ABSTRACT
Non-Hodgkin B-cell lymphoma (B-NHL) are aggressive lymphoid malignancies that develop in patients due to oncogenic activation, chemo-resistance, and immune evasion. Tumor biopsies show that B-NHL frequently uses several immune escape strategies, which has hindered the development of checkpoint blockade immunotherapies in these diseases. To gain a better understanding of B-NHL immune editing, we hypothesized that the transcriptional hallmarks of immune escape associated with these diseases could be identified from the meta-analysis of large series of microarrays from B-NHL biopsies. Thus, 1446 transcriptome microarrays from seven types of B-NHL were downloaded and assembled from 33 public Gene Expression Omnibus (GEO) datasets, and a method for scoring the transcriptional hallmarks in single samples was developed. This approach was validated by matching scores to phenotypic hallmarks of B-NHL such as proliferation, signaling, metabolic activity, and leucocyte infiltration. Through this method, we observed a significant enrichment of 33 immune escape genes in most diffuse large B-cell lymphoma (DLBCL) and follicular lymphoma (FL) samples, with fewer in mantle cell lymphoma (MCL) and marginal zone lymphoma (MZL) samples. Comparing these gene expression patterns with overall survival data evidenced four stages of cancer immune editing in B-NHL: non-immunogenic tumors (stage 1), immunogenic tumors without immune escape (stage 2), immunogenic tumors with immune escape (stage 3), and fully immuno-edited tumors (stage 4). This model complements the standard international prognostic indices for B-NHL and proposes that immune escape stages 3 and 4 (76% of the FL and DLBCL samples in this data set) identify patients relevant for checkpoint blockade immunotherapies.
KEYWORDS:
Abbreviations
CLL | = | chronic lymphocytic leukemia |
DLBCL | = | diffuse large B-cell lymphoma |
DLBCL ABC | = | activated B-cell subtype of DLBCL |
DLBCL GCB | = | germinal center B-cell subtype of DLBCL |
FL | = | follicular lymphoma |
KS | = | Kolmogorov–Smirnov |
SES | = | sample enrichment score |
ssGSEA | = | single sample gene set enrichment analysis |
W | = | Wilcoxon |
Introduction
Cancers must evolve strategies of immune escape to develop within immunocompetent hosts. In B-NHL, genetic and epigenetic alterations create tumor phenotypes that are protected against immune cytolysis.Citation1-7 Immune escape also evolves through the concerted upregulation of gene expression.Citation8 For example, the B-NHL subtypes FL and DLBCL upregulate the expression of genes involved in the PD1/PDL1-2 axis, the CTLA4 ligand axis, the biosynthesis of immunosuppressive Galectin 3, and the genes IDO1, VEGFA, PGE2, IL10, and HGF, among several others. Although the immune escape strategies in B-NHL may vary between individuals, the phenotypic and transcriptomic analysis of their biopsies consistently identifies a combination of several pathways.Citation8
This issue is particularly relevant when considering the ongoing trials of immune checkpoint-targeting therapeutic regimens for these aggressive lymphomas.Citation9-13 To maximize the effects of these innovative therapies, the determinants of response in B-NHL patients must be better understood. In particular, the various immune escape pathways might constitute a mechanism for immune resistance and beyond, to immune checkpoint therapeutic resistance. Thus, the development of tools to assess the global status of immune escape in B-NHL patients is required.
Because gene expression profiles reflect the function and activity status of a cell, transcriptome data mining on a large scale allows us to detect genes that are differentially expressed between groups of samples.Citation14-18 The functional significance of these differentially-expressed gene sets is classically determined by gene set enrichment analysis (GSEA).Citation19 This method requires a priori-defined groups of samples (e.g., group A vs. group B), and therefore cannot analyze single samples. In addition, it produces excessive false discovery rates and p values since it inappropriately uses the Fischer exact test.Citation20 Single sample GSEA (ssGSEA) have also been used although carry the same statistical flaw,Citation21,22,23 and their long computing time is an issue for large datasets. Another method for ssGSEA is based on z scores per gene set per sample Citation24,25 and avoids this pitfall but still requires cohorts of samples to compute each sample score.
Here, we report a novel and algorithmically-optimized method for scoring the enrichment of pre-defined gene sets in single samples without pitfalls such as those described above. We used this method to score the enrichment of various gene sets, including a gene set for immune escape, in ∼1500 transcriptomes from B-NHL biopsies. This was validated by comparing these results with the main phenotypic hallmarks of B-NHLs, and allowed us to detect four stages of immune escape in B-NHL.
Results
Quality control for SES
We downloaded and assembled a series of 1,446 transcriptomes from 7 distinct B-NHL and 32 samples of normal B-cell controls. Each transcriptome was rank-ordered by decreasing expression levels and scored against a range of gene sets (downloaded from the specified sources: see Methods section). The score for the enrichment of a gene set in a sample (SES) was defined as: SES = (−log10 (p)), using p values from testing the alternative: “empirical distribution of the gene set in the transcriptome greater than uniform distribution”. To determine which of the Kolmogorov–Smirnov (KS), t-test (T), and Wilcoxon (W) tests yielded SES that best matched the phenotype, these tests were compared as follows.
First, the phenotypic data indicated that this data set included both highly mitotic samples (centrocytes, centroblasts, lymphomas, and lymphoid tissue-derived CLL biopsies; n = 796) and weakly mitotic samples (naive B-cells, blood-purified B-cells, and blood-derived CLL and HCL; n = 635). This dichotomy allowed us to compare the KS, T, and W tests to determine which was best for discriminating between these two classes, using SES calculated for the “mitosis”, “cell proliferation”, “cell division”, and “DNA replication” gene sets. Comparing the respective ROC curves revealed that the greatest area under the curves was with the W test for all four gene sets, indicating that its SES most accurately reflected the mitotic phenotype (, ).
Figure 1. Quality control of the sample scoring test. (A) Performance of scoring methods by the Kolmogorov–Smirnov (KS), T-test, and Wilcoxon tests, compared by ROC curves for the gene sets “DNA replication”, “cell division”, “cell proliferation”, and “mitosis” in the cohort of (n = 1446) B-NHL transcriptomes split according to their proliferating or non-proliferating status (determined by phenotypic analysis). (B) Dot plots of sample enrichment scores (SES) for “female” versus “male” gene sets in the (n = 553) gender-annotated samples within the dataset, using the Wilcoxon test (top), or KS-, T-test-, or Wilcoxon-based scoring (bottom). The rate of discordant cases (DC) for molecularly-inferred versus clinically-annotated gender is indicated. (C) 3D plot of the (n = 553) subtype-specified DLBCL samples using Wilcoxon-based scores for the “ABC”, “GCB”, and “PMBL” gene sets (see Fig. S2).
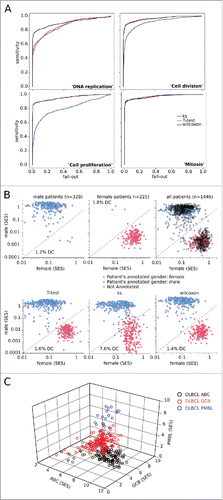
Table 1. AUC for ROC curves.
Second, we considered gene set-defined gender. Sexually dimorphic genes defined the “male” and “female” gene sets (Table S2) Citation26 and their respective SES were computed for the 553 gender-annotated samples. The plots for female versus male allowed us to partition most samples correctly and showed the lowest discordance rate (<2%) with the W test, the same value as has been reported elsewhere ().Citation14 Whether the discordance between the clinically-annotated and molecularly-inferred gender reflected errors of scoring or of annotation could not be determined. However, this method allowed us to infer gender for all of the other unannotated samples, yielding no discordance for the DLBCL of testicular subtype (not shown).
Third, DLBCL are comprised of distinct subtypes such as activated B-cell (ABC), germinal center B-cell (GCB), and primary mediastinal large B-cell lymphoma (PMBL), which are each defined by specific gene expression patterns.Citation27-29 The W test was used to score each of the 553 DLBCL samples for the three subtype-specific gene sets, and yielded plots which partitioned these three groups (). On this basis, the subtype of the other DLBCL samples annotated by tissue of origin or listed as unclassified could also be unambiguously assigned (Fig. S2).
Finally, this ssGSEA method discriminated between relevant and irrelevant (simulated) sets of data and was not sensitive to different sources of RMA-normalized data (Fig. S3). Therefore, these control studies qualified the Wilcoxon test-based scoring of gene set enrichment in single transcriptomes. Although its performance cannot be assessed a priori for any new gene sets, this method scored each sample independently and yielded scores matched to phenotype.
SES detect hallmarks of B-NHLs
We then scored for enrichment of gene sets from the KEGG and GO databases that correspond to well-known phenotypic hallmarks of B-NHL. First, we assessed for proliferation: for the gene set “Mitosis”, the mean SES was 4.8 for centrocytes and centroblasts, 0.3 for naive and blood B-cells, 0.5 for blood CLL, 0.8 for HCL, 5.3 for all DLBCL samples, 7.2 for BL, 2.5 for FL, 2.3 for MCL, and 1.8 for MZL (). These results matched the respective mitotic phenotypes of such samples, as B-cells from healthy donor blood are non-proliferating in contrast to the highly mitotic B-centrocytes and centroblasts from lymphoid tissues. CLL and HCL blood samples also do not usually have a proliferative phenotype,Citation30 with a higher level of mitosis occuring in nodal CLL. In contrast, lymphoma samples are usually very mitotic, with BL reported to have nearly 100% of cells proliferating.Citation31 Similar results were obtained by scoring other gene sets related to cell proliferation (Fig. S4A).
Figure 2. SES of the B-NHL data set matched to sample phenotype. Sample enrichment scores (SES) were computed for each of the 1,446 samples of the B-NHL data set and for the gene sets indicated on the y-axis. Each sample is shown by a dot, red bars are the means of the specified group. CC: centroblasts, CB: centrocytes. (A) SES for gene sets according to proliferation phenotype. (B) SES for tight junctions with sample groups split according to sample preparation. (C) SES for the KEGG gene sets “BCR signaling”, “glycolysis”, and “oxydative phosphorylation”, classified according to lymphoid malignancy.
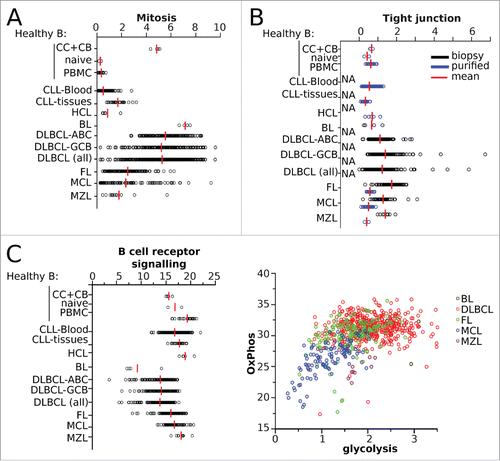
Second, we assessed the cell adhesion phenotype characteristic of lymphoma in our transcriptomic dataset. The SES of the “tight junction” gene set were higher in lymphoma biopsies than in purified B-cell samples (mean SES = 1.7 vs. 0.6, respectively, unpaired Student's p < 10−40), as expected Citation32 ().
Third, different signaling and metabolic pathways were assessed. As expected, all samples scored high for the “BCR signaling pathway” gene set, although MYC-induced BL scored lower than the others Citation33 (mean SES = 9.2 for BL vs. 16.5 for all others, p = 0.003) (). Glycolysis and oxidative phosphorylation are metabolic hallmarks of cancer cells, and in accordance with this the SES for “oxidative phosphorylation” were high in all samples. The SES for “glycolysis and gluconeogenesis” were low in CLL, HCL, MCL, and resting normal B-cells, whereas such scores were significantly higher in normal proliferating B-cells, BL, DLBCL, FL, and MZL, in line with results from 16FDG-PET imaging of these tumors.Citation34-36 Combining the SES for “Glycolysis” and “OxPhos” illustrated the distinct metabolic profiles of the different neoplasms ( and Fig. S4B). Scores for the additional gene sets “acetyltransferase activity”, “histone modification”, and “T-cell activation” also matched the hallmarks of B-NHL (Fig. S4C).
Fourth, leucocyte infiltration of cancer biopsies can be assessed by the gene expression-based quantification of 22 gene sets composed of differentially-expressed genes (known as TM22).Citation14,18,23 These 22 gene sets were scored against the data set and the results were compared for each type of B-NHL. In agreement with previous reports,Citation14,18 all tumors presented the signature of their B-cell-of-origin and had very low mastocyte and granulocyte scores. The presence of other leucocyte subsets differed according to the disease (). On average, the DLBCL samples showed similar levels of lymphoid (T and NK cells) and myeloid (monocytes, macrophages, and DC) signatures, with M1 macrophages dominating in the latter. The CLL samples comprised 4-fold lower myeloid levels than those found for DLBCL. In contrast, the FL, MCL, and MZL samples had the highest T-cell compartments, as expected.Citation37 A similar procedure comparing the various subtypes of DLBCL evidenced a significant deficit of leucocyte infiltrates in central nervous system DLBCL and showed the divergent cellular and metabolic profiles of GCB and ABC (Fig. S5).
Scoring immune escape in B-NHL
B-NHL express immune checkpoint PD1 and CTLA4 markers that can be targeted by new therapeutic antibodies (see refs.Citation9,10 for review). In B-NHL other markers of immune escape frequently co-evolve with these, as shown through immunohistochemistry analysis of tissue microarrays and the expression of these immune escape markers in DLBCL and FL biopsies.Citation8 We compared the expression of these immune escape genes at the single gene level in control B-cells, FL, and DLBCL biopsies using the Oncomine resource, and found a significant upregulation of several genes in both the FL and DLBCL lymphoma samples (listed by decreasing significance relative to controls): CCL2, IL6ST, IDO1, TIMP1, LGALS3, VEGFA, HAVCR2, MRC1, TIGIT, CD163, IL10, PDCD1LG2, CTLA4, LAG3, LGALS1, CSF1, MSR1, JAK2, SOCS3, CD274, ICOS, HGF, IL23A, GDF15, FOXP3, PVR, MCL1, PDCD1, CCL22, LAIR1, CD86, IDO2, and KIR2DL1. Each of these genes was upregulated to a different extent in each sample, as illustrated by the fact that the most upregulated gene was either MRC1 or PTGS2 in two different samples of FL, and CTLA4 or LAG3 in two different samples of DLBCL (Fig. S4). Hence, despite the lack of a specific gene expression signature, these distinct patterns suggested a functional convergence toward immune escape.
We therefore defined a gene set (IEGS33, Table S3) that encompassed the above 33 most shared and upregulated genes to investigate their collective contribution to immune escape at the single sample level in B-NHL. The SES for IEGS33 was computed for the 1,446 B-NHL dataset samples. A preliminary check-up of the distribution of these scores with these actual or 500 simulated samples confirmed that IEGS33 is not a random gene set and carries a significant amount of information about these samples (). In the B-NHL cohort, we found that 24.9% of samples had a significantly higher IEGS33 score, while healthy B-cells did not (mean SES = 0.3). In addition, most CLL and HCL scored nearly as low as normal B-cells for IEGS33 (group mean of SES = 0.2 for CLL and HCL), whereas few CLL blood samples were elevated, confirming the heterogeneity of immune escape in these diseases.Citation38,39 The IEGS33 scores of BL were slightly higher (mean SES = 0.6), suggesting that these lymphoma escape immunity through different pathways.Citation40,41 Low scores were also found in most but not all MCL (mean SES = 0.4 and 1 for MCL and MZL, respectively), consistent with earlier reports Citation42,43 that included PD1 defects and autoimmunity in MZL.Citation44,45 In contrast, IEGS33 scores were significantly elevated in 73% of FL (mean SE = 1.4) and 78% of DLBCL (mean SES = 1.6) ().Citation8 Likewise, the DLBCL ABC, as well as PMBL samples, generally scored higher than the DLBCL GCB (mean SES = 1.6 vs. 1.3, unpaired Student p = 0.0008 for ABC vs. GCB) (). In addition, the DLBCL samples that were clinically annotated as “nodal” and “extra-nodal testicular” had the highest IEGS33 scores and were of ABC subtype (Fig. S2). Although primary CNS DLBCL were also of ABC subtype, their IEGS33 scores were the lowest among the DLBCL samples (). Similar results were obtained with the IEGS51 gene set depicted elsewhere.Citation8
Figure 4. Scores for the immune escape gene set IEGS33 in 1,446 B-NHL samples. (A) Quality control of the IEGS33 gene set. The right skewed distribution of SES for IEGS33 in the 1446 B-NHL samples or in 500 simulated samples (shown in Fig. S2) demonstrates that this gene set carries relevant information about these samples. For B-NHL samples 24.9% of SES are above 1.3 whereas less than 5% are predicted for the simulated samples. (B–C) IEGS33 scores of the B-NHL samples classified by malignancy (B) and subtype of DLBCL (C). Red line shows group means. CC: centroblasts, CB: centrocytes.
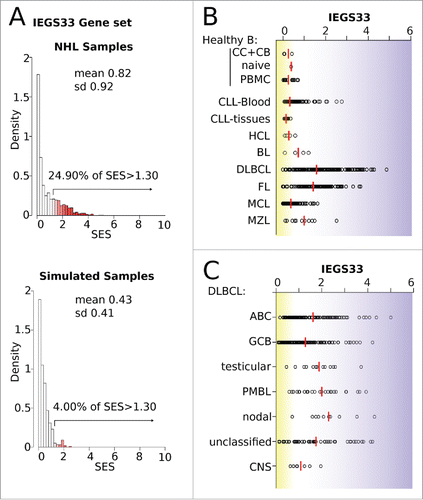
Together, these results pinpoint qualitative and quantitative differences in the immune escape strategies of NHL, with three quarters of FL and DLBCL upregulating the collective expression of immune escape genes.
Four stages of immune escape in NHL
Since the activation of immune effectors represents the substrate of immune escape, we first analyzed the relationship between these features by plotting the SES of the IEGS33 gene set versus the “T-cell activation” gene set. We arbitrarily set gates to define the following groups of samples: group I (T-cell activation− IEGS33−), group II (T-cell activation+ IEGS33−), group III (T-cell activation+ IEGS33+), and group IV (T-cell activation− IEGS3+). As expected, the normal B-cell samples fell into group I. Most CLL and HCL samples were also in group I, with the others in group II. BL samples were in groups I and IV only. Of the MCL samples, 57.4% were in group I, 30% in group II, and 12% in group III, with none in group IV. In contrast, the MZL, FL, and DLBCL samples were distributed between all four groups. For MZL, 25% of samples were in group I, 16.7% in group II, 50% in group III, and 8% in group IV. For FL, these figures were 13.4%, 6.7%, 72.2%, and 0.7% in groups I–IV, respectively, and for DLBCL they were 19.6%, 2.3%, 53.3%, and 24.8%, respectively. Among the three subtypes of DLBCL, we observed 21.4% of ABC, 23.3% of GCB, and 12.5% of PMBL samples in group I, 0.7% of ABC, 5% of GCB, and 0% of PMBL samples in group II, 57.9% of ABC, 51.6% of GCB, and 66.7% of PMBL samples in group III, and 20% of ABC, 20.1% of GCB, and 20.8% of PMBL samples in group IV ().
Figure 5. Stages of immune escape in B-NHL. (A) SES dot plots for IEGS33 versus T-cell activation for the 1,446 samples. CC: centroblasts, CB: centrocytes. Groups of phenotypes were arbitrarily defined according to the dotted lines: group I (IEGS33− T-cell activation−), group II (IEGS33− T-cell activation+), group III (IEGS33+ T-cell activation+), group IV (IEGS33+ T-cell activation−). (B) Left: four groups. Right: distribution of samples in these groups (group I in black, group II in green, group III in red, and group IV in blue) shown by malignancy. (C) Overall survival (OS) of the (n = 580) DLBCL patients according to the four immune escape groups (Log rank p = 0.04).
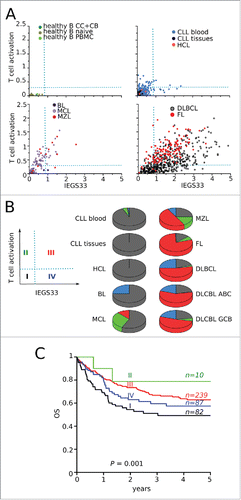
To further characterize these four groups of samples, we compared their respective scores for various other gene sets, restricting this analysis to the 418 DLBCL samples to avoid interpretation bias. Samples from all groups scored similarly for most metabolic and housekeeping gene sets, as well as for B-cell signature gene sets, typifying tumor cells. For mitosis gene sets, samples from groups I and IV had significantly higher scores than groups II and III (Student's p <0.05), indicating higher proliferation rates. The same pattern was found for gene sets related to genetic instability, such as “DNA repair”, “response to DNA damage stimulus”, and “DNA integrity checkpoint”, indicating higher mutation rates. The level of total leucocyte infiltration was significantly lower in group I than elsewhere (Student's p < 10−10), and was highest in groups II and III, with the “T-cell-specific” and “NK cell-specific” scores also highest in groups II and III. In parallel, the scores for “apoptosis” and “programmed cell death” were highest in groups II and III. However, myeloid infiltration (including the macrophage and DC subsets) scored significantly higher in groups III and IV (Student's p < 10−27) ().
Table 2. SES in the four groups defined by T-cell activation and IEGS33.
Together, these group characteristics were consistent with the following four stages of cancer immune editing:
Stage 1: Non-immunogenic tumor. Group I scores showed that cells were proliferating and genetically unstable but with very low immune infiltrates;
Stage 2: Immunogenic tumor without immune escape. Compared to stage 1, group II scores were increased for “T- and NK cell-specific”, “T-cell activation”, and “programmed cell death” gene sets, but decreased for “proliferation” and “DNA repair” gene sets, presumably due to tumor lysis;
Stage 3: Immunogenic tumor with immune escape. Group III scores were as those for stage 2 but with higher scores for the “IEGS33” and “myeloid cell infiltrates (monocytes, macrophages, DC)“ gene sets;
Stage 4: Fully immuno-edited tumor. Group IV scores were as those for stage 3, but collapsed for “T- and NK cell infiltrates”, “T-cell activation”, and “programmed cell death”, while scores for “proliferation” and “DNA repair” were increased. Stage 4 is very similar to stage 1 but with immune infiltrates.
This model of four successive stages of cancer immune editing predicts a worse clinical outcome for B-NHL patients with a tumor score characterizing either the earliest or the most advanced stages of immune escape, since both lack effective immuno-protection. Using the 418 clinically annotated DLBCL samples from the data set, we compared the clinical outcomes of DLBCL patients with tumors in the different groups described above. This clearly showed that overall survival at 5 y (OS) was longest for group II and shortest for group I. In agreement with the four stage model, OS gradually decreased from group II (OS = 0.80, n = 10) to group III patients (OS = 0.62, n = 239) and group IV patients (OS = 0.58, n = 87) (Log Rank p = 0.001), with group IV patients having the same OS rates as those in group I (OS = 0.50, n = 82). Similar results were obtained for OS at 10 y (p = 0.015) and 20 y (p = 0.04) (not shown). Together these results fully support a model with four stages of cancer immune editing in B-NHL.
Finally, we correlated our four-stage immune escape model with the standard DLBCL international prognostic index (IPI) scoring system and found that all cases classified within the favorable immune escape stage 2 had a low IPI. Reciprocally however, all of the IPI classes each had a similar distribution of immune escape stages (Fischer exact test p = 0.6) (Table S4). In addition, DLBCL of GCB subtype encompassed significantly higher rates of stage 2 cases than ABC and other subtypes (Fischer exact test p = 0.04). Hence, immune escape staging is distinct from but complements the standard prognostic scores of DLBCL.
Discussion
To conclude, we used a novel data mining method that scored the enrichment of gene sets in single microarrays, and detected four stages of cancer immune editing in humans, an issue so far deemed to be “a significant technical challenge” with predictive and prognostic significance.Citation46 Current ssGSEA methods rely on clinical classification or prior information on sample phenotype, and require either cohorts to z-score each sample Citation24,25 or the application of statistics involving equiprobable genes as inadequate null hypotheses.Citation21-23,47 Our method avoids such pitfalls and its algorithmic optimization allows for the rapid analysis of large series of data, such as labtop-based scoring within seconds for 1,500 gene sets in 1,446 microarrays. Although RNASeq or proteome datasets are rich sources of material for such studies, nearly two decades of microarray-based research in cancer means that large and precious amounts of this publicly-available resource have accumulated. Collecting large series of microarray data from GEO data sets involves collating many different datasets, whose eventual inconsistencies might introduce confounding divergences.Citation48 Here, by associating robust statistics and selecting compatibility-based data sets, we were able to assemble 1,446 consistent transcriptomes from 33 different datasets.
Although this approach is capable of analyzing other types of large-scale data sets from NGS, the microarray-based study reported here is able to detect the hallmarks of B-NHL and their specific stages of immune editing. Indeed, immune escape by B-NHL involves not only changes in gene expression but also somatic mutation-driven pathways.Citation5 Our technique cannot detect somatic mutations including those that target immune activation, the downregulation of immuno-activating genes, or the upregulation of genes not in IEGS33. Nevertheless, it can detect the first stage of immune escape in DLBCL, which is characterized by high proliferation and mutation rates but a significantly low level of immune cell infiltration. This suggests that tumors in this first stage lack immunity due to an impaired primary activation. Most HLA-mutated B-NHL tumorsCitation1,3,5,6 presumably lie at this stage, which presents the worst clinical outcome (). However, this might not be the case for B-NHL tumors at stage 2, which show the best survival rates and transcriptional hallmarks of functional cytotoxic immunity: cell infiltration prominently by T- and NK cells, T-cell activation and an increase in cell death alongside decreased proliferation and genetic instability. Stage 2 DLBCL patients were also found to have low IPI scores (Table S4), supporting a progressive model. However, the low number of cases meant that this finding was not significant, hence from this data we conclude that immune escape stages and IPI scores are related but distinct prognostic criteria. The stage 2 pattern of immune activation was also found in stage 3, but was accompanied by obvious signs of immune escape, as indicated by increased scores for IEGS33 and myeloid cell infiltrates. When considering cancer immune editing in terms of Darwinian evolution, through elimination, equilibrium and escape phases,Citation49 stage 3 would correspond to the end equilibrium-early escape and, in line with this, DLBCL patients at this stage have shorter survival rates than those at stage 2 (). This progression can lead to fully immuno-edited stage 4 tumors whose immune escape collapses the host-protective immunity and allows the re-emergence of highly proliferating and mutated tumors despite the presence of a significant but non-protective immune cell infiltrate. Stage 4 clearly corresponds to the late escape phase of the cancer immuno-editing process,Citation46 and DLBCL patients at this stage have shorter OS than those at stage 3.
It could be argued that B-NHL tumors which are either immunologically undetected or detected by an ineffective immunity should ultimately grow in the same way, a view supported by the nearly similar OS rates of DLBCL patients at stages 1 and 4 (). Nevertheless, these stages represent clearly distinct contexts since in stage 4 (but not stage 1) cellular targets for the emerging immune checkpoint therapeutics are present. We report that a total of 78% of DLBCL, 73% of FL, and 58% of MZL are tumors at stages 3 and 4 of immune escape. We thus propose that the immune escape stages 3 and 4 constitute a major criterion to stratify B-NHL patients to identify those most likely to benefit from immune checkpoint blockade therapies.
Materials and methods
Datasets
We first screened the GEO Citation50 repository for gene expression profiles of B-NHL obtained with the Affymetrix HG U133 plus 2.0 microarray and downloaded 47 series of RMA-normalized data sets (GSE) as txt files, together with gene sets from Gene Ontology,Citation51 KEGG (http://www.genome.jp/kegg/pathway.html), the Molecular Signatures Data base (MSigDB 3.0) Citation52 and TM22,Citation14,18 and the gene sets defined in the text. Each series of transcriptomes was then collapsed to common protein-encoding genes (using the HUGO nomenclature) and the compatibility for merging of the datasets was assessed. The gene expression data of each sample (GSM) were transformed into van der Waerden's scores,Citation53,54 then each data set was reduced to a per gene median of samples and the pairwise correlation matrix of these 47 datasets was computed (Fig. S1).Citation48 The 33 data sets with correlations >0.8 were deemed compatible for merging while others less homogeneous were discarded (). Since these 33 datasets comprised malignant samples as well as various normal cell controls, compatibility screening was repeated at the sample level for the GSM groups from either all malignant samples or all non-cancer cell controls (Fig. S2). The samples deemed irrelevant for this meta-analysis (e.g., those from a microarray-based study of in vitro drug activity on cell lines) were rejected. This yielded a data set composed of 1,446 samples from 7 distinct malignant histologies. These included 489 DLBCL, 149 FL, 125 MCL, 12 MZL, 4 Burkitt's lymphoma (BL), 630 chronic lymphocytic leukemia (CLL), 5 hairy cell leukemia (HCL), and control cells. The control cells included 32 normal B-cell samples including CD20 B-cells purified from peripheral blood, naive B-cells, centrocytes, and centroblasts purified from the tonsils of non-cancer donors.
Sample enrichment scores (SES)
The RMA-normalized expression data from each collapsed sample (GSM) were rank-ordered by decreasing the expression level along the [0–1] segment (highest expression level = 0, lowest expression level = 1), to yield v, a vector of ranks. Since each series from the NHL data set had been pre-selected for a high pairwise correlation (see above), their rank-ordered gene distributions were also highly correlated, despite distinct batch RMA normalizations (Fig. S3).
We rationalized that if gene set g was a subset of v then assessing the enrichment of g would mean testing g versus v. However the human genome comprises many pleiotropic genes that are involved in various different pathways, functions and cell compartments and so are more frequently upregulated in all samples and are over-represented in gene sets and database.Citation20 Since genes are far from equiprobable in gene sets, the adequate null hypothesis for g versus v gives genes with probabilities proportional to their frequency in the gene set database Citation55 and requires the KS, T-, and W-tests with frequency-corrected null hypotheses (see refs.Citation56-58 for review). In addition, scoring the enrichment of the ∼1500 KEGG and GO gene sets in >1,000 samples would mean computing ∼1.5 million tests with a high accuracy for the most significant results. Therefore, algorithmic optimization is necessary to i) compute scores in a reasonable time, ii) precisely compute the most significant (smallest) p values, iii) allow the gene frequency correction, and iv) allow testing of the three alternatives, namely “greater” (indicating an enriched gene set), “less” (a downregulated gene set), and “two-sided” (when the gene set is enriched or downregulated). We developed an R script for which the source codes are available at: https://sites.google.com/site/fredsoftwares/products/autocompare_ses. The current version of it computes the enrichment of ∼1500 gene sets within 0.1 sec for a single sample.
Disclosure of potential conflicts of interest
No potential conflicts of interest were disclosed.
KONI_A_1188246_s02.pdf
Download PDF (5.3 MB)Acknowledgments
We acknowledge all members of JJF's team for their stimulating comments on this work and Dr Kelly Thornber for English editing of the text.
Funding
This work was supported in part by institutional grants from the Institut National de la Santé et de la Recherche Médicale (INSERM), the Université Toulouse III: Paul Sabatier, the Center National de la Recherche Scientifique (CNRS), the Laboratoire d'Excellence Toulouse Cancer (TOUCAN) (contract ANR11-LABX) and the Program Hospitalo-Universitaire en Cancérologie CAPTOR (contract ANR11-PHUC0001).
References
- Amiot L, Onno M, Lamy T, Dauriac C, Le Prise PY, Fauchet R, Drenou B. Loss of HLA molecules in B lymphomas is associated with an aggressive clinical course. Br J Haematol 1998; 100:655-63; PMID:9531330; http://dx.doi.org/10.1046/j.1365-2141.1998.00631.x
- Challa-Malladi M, Lieu YK, Califano O, Holmes AB, Bhagat G, Murty VV, Dominguez-Sola D, Pasqualucci L, Dalla-Favera R. Combined genetic inactivation of beta2-Microglobulin and CD58 reveals frequent escape from immune recognition in diffuse large B cell lymphoma. Cancer Cell 2011; 20:728-40; PMID:22137796; http://dx.doi.org/10.1016/j.ccr.2011.11.006
- Pasqualucci L, Dalla-Favera R. SnapShot: diffuse large B cell lymphoma. Cancer Cell 2014; 25:132-e1; PMID:24434215; http://dx.doi.org/10.1016/j.ccr.2013.12.012
- Pasqualucci L, Khiabanian H, Fangazio M, Vasishtha M, Messina M, Holmes AB, Ouillette P, Trifonov V, Rossi D, Tabb∫ F et al. Genetics of follicular lymphoma transformation. Cell Rep 2014; 6:130-40; PMID:24388756; http://dx.doi.org/10.1016/j.celrep.2013.12.027
- Pasqualucci L, Dalla-Favera R. The genetic landscape of diffuse large B-cell lymphoma. Semin Hematol 2015; 52:67-76; PMID:25805586; http://dx.doi.org/10.1053/j.seminhematol.2015.01.005
- Green MR, Kihira S, Liu CL, Nair RV, Salari R, Gentles AJ, Irish J, Stehr H, Vicente-Dueñas C, Romero-Camarero I et al. Mutations in early follicular lymphoma progenitors are associated with suppressed antigen presentation. Proc Natl Acad Sci U S A 2015; 112:E1116-25; PMID:25713363; http://dx.doi.org/10.1073/pnas.1501199112
- Jiang Y, Melnick A. The epigenetic basis of diffuse large B-cell lymphoma. Semin Hematol 2015; 52:86-96; PMID:25805588; http://dx.doi.org/10.1053/j.seminhematol.2015.01.003
- Laurent C, Charmpi K, Gravelle P, Tosolini M, Franchet C, Ysebaert L, Brousset P, Bidaut A, Ycart B, Fournié JJ. Several immune escape patterns in non-Hodgkin's lymphomas. OncoImmunology 2015; 4:e1026530; PMID:26405585; http://dx.doi.org/10.1080/2162402X.2015.1026530
- Armand P. Checkpoint blockade in lymphoma. Hematology Am Soc Hematol Educ Program 2015; 2015:69-73; PMID:26637703; http://dx.doi.org/0.1182/asheducation-2015.1.69
- Armand P. Immune checkpoint blockade in hematologic malignancies. Blood 2015; 125:3393-400; PMID:25833961; http://dx.doi.org/10.1182/blood-2015-02-567453
- Ansell SM, Lesokhin AM, Borrello I, Halwani A, Scott EC, Gutierrez M, Schuster SJ, Millenson MM, Cattry D, Freeman GJ et al. PD-1 blockade with nivolumab in relapsed or refractory Hodgkin's lymphoma. N Engl J Med 2015; 372:311-9; PMID:25482239; http://dx.doi.org/10.1056/NEJMoa1411087
- Berger R, Rotem-Yehudar R, Slama G, Landes S, Kneller A, Leiba M, Koren-Michowitz M, Shimoni A, Nagler A. Phase I safety and pharmacokinetic study of CT-011, a humanized antibody interacting with PD-1, in patients with advanced hematologic malignancies. Clin Cancer Res 2008; 14:3044-51; PMID:18483370; http://dx.doi.org/10.1158/1078-0432.CCR-07-4079
- Westin JR, Chu F, Zhang M, Fayad LE, Kwak LW, Fowler N, Romaguera J, Hagemeister F, Fanale M, Samaniego F et al. Safety and activity of PD1 blockade by pidilizumab in combination with rituximab in patients with relapsed follicular lymphoma: a single group, open-label, phase 2 trial. Lancet Oncol 2014; 15:69-77; PMID:24332512; http://dx.doi.org/10.1016/S1470-2045(13)70551-5
- Gentles AJ, Newman AM, Liu CL, Bratman SV, Feng W, Kim D, Nair VS, Xu Y, Khuong A, Hoang CD et al. The prognostic landscape of genes and infiltrating immune cells across human cancers. Nat Med 2015; 21:938-45; PMID:26193342; http://dx.doi.org/10.1038/nm.3909
- Hebestreit K, Grottrup S, Emden D, Veerkamp J, Ruckert C, Klein HU, Müller-Tidow C, Dugas M. Leukemia gene atlas–a public platform for integrative exploration of genome-wide molecular data. PLoS One 2012; 7:e39148; PMID:22720055; http://dx.doi.org/10.1371/journal.pone.0039148
- Lee HK, Hsu AK, Sajdak J, Qin J, Pavlidis P. Coexpression analysis of human genes across many microarray data sets. Genome Res 2004; 14:1085-94; PMID:15173114; http://dx.doi.org/10.1101/gr.1910904
- Mizuno H, Nakanishi Y, Ishii N, Sarai A, Kitada K. A signature-based method for indexing cell cycle phase distribution from microarray profiles. BMC Genomics 2009; 10:137; PMID:19331659; http://dx.doi.org/10.1186/1471-2164-10-137
- Newman AM, Liu CL, Green MR, Gentles AJ, Feng W, Xu Y, Hoang CD, Diehn M, Alizadeh AA. Robust enumeration of cell subsets from tissue expression profiles. Nat Methods 2015; 12:453-7; PMID:25822800; http://dx.doi.org/10.1038/nmeth.3337
- Subramanian A, Tamayo P, Mootha VK, Mukherjee S, Ebert BL, Gillette MA, Paulovich A, Pomeroy SL, Golub TR, Lander ES et al. Gene set enrichment analysis: A knowledge-based approach for interpreting genome-wide expression profiles. Proc Natl Acad Sci U S A 2005; 102:15545-50; PMID:16199517; http://dx.doi.org/10.1073/pnas.0506580102
- Ycart B, Pont F, Fournie JJ. Curbing false discovery rates in interpretation of genome-wide expression profiles. J Biomed Inform 2014; 47:58-61; PMID:24060601; http://dx.doi.org/10.1016/j.jbi.2013.09.004
- Barbie DA, Tamayo P, Boehm JS, Kim SY, Moody SE, Dunn IF, Schinzel AC, Sandy P, Meylan E, Scholl C et al. Systematic RNA interference reveals that oncogenic KRAS-driven cancers require TBK1. Nature 2009; 462:108-12; PMID:19847166; http://dx.doi.org/10.1038/nature08460
- Verhaak RG, Hoadley KA, Purdom E, Wang V, Qi Y, Wilkerson MD, Miller CR, Ding L, Golub T, Mesirov JP et al. Integrated genomic analysis identifies clinically relevant subtypes of glioblastoma characterized by abnormalities in PDGFRA, IDH1, EGFR, and NF1. Cancer Cell 2010; 17:98-110; PMID:20129251; http://dx.doi.org/10.1016/j.ccr.2009.12.020
- Yoshihara K, Shahmoradgoli M, Martinez E, Vegesna R, Kim H, Torres-Garcia W, Treviño V, Shen H, Laird PW, Levine DA et al. Inferring tumour purity and stromal and immune cell admixture from expression data. Nat Commun 2013; 4:2612; PMID:24113773; http://dx.doi.org/10.1038/ncomms3612
- Gundem G, Lopez-Bigas N. Sample-level enrichment analysis unravels shared stress phenotypes among multiple cancer types. Genome Med 2012; 4:28; PMID:22458606; http://dx.doi.org/10.1186/gm327
- Ferreira PG, Jares P, Rico D, Gomez-Lopez G, Martinez-Trillos A, Villamor N, Ecker S, González-Pérez A, Knowles DG, Monlong J et al. Transcriptome characterization by RNA sequencing identifies a major molecular and clinical subdivision in chronic lymphocytic leukemia. Genome Res 2014; 24:212-26; PMID:24265505; http://dx.doi.org/10.1101/gr.152132.112
- Isensee J, Witt H, Pregla R, Hetzer R, Regitz-Zagrosek V, Noppinger PR. Sexually dimorphic gene expression in the heart of mice and men. J Mol Med (Berl) 2008; 86:61-74; PMID:17646949; http://dx.doi.org/10.1007/s00109-007-0240-z
- Alizadeh AA, Eisen MB, Davis RE, Ma C, Lossos IS, Rosenwald A, Boldrick JC, Sabet H, Tran T, Yu X et al. Distinct types of diffuse large B-cell lymphoma identified by gene expression profiling. Nature 2000; 403:503-11; PMID:10676951; http://dx.doi.org/10.1038/35000501
- Rosenwald A, Wright G, Leroy K, Yu X, Gaulard P, Gascoyne RD, Chan WC, Zhao T, Haioun C, Greiner TC et al. Molecular diagnosis of primary mediastinal B cell lymphoma identifies a clinically favorable subgroup of diffuse large B cell lymphoma related to Hodgkin lymphoma. J Exp Med 2003; 198:851-62; PMID:12975453; http://dx.doi.org/10.1084/jem.20031074
- Wright G, Tan B, Rosenwald A, Hurt EH, Wiestner A, Staudt LM. A gene expression-based method to diagnose clinically distinct subgroups of diffuse large B cell lymphoma. Proc Natl Acad Sci U S A 2003; 100:9991-6; PMID:12900505; http://dx.doi.org/10.1073/pnas.1732008100
- Kluin-Nelemans HC, Beverstock GC, Mollevanger P, Wessels HW, Hoogendoorn E, Willemze R et al. Proliferation and cytogenetic analysis of hairy cell leukemia upon stimulation via the CD40 antigen. Blood 1994; 84:3134-41; PMID:7524766
- Ferry JA. Burkitt's lymphoma: clinicopathologic features and differential diagnosis. Oncologist 2006; 11:375-83; PMID:16614233; http://dx.doi.org/10.1634/theoncologist.11-4-375
- Lenz G, Wright G, Dave SS, Xiao W, Powell J, Zhao H, Xu W, Tan B, Goldschmidt N, Iqbal J et al. Stromal gene signatures in large-B-cell lymphomas. N Engl J Med 2008; 359:2313-23; PMID:19038878; http://dx.doi.org/10.1056/NEJMoa0802885
- Schmitz R, Young RM, Ceribelli M, Jhavar S, Xiao W, Zhang M, Wright G, Shaffer AL, Hodson DJ, Buras E et al. Burkitt lymphoma pathogenesis and therapeutic targets from structural and functional genomics. Nature 2012; 490:116-20; PMID:22885699; http://dx.doi.org/10.1038/nature11378
- Meignan M. Quantitative FDG-PET: a new biomarker in PMBCL. Blood 2015; 126:924-6; PMID:26294712; http://dx.doi.org/10.1182/blood-2015-07-653386
- Wu X, Pertovaara H, Korkola P, Vornanen M, Eskola H, Kellokumpu-Lehtinen PL. Glucose metabolism correlated with cellular proliferation in diffuse large B-cell lymphoma. Leuk Lymphoma 2012; 53:400-5; PMID:21913807; http://dx.doi.org/10.3109/10428194.2011.622420
- Xie M, Wu K, Liu Y, Jiang Q, Xie Y. Predictive value of F-18 FDG PET/CT quantization parameters in diffuse large B cell lymphoma: a meta-analysis with 702 participants. Med Oncol 2015; 32:446; PMID:25511321; http://dx.doi.org/10.1007/s12032-014-0446-1
- Dave SS, Wright G, Tan B, Rosenwald A, Gascoyne RD, Chan WC, Fisher RI, Braziel RM, Rimsza LM, Grogan TM et al. Prediction of survival in follicular lymphoma based on molecular features of tumor-infiltrating immune cells. N Engl J Med 2004; 351:2159-69; PMID:15548776; http://dx.doi.org/10.1056/NEJMoa041869
- Guillaume N, Marolleau JP. Is immune escape via human leukocyte antigen expression clinically relevant in chronic lymphocytic leukemia? Focus on the controversies. Leuk Res 2013; 37:473-7; PMID:23347904; http://dx.doi.org/10.1016/j.leukres.2012.12.021
- Ysebaert L, Fournie JJ. Genomic and phenotypic characterization of nurse-like cells that promote drug resistance in chronic lymphocytic leukemia. Leuk Lymphoma 2011; 52:1404-6; PMID:21699388; http://dx.doi.org/10.3109/10428194.2011.568078
- Germain C, Guillaudeux T, Galsgaard ED, Hervouet C, Tekaya N, Gallouet AS, Fassy J, Bihl F, Poupon G, Lazzari A et al. Lectin-like transcript 1 is a marker of germinal center-derived B-cell non-Hodgkin's lymphomas dampening natural killer cell functions. Oncoimmunology 2015; 4:e1026503; PMID:26405582; http://dx.doi.org/10.1080/2162402X.2015.1026503
- God JM, Zhao D, Cameron CA, Amria S, Bethard JR, Haque A. Disruption of HLA class II antigen presentation in Burkitt lymphoma: implication of a 47,000 MW acid labile protein in CD4+ T-cell recognition. Immunology 2014; 142:492-505; PMID:24628049; http://dx.doi.org/10.1111/imm.12281
- Neelapu SS, Kwak LW, Kobrin CB, Reynolds CW, Janik JE, Dunleavy K, White T, Harvey L, Pennington R, Stetler-Stevenson M et al. Vaccine-induced tumor-specific immunity despite severe B-cell depletion in mantle cell lymphoma. Nat Med 2005; 11:986-91; PMID:16116429; http://dx.doi.org/10.1038/nm1290
- Wang L, Qian J, Lu Y, Li H, Bao H, He D, Liu Z, Zheng Y, He J, Li Y et al. Immune evasion of mantle cell lymphoma: expression of B7-H1 leads to inhibited T-cell response to and killing of tumor cells. Haematologica 2013; 98:1458-66; PMID:23508008; http://dx.doi.org/10.3324/haematol.2012.071340
- Xerri L, Chetaille B, Serriari N, Attias C, Guillaume Y, Arnoulet C, Olive D. Programmed death 1 is a marker of angioimmunoblastic T-cell lymphoma and B-cell small lymphocytic lymphoma/chronic lymphocytic leukemia. Hum Pathol 2008; 39:1050-8; PMID:18479731; http://dx.doi.org/10.1016/j.humpath.2007.11.012
- Dasanu CA, Bockorny B, Grabska J, Codreanu I. Prevalence and Pattern of Autoimmune Conditions in Patients with Marginal Zone Lymphoma: A Single Institution Experience. Conn Med 2015; 79:197-200; PMID:26259295
- Mittal D, Gubin MM, Schreiber RD, Smyth MJ. New insights into cancer immunoediting and its three component phases–elimination, equilibrium and escape. Curr Opin Immunol 2014; 27:16-25; PMID:24531241; http://dx.doi.org/10.1016/j.coi.2014.01.004
- Verhaak RG, Tamayo P, Yang JY, Hubbard D, Zhang H, Creighton CJ, Fereday S, Lawrence M, Carter SL, Mermel CH et al. Prognostically relevant gene signatures of high-grade serous ovarian carcinoma. J Clin Invest 2013; 123:517-25; PMID:23257362; http://dx.doi.org/10.1172/JCI65833
- Ycart B, Charmpi K, Rousseaux S, Fournie JJ. Large scale statistical analysis of GEO datasets. Gene Technol 2014; 3:1-9; http://dx.doi.org/10.4172/2329-6682.1000113
- Dunn GP, Old LJ, Schreiber RD. The three Es of cancer immunoediting. Annu Rev Immunol 2004; 22:329-60; PMID:15032581; http://dx.doi.org/10.1146/annurev.immunol.22.012703.104803
- Edgar R, Domrachev M, Lash AE. Gene Expression Omnibus: NCBI gene expression and hybridization array data repository. Nucleic Acids Res 2002; 30:207-10; PMID:11752295; http://dx.doi.org/10.1093/nar/30.1.207
- Harris MA, Clark J, Ireland A, Lomax J, Ashburner M, Foulger R, Eilbeck K, Lewis S, Marshall B, Mungall C et al. The Gene Ontology (GO) database and informatics resource. Nucleic Acids Res 2004; 32:D258-61; PMID:14681407; http://dx.doi.org/10.1093/nar/gkh066
- Liberzon A, Subramanian A, Pinchback R, Thorvaldsdottir H, Tamayo P, Mesirov JP. Molecular signatures database (MSigDB) 3.0. Bioinformatics 2011; 27:1739-40; PMID:21546393; http://dx.doi.org/10.1093/bioinformatics/btr260
- Tsodikov A, Szabo A, Jones D. Adjustments and measures of differential expression for microarray data. Bioinformatics 2002; 18:251-60; PMID:11847073; http://dx.doi.org/10.1093/bioinformatics/18.2.251
- Warnat P, Eils R, Brors B. Cross-platform analysis of cancer microarray data improves gene expression based classification of phenotypes. Bmc Bio Informatics 2005; 6:265; PMID:16271137; http://dx.doi.org/10.1186/1471-2105-6-265
- Charmpi K, Ycart B. Weighted Kolmogorov Smirnov testing: an alternative for Gene Set Enrichment Analysis. Statistical Applications Genetics Mol Biol 2015; 14:279-93; PMID:26030794; http://dx.doi.org/10.1515/sagmb-2014-0077
- Cui X, Churchill GA. Statistical tests for differential expression in cDNA microarray experiments. Genome Biol 2003; 4:210; PMID:12702200; http://dx.doi.org/10.1186/gb-2003-4-4-210
- Irizarry RA, Wang C, Zhou Y, Speed TP. Gene set enrichment analysis made simple. Stat Methods Med Res 2009; 18:565-75; PMID:20048385; http://dx.doi.org/10.1177/0962280209351908
- Mootha VK, Lindgren CM, Eriksson KF, Subramanian A, Sihag S, Lehar J, Puigserver P, Carlsson E, Ridderstråle M, Laurila E et al. PGC-1alpha-responsive genes involved in oxidative phosphorylation are coordinately downregulated in human diabetes. Nat Genet 2003; 34:267-73; PMID:12808457; http://dx.doi.org/10.1038/ng1180