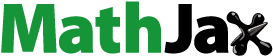
ABSTRACT
Chronic inflammation promotes breast tumor growth and invasion by accelerating angiogenesis and tissue remodeling in the tumor microenvironment. There is a complex relationship between inflammation and estrogen, which drives the growth of 70 percent of breast tumors. While low levels of estrogen exposure stimulate macrophages and other inflammatory cell populations, very high levels are immune suppressive. Breast tumor incidence is increased by obesity and age, which interact to influence inflammatory cell populations in normal breast tissue. To characterize the impact of these factors on tumors and the tumor microenvironment, we measured gene expression in 195 breast adenocarcinomas and matched adjacent normal breast tissue samples collected at Akershus University Hospital (AHUS). Age and Body Mass Index (BMI) were independently associated with inflammation in adjacent normal tissue but not tumors. Estrogen Receptor (ER)-negative tumors had elevated macrophage expression compared with matched normal tissue, but ER-positive tumors showed an unexpected decrease in macrophage expression. We found an inverse relationship between the increase in tumor estrogen pathway expression compared with adjacent normal tissue and tumor macrophage score. We validated this finding in 126 breast tumor-normal pairs from the previously published METABRIC cohort. We developed a novel statistic, the Rewiring Coefficient, to quantify the rewiring of gene co-expression networks at the level of individual genes. Differential correlation analysis demonstrated distinct pathways were rewired during tumorigenesis. Our data support an immune suppressive effect of high doses of estrogen signaling in breast tumor microenvironment, suggesting that this effect contributes to the greater presence of prognostic and therapeutically relevant immune cells in ER-negative tumors.
Abbreviations
BMI | = | Body Mass Index |
CTL | = | Cytotoxic Lymphocyte |
ER | = | Estrogen Receptor |
FDR | = | False Discovery Rate |
RC | = | Rewiring Coefficient |
TAM | = | Tumor Associated Macrophages |
Introduction
Increasing age affects breast cancer predisposition and development in complex ways. During the last century, epidemiologists noted that the slope of the breast cancer incidence curve shifts at approximately the age of menopause, and theorized that there were 2 distinct forms of breast cancer distinguished by the activity of hormones.Citation1,2 It is now known that breast tumors arising after menopause more frequently respond to mitogenic signaling through the estrogen receptor, and a woman's lifetime risk for breast cancer is correlated with her total estrogen exposure.Citation3 Circulating 17β-estradiol is a hormone primarily produced in the ovaries, but organs including the skin and breast can metabolize estrogen independently of the ovaries.Citation4 Estrogen has wide-ranging and complex effects on normal human physiology that change during sexual maturation, pregnancy, and menopause. Its most important and visible effects involve crucial roles in development and functioning of the post-natal female reproductive system. Circulating estrogen levels are highest in the pre-ovulatory phase of the menstrual cycle and during pregnancy and decrease precipitously post-menopause,Citation5 which typically occurs around age 50. Breast tumors have aromatase activity and can produce estrogen even after circulating estrogen levels have dropped to very low levels.Citation6
The response of macrophages and other inflammatory cell populations to estrogen stimulation depends on both the dose and the tissue context [reviewed inCitation7]. Moderate levels of estrogen increase the pro-angiogenic activity of inflammatory cells. However, estrogen exposure at levels found during pregnancy decreases cytokine production and suppresses the activity of inflammatory pathways such as NFKB.Citation8-13 During pregnancy, women with immune and inflammatory disorders such as rheumatoid arthritis often experience temporary relief of their symptoms.Citation14 Topical estrogen has been shown to improve epithelial wound healing in vivo by reducing the macrophage response.Citation15 There is therefore abundant evidence in vivo that elevated estrogen exposure can have immune suppressive and anti-inflammatory effects. The role of estrogen in inflammatory responses is particularly relevant to breast cancer, as the tumor microenvironment of breast cancer is heavily influenced by the tumor-promoting effects of tumor-associated macrophages (TAM).Citation16
Tumorigenesis results in dramatic changes in the overall levels of gene expression compared with normal tissue, in part due to changes in the proportions of different cell populations and deregulated growth signaling. However, not all important signaling and morphological changes can be detected by comparing gene expression levels. Differential correlation and differential network analysis have been previously proposed as methods to identify how relationships between genes or proteins change.Citation17 Some methods determine whether pre-specified gene sets are differently correlated in 2 conditions,Citation18,19 while others discover gene sets directly from the data.Citation20 We developed a new gene-level differential correlation summary statistic called the Rewiring Coefficient (RC) and use this approach to dissect the effect of tumorigenesis on both gene expression levels and relationships between genes.
The present study compared gene expression profiles of breast adenocarcinomas to matched adjacent normal tissue and tissue from disease-free women to characterize how gene networks affecting estrogen, basal keratins, and the tumor microenvironment are altered by tumorigenesis. Our observations suggest that the degree of prognostic and therapeutically relevant immune cells in ER-negative tumors is linked inversely to increases in estrogen signaling.
Results
Inflammation expression was elevated in adjacent normal tissue
We measured gene expression in 195 breast adenocarcinomas paired with adjacent normal tissue taken from the ipsilateral breast, for a total of 390 samples. Patient age, BMI, and immunohistochemical tumor types of these patients are summarized in . We also measured gene expression in breast tissue from 43 disease-free samples donated by healthy women undergoing mammaplastic reduction surgery. We first explored how the presence of a tumor altered the gene expression profile of nearby pathologically normal tissue, using mammaplastic reduction samples as controls. Principle component analysis of the entire data set indicated that adjacent normal and mammaplastic reduction samples were much more similar to each other than to tumor samples (Fig. S1). Comparison of reduction mammaplasty tissue to adjacent normal and tumor tissue identified 7,505 and 11,173 differentially expressed genes, respectively; comparison of comparing adjacent normal tissue to tumor tissue identified 12,590 genes to be differentially expressed. We examined markers of adipocytes, basal keratins, and the extracellular matrix, 3 of the key cell populations that make up breast tissue. Expression levels of the adipocyte-derived hormone adiponectin (ADIPOQ), the basal keratinocyte marker keratin 5 (KRT5), and the extracellular matrix adhesion protein fibronectin (FN1) were not significantly different between mammaplastic reduction and adjacent normal samples (). In both non-malignant sample types, expression levels of basal and adipose genes were inversely correlated, a relationship that was abolished in tumors (). Similarly, expression of basal and luminal keratin markers were directly correlated in non-malignant samples, but this correlation was abolished in tumors (). These results were compatible with non-malignant samples having comparable proportions and quantities of adipocytes, keratinocytes, and matrix cells ().
Table 1. Clinical summary of AHUS patients.
Figure 1. Adjacent normal tissue has an elevated acute inflammatory response (a) Box plots of gene expression for the adipose marker ADIPOQ, basal keratin 5 (KRT5), and extracellular matrix protein fibronectin 1 (FN1) in mammaplastic reduction (mam. red.), adjacent normal (adj. normal), and tumor tissue. All 3 genes show similar expression levels in levels in adjacent normal and mammaplastic reduction samples. (b) Scatter plots of expression of ADIPOQ vs. KRT5 and KRT8 vs. KRT5 show correlation relationships are consistent in normal adjacent and mammaplastic reduction tissue but disrupted in tumors. (c) Box plots of early response cytokines interleukin 1 β (IL1B), tumor necrosis factor (TNF) and suppressor of cytokine signaling 3 (SOCS3) show elevated expression in adjacent normal tissue, as do (d) box plots of the macrophage marker myeloid cell nuclear differentiation antigen (MNDA) and the dendritic cell marker CD83. (e) Scatter plots of age vs. expression of MSR1, GZMA, and CD247, and of BMI vs. ADIPOQ, LEP, and MSR1, all in adjacent normal tissue. All plots show statistically significant positive correlations except BMI vs. ADIPOQ.
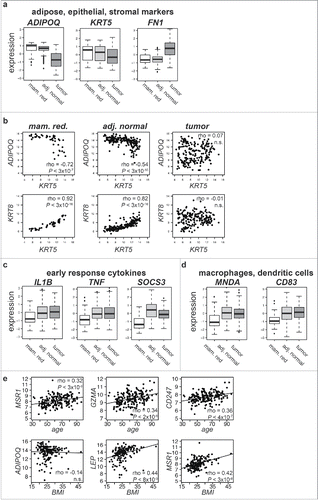
In contrast to expression of epithelial, matrix, and adipose markers, adjacent normal tissue had significantly elevated expression of cytokines associated with the acute inflammatory response, such as interleukin 1B (IL1B), tumor necrosis factor (TNF), and suppressor of cytokine signaling 3 (SOCS3), when compared with mammaplastic reduction samples (). Expression of markers specifically associated with macrophages, such as myeloid cell nuclear differentiation antigen (MNDA) and markers of dendritic cells, such as CD83, were also elevated in adjacent normal tissue (). Although the tissue adjacent to the tumor was pathologically benign and similar to disease-free breast tissue in its overall expression profile, it demonstrated expression features associated with elevated levels of cytokine activity and immune cell populations that respond to those cytokine signals when compared with mammaplastic reduction samples.
Age and BMI are independently associated with lymphocyte and macrophage expression in normal tissue
Breast cancer incidence increases with age due to a combination of age-related physiologic changes, exposure to environmental carcinogens, and random DNA mutations. Aging is also associated with decreased adaptive immune efficacy and increases in circulating monocytes.Citation21-23 Patient age was significantly correlated with the expression of 53 genes in adjacent normal tissues (P < 0.05, Holm's correction, Supplementary Table 3). This gene list was significantly enriched for genes important in the inflammatory response and positive regulation of T cell activation, such as the Macrophage Scavenger Receptor (MSR1), the cytolytic Granzyme A (GZMA), and the T cell receptor zeta (CD247) (). We summarized macrophage and cytotoxic lymphocyte (CTL) gene expression as pathway expression scores (Methods) and found that the scores were significantly associated with age in adjacent normal tissue (Macrophage score rho = 0.25, P = 0.0004; CTL score rho = 0.19, P = 0.007, Fig. S2) but not in tumors.
Obesity has been associated with increased macrophage infiltration in adipose tissue, including in normal breast tissue,Citation24-26 and with increased breast tumor incidence in post-menopausal women.Citation27,28 In the AHUS patients, BMI was not significantly associated with age or tumor subtype. Expression of markers of adipocytes such as ADIPOQ was not significantly correlated with patient BMI in adjacent normal tissue, but BMI was significantly associated with expression of leptin (LEP), a hormone secreted in adipose tissue whose levels are associated with BMICitation29 (). BMI was also significantly associated with MSR1 expression () and with macrophage pathway expression levels in adjacent normal tissue (BMI vs. macrophage adjusted rho = 0.48, P = 8 × 10−11, Fig. S3) but not in tumors. The fact that both age and BMI were positively associated with levels of inflammatory cell expression in non-malignant tissue is consistent with a model where these factors affect the microenvironment in which the tumor forms.
Later-onset ER-positive tumors have elevated expression of ESR1 mRNA and protein
It is unknown what tumor intrinsic or extrinsic factors may predispose the development of particular breast tumor subtypes. We attempted to identify associations between tumor subtype and gene expression in adjacent normal tissue. Considering all 195 patients together, no gene was significantly differentially expressed in adjacent normal tissue from patients with ER-positive tumors compared with that of patients with ER-negative tumors, and no gene pair was significantly differentially correlated between these groups. We next performed an analysis stratified by patient age, categorizing patients into early, middle, and late disease onset age groups (<50 years, 50 to 70 years, and >70 y respectively). We chose 50 y because it is the average age of the onset of menopause. We summarized gene expression levels of key genes and pathways in normal and tumor tissue grouped by age and immunohistochemical subtype, plotting the median expression in the gene or pathway standardized against its expression in mammaplastic reduction samples as a baseline (a). Performing differential expression analysis within each age group revealed that 3,980 genes were significantly differentially expressed in the adjacent normal tissue of women with early-onset disease when stratified by the ER status of their tumors (Supplementary Table 4).
Figure 2. Expression of key genes and pathways in adjacent normal and tumor samples. (a) Relative expression of individual genes and pathways compared with 43 disease-free mammaplastic reduction tissue samples, grouped by age and hormone receptor status. Values were standardized by subtracting the corresponding median value for in mammaplastic reduction samples and dividing by the standard deviation across groups. Darker blue indicates lower expression than the median expression of all mammaplastic reduction, darker red indicates higher expression. (b) scatter plot of the fold-change in ESR1 expression plotted against patient age in ER-positive HER2-negative AHUS patients with a linear regression line fitted, showing a significant direct correlation.
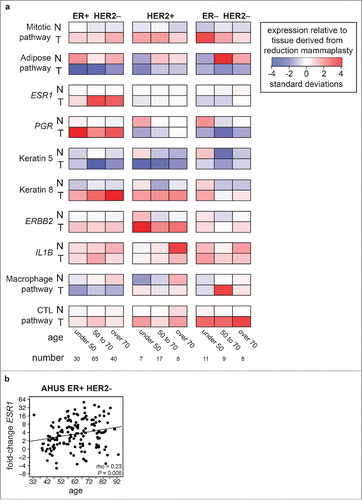
The expression level of estrogen receptor α (ESR1) in adjacent normal tissue was not significantly associated with age of disease onset or tumor ER status (a, Fig. S4). However, women with early-onset ER-negative tumors had significantly higher expression of estrogen response pathway genes, luminal, and basal markers, and significantly lower expression of markers of adipocytes in adjacent normal tissue (a, Fig. S4). These differences were not simply the result of age-related reductions in circulating hormone levels or decreases in parenchymal density, as age-matched women with ER-positive tumors did not show this expression phenotype. It was unlikely that elevated hormone levels in normal tissue resulted from the presence of elevated estrogen levels in nearby tumor tissue, since this expression pattern was identified only in women with ER-negative tumors. These differences were compatible with early-onset ER-negative women possessing denser breast tissue, which would result in a higher relative expression of epithelial markers such as KRT5 and KRT8.
The largest significant differences in expression levels when comparing adjacent normal tissue to tumors were increases in expression of mitotic pathway genes and decreases in adipogenesis pathway genes (). Women with later-onset ER-positive tumors had significantly higher increases in the expression of ESR1 compared with women with early-onset ER-positive tumors (1.6-fold vs. 4.3-fold, P = 5 × 10−6, Wilcoxon rank sum test, ). Constructing a linear model for change in ESR1 expression identified a modest but significant relationship between later age of onset for ER-positive tumors and larger increases in ESR1 expression (rho = 0.23, P = 0.005, ). This result confirmed earlier studies performed by tissue microarray.Citation30,31
We validated the association between older age and levels of tumor ESR1 using mRNA from the METABRIC cohort (rho = 0.139, P < 2 × 10−16, Fig. S5a).Citation32 To rule out the possibility that this effect was confounded by the larger proportion of Luminal B tumors in older women compared with younger women, we confirmed this effect was present within Luminal A METABRIC tumors (Fig. S5b). This association was also found in protein expression data from 408 breast tumors published by the TCGA (rho= 0.42, P = 9 × 10−15, Fig. S5c).Citation33 These data emphasize that age is an important covariate when assessing ESR1 mRNA and protein expression levels in breast tumors.
Change in macrophage score varied inversely with change in ESR1 expression in ER-positive tumors
We next performed a more detailed analysis of immune cell gene expression. All types of tumors had significantly higher CTL pathway scores compared with mammaplastic reduction or adjacent normal tissue (). CTL pathway scores were highest in HER2-positive and ER-negative/HER2-negative tumors. The difference in tumor CTL scores compared with matched adjacent normal tissue was 2-fold higher in patients with ER-negative tumors compared with patients with ER-positive tumors (). Unlike the CTL scores, macrophage pathway scores were not significantly different between tumors and adjacent normal tissue when considering all 195 patients. However, when we stratified the analysis by tumor subtype, we found that macrophage scores were significantly lower in ER-positive, and in particular ER-positive/HER2-negative tumors, than in matched adjacent normal tissue (, ).
Table 2. Difference in paired macrophage and CTL scores by tumor subtype.
Figure 3. Stronger estrogen pathway expression in ER-positive tumors is associated with lower tumor inflammation (a) Macrophage pathway expression score in paired adjacent normal (black points) and tumors (blue points) in ER-positive/HER2-negative, ER-positive/HER2-positive, ER-negative/HER2-positive, and ER-negative/HER2-negative patients. Samples from the same patient are connected by a vertical line. The majority of ER-positive tumors express lower levels of macrophage markers than their matched adjacent normal tissue. (b,c) Fold-change in expression of ESR1 plotted against fold-change in macrophage score in ER-positive HER2-negative (b) AHUS and (c) METABRIC samples, demonstrating a significant inverse linear relationship.
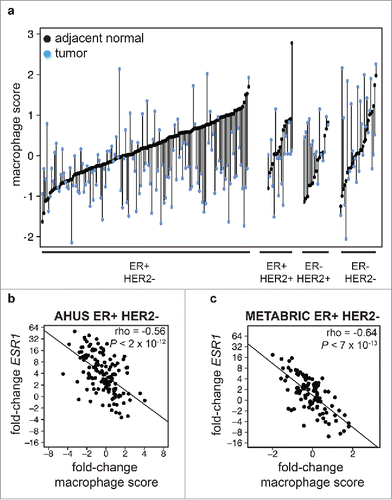
To identify potential drivers of macrophage activity in ER-positive tumors, we identified genes whose difference in expression was significantly correlated with the change in macrophage score. The change in macrophage pathway score was inversely correlated with the change in ESR1 expression in ER-positive but not ER-negative patients (rho = −0.56, P < 2 × 10−12, ). Women with the largest increase in ESR1 expression in their tumor compared with adjacent normal tissue had the largest decrease in tumor macrophage pathway score. We confirmed this finding in an independent cohort of 124 matched tumor and adjacent normal samples from the METABRIC study (rho = −0.64, P < 7 × 10−13, ). These results were compatible with increased estrogen in ER-positive tumors acting in an immune-suppressive manner on macrophages, although other explanations cannot be ruled out from these correlative observations.
Basal gene networks were rewired in ER-positive tumors compared with adjacent normal tissue
We next combined correlation analysis and differential expression analysis to dissect how the pathways described above are rewired during tumorigenesis. In normal breast ducts, luminal and basal cells grow in a bilayer, and expression levels of luminal and basal keratins are highly correlated. Luminal and estrogen-responsive genes such as GATA3 were strongly upregulated in ER-positive tumors compared with adjacent normal tissue (). Correlation between expression of GATA3 and basal genes such as KIT and KRT5 was significant in adjacent normal tissue but not significant in ER-positive tumors (). In contrast to estrogen-driven and luminal markers, basal genes such as KRT14, TRIM29, and PROM1 did not significantly change expression levels in ER-positive tumors. These genes lost correlation with the luminal and mesenchymal pathways with which they were very strongly correlated in adjacent normal tissue (). The loss of correlation without changes in expression levels suggested an increase in the luminal but not the basal compartment of the tissue. Genes with roles regulating cell migration, epithelial-mesenchymal interactions, and epithelial differentiation such as Fibroblast growth factor 7 (FGF7), an epithelial mitogen produced in mesenchymal cells,Citation34 tended to gain correlation but decrease in expression levels in tumors. FGF7 was expressed at 1.8-fold lower levels in ER-positive tumors than in adjacent normal tissue, but only in tumors was its expression inversely correlated with proliferation and luminal markers, and only in tumors was its expression directly correlated with that of other markers of epithelial differentiation such as decorin, caveolin 1, and ZEB2 ().
Figure 4. Gene expression network rewiring in ER-positive tumors (a-c) Heat maps of standardized gene expression for genes significantly differentially correlated with (a) GATA3 (b) KRT14 and (c) FGF7 in matched adjacent normal and ER-positive tumors, sorted by GATA3, KRT14, and FGF7 expression respectively. The condition where the target gene was significantly correlated with the other genes in each plot is indicated (all P < 1 × 10−17). Correlation measurements are listed in Table S5. (d-e) Scatter plot of Rewiring Coefficient (RC) analysis of gene expression in adjacent normal compared with matched ER-positive tumors, with RC for each gene plotted on the X axis and the t statistic for difference in means plotted on the Y axis. Genes with significant differential correlation (P < 0.05 after Holm's correction) plotted as round points; non-significant genes plotted as open triangles. Point color indicates whether correlation was stronger in tumors (darker red) or adjacent normal tissue (darker blue). (e) RC analysis using plotted using data from (d), showing representative pathway members listed in Table S6.
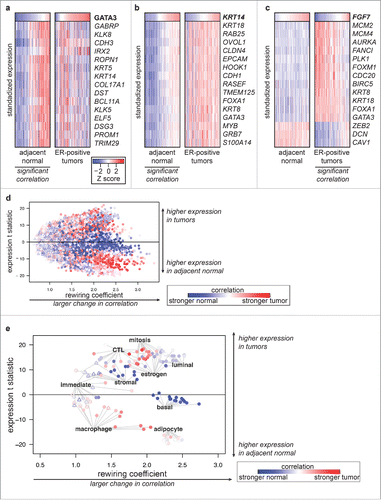
To analyze these trends in an unbiased, genome-wide manner we developed a novel summary statistic, the Rewiring Coefficient (RC). This statistic quantifes the extent to which each gene's expression relationships are altered between 2 states (Methods). We then plotted each gene's RC value against its differential expression statistic to identify pathway alterations (). Highlighting the correlation and expression level alterations of genes representing the basal, luminal, CTL, and other pathways () illustrated several routes to deregulation of homeostatic pathways: 1) higher expression and loss of existing correlation networks (luminal, estrogen response, stromal genes); 2) higher expression and gain of new correlation networks (mitotic genes); 3) lower expression and gain of correlation networks (macrophage genes); 4) no change in expression levels, and loss of correlation networks (basal genes). In the case of the basal gene network, differential correlation identified a pathway alteration that would not have been identified from differential expression analysis alone.
Discussion
This study compared gene expression in breast adenocarcinomas to gene expression in matched adjacent normal tissue and tissue from women who underwent mammaplastic reduction surgery. Both adjacent normal and mammaplastic reduction tissues have been used as normal controls in studies of breast tumors. Adjacent normal tissue has the advantage that it is easily obtainable at the time of surgery and is matched to tumor tissue in germline genotypes, age, BMI, and other covariates. Adjacent normal tissue from women with tumors had elevated expression of inflammatory genes and a macrophage gene signature compared with samples from mammaplastic reduction surgery, arguing that while these samples are a valuable source of information about the milieu in which the tumor formed, they are not truly normal. Breast adenocarcinomas are initiated in luminal ductal or lobular cells, but the tumors are a product of both the initiated epithelium and the stroma that surrounds and pervades that epithelium. Expression analysis of breast tumor stroma has been shown to have predictive power for clinical outcome,Citation35,36 evidence of cross-talk between the tumor and adjacent non-malignant tissue. The tumor microenvironment is a complex mix of structural, inflammatory, and immune cells.Citation37-40
Differences in patient age and BMI between breast cancer patients and mammaplastic reduction patients complicate the interpretation of this study.
One potential disadvantage of using samples from mammaplastic reduction surgery as true normal controls is that these patients are on average 20 y younger than women with non-familial breast cancer, and are more likely to be overweight or obese.Citation41 Women generally undergo mammaplastic reduction surgery before the average age of breast cancer onset, and we showed that increasing age had a modest but significant influence on macrophage gene expression scores. However, increased BMI also had a significant influence on macrophage gene expression scores, so it is not obvious to what extent or direction controls from younger women with higher BMI would be confounded when compared with older cases with a range of BMI scores. A previous report comparing gene expression in mammaplastic reduction and adjacent normal samples did not identify a statistically significant difference in expression levels.Citation42 In that study Finak et al. analyzed microdissected samples from a smaller number of individuals than the present report (adjacent normal tissue from 34 patients with invasive ductal carcinoma and 10 patients undergoing reduction). Microdissection provides targeted insights into the physiology of specific cell compartments, which is complementary analysis to whole-tissue samples, which have the potential to provide information about the relationship between epithelial and immune cells in the tumor microenvironment.
The use of BMI as a surrogate measure of obesity has been criticized because BMI does not distinguish between mass due to fat vs. mass due to lean tissue such as muscle.Citation43 Although BMI is an imperfect measure of obesity, BMI has been shown to be an meaningful proxy for direct measurement of obesity in studies of female sex hormone concentration,Citation44 and meta-analysis of large-scale population studies has shown elevated BMI is associated with increased post-menopausal breast cancer risk,Citation45,46 and increased weight is linked to increased quantities of available circulating estrogen.Citation27,28 Excess android (abdominal and upper-body) adipose accumulation is also associated with increased levels of inflammatory activity driven by macrophages resident in adipose tissue.Citation24,26,47 Our analysis identified age- and BMI-associated increases in inflammation and suggested that these effects act independently and additively (summarized in ). Although BMI may also influence macrophage infiltration into tumors, we did not observe a direct association between BMI and macrophage score in any subtype of tumor. This is compatible with the primary effect of BMI on breast cancer susceptibility acting indirectly on inflammation and estradiol production in the tumor microenvironment rather than within the tumor itself. This result was consistent with the finding presented above that BMI was strongly associated with elevated macrophage expression score in all adjacent normal tissue samples. It may be that women with higher BMI have smaller increases in their macrophage expression score because of the microenvironment surrounding their tumors already had an elevated presence of macrophages.
Figure 5. Summary of age, BMI, and differential correlation results (a) BMI and age independently increase inflammation in adjacent normal tissue (arrow). Older age is associated with larger increases in ESR1 expression in tumors (arrow). Larger increases in estrogen levels from adjacent normal to tumor are inversely associated with tumor macrophage scores (dotted line). (b,c) Differential correlation pathway summary, where solid lines indicate direct correlation and dotted lines indicate inverse correlation in either (b) adjacent normal or (c) ER-positive tumors. Node color indicates relative expression levels compared with adjacent normal (gray), where red indicates increased and blue indicates decreased expression. Individual genes emblematic of the pathway are noted on the figure.
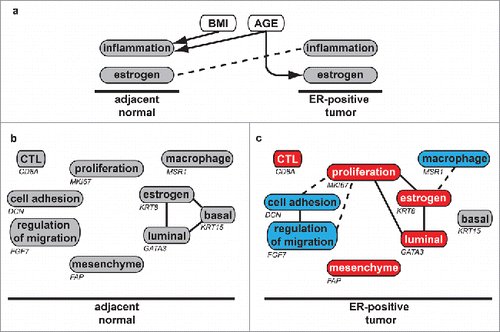
ER-negative tumors tend to be larger, of higher grade, and with more developed vascular infrastructure than ER-positive tumors. ER-negative, Basal-like tumors have been associated with elevated expression of a Colony Stimulating Factor 1 (CSF1) expression signature,Citation48,49 reviewed in.Citation50 The present study found that tumors from women with ER-negative disease had elevated expression of macrophage pathways compared with matched adjacent normal tissue. In contrast, we found that in women with ER-positive tumors, there was an overall decrease in macrophage pathway expression, and that the larger the increase in ESR1 expression in the tumor compared with adjacent normal tissue, the lower the macrophage expression score ().
This study did not directly quantify either the amount of estradiol in tumors and adjacent normal tissue or the number of infiltrating macrophages in tumors. These data are compatible with an inverse correlation between macrophage activity and estrogen exposure in ER-positive but not ER-negative tumors, which could be explained by the anti-inflammatory effects of estrogen which have been extensively described.Citation7 However, we cannot rule out the opposite effect: that reduced macrophage infiltration in ER-positive tumors causes larger production of ESR1 and other genes in the estrogen response pathway. Estradiol and cytokine production in the tumor and tumor microenvironment likely influence each other both positively and negatively. Macrophages in the breast can contribute to estrogen production in situ,Citation51,52 and macrophage and lymphocyte synthesis of cytokines may be a major source of estradiol in breast tumors.Citation53 The presence in tumors of infiltrating cytotoxic T cells is significantly associated with better survival in ER-negative but not ER-positive breast tumors.Citation54
This study used differential correlation analysis to illustrate major pathway and cell composition alterations that occur during the transition from normal tissue to ER-positive breast cancer (summarized in , ). We defined the Rewiring Coefficient, method that characterizes the extent to which a gene's relationship to the rest of the genome is altered between 2 states. ER-positive tumors are sometimes characterized as expressing lower levels of canonical basal markers such as KRT5 and EGFR. We found that while markers of luminal gene expression such as KRT8 were expressed at higher levels in ER-positive tumors compared with matched adjacent normal tissue, basal marker expression remained constant. Differential correlation analysis indicated that despite similar levels of expression, there was a profound rewiring of the relationship between basal keratins, luminal keratins, and the estrogen response pathway.
This study provides evidence that the association of the levels of both adaptive and innate immune cells in breast tumors with ER status may be directly linked to the anti-inflammatory and anti-immune effects of estrogen. Elevated BMI was associated with molecular markers of inflammation in normal tissue but not tumors. The Rewiring Coefficient method has the potential to identify different cell populations and deregulated growth signaling and may be useful in studies of tumor heterogeneity.
Materials and methods
Statistical methods
Statistical calculations were performed in the R statistical programming environment.Citation55 Where adjusted r2 is reported, the test performed was a linear regression. Spearman rank correlation rho was reported for correlation analysis. Interaction was tested by ANOVA. Differential expression was calculating using Significance Analysis of MicroarraysCitation56 with significant differences assessed at a 5% False Discovery Rate. Correlation between age or BMI and gene expression was corrected for multiple testing using Holm's method. Pathway enrichment was calculated using BiNGOCitation57 with P values corrected by Benjamini & Hochberg's FDR method. Values in were calculated by paired t test. Gene signatures were downloaded from MSigDB version 4.0Citation58 Macrophage pathway genes were selected calculating correlation between expression of CD14, CD11B, and CD163 and all other genes in all AHUS samples, identifying the top 100 genes from each of the 3 calculations, and then selecting those genes which appeared on the intersection of all 3 lists. Basal and Luminal pathway genes were selected as the 40 genes whose expression was most significantly correlated with expression of KRT5 and KRT8 respectively. The gene composition of all signatures used in this manuscript is listed in Supplementary Table 1. METABRIC gene expression data were downloaded from the European Genome-Phenome Archive, accession EGAS00000000083. TCGA protein expression data were downloaded from the TCGA data portal (https://tcga-data.nci.nih.gov/tcga). R code to reproduce the microarray normalization and the complete analysis can be downloaded from http://davidquigley.com/publications.
Rewiring coefficient analysis
Fisher's Z transformation converts Pearson's r or Spearman's rho to a normally distributed z′ value with standard error (se) described as:where n is the number of elements. The difference of 2 z′ values, zdiff, is approximately standard normally distributed and is calculated as:
The Rewiring Coefficient (RC) for a gene is the sum of zdiff scores for differential correlation between a gene and all other genes under consideration, normalized by the number of genes. For N genes g1..N the RC for gene i is:
RC significance was calculated by permutation testing, which randomly shuffled class assignments and generated empirical permutation P values for each gene. False Discovery Rate estimation was then performed using the qvalue package in R. We implemented the RC calculation in an open-source C++ software package called spear, which also calculates correlation and differential correlation. The spear program is multi-threaded and can natively exploit multi-core cluster environments. spear source code and executable code is freely available at http://github.com/davidquigley/QuantitativeGenetics.
Patient population
The 195 breast cancer patients in this study (the AHUS cohort) were seen at the Akershus hospital in Norway during the last 10 y. BMI was available for 162 patients and ranged from 16.9 to 46.8, with a median of 24.8. Forty-seven percent of the patients had a BMI above 25 and 16% had a BMI above 30, frequently used standards for overweight and obesity. AHUS patient's ages at the time of surgery ranged from 31 to 96 years, with a median value of 61.2 y. This study was approved by REC SouthEast (Regional Ethical Committee for Medical and Health Research Ethics) and by the Ethical Committee for Aarhus county and by “Datatilsynet” (The Data Inspectorate, an independent administrative body under the Ministry of Government Administration and Reform). All patients gave consent to the use of material for research purposes in adherence with the Declaration of Helsinki Principles. Supplementary Table 2 provides clinical characterization for all subjects including BMI, age, and tumor staging.
Sample preparation
Tissue samples were stabilized with RNAlater at the time of surgery. After surgery, RNAlater was removed and tissue was stored at −80°C. RNA was extracted with TRIzol (Invitrogen, Carlsbad CA) or TRI reagent (Life Technologies, Gaithersburg MD) and further purified on RNeasy columns in combination with the RNeasy Mini Kit (Qiagen, Valenca, CA). RNA was quantified using a NanoDrop ND-1000 spectrophotometer (Thermo Scientific, Wilmington DE) and quality checked with an Agilent 2100 Bioanalyzer (Agilent Technologies, Santa Clara, CA). mRNA expression levels were measured using the Agilent Technologies Agilent-014850 Whole Human Genome Microarray 4 × 44K and the Agilent G4851A SurePrint G3 Human Gene Expression 8 × 60K microarray platforms scanned by an Agilent scanner (Agilent Technologies, Santa Clara USA). Raw microarray data were quantile normalized using the limma package in R.Citation59 Missing values were imputed using the impute package in R. Individual probes were re-annotated by aligning published probe sequences to human reference sequence HG19 using BLAT.Citation60 Platforms were combined by collapsing all probes annotated to a single gene into one value. To combine multiple probes for one gene into a single value we used the mean of multiple probes annotated to match a single gene if they were correlated at rho ≥ 0.8; if no pair of probes was correlated at rho ≥ 0.8, the probe with the highest variance was used. Platforms were then batch corrected using ComBat.Citation61 14,637 genes were expressed above background levels.
Data availability
Microarray data can be accessed from the GEO database, (http://www.ncbi.nlm.nih.gov/geo) with accession number GSE70951.
Disclosure of potential conflicts of interest
No potential conflicts of interest were disclosed.
Authors contribution
DAQ performed bioinformatic analysis and wrote the manuscript. AT performed microarray assays and helped draft the manuscript. TL and MHR performed microarray assays. AB and ALBD supervised the study. IRB contributed clinical samples. VK conceived of, designed, and supervised the study. All authors read and approved the final manuscript.
Database References
GEO: GSE70951
KONI_A_1356142_Supplementary_materials.zip
Download Zip (3.6 MB)Acknowledgments
We are thankful to Dr. Torill Sauer and Dr. Ying Chenfor immunostaining and pathological review. This study was supported by NCI grants CA084244–15 and CA141455–01 (A. Balmain), DNK grants 4196283563 (to A.-L. Børresen-Dale) and 4196163832 (to V. Kristensen), NFR-FUGE grant 193387/V50 (to A.-L. Børresen-Dale and V. Kristensen) from the Norwegian Research Council, and Helse Sør-Øst grant 2011042 from the South-Eastern Norway Regional Health Authority. AT was a PhD fellow of the Norwegian Health authorities, region South-East, grant number 271914, and the operating costs for the array analysis were covered by grant number 272904 from the same agency.
References
- Clemmesen J. Carcinoma of the breast; results from statistical research. Br J Radiol. 1948;21(252):583-90. doi:10.1259/0007-1285-21-252-583. PMID:18099749
- de Waard F, de Laive J, Baanders-Vanhalewijn EA. On the bimodal age distribution of mammary carcinoma. Br J Cancer. 1960;14:437-48. doi:10.1038/bjc.1960.47. PMID:13782349
- Paffenbarger RS Jr., Kampert JB, Chang HG. Characteristics that predict risk of breast cancer before and after the menopause. Am J Epidemiol. 1980;112(2):258-68. doi:10.1093/oxfordjournals.aje.a112992. PMID:7416152
- Ohnemus U, Uenalan M, Inzunza J, Gustafsson JA, Paus R. The hair follicle as an estrogen target and source. Endocr Rev. 2006;27(6):677-706. doi:10.1210/er.2006-0020. PMID:16877675
- Gruber CJ, Tschugguel W, Schneeberger C, Huber JC. Production and actions of estrogens. N Engl J Med. 2002;346(5):340-52. doi:10.1056/NEJMra000471. PMID:11821512
- Lu Q, Nakmura J, Savinov A, Yue W, Weisz J, Dabbs DJ, Wolz G, Brodie A. Expression of aromatase protein and messenger ribonucleic acid in tumor epithelial cells and evidence of functional significance of locally produced estrogen in human breast cancers. Endocrinology. 1996;137(7):3061-8. doi:10.1210/endo.137.7.8770932. PMID:8770932
- Straub RH. The complex role of estrogens in inflammation. Endocr Rev. 2007;28(5):521-74. doi:10.1210/er.2007-0001. PMID:17640948
- Stein B, Yang MX. Repression of the interleukin-6 promoter by estrogen receptor is mediated by NF-kappa B and C/EBP beta. Mol Cell Biol. 1995;15(9):4971-9. doi:10.1128/MCB.15.9.4971. PMID:7651415
- Ghisletti S, Meda C, Maggi A, Vegeto E. 17beta-estradiol inhibits inflammatory gene expression by controlling NF-kappaB intracellular localization. Mol Cell Biol. 2005;25(8):2957-68. doi:10.1128/MCB.25.8.2957-2968.2005. PMID:15798185
- Chadwick CC, Chippari S, Matelan E, Borges-Marcucci L, Eckert AM, Keith JC Jr, Albert LM, Leathurby Y, Harris HA, Bhat RA, et al. Identification of pathway-selective estrogen receptor ligands that inhibit NF-kappaB transcriptional activity. Proc Natl Acad Sci U S A. 2005;102(7):2543-8. doi:10.1073/pnas.0405841102. PMID:15699342
- Galien R, Garcia T. Estrogen receptor impairs interleukin-6 expression by preventing protein binding on the NF-kappaB site. Nucleic Acids Res. 1997;25(12):2424-9. doi:10.1093/nar/25.12.2424. PMID:9171095
- Polan ML, Daniele A, Kuo A. Gonadal steroids modulate human monocyte interleukin-1 (IL-1) activity. Fertil Steril. 1988;49(6):964-8. doi:10.1016/S0015-0282(16)59945-2. PMID:2967196
- Rogers A, Eastell R. The effect of 17beta-estradiol on production of cytokines in cultures of peripheral blood. Bone. 2001;29(1):30-4. doi:10.1016/S8756-3282(01)00468-9. PMID:11472888
- Da Silva JA, Spector TD. The role of pregnancy in the course and aetiology of rheumatoid arthritis. Clin Rheumatol. 1992;11(2):189-94. doi:10.1007/BF02207955. PMID:1617891
- Ashcroft GS, Mills SJ, Lei K, Gibbons L, Jeong MJ, Taniguchi M, Burow M, Horan MA, Wahl SM, Nakayama T. Estrogen modulates cutaneous wound healing by downregulating macrophage migration inhibitory factor. J Clin Invest. 2003;111(9):1309-18. doi:10.1172/JCI16288. PMID:12727922
- Pollard JW. Tumour-educated macrophages promote tumour progression and metastasis. Nat Rev Cancer. 2004;4(1):71-8. doi:10.1038/nrc1256. PMID:14708027
- Ideker T, Krogan NJ. Differential network biology. Mol Syst Biol. 2012;8:565. doi:10.1038/msb.2011.99. PMID:22252388
- Braun R, Cope L, Parmigiani G. Identifying differential correlation in gene/pathway combinations. BMC Bioinformatics. 2008;9:488. doi:10.1186/1471-2105-9-488. PMID:19017408
- Choi Y, Kendziorski C. Statistical methods for gene set Co-expression analysis. Bioinformatics. 2009;25:2780-6. doi:10.1093/bioinformatics/btp502. PMID:19689953
- Watson M. CoXpress: Differential co-expression in gene expression data. BMC Bioinformatics. 2006;7:509. doi:10.1186/1471-2105-7-509. PMID:17116249
- Krabbe KS, Pedersen M, Bruunsgaard H. Inflammatory mediators in the elderly. Exp Gerontol. 2004;39(5):687-99. doi:10.1016/j.exger.2004.01.009. PMID:15130663
- Della Bella S, Bierti L, Presicce P, Arienti R, Valenti M, Saresella M, Vergani C, Villa ML. Peripheral blood dendritic cells and monocytes are differently regulated in the elderly. Clin Immunol. 2007;122(2):220-8. doi:10.1016/j.clim.2006.09.012. PMID:17101294
- Shaw AC, Joshi S, Greenwood H, Panda A, Lord JM. Aging of the innate immune system. Curr Opin Immunol. 2010;22(4):507-13. doi:10.1016/j.coi.2010.05.003. PMID:20667703
- Sun X, Casbas-Hernandez P, Bigelow C, Makowski L, Joseph Jerry D, Smith Schneider S, Troester MA. Normal breast tissue of obese women is enriched for macrophage markers and macrophage-associated gene expression. Breast Cancer Res Treat. 2012;131(3):1003-12. doi:10.1007/s10549-011-1789-3. PMID:22002519
- Pirone JR, D'Arcy M, Stewart DA, Hines WC, Johnson M, Gould MN, Yaswen P, Jerry DJ, Smith Schneider S, Troester MA. Age-associated gene expression in normal breast tissue mirrors qualitative age-at-incidence patterns for breast cancer. Cancer Epidemiol Biomarkers Prev. 2012;21(10):1735-44. doi:10.1158/1055-9965.EPI-12-0451. PMID:22859400
- Weisberg SP, McCann D, Desai M, Rosenbaum M, Leibel RL, Ferrante AW Jr. Obesity is associated with macrophage accumulation in adipose tissue. J Clin Invest. 2003;112(12):1796-808. doi:10.1172/JCI200319246. PMID:14679176
- Key TJ, Appleby PN, Reeves GK, Roddam A, Dorgan JF, Longcope C, Stanczyk FZ, Stephenson HE Jr, Falk RT, Miller R, et al. Body mass index, serum sex hormones, and breast cancer risk in postmenopausal women. J Natl Cancer Inst. 2003;95(16):1218-26. doi:10.1093/jnci/djg022. PMID:12928347
- van den Brandt PA, Spiegelman D, Yaun SS, Adami HO, Beeson L, Folsom AR, Fraser G, Goldbohm RA, Graham S, Kushi L, et al. Pooled analysis of prospective cohort studies on height, weight, and breast cancer risk. Am J Epidemiol. 2000;152(6):514-27. doi:10.1093/aje/152.6.514. PMID:10997541
- Maffei M, Halaas J, Ravussin E, Pratley RE, Lee GH, Zhang Y, Fei H, Kim S, Lallone R, Ranganathan S, et al. Leptin levels in human and rodent: Measurement of plasma leptin and ob RNA in obese and weight-reduced subjects. Nat Med. 1995;1(11):1155-61. doi:10.1038/nm1195-1155. PMID:7584987
- Eppenberger-Castori S, Moore DH Jr, Thor AD, Edgerton SM, Kueng W, Eppenberger U, Benz CC. Age-associated biomarker profiles of human breast cancer. Int J Biochem Cell Biol. 2002;34(11):1318-30. doi:10.1016/S1357-2725(02)00052-3. PMID:12200028
- Yau C, Fedele V, Roydasgupta R, Fridlyand J, Hubbard A, Gray JW, Chew K, Dairkee SH, Moore DH, Schittulli F, et al. Aging impacts transcriptomes but not genomes of hormone-dependent breast cancers. Breast Cancer Res. 2007;9(5):R59. doi:10.1186/bcr1765. PMID:17850661
- Curtis C, Shah SP, Chin SF, Turashvili G, Rueda OM, Dunning MJ, Speed D, Lynch AG, Samarajiwa S, Yuan Y, et al. The genomic and transcriptomic architecture of 2,000 breast tumours reveals novel subgroups. Nature. 2012;486(7403):346-52. doi:10.1038/nature10983. PMID:22522925
- Cancer_Genome_Atlas_Network. Comprehensive molecular portraits of human breast tumours. Nature. 2012;490(7418):61-70. doi:10.1038/nature11412. PMID:23000897
- Finch PW, Rubin JS, Miki T, Ron D, Aaronson SA. Human KGF is FGF-related with properties of a paracrine effector of epithelial cell growth. Science. 1989;245(4919):752-5. doi:10.1126/science.2475908. PMID:2475908
- Finak G, Bertos N, Pepin F, Sadekova S, Souleimanova M, Zhao H, Chen H, Omeroglu G, Meterissian S, Omeroglu A, et al. Stromal gene expression predicts clinical outcome in breast cancer. Nat Med. 2008;14(5):518-27. doi:10.1038/nm1764. PMID:18438415
- Ma XJ, Dahiya S, Richardson E, Erlander M, Sgroi DC. Gene expression profiling of the tumor microenvironment during breast cancer progression. Breast Cancer Res. 2009;11(1):R7. doi:10.1186/bcr2222. PMID:19187537
- Coussens LM, Pollard JW. Leukocytes in mammary development and cancer. Cold Spring Harb Perspect Biol. 2011;3(3):1-2. doi:10.1101/cshperspect.a003285. PMID:21123394
- Condeelis J, Pollard JW. Macrophages: Obligate partners for tumor cell migration, invasion, and metastasis. Cell. 2006;124(2):263-6. doi:10.1016/j.cell.2006.01.007. PMID:16439202
- Hanahan D, Coussens LM. Accessories to the crime: Functions of cells recruited to the tumor microenvironment. Cancer Cell. 2012;21(3):309-22. doi:10.1016/j.ccr.2012.02.022. PMID:22439926
- Sica A, Allavena P, Mantovani A. Cancer related inflammation: The macrophage connection. Cancer Lett. 2008;267(2):204-15. doi:10.1016/j.canlet.2008.03.028. PMID:18448242
- Fryzek JP, Ye W, Nyrén O, Tarone RE, Lipworth L, McLaughlin JK. A nationwide epidemiologic study of breast cancer incidence following breast reduction surgery in a large cohort of Swedish women. Breast Cancer Res Treat. 2006;97(2):131-4. doi:10.1007/s10549-005-9099-2. PMID:16328720
- Finak G, Sadekova S, Pepin F, Hallett M, Meterissian S, Halwani F, Khetani K, Souleimanova M, Zabolotny B, Omeroglu A, et al. Gene expression signatures of morphologically normal breast tissue identify basal-like tumors. Breast Cancer Res. 2006;8(5):R58. doi:10.1186/bcr1608. PMID:17054791
- Prentice AM, Jebb SA. Beyond body mass index. Obes Rev. 2001;2(3):141-7. doi:10.1046/j.1467-789x.2001.00031.x. PMID:12120099
- Mahabir S, Baer DJ, Johnson LL, Hartman TJ, Dorgan JF, Campbell WS, Clevidence BA, Taylor PR. Usefulness of body mass index as a sufficient adiposity measurement for sex hormone concentration associations in postmenopausal women. Cancer Epidemiol Biomarkers Prev. 2006;15(12):2502-7. doi:10.1158/1055-9965.EPI-06-0499. PMID:17164376
- Renehan AG, Tyson M, Egger M, Heller RF, Zwahlen M. Body-mass index and incidence of cancer: A systematic review and meta-analysis of prospective observational studies. Lancet. 2008;371(9612):569-78. doi:10.1016/S0140-6736(08)60269-X. PMID:18280327
- Huang Z, Hankinson SE, Colditz GA, Stampfer MJ, Hunter DJ, Manson JE, Hennekens CH, Rosner B, Speizer FE, Willett WC. Dual effects of weight and weight gain on breast cancer risk. JAMA. 1997;278(17):1407-11. doi:10.1001/jama.1997.03550170037029. PMID:9355998
- Wisse BE. The inflammatory syndrome: The role of adipose tissue cytokines in metabolic disorders linked to obesity. J Am Soc Nephrol. 2004;15(11):2792-800. doi:10.1097/01.ASN.0000141966.69934.21. PMID:15504932
- Beck AH, Espinosa I, Edris B, Li R, Montgomery K, Zhu S, Varma S, Marinelli RJ, van de Rijn M, West RB. The macrophage colony-stimulating factor 1 response signature in breast carcinoma. Clin Cancer Res. 2009;15(3):778-87. doi:10.1158/1078-0432.CCR-08-1283. PMID:19188147
- DeNardo DG, Brennan DJ, Rexhepaj E, Ruffell B, Shiao SL, Madden SF, Gallagher WM, Wadhwani N, Keil SD, Junaid SA, et al. Leukocyte complexity predicts breast cancer survival and functionally regulates response to chemotherapy. Cancer Discov. 2011;1(1):54-67. doi:10.1158/2159-8274.CD-10-0028. PMID:22039576
- Qian BZ, Pollard JW. Macrophage diversity enhances tumor progression and metastasis. Cell. 2010;141(1):39-51. doi:10.1016/j.cell.2010.03.014. PMID:20371344
- Miller WR, O'Neill J. The importance of local synthesis of estrogen within the breast. Steroids. 1987;50(4–6):537-48. doi:10.1016/0039-128X(87)90037-7. PMID:3504615
- Mor G, Yue W, Santen RJ, Gutierrez L, Eliza M, Berstein LM, Harada N, Wang J, Lysiak J, Diano S, et al. Macrophages, estrogen and the microenvironment of breast cancer. J Steroid Biochem Mol Biol. 1998;67(5–6):403-11. doi:10.1016/S0960-0760(98)00143-5. PMID:10030689
- Purohit A, Newman SP, Reed MJ. The role of cytokines in regulating estrogen synthesis: Implications for the etiology of breast cancer. Breast Cancer Res. 2002;4(2):65-9. doi:10.1186/bcr425. PMID:11879566
- Ali H, Provenzano E, Dawson SJ, Blows FM, Liu B, Shah M, Earl HM, Poole CJ, Hiller L, Dunn JA, et al. Association between CD8+ T-cell infiltration and breast cancer survival in 12 439 patients. Ann Oncol. 2014;25:1536-43. doi:10.1093/annonc/mdu191. PMID:24915873
- R_Core_Team. R: A language and environment for statistical computing. Vienna. 2012. http://www.R-project.org
- Tusher VG, Tibshirani R, Chu G. Significance analysis of microarrays applied to the ionizing radiation response. Proc Natl Acad Sci USA. 2001;98(9):5116-21. doi:10.1073/pnas.091062498. PMID:11309499
- Maere S, Heymans K, Kuiper M. BiNGO: A Cytoscape plugin to assess overrepresentation of gene ontology categories in biological networks. Bioinformatics. 2005;21(16):3448-9. doi:10.1093/bioinformatics/bti551. PMID:15972284
- Subramanian A, Tamayo P, Mootha VK, Mukherjee S, Ebert BL, Gillette MA, Paulovich A, Pomeroy SL, Golub TR, Lander ES, et al. Gene set enrichment analysis: A knowledge-based approach for interpreting genome-wide expression profiles. Proc Natl Acad Sci U S A. 2005;102(43):15545-50. doi:10.1073/pnas.0506580102. PMID:16199517
- Smyth G. Limma: Linear models for microarray data. In Gentleman R, editor. Bioinformatics and computational biology solutions using R and bioconductor. New York: Springer. 2005. doi:10.1007/0-387-29362-0
- Kent WJ. BLAT–the BLAST-like alignment tool. Genome Res. 2002;12(4):656-64. doi:10.1101/gr.229202. PMID:11932250
- Johnson WE, Li C, Rabinovic A. Adjusting batch effects in microarray expression data using empirical Bayes methods. Biostatistics. 2007;8(1):118-27. doi:10.1093/biostatistics/kxj037. PMID:16632515