biomarkers into a relatively simple, rapid, reliable, low-cost assay (e.g. immunoassay) and make it commercially available in malaria endemic regions.
Figure 7. Utility of omics technologies to accelerate the identification and validation of clinical biomarkers for SM.
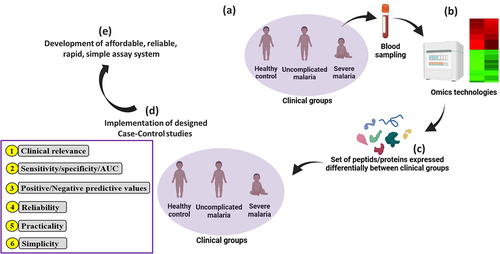
Table 2. Some proposed severe malaria biomarkers for which clinical performances were not evaluated.
Conclusion
The present review concluded that the identification and validation of biomarkers of SM are still in an early phase. Some candidate biomarkers seem to be promising for SM prognosis, but further studies are still required to confirm their prognostic value. Also, this review presented different aspects for which the prognostic value of candidate biomarkers was evaluated and summarized these findings from different studies. The authors also point out the need for further studies/research in the identification of predictive and therapeutic biomarkers, which are other critical links to be addressed for efficient control of SM. Although prognostic marker validation is relatively easy, more stringent criteria are required for the validation of predictive and therapeutic biomarkers for SM. Also, the concurrent and mixed Plasmodium infections are major obstacles for a better understanding of the relationship between the candidate biomarker and SM, and their evaluation as a potential biomarker needs to be carried out in further research studies. The advent of omics technologies would also be helpful in enhancing the identification and validation of clinical SM biomarkers, but affordable alternative assay systems need to be developed for translation in the clinical context of malaria.
Authors’ contributions
AS and VS designed the study. LPKF, GN, ST, JJ, and JH conducted literature review and extracted analyzed data from papers. LPKF and JH conceived the figures and maps. LPKF supervised the literature review stage, data extraction, and analysis and finalized the first version of the final manuscript with the help of GN, ST, JJ, and JH. AS and VS critically revised the paper for important intellectual content and supervised the work at all stages. All authors read and approved the final version of the paper.
Abbreviations
AMDA | = | Asymmetric dimethylarginine |
AHSG | = | Alpha-2-Heremans-Schmid Glycoprotein |
Ang | = | Angiopoietin |
ARDS | = | Acute respiratory distress syndrome |
AUC | = | Area under the curve |
BAFF | = | B-cell-activating factor |
CAMs | = | Cell adhesion molecules |
CCL | = | Chemokine ligand |
CHI3L1 | = | Chitinase-3-like 1 protein |
CI | = | Confidence interval |
CM | = | Cerebral malaria |
CMNS | = | Cerebral malaria nonsurvivors |
CMS | = | Cerebral malaria survivors |
CP | = | Convalescent patients |
CRP | = | C-reactive protein |
crt-o | = | Chloroquine resistance transporter-Orthologue |
CSF | = | Cerebrospinal fluid |
DNA | = | Deoxyribonucleic acid |
ECs | = | Endothelial cells |
ELISA | = | Enzyme-linked immunosorbent assay |
EPCR | = | Endothelial protein C receptor |
Epo | = | Erythropoietin |
EVs | = | Extracellular vesicles |
Gal-9 | = | Galectin-9 |
GrzB | = | Granzyme B |
Hb | = | Hemoglobin |
HC | = | Healthy control |
Hct | = | Hematocrit |
HMGB1 | = | High-mobility group box protein 1 |
Hp | = | Haptoglobin |
Hpx | = | Hemopexin |
HRP2 | = | Histidine-rich protein 2 |
IFN | = | Interferon |
IL | = | Interleukin |
IP-10 | = | 10-kDa INF gamma-induced protein |
LDR-FMA | = | Ligase detection reaction-fluorescent microsphere assay |
LM | = | Light microscopy |
LODS | = | Lambaréné Organ Dysfunction Score |
mdr1 | = | Multidrug resistance gene 1 |
MM | = | Mild malaria |
MP | = | Microparticles |
NLR | = | Negative likelihood ratio |
NPV | = | Negative predictive value |
OR | = | Odd ratio |
PA | = | Pantetheinase |
PD | = | Parasite density |
PCM/PCN | = | Pigment-containing monocytes/neutrophils |
PCR | = | Polymerase chain reaction |
PCT | = | Procalcitonin |
Pf | = | Plasmodium falciparum |
PfEMP1 | = | Pf erythrocyte membrane protein 1 |
Pk | = | Plasmodium knowlesi |
Pm | = | Plasmodium malariae |
Po | = | Plasmodium ovale |
Pv | = | Plasmodium vivax |
PLR | = | Positive likelihood ratio |
PPV | = | Positive predictive value |
PWD | = | Platelet width distribution |
QBC | = | Quantitative buffy coat |
RBCDW | = | Red blood cell distribution width |
RBP4 | = | Retinol-binding protein 4 |
RD | = | Respiratory distress |
RDT | = | Rapid diagnostic test |
RN | = | Retinopathy negative |
RNA | = | Ribonucleic acid |
RP | = | Retinopathy positive |
ROC | = | Receiver operating characteristic |
SBP | = | Systolic blood pressure |
sICAM-1 | = | Soluble intercellular cell adhesion molecule 1 |
Se | = | Sensitivity |
SEA | = | South East Asia |
SERPINA3 | = | Serpin peptidase inhibitor clade A member 3 |
SM | = | Severe malaria |
SMA | = | Severe malarial anemia |
Sp | = | Specificity |
sSA | = | Sub-Saharan Africa |
sTie-2 | = | Soluble cognate receptor |
suPAR | = | Soluble urokinase-type plasminogen activator |
TGF | = | Transforming growth factor |
TNF | = | Tumor necrosis factor |
UM | = | Uncomplicated malaria |
VCAM-1 | = | Vascular adhesion molecule 1 |
VEGF | = | Vascular endothelial growth factor |
vWF | = | von Willebrand factor |
WHO | = | World Health Organization |
Supplemental Material
Download Zip (217.8 KB)Disclosure statement
No potential conflict of interest was reported by the author(s).
Data availability statement
All data included in this study are fully available from the corresponding author on reasonable request.
Supplementary material
Supplemental data for this article can be accessed here
Additional information
Funding
References
- WHO. World Malaria Report. Geneva: World Health Organization; 2021. Available from: https://www.who.int/publications/i/item/9789240015791
- Cowman AF, Healer J, Marapana D, et al. Malaria: biology and disease. Cell [Internet]. 2016;167(3):610–624. DOI:http://dx.doi.org/10.1016/j.cell.2016.07.055.
- Trampuz A, Jereb M, Muzlovic I, et al. Clinical review: severe malaria. Crit Care [Internet]. 2003;7(4):315–323. https://doi.org/10.1186/cc2183.
- Kotepui M, Kotepui KU, Milanez GD, et al. Severity and mortality of severe Plasmodium ovale infection: a systematic review and meta-analysis. PLoS One [Internet]. 2020;15(6):e0235014. http://dx.doi.org/10.1371/journal.pone.0235014.
- Kotepui M, Kotepui KU, Milanez GD, et al. Prevalence of severe Plasmodium knowlesi infection and risk factors related to severe complications compared with non-severe P. knowlesi and severe P. falciparum malaria: a systematic review and meta-analysis. Infect Dis Pov [Internet]. 2020;9(1):106. https://doi.org/10.1186/s40249-020-00727-x.
- Kotepui M, Kotepui KU, Milanez GD, et al. Global prevalence and mortality of severe Plasmodium malariae infection: a systematic review and meta-analysis. Malar J [Internet]. 2020;19(1):274. https://doi.org/10.1186/s12936-020-03344-z.
- Makumbe B, Tshuma C, Shambira G, et al. Evaluation of severe malaria case management in Mazowe district, Zimbabwe, 2014. Pan Afr Med J [Internet]. 2017;27:33. DOI:10.11604/pamj.2017.27.33.11081.
- Elnour FA, Alagib MEA, Bansal D, et al. Severe malaria management: Current situation, challenges and lessons learned from Gezira State, Sudan. Malar J [Internet]. 2019;18(1):170. https://doi.org/10.1186/s12936-019-2805-z.
- WHO. World malaria report 2019 [Internet]. Geneva; 2019. Available from: www.who.int
- Taylor C, Namaste SML, Lowell J, et al. Estimating the fraction of severe malaria among malaria-positive children: analysis of household surveys in 19 malaria-endemic countries in Africa. Am J Trop Med Hyg [InternetAhead of print]. 2021;104(4):1375–1382. https://doi.org/10.4269/ajtmh.20-1351.
- Kojom Foko LP, Arya A, Sharma A, et al. Epidemiology and clinical outcomes of severe Plasmodium vivax malaria in India. J Infect. 2021;82(6):231–246. https://doi.org/10.1016/j.jinf.2021.03.028.
- Greenhalgh S, Chandwani V. Advocating an attack against severe malaria: a cost-effectiveness analysis. BMC Public Health. 2020;20(1):17.
- WHO. Severe falciparum malaria. Trans R Soc Trop Med Hyg. 2000;94:S1–S90.
- WHO. Guidelines for treatment of malaria. Geneva: The World health Organization; 2006.
- WHO. Guidelines for the treatment of malaria [Internet]. Second Edition. Geneva; 2010. Available from: https://www.ncbi.nlm.nih.gov/books/NBK254223/pdf/Bookshelf_NBK254223.pdf
- WHO. Management of severe malaria. 3rd ed. Geneva: World Health Organization; 2012.
- WHO. Severe malaria. Trop Med Int Health. 2014;19:7–131.
- WHO. Guidelines for the treatment of malaria - Third edition [Internet]. Geneva; 2015. Available from: https://www.ncbi.nlm.nih.gov/books/NBK294440/pdf/Bookshelf_NBK294440.pdf
- Sypniewska P, Duda JF, Locatelli I, et al. Clinical and laboratory predictors of death in African children with features of severe malaria: a systematic review and meta-analysis. BMC Med. 2017;15:147. Available from:
- Jallow M, Casals-Pascual C, Ackerman H, et al. Clinical features of severe malaria associated with death: a 13-year observational study in the Gambia. PLoS One. 2012;7(9):e45645. DOI:10.1371/journal.pone.0045645.
- Schwartz E, Sadetzki S, Murad H, et al. Age as a risk factor for severe Plasmodium falciparum malaria in nonimmune patients. Clin Infec Dis. 2001;33(10):1774–1777. https://doi.org/10.1086/322522.
- Barcus MJ, Basri H, Picarima H, et al. Demographic risk factors for severe and fatal vivax and falciparum malaria among hospital admissions in Northeastern Indonesian Papua. Am J Trop Med Hyg. 2007;77:984–991.
- Fried M, Duffy PE. Parasite burden and severity of malaria in Tanzanian children. N Engl J Med. 2014;370:1799–1808.
- Mpimbaza A, Ndeezi G, Katahoire A, et al. Demographic, socioeconomic, and geographic factors leading to severe malaria and delayed care seeking in Ugandan children: a case–control study. Am J Trop Med Hyg. 2017;97(5):1513–1523. https://doi.org/10.4269/ajtmh.17-0056.
- Mutsigiri-Murewanhema F, Mafaune PT, Shambira G, et al. Factors associated with severe malaria among children below ten years in Mutasa and Nyanga districts, Zimbabwe, 2014-2015. Pan Afr Med J. 2017;27:23.
- Khuu D, Eberhard ML, Bristow BN, et al. Risk factors for severe malaria among hospitalized patients in the United States, 2000–2014. Infect Dis Health. 2018;23(2):93–106. https://doi.org/10.1016/j.idh.2018.01.002.
- Kwan JL, Seitz AE, Fried M, et al. Seroepidemiology of helminths and the association with severe malaria among infants and young children in Tanzania. PLoS Negl Trop Dis. 2018;12(3):e0006345. https://doi.org/10.1371/journal.pntd.0006345.
- Xia J, Wu D, Wu K, et al. Epidemiology of Plasmodium falciparum malaria and risk factors for severe disease in Hubei Province, China. Am J Trop Med Hyg. 2020;103(4):1534–1539. https://doi.org/10.4269/ajtmh.20-0299.
- Phillips A, Bassett P, Zeki S, et al. Risk factors for severe disease in adults with falciparum malaria. Clin Infec Dis. 2009;48(7):871–878. https://doi.org/10.1086/597258.
- Lucchi NW, Jain V, Wilson NO, et al. Potential serological biomarkers of cerebral malaria. Dis Markers. 2011;31:327–335.
- Clark GM. Prognostic factors versus predictive factors : examples from a clinical trial of erlotinib 5. Mol Oncol. 2008;1(4):406–412.
- Italiano A. Prognostic or predictive? It’s time to get back to definitions! J Clin Oncol. 2011;29(35):4718.
- Hunter DJ, Losina E, Guermazi A, et al. A pathway and approach to biomarker validation and qualification for osteoarthritis clinical trials. Curr Drug Targets. 2010;11(5):536–545. https://doi.org/10.2174/138945010791011947.
- Lesko L, Atkinson A Jr. Use of biomarkers and surrogate endpoints in drug development and regulatory decision making: Criteria, validation, strategies. Annu Rev Pharmacol Toxicol. 2001;41(1):347–366.
- No author listed. Consensus Report of the Working Group on: “Molecular and Biochemical Markers of Alzheimer’s Disease”. Neurobiol Aging. 1998;19(2):109–116. DOI:10.1016/S0197-4580(98)00022-0.
- Akobeng AK. Understanding diagnostic tests 3: receiver operating characteristic curves. Acta Paediatr. 2007;96(5):644–647.
- Mandrekar JN. Receiver operating characteristic curve in diagnostic test assessment. J Thorac Oncol. 2010;5(9):1315–1316.
- Gupta P, Guddattu V, Saravu K. Characterization of platelet count and platelet indices and their potential role to predict severity in malaria. Pathog Glob Health. 2019;113(2):86–93.
- Mohapatra MK, Thomas GA, Kumar Bariha P, et al. Serum procalcitonin: as a Triage tool for severe Plasmodium falciparum malaria. J Trop Dis. 2013;1:4.
- Bhardwaj N, Ahmed M, Sharma S, et al. C-Reactive protein as a prognostic marker of Plasmodium falciparum malaria severity. J Vector Borne Dis. 2019;56:122–126.
- Gérardin P, Rogier C, Ka AS, et al. Prognostic value of thrombocytopenia in African children with falciparum malaria. Am J Trop Med Hyg. 2002;66:686–691.
- Righi E, Merelli M, Arzese A, et al. Determination of PCT on admission is a useful tool for the assessment of disease severity in travelers with imported Plasmodium falciparum malaria. Acta Parasitol. 2016;61(2):412–418. DOI:10.1515/ap-2016-0055.
- Salih MM, Eltahir HG, Abdallah TM, et al. Haematological parameters, haemozoin-containing leukocytes in Sudanese children with severe Plasmodium falciparum malaria. J Infect Dev Ctries. 2018;12:273–278.
- Oluboyo A, Chukwu S, Oluboyo B, et al. Evaluation of angiopoietins 1 and 2 in malaria-infested children. J Environ Public Health. 2020;2020:2169763.
- Erdman LK, Dhabangi A, Musoke C, et al. Combinations of host biomarkers predict mortality among Ugandan children with severe malaria: a retrospective case-control study. PLoS One. 2011;6(2):e17440. DOI:10.1371/journal.pone.0017440.
- Conroy AL, Phiri H, Hawkes M, et al. Endothelium-Based biomarkers are associated with cerebral malaria in Malawian children: a retrospective case-control study. PLoS One. 2010;5(12):e15291. DOI:10.1371/journal.pone.0015291.
- Conroy AL, Glover SJ, Hawkes M, et al. Angiopoietin-2 levels are associated with retinopathy and predict mortality in Malawian children with cerebral malaria: a retrospective case-control study. Cri Care Med. 2012;40(3):952–959. DOI:10.1097/CCM.0b013e3182373157.
- Stauga S, Hahn A, Brattig NW, et al. Clinical relevance of different biomarkers in imported Plasmodium falciparum malaria in adults: a case control study. Malar J. 2013;12(1):246. DOI:10.1186/1475-2875-12-246.
- Park GS, Ireland KF, Opoka RO, et al. Evidence of endothelial activation in asymptomatic Plasmodium falciparum parasitemia and effect of blood group on levels of von Willebrand factor in Malaria. J Pediatric Infect Dis Soc. 2012;1(1):16–25. DOI:10.1093/jpids/pis010.
- Andrade BB, Reis-Filho A, Souza-Neto SM, et al. Plasma superoxide dismutase-1 as a surrogate marker of vivax malaria severity. PLoS Med. 2010;4:e650.
- Jain V, Lucchi NW, Wilson NO, et al. Plasma levels of angiopoietin-1 and -2 predict cerebral malaria outcome in Central India. Malar J [ Available from]. 2011;10(1):383. DOI:10.1186/1475-2875-10-383.
- Raza A, Ghanchi NK, Zubairi ABS, et al. Tumor necrosis factor -α, interleukin-10, intercellular and vascular adhesion molecules are possible biomarkers of disease severity in complicated Plasmodium vivax isolates from Pakistan. PLoS One. 2013;8(12):e81363. DOI:10.1371/journal.pone.0081363.
- Willmann M, Ahmed A, Siner A, et al. Laboratory markers of disease severity in Plasmodium knowlesi infection: a case control study. Malar J. 2012;11(1):363. DOI:10.1186/1475-2875-11-363.
- Barber BE, Grigg MJ, Piera KA, et al. Intravascular haemolysis in severe Plasmodium knowlesi malaria: association with endothelial activation, microvascular dysfunction, and acute kidney injury. Emerg Microbes Infect. 2018;7(1):106. DOI:http://dx.doi.org/10.1038/s41426-018-0105-2.
- Hawadak J, Dongang Nana RR, Singh V. Global trend of Plasmodium malariae and Plasmodium ovale spp. malaria infections in the last two decades (2000–2020): a systematic review and meta-analysis. Parasite Vectors. 2021;14(1):297.
- Conroy AL, Hawkes M, McDonald CR, et al. Host biomarkers are associated with response to therapy and long-term mortality in pediatric severe malaria. Open Forum Infect Dis. 2016;3(3):ofw134. DOI:10.1093/ofid/ofw134.
- Yeo TW, Lampah DA, Kenangalem E, et al. Increased carboxyhemoglobin in adult falciparum malaria is associated with disease severity and mortality. J Infect Dis. 2013;208(5):813–817. DOI:10.1093/infdis/jit253.
- Huang H, Ideh RC, Gitau E, et al. Discovery and validation of biomarkers to guide clinical management of pneumonia in African children. Clin Infect Dis. 2014;58(12):1707–1715. DOI:10.1093/cid/ciu202.
- Mahanta A, Kar SK, Kakati S, et al. Heightened inflammation in severe malaria is associated with decreased IL-10 expression levels and neutrophils. Innate Immun. 2015;21(5):546–552. DOI:10.1177/1753425914561277.
- Tahar R, Albergaria C, Zeghidour N, et al. Plasma levels of eight different mediators and their potential as biomarkers of various clinical malaria conditions in African children. Malar J. 2016;15(1):337. DOI:10.1186/s12936-016-1378-3.
- Vera IM, Kessler A, Ting LM, et al. Plasma cell-free DNA predicts pediatric cerebral malaria severity. JCI Insight. 2020;5(12):e136279. https://doi.org/10.1172/jci.insight.136279.
- Duffy F, Bernabeu M, Babar PH, et al. Meta-analysis of Plasmodium falciparum var signatures contributing to severe malaria in African children and Indian adults. Mbio. 2019;10(2):e00217–19. https://doi.org/10.1128/mBio.00217-19.
- Imwong M, Woodrow CJ, Hendriksen ICE, et al. Plasma concentration of parasite DNA as a measure of disease severity in falciparum malaria. J Infect Dis. 2015;211(7):1128–1133. DOI:10.1093/infdis/jiu590.
- Ray S, Patel SK, Venkatesh A, et al. Clinicopathological analysis and multipronged quantitative proteomics reveal oxidative stress and cytoskeletal proteins as possible markers for severe vivax malaria. Sci Rep. 2016;6(1):24557. DOI:http://dx.doi.org/10.1038/srep24557.
- Kumar V, Ray S, Aggarwal S, et al. Multiplexed quantitative proteomics provides mechanistic cues for malaria severity and complexity. Commun Biol. 2020;3(1):683. DOI:http://dx.doi.org/10.1038/s42003-020-01384-4.
- Öner D, Drysdale SB, McPherson C, et al. Biomarkers for disease severity in children infected with respiratory syncytial virus: a systematic literature review. J Infect Dis. 2020;222:S648–S657.
- Danwang C, Endomba FT, Nkeck JR, et al. A meta-analysis of potential biomarkers associated with severity of coronavirus disease 2019 (COVID-19). Biomarker Res. 2020;8(1):37. DOI:10.1186/s40364-020-00217-0.
- Samprathi M, Jayashree M. Biomarkers in COVID-19: an up-to-date review. Front Pediatr. 2021;8:607647.
- Achonduh-Atijegbe O, Mbange AE, Atogho-Tiedeu B, et al. Predominance of Plasmodium malariae-falciparum co-infection by molecular speciation in Bangolan, North West Region of Cameroon. J Life Sci. 2013;7:599–606.
- Kotepui M, Kotepui KU, De Jesus Milanez G, et al. Plasmodium spp. mixed infection leading to severe malaria: a systematic review and meta-analysis. Sci Rep. 2020;10(1):11068. https://doi.org/10.1038/s41598-020-68082-3.
- Kojom LP, Singh V. Prevalence of Plasmodium falciparum field isolates with deletions in histidine-rich protein 2 and 3 genes in context with sub-Saharan Africa and India: a systematic review and meta-analysis. Malar J [Internet]. 2020;19(1):46. https://doi.org/10.1186/s12936-019-3090-6.
- Singh V, Kojom LP. Deletions in the Plasmodium falciparum histidine-rich protein 2 gene: an emerging threat to the elimination of malaria in India. J Vector Borne Dis. 2019;56(1):85–86.
- Gendrot M, Fawaz R, Dormoi J, et al. Genetic diversity and deletion of Plasmodium falciparum histidine-rich protein 2 and 3: a threat to diagnosis of P. falciparum malaria. Clin Microbiol Infect. 2019;25(5):580–585. DOI:10.1016/j.cmi.2018.09.009.
- Cox-Singh J. Zoonotic malaria: Plasmodium knowlesi, an emerging pathogen. Curr Opin Infect Dis. 2012;25(5):530–536.
- William T, Jelip J, Menon J, et al. Changing epidemiology of malaria in Sabah, Malaysia: increasing incidence of Plasmodium knowlesi. Malar J. 2014;13(1):390. DOI:10.1186/1475-2875-13-390.
- Barber BE, Rajahram GS, Grigg MJ, et al. World malaria report: time to acknowledge Plasmodium knowlesi malaria. Malar J. 2017;16(1):135. DOI:10.1186/s12936-017-1787-y.
- Chen Y, Chan CK, Kerishnan JP, et al. Identification of circulating biomarkers in sera of Plasmodium knowlesi-infected malaria patients – comparison against Plasmodium vivax infection. BMC Infect Dis. 2015;15(1):49. DOI:10.1186/s12879-015-0786-2.
- Ray S, Kumar V, Bhave A, et al. Proteomic analysis of Plasmodium falciparum induced alterations in humans from different endemic regions of India to decipher malaria pathogenesis and identify surrogate markers of severity. J Proteomics. 2015;127:103–113.
- Lee HJ, Georgiadou A, Otto TD, et al. Transcriptomic studies of malaria: a Paradigm for investigation of systemic host-pathogen interactions. Microbiol Mol Biol Rev. 2018;82(2):1–37. DOI:10.1128/MMBR.00071-17.
- Moussa EM, Huang H, Thézénas ML, et al. Proteomic profiling of the plasma of Gambian children with cerebral malaria. Malar J. 2018;17(1):337. https://doi.org/10.1186/s12936-018-2487-y.
- Cortez MA, Bueso-Ramos C, Ferdin J, et al. MicroRnas in body fluids—the mix of hormones and biomarkers. Nat Rev Clin Oncol. 2011;8(8):467–477. DOI:10.1038/nrclinonc.2011.76.
- Chamnanchanunt S, Fucharoen S, Umemura T. Circulating microRnas in malaria infection: Bench to bedside. Malar J. 2017;16(1):334.
- Rangel G, Teerawattanapong N, Chamnanchanunt S, et al. Candidate microRnas as biomarkers in malaria infection: a systematic review. Curr Mol Med. 2019;20(1):36–43. DOI:10.2174/1566524019666190820124827.
- Gupta H, Rubio M, Sitoe A, et al. Plasma MicroRNA profiling of Plasmodium falciparum biomass and association with severity of malaria disease. Emerg Infect Dis. 2021;27(2):430–442. DOI:10.3201/eid2702.191795.
- Casals-Pascual C, Idro R, Gicheru N, et al. High levels of erythropoietin are associated with protection against neurological sequelae in African children with cerebral malaria. Proc Natl Acad Sci, USA. 2008;105(7):2634–2639. DOI:10.1073/pnas.0709715105.
- Dembele BP, Chagan-Yasutan H, Niki T, et al. Plasma levels of Galectin-9 reflect disease severity in malaria infection. Malar J. 2016;15(1):403. DOI:10.1186/s12936-016-1471-7.
- Kaminski L-C, Riehn M, Abel A, et al. Cytotoxic T cell-derived granzyme B is increased in severe Plasmodium falciparum malaria. Front Immunol. 2019;10:2917.
- Pankoui Mfonkeu JB, Gouado I, Fotso Kuaté H, et al. Elevated cell-specific microparticles are a biological marker for cerebral dysfunctions in human severe malaria. PLoS One. 2010;5(10):e13415. DOI:10.1371/journal.pone.0013415.
- Thonsranoi K, Glaharn S, Punsawad C, et al. Increased synapsin I expression in cerebral malaria. Int J Clin Exp Pathol. 2015;8(11):13996–14004.
- Rommelaere S, Millet V, Rihet P, et al. Serum pantetheinase/vanin levels regulate erythrocyte homeostasis and severity of malaria. Am J Pathol. 2015;185(11):3039–3052. DOI:http://dx.doi.org/10.1016/j.ajpath.2015.07.011.
- Cheng IS, Sealy BC, Tiberti N, et al. Extracellular vesicles, from pathogenesis to biomarkers: the case for cerebral malaria. Vessel Plus. 2020;4:17.
- Tolosano E, Altruda F. Hemopexin: Structure, function, and regulation. DNA Cell Biol. 2002;21(4):297–306.
- Elphinstone RE, Conroy AL, Hawkes M, et al. Alterations in systemic extracellular heme and hemopexin are associated with adverse clinical outcomes in Ugandan children with severe malaria. J Infect Dis. 2016;214(8):1268–1275. DOI:10.1093/infdis/jiw357.
- Orzheshkovskyi V, Trishchynska M. Ceruloplasmin: Its role in the physiological and pathological processes. Neurophysiol. 2019;51(2):141–149.
- Steinhoff JS, Lass A, Schupp M. Biological functions of RBP4 and its relevance for human diseases. Front Physiol. 2021;12:659977.
- Aisina RB, Mukhametova LI. Structure and function of plasminogen/plasmin system. Russ J Bioorg Chem. 2014;40(6):590–605.
- Vlad C, Burlacu A, Florea L, et al. A comprehensive review on apolipoproteins as nontraditional cardiovascular risk factors in end-stage renal disease: current evidence and perspectives. Int Urol Nephrol. 2019;51(7):1173–1189. https://doi.org/10.1007/s11255-019-02170-w.
- Dietmann A, Helbok R, Lackner P, et al. Endoglin in African children with Plasmodium falciparum malaria: a novel player in severe malaria pathogenesis? J Infect Dis. 2009;200(12):1842–1848. DOI:10.1086/648476.
- Nduati E, Gwela A, Karanja H, et al. The plasma concentration of the B cell activating factor is increased in children with acute malaria. J Infect Dis. 2011;204(6):962–970. DOI:10.1093/infdis/jir438.
- Wenisch C, Spitzauer S, Florris-Linau K, et al. Complement activation in severe Plasmodium falciparum malaria. Clin Immunol Immunopathol. 1997;85(2):166–171. DOI:10.1006/clin.1997.4417.
- Urban BC, Cordery D, Shafi MJ, et al. The frequency of BDCA3-positive dendritic cells is increased in the peripheral circulation of Kenyan children with severe malaria. Infect Immun. 2006;74(12):6700–6706. DOI:10.1128/IAI.00861-06.
- Harawa V, Njie M, Keller T, et al. Malawian children with uncomplicated and cerebral malaria have decreased activated Vγ9Vδ2 γδ T cells which increase in convalescence. PLoS One. 2019;14(10):e0223410. DOI:10.1371/journal.pone.0223410.
- Barber BE, William T, Grigg MJ, et al. Asymmetric dimethylarginine in adult falciparum malaria: relationships with disease severity, antimalarial treatment, hemolysis, and inflammation. Open Forum Infect Dis. 2016;3(1):ofw027. DOI:10.1093/ofid/ofw027.
- Barber BE, Grigg MJ, Piera KA, et al. Endothelial glycocalyx degradation and disease severity in Plasmodium vivax and Plasmodium knowlesi malaria. Sci Rep. 2021;11(1):9741. https://doi.org/10.1038/s41598-021-88962-6.
- Melo GC, Monteiro WM, Siqueira AM, et al. Expression levels of pvcrt-o and pvmdr-1 are associated with chloroquine resistance and severe Plasmodium vivax malaria in patients of the Brazilian Amazon. PLoS One. 2014;9:e105922.
- Buyon LE, Elsworth B, Duraisingh MT. The molecular basis of antimalarial drug resistance in Plasmodium vivax. Int J Parasitol Drugs Drug Resist. 2021;16:23–37.