ABSTRACT
The relation between tumor mutation burden (TMB) and outcome of cancer patients receiving immunotherapy has been reported. This study aimed to evaluate the prognostic role of TMB in cancer patients receiving immunotherapy. Databases including Embase, PubMed, and the Cochrane library were systematically searched to identify potentially eligible studies until Sep 2018 without language limitation. Studies assessing high versus low TMB in predicting survival of various cancer patients were selected. The pooled analyses were conducted using hazard ratio (HR) of high versus low TMB for overall survival (OS) and progression-free survival (PFS), and the odds ratio (OR) for overall response rate (ORR). The primary endpoint was OS. Secondary outcomes were PFS and ORR. A total of 45 studies consisting of 103078 cancer patients were included. The combined results showed that high TMB was associated with better OS (HR = 0.40; 95% confidence interval (CI):0.30–0.53; p< .00001), PFS (HR = 0.37; 95% CI: 0.26–0.53; p< .00001) and ORR (OR = 4.62; 95%CI: 2.90–7.34; p< .0001) when treated with immunotherapy. In studying patients with high TMB, these patients had improved OS (HR = 0.69; 95%CI: 0.47–1.03; p= .07) when comparing immunotherapy to chemotherapy. Subgroup analyses suggested that the prognostic role of TMB was independent of cancer types and TMB detection methods (all p< .05). Our findings suggest that high TMB is associated with better survival in cancer patients receiving immunotherapy. For cancer patients with high TMB, immunotherapy could be considered.
Introduction
In recent years, the treatment landscape of cancer has changed dramatically with promising outcomes.Citation1,Citation2 These treatments include immunotherapy and targeted therapy. Along with these improvements, the biomarkers have also changed from imaging to genetic alterations.Citation3 These factors, such as programmed cell death 1/ligand 1(PD-1/L-1) agents,Citation3,Citation4 are widely used in anti-cancer treatments. One of the emerging prognostic factors is tumor mutation burden (TMB).Citation3
With the advantage of sequencing technology, it is able to determine the TMB in different cancer types and to evaluate its correlation with outcomes.Citation5,Citation6 In 2013, a studyCitation7 by Birkbak and colleagues evaluated the relationship between progression-free survival (PFS) and overall survival (OS) and tumor mutation burden in high-grade serous ovarian cancers, and found that high mutation burden patients had good outcomes from surgery and chemotherapy. Later, the associations between TMB and efficacy of immunotherapy in melanoma and lung cancer patients were evaluated. The studyCitation8 of Snyder et al. showed that clinical benefits of immune checkpoint inhibitors (ICIs) were related to TMB in melanoma patients. Recently, other studiesCitation5,Citation9 also suggested that TMB was correlated with the efficacy of immunotherapy in lung cancer patients. Investigators also evaluated the association between TMB and outcomes of cancer patients treated with chemotherapy. However, the results are not in accordance and still in debate.
In this study, we evaluated the prognostic effect of high versus low TMB on OS, PFS, and ORR in various cancer patients treated with ICIs and/or non-ICIs, aimed to answer the following concerns: First, is high TMB a reliable biomarker for immunotherapy, regardless of cancer types, and TMB detection methods? Second, for patients with high TMB, could they have better outcomes when treated with immunotherapy compared to chemotherapy.
Results
Search results
Through preliminary search, 650 articles were obtained. After removing duplication, 174 studies were discarded and the remaining 476 articles were initially included. After reading the title and the abstract, 419 were further excluded. For the remaining 57 studies, another 12 studies were discarded after screening the full-text. Forty-five studiesCitation5–Citation49 were finally considered as eligible. Altogether 103078 patients diagnosed with lung cancer, melanoma, breast cancer, multiple myeloma, and other types were included. The literature screening process and study selection results are shown in Supplemental Figure 1.
The baseline characteristics of the included studies are listed in . Briefly, four of these studies were high-quality RCTs and the rest were retrospective studies. The publication dates ranged from 2013 to 2018. Most (35/45) of the studies were reported from North America. The reported treatments were immunotherapy, surgery, chemotherapy, and small molecular-targeted therapy. The agents of immunotherapy were mainly immune checkpoint inhibitors, such as Nivolumab, Atezolizumab, Pembrolizumab, and Ipilimumab. The cutoff value for defining high versus low TMB ranged from 3.3 to 203.
Table 1. Baseline characteristics of included studies.
Overall quality of the included studies
88.9% of the included studies were considered with moderate to high quality (Supplemental Table 1). Four randomized trials were included and considered with high quality. The NOS was used to assess the overall quality of the included studies. Five studies were considered as low quality because they had less than 6 criteria star. Selection and outcome bias were the main reasons for lowering the overall quality.
Tumor mutation burden on survival and efficacy of anti-cancer treatments
There were 25 studiesCitation5,Citation8–Citation11,Citation13,Citation14,Citation16,Citation17,Citation20–Citation23,Citation25,Citation27,Citation29–Citation32,Citation34,Citation38,Citation39,Citation42,Citation44,Citation46 reported survival of cancer patients treated with ICIs, and 11 studiesCitation7,Citation26,Citation33,Citation35–Citation37,Citation41–Citation43,Citation47,Citation48 reported survival in patients with non-ICIs.
Overall survival
To assess the relationship between TMB and OS, we extracted HRs of high versus low TMB for OS and performed meta-analyses. With regard to the OS of cancer patients treated with ICIs, a number of 10Citation8,Citation10,Citation16,Citation17,Citation27,Citation29,Citation31,Citation34,Citation39,Citation40 studies were included. As illustrated in , the high risk of heterogeneity was indicated by I2 so the random effect model was applied. For patients treated with ICIs, the pooled result suggested that the OS was significantly improved in cancer patients with high TMB (HR = 0.40; 95%CI: 0.30–0.53; p< .00001), when compared with those with low TMB. In order to evaluate the impact of time on the reliance of the pooled OS, we also performed cumulative analysis (Supplemental Figure 2). The result was in accordance with the above finding, indicating a good reliance. For OS of patients treated with non-ICIs, the combined result found a negative role of high TMB (HR = 1.61; 95%CI: 0.85–3.06; p= .14) on OS compared to those with low TMB, though it was not significant () lower section). In studying patients with high TMB in trials that compared ICI to chemotherapy, high TMB patients had better OS when treated with ICI compared to chemotherapy (HR = 0.69; 95%CI: 0.47–1.03; p= .07), though there was no statistical significance ()).
Figure 1. Combined analysis of HR of high versus low tumor mutation burden for OS in various cancer patients treated with or without immunotherapy, and immunotherapy versus chemotherapy in cancer patients with high tumor mutation burden. (a): Above, pooled HR for patients treated with immunotherapy. High tumor mutation burden is in favor of improved OS (n = 620, p< .01); Below, Pooled HR for patients without immunotherapy. High tumor mutation burden is associated with increased risk of death in these patients (n = 3286, p= .14). (b): Evaluating effects of immunotherapy versus chemotherapy on OS in cancer patients with high tumor mutation burden. OS was improved for patients from the immunotherapy group, compared to those in the chemotherapy group (n = 1462, p= .07).
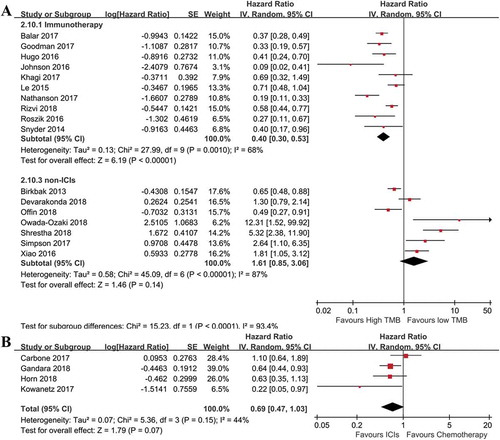
Subgroup analysis of overall survival
Due to the significant heterogeneity, we introduced subgroup analyses to minimize the impact of differences across studies on the pooled results. These analyses were performed in terms of cancer types, and TMB detection methods.
We examined the impact of cancer types on OS. High TMB was associated with prolonged OS in melanoma, lung cancer, and other cancer types, indicating TMB could be a universal prognostic factor for immunotherapy ()). To determine whether different TMB detection methods could influence the results, the WES and targeted NGS subgroups were introduced. The synthesized HR for OS was 0.55(95%CI: 0.44–0.69; p< .00001) for the WES subgroup, and 0.27(95%CI: 0.18–0.42; p< .00001) for the targeted NGS group ()), suggesting that high TMB determined by targeted NGS may be correlated with better OS than that of WES.
Figure 2. Subgroup analyses of factors that may affect the pooled HR for OS. (a): Subgroup analysis of OS in term of cancer types. The prognostic role of tumor mutation burden was independent of cancer types (n = 196 for melanoma, n = 240 for NSCLC, n = 1484 for multiple cancers. overall p< .01). (b): Subgroup analysis of OS with regard to detection methods of tumor mutation burden. The prognostic role of tumor mutation burden was independent of detection methods (p< .01).
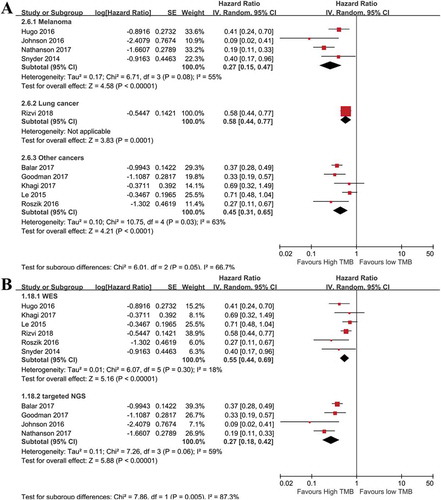
For patients with non-ICIs treatment, the subgroup analysis with regard to cancer types showed that high TMB was correlated with worse OS in patients with lung cancer, HCC, and melanoma, but not for ovarian cancer patients. This indicated the predictive roles of TMB on OS should be determined based on the cancer type (Supplemental Figure 3).
Progression-free survival
After pooling relevant data together, significant heterogeneity between the included studies was found (I2% = 79%), and the random effect model was applied. The overall result from ) presented that ICIs treated cancer patients with high TMB had a significantly lower risk of disease progression (HR = 0.37; 95%CI: 0.26–0.53; p< .00001). The cumulative analysis result indicated a good reliance (Supplemental Figure 4). For patients with high TMB, a significantly better PFS (HR = 0.61; 95%CI: 0.51–0.73; p< .00001) was observed in patients treated with ICIs compared to chemotherapy ()). However, in cancer patients treated with non-ICIs, high TMB was associated with increased risk of disease progression when compared to patients with low TMB. The above results suggested that patients with high TMB may be an indicator of immunotherapy rather than chemotherapy.
Figure 3. Combined analysis of HR of high versus low tumor mutation burden for PFS in various cancer patients treated with or without immunotherapy, and immunotherapy versus chemotherapy in cancer patients with high tumor mutation burden. (a): pooled HR of high versus low tumor mutation burden for patients treated with immunotherapy or not. High tumor mutation burden is in favor of improved OS (n = 440 for immunotherapy, p< .01), but associated with worse outcomes for patients treated without immunotherapy (n = 3480 for non-immunotherapy, p= .01). (b): Evaluating the effects of immunotherapy versus chemotherapy on PFS in cancer patients with high tumor mutation burden. PFS was significantly prolonged in patients receiving immunotherapy, compared to those with chemotherapy (n = 2078, p< .01).
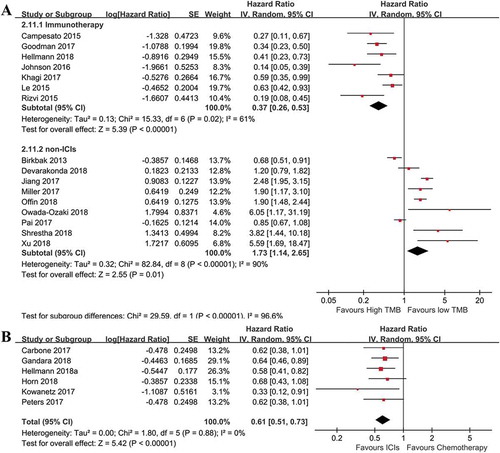
Subgroup analysis of progression-free survival
Subgroup analyses for PFS were performed similarly as those of the OS. As shown in , the prognostic role of TMB on PFS was independent of cancer types ()) and TMB detection methods ()). The HRs of high versus low TMB for PFS were 0.41(95%CI: 0.22–0.76; p= .005), 0.14(95%CI: 0.05–0.39; p= .0002), and 0.50(95%CI: 0.33–0.74; p= .0007) in lung cancer, melanoma, and other cancer patients, respectively. With regard to non-ICIs treatment, high TMB patients with various cancers exhibited different outcomes (Supplemental Figure 5), suggesting TMB in determining the prognosis of patients treated with non-ICIs should be dependent on specific cancer types.
Figure 4. Subgroup analyses of PFS with regard to cancer types, and tumor mutation burden detection methods. (a): Subgroup analysis of OS in term of cancer types. The prognostic role of tumor mutation burden was similar in enrolled cancer types (n = 65 for melanoma, n = 2442 for NSCLC, n = 261 for multiple cancers. overall p< .01). (b): Subgroup analysis of PFS using different detection methods of tumor mutation burden. The prognostic role of tumor mutation burden was not significantly affected by detection methods (p< .05).
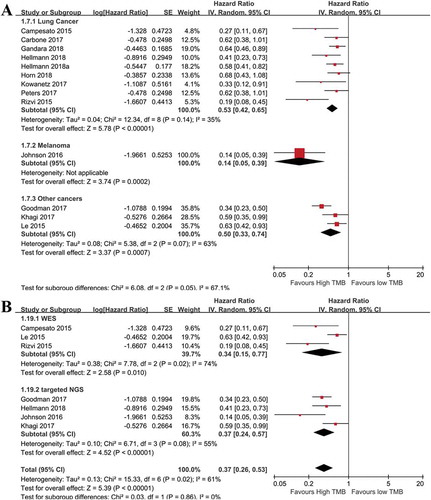
Objective response
Seventeen studiesCitation5,Citation6,Citation8,Citation10,Citation13, Citation14,Citation16,Citation17,Citation20,Citation21,Citation27,Citation29,Citation30,Citation32,Citation34,Citation39,Citation40 reported median value of TMB in cancer patients, and 11 studies presented the median value of TMB in responders and non-responders to immunotherapy. As shown in Supplemental Table 2, the median TMB values of responders (ranged from 8.5 to 654) were higher than those without responding (ranges: 5–281).
Twelve studies compared the TMB of responders vs. non-responders to immunotherapy with quantitative analysis. Most of the statistical methods used were Mann–Whitney, and Wilcoxon rank sum analysis (Supplemental Table 3). We combined the individual data by the Fisher method and found that high TMB was significantly associated with response to immunotherapy (Chi2= 137.46, p< .0001).
Next, this effect of TMB on response to ICIs was validated by pooling complete response and partial response data. As illustrated in ), there were 155 (44.03%) patients in the high TMB group (n = 352), and 134(17.72%) patients in the low TMB group (n = 756), and the meta-analysis result showed that patients with high TMB had a significantly better objective response to ICIs than those with low TMB (ORR = 4.62; 95%CI: 2.90–7.34; p< .00001).
Figure 5. Meta-analysis of overall response rate in immunotherapy treated patients with high versus low tumor mutation burden, and publication bias assessment. (a): Meta-analysis of overall response rate in cancer patients with high versus low tumor mutation burden after immunotherapy (N = 1108, p< .01). (b): Funnel plot of OS from included studies. (c): Funnel plot of PFS from included studies.
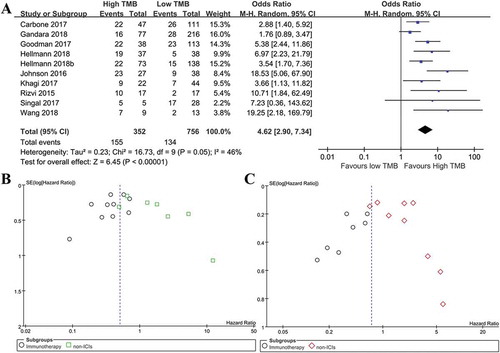
Tumor mutation burden
Correlation between different tumor mutation burden detection methods
There are several methods to determine the TMB, and WES and targeted NGS are the most common ones. WES has an advantage of a wide range to detect mutations. In recent years, studies are focusing on optimizing coverage of targeted genes to make TMB test more accurate and convenient. Nine studies evaluated the correlation between different TMB detection methods.Citation9,Citation11,Citation12,Citation15,Citation18,Citation22,Citation24,Citation27,Citation39 As shown in Supplemental Table 4, the correlation between WES and targeted NGS ranged from 0.24 to 0.995, indicating TMB measured by targeted NGS was not so reliable. However, the panel of targeted genes for NGS varied between studies.
Correlation between PD-1/L-1 and tumor mutation burden
PD-1 and PD-L1 are important biomarkers for predicting the efficacy of immunotherapy. There were seven studiesCitation11,Citation17,Citation19,Citation22,Citation39,Citation45,Citation49 aimed to explore the relation between PD-1/L-1 expression and TMB (Supplemental Table 5). The results showed that the correlation between these different biomarkers was not significant (all p> .05).
Publication bias assessment
The funnel plot was conducted using OS and PFS data. The symmetry of the funnel graphs are good for OS and PFS (), suggesting that the effects of TMB on OS (Begg’s Test: p= .837; Egger’s test: p= .380) and PFS (Begg’s Test: p= .753; Egger’s test: p= .332) are less likely to be affected by publication bias.
Discussion
Immunotherapy has been widely used in clinical practice for treating various cancers, especially in NSCLC and melanoma. However, only a subset of these patients benefits from this promising treatment. Therefore, it is urgent to identify promising prognostic markers for immune checkpoint blockades to help to guide the personalized treatment. In this study, we included studies evaluating the prognostic role of TMB on the survival of cancer patients with various anti-cancer treatments. The results confirmed that the TMB was a reliable biomarker to predict the efficacy of immunotherapy, regardless of cancer types and TMB detection methods. For studies assessing the efficacy of immunotherapy versus chemotherapy in high TMB patients, immunotherapy treated cases showed better outcomes. However, for various cancer patients treated with surgery or chemotherapy, the prognostic role of TMB was dependent on cancer type. With regard to response to ICIs, high TMB group had a significantly higher response rate than that in the low TMB group.
Interestingly, we find that in studies comparing the efficacy of immunotherapy with chemotherapy in high TMB patients, immunotherapy treated patients have better survival when compared to chemotherapy. It seems immunotherapy is a better option for patients with high TMB. However, it still needs to be validated in future trials. Our findings suggest that high TMB is associated with clinical benefits of ICIs compared to low TMB group, both in survival and response rates. This predictive role of TMB is not influenced by cancer type and detection methods of TMB. Whether other factors could affect its predictive role in immunotherapy, it is not examined in the present analysis and still worthy to clarify in the future studies. These factors are the definition of TMB, cutoff values for defining high TMB, units of TMB, sample types, sample obtain timing, gene panel of targeted NGS, and region. In our study, the cutoff values for high TMB ranged from 3.3 to 203. This wide range of TMB may be caused by the different definitions, and calculation method of TMB across included studies, as well as patient and disease heterogeneity. Another concern is the samples for detecting TMB. The tumor samples are difficult to obtain after diagnosis and treatment, especially in patients with advanced diseases. So researches are focusing on the correlation between the blood sample and tissue sample in determining TMB. The results from those studies showed a good correlation between TMB detected from blood and tissue samples. But this evidence still needs to be validated in more clinical studies.
Though high TMB is an indicator for immunotherapy, it is not the same in cancer patients treated with non-ICIs. The results of our study find that TMB is still associated with outcomes, but it depends on tumor types. Our pooled analysis suggests that high TMB is associated with worse survival in NSCLC, melanoma, multiple myeloma, hepatocellular carcinoma, and breast cancer patients, but correlated with better survival in ovarian cancer patients. With regard to treatments, chemotherapy, and surgery are the most common options for these patients. When cancer patients harboring different levels of TMB, it is important to consider what kind of treatments should be administrated in order to expect a better outcome.
Previous studies have revealed several promising biomarkers for predicting the efficacy of ICIs, such as immune-related adverse events (irAEs),Citation50 PD-1/L-1 expression,Citation51 neutrophil-lymphocyte ratio (NLR),Citation52 mismatch repair deficiency (MRD),Citation53 and tumor-infiltrating lymphocytes (TIL).Citation19 Most of these markers have been evaluated both in clinical trials and meta-analysis and exhibited varied advantages and disadvantages. There are evidencesCitation50,Citation54 supporting that irAEs are associated with better survival in patients receiving antibody of immune checkpoints. This may be reflecting the enhanced immune function by ICIs. However, these markers cannot be detected or observed before treatment, limiting its application in selecting patients. Though positive PD-1/L1 expression is suggested to be connected with the efficacy of ICIs,Citation17,Citation19 the samples are difficult to obtain during and after treatment. The cutoff value and definition of positive expression of PD-1/L1 may be different between studies. Our previous studyCitation52 suggests that baseline NLR is a reliable and feasible biomarker for immunotherapy. It has the advantages of easy measurement and low cost and should be evaluated in more RCTs. For MRD, the FDA has approved pembrolizumab for patients with this genetic abnormality. These cancers share a common feature of high mutations.Citation53 The time to approve ICIs for cancer patients with high TMB may be not long. There will be more immunotherapy predictors emerging in the future, and optimal utilization of these markers is needed. For example, the combination of baseline TMB, MRD, PD-1/L-1 expression and/or NLR is used to select patients who may benefit from ICIs before treatment, the irAEs are used to assistant monitoring efficacy. However, this should be validated in clinical practice first.
There are several limitations within the present study. First, the heterogeneity across studies is significant, though we performed subgroup analysis and sensitivity analysis. The baseline characteristics varied between studies, such as treatments, samples, and detection methods of TMB. The sources of heterogeneity are mainly from study design, patient types, and the cutoff value of TMB. The subgroup analysis of cutoff value was not done due to insufficient data. In this study, we just used the definition of high versus low TMB from the original-included studies and combined them together to explore the impact of TMB on the efficacy of immunotherapy. To minimize the influence of heterogeneity of cutoff value, we introduced various subgroup analyses. Secondly, reporting of primary and secondary endpoints are limited in some included studies and resulted in less data for meta-analysis. While some studies reported HR for OS, they did not present HR for PFS and/or ORR. In addition, the publication bias assessment of PFS finds a significant risk of selection bias, indicating grey literature or unpublished data could not be included in the analysis. These may lower or overestimate the overall effect of TMB on predicting outcomes. Thirdly, only a few RCTs are included and others were retrospective ones. This may increase the selection bias, though we performed the quality assessment. Besides, five included studies were only conference abstracts, decreasing the overall quality of the included studies. To overcome these shortcomings, we introduced subgroup analysis, sensitivity analysis, publication bias assessment, and cumulative meta-analysis to minimize the impact of heterogeneity on the combined analysis. The strength of our findings remains reliable.
Conclusion
The results of this study show that TMB is a reliable biomarker in predicting treatment outcomes of cancer patients receiving ICIs, and this effect is independent of cancer types and TMB detection methods. The prognostic role of TMB varies in cancer patients treated with non-ICIs and is dependent on tumor types. Further RCTs are needed to verify our findings.
Materials and methods:
Literature search and selection criteria
Databases including Embase, PubMed, and the Cochrane library were systematically searched to identify potentially eligible studies until Sep 2018 without language limitation. Recommended reference from reviews, clinical guidelines, comments, and relevant publications were also searched and considered. Studies assessing high TMB versus low TMB in predicting efficacy and survival of various cancer patients were selected. The processes of study identification, data extraction, and quality assessment were performed by two reviewers, independently. The studies were considered as eligible if they met the following criteria: 1) Patients: cancer diagnosed by cytology or pathology and imaging exams; 2) Type of Study: Randomized controlled clinical trial (RCT) and/or retrospective studies; 3) Intervention: ICIs and/or non-ICIs; 4) Outcomes: Primary endpoint was OS, and secondary endpoints were PFS, and objective response rate (ORR). Reviews, guidelines, conference abstracts without sufficient data about primary and secondary outcomes, comments, expert opinion, and animal studies were discarded.
Data collection and quality assessment
For baseline characteristics, the information about the first author, publication year, number of participants, male, study design, region, age, disease type, treatment, the cutoff value of TMB, detection method of TMB, and main reporting outcomes were collected. For combined analysis, HRs of high versus low TMB for OS and PFS in cancer patients with various treatments were extracted. The number of responders to immunotherapy in high and low TMB groups was collected. The spearman values for evaluating the correlation between different TMB detection methods (mainly WES and targeted next-generation sequencing (NGS)) as well as the association between PD-1/L1 expression and TMB level were also extracted. These data were evaluated by two reviewers, independently.
To assess the overall quality of the included studies, the Newcastle Ottawa Scale (NOS) that recommended by the Cochrane Non-Randomized Studies Methods Working Group was used.Citation55 According to the protocol,Citation56 selection, comparability, and outcome were mainly evaluated. There are four, one and three criteria items in concerns of selection, comparability and outcome, independently. The definition of a high-quality study was at least six NOS criteria stars. The process of quality assessment was performed by D Cao and H Xu, independently. A third reviewer (X Xu) was involved if there was a disagreement.
Statistical analyses
The software RevMan 5.3 was used to perform the meta-analysis. The general statistical methods were the same as previously described.Citation57 The definition of high or low TMB was recorded according to the individual included studies. In specific, the pooled analyses were conducted using hazard ratio (HR) of high TMB versus low TMB for progression-free survival and overall survival, and odds ratio (OR) for objective response rate. The chi-squared (χ2 or Chi2) test and I2 value were used to assess the heterogeneity of the included studies, and different I2 indicated a variable degree of heterogeneity according to the Cochrane Handbook 5.1.Citation55 The random effect model was used if indicated by I2 result. Otherwise, the fixed effect model was used. If HR was not directly reported by the study, it was calculated using methods previously described.Citation58,Citation59
The subgroup analyses of OS and PFS were performed in terms of cancer types, treatment types, region, and TMB detection methods. Cumulative meta-analysis was performed to evaluate the influence of time on the combined prognostic effect of TMB on OS and PFS. Sensitivity analysis was also performed to find the sources of heterogeneity if necessity. Egger’s test and Begg’s test were used to assess the publication bias. For heterogeneity assessment, a p less than 0.1 is suggested a significance. For all combined analysis, a p that less than 0.05 is considered as there is a statistical significance.
Disclosure of Potential Conflicts of Interest
No potential conflicts of interest were disclosed.
Novelty and Impact
For patients treated with immunotherapy, high TMB is a favorable factor for survival and efficacy. For cancer patients with high TMB, they could have better clinical benefits from immunotherapy compared to chemotherapy.
Supplemental Material
Download MS Word (24 KB)Supplemental Material
Download MS Power Point (1.7 MB)Supplemental Material
Download MS Word (12.6 KB)Supplementary material
Supplemental data for this article can be accessed on the publisher’s website.
Additional information
Funding
References
- Staňková K, Brown JS, Dalton WS, Gatenby RA. Optimizing cancer treatment using game theory: A review. JAMA Oncol. 2019;5(1):96–103. doi:10.1001/jamaoncol.2018.3395.
- Dijkstra KK, Voabil P, Schumacher TN, Voest EE. Genomics- and transcriptomics-based patient selection for cancer treatment with immune checkpoint inhibitors: a review. JAMA Oncol. 2016;2:1490–1495. doi:10.1001/jamaoncol.2016.2214.
- Steuer CE, Ramalingam SS. Tumor mutation burden: leading immunotherapy to the era of precision medicine? J Clin Oncol. 2018;36:631–632. doi:10.1200/JCO.2017.76.8770.
- Lemery S, Keegan P, Pazdur R. First FDA approval agnostic of cancer site — when a biomarker defines the indication. N Engl J Med. 2017;377:1409–1412. doi:10.1056/NEJMp1709968.
- Rizvi NA, Hellmann MD, Snyder A, Kvistborg P, Makarov V, Havel JJ, Lee W, Yuan J, Wong P, Ho TS, et al. Cancer immunology. Mutational landscape determines sensitivity to PD-1 blockade in non-small cell lung cancer. Science. 2015;348:124–128. doi:10.1126/science.aaa1348.
- Van AEM, Miao D, Schilling B, Sa S, Blank C, Zimmer L, Sucker A, Hillen U, Mhg F, Sm G, et al. Genomic correlates of response to CTLA-4 blockade in metastatic melanoma. Science. 2015;350:207–211. doi:10.1126/science.aad0095.
- Birkbak NJ, Kochupurakkal B, Izarzugaza JM, Eklund AC, Li Y, Liu J, Szallasi Z, Matulonis UA, Richardson AL, Iglehart JD, et al. Tumor mutation burden forecasts outcome in ovarian cancer with BRCA1 or BRCA2 mutations. PloS One. 2013;8:e80023. doi:10.1371/journal.pone.0080023.
- Snyder A, Makarov V, Merghoub T, Yuan J, Zaretsky JM, Desrichard A, Walsh LA, Postow MA, Wong P, Ho TS, et al. Genetic basis for clinical response to CTLA-4 blockade in melanoma. N Engl J Med. 2014;371:2189–2199. doi:10.1056/NEJMoa1406498.
- Gandara DR, Paul SM, Kowanetz M, Schleifman E, Zou W, Li Y, Rittmeyer A, Fehrenbacher L, Otto G, Malboeuf C, et al. Blood-based tumor mutational burden as a predictor of clinical benefit in non-small-cell lung cancer patients treated with atezolizumab. Nat Med. 2018;24:1441–1448. doi:10.1038/s41591-018-0134-3.
- Hugo W, Zaretsky JM, Sun L, Song C, Moreno BH, Hu-Lieskovan S, Berent-Maoz B, Pang J, Chmielowski B, Cherry G, et al. Genomic and transcriptomic features of response to anti-PD-1 therapy in metastatic melanoma. Cell. 2016;165:35–44. doi:10.1016/j.cell.2016.02.065.
- Hellmann MD, Callahan MK, Awad MM, Calvo E, Ascierto PA, Atmaca A, Rizvi NA, Hirsch FR, Selvaggi G, Szustakowski JD, et al. Tumor mutational burden and efficacy of nivolumab monotherapy and in combination with ipilimumab in small-cell lung cancer. Cancer Cell. 2018;33:853–61 e4. doi:10.1016/j.ccell.2018.04.001.
- Chalmers ZR, Connelly CF, Fabrizio D, Gay L, Ali SM, Ennis R, Schrock A, Campbell B, Shlien A, Chmielecki J, et al. Analysis of 100,000 human cancer genomes reveals the landscape of tumor mutational burden. Genome Med. 2017;9:34. doi:10.1186/s13073-017-0424-2.
- Carbone DP, Reck M, Paz-Ares L, Creelan B, Horn L, Steins M, Felip E, van Den Heuvel MM, Ciuleanu TE, Badin F, et al. First-line nivolumab in stage IV or recurrent non-small-cell lung cancer. N Engl J Med. 2017;376:2415–2426. doi:10.1056/NEJMoa1613493.
- Campesato LF, Barroso-Sousa R, Jimenez L, Correa BR, Sabbaga J, Hoff PM, Reis LF, Galante PA, Camargo AA. Comprehensive cancer-gene panels can be used to estimate mutational load and predict clinical benefit to PD-1 blockade in clinical practice. Oncotarget. 2015;6:34221–34227. doi:10.18632/oncotarget.5950.
- Davis AA, Chae YK, Agte S, Pan A, Simon NI, Taxter TJ, Behdad A, Carneiro BA, Cristofanilli M, Giles FJ. Comparison of tumor mutational burden (TMB) across tumor tissue and circulating tumor DNA (ctDNA). J Clin Oncol. 2017;35.
- Goodman AM, Kato S, Bazhenova L, Patel SP, Frampton GM, Miller V, Stephens PJ, Daniels GA, Kurzrock R. Tumor mutational burden as an independent predictor of response to immunotherapy in diverse cancers. Mol Cancer Ther. 2017;16:2598–2608. doi:10.1158/1535-7163.MCT-17-0386.
- Balar AV, Galsky MD, Rosenberg JE, Powles T, Petrylak DP, Bellmunt J, Loriot Y, Necchi A, Hoffman-Censits J, Perez-Gracia JL, et al. Atezolizumab as first-line treatment in cisplatin-ineligible patients with locally advanced and metastatic urothelial carcinoma: a single-arm, multicentre, phase 2 trial. Lancet (London, England). 2017;389:67–76. doi:10.1016/S0140-6736(16)32455-2.
- Chen L. Predicting tumor mutational burden (TMB) and tumor neoantigen burden (TNB) of east Asian ANSCLC patients by a targeted genomic profiling. J Thoracic Oncol. 2017;12:S2006. doi:10.1016/j.jtho.2016.09.002.
- Forde PM, Chaft JE, Smith KN, Anagnostou V, Cottrell TR, Hellmann MD, Zahurak M, Yang SC, Jones DR, Broderick S, et al. Neoadjuvant PD-1 blockade in resectable lung cancer. Cancer Res. 2018;78:CT079–CT.
- Hanna GJ, Lizotte P, Cavanaugh M, Kuo FC, Shivdasani P, Frieden A, Chau NG, Schoenfeld JD, Lorch JH, Uppaluri R, et al. Frameshift events predict anti-PD-1/L1 response in head and neck cancer. JCI Insight. 2018;3(4):e98811. doi:10.1172/jci.insight.98811.
- Hellmann MD, Nathanson T, Rizvi H, Creelan BC, Sanchez-Vega F, Ahuja A, Ni A, Novik JB, Mangarin LMB, Abu-Akeel M, et al. Genomic features of response to combination immunotherapy in patients with advanced non-small-cell lung cancer. Cancer Cell. 2018;33:843–52 e4. doi:10.1016/j.ccell.2018.03.018.
- Hellmann MD, Ciuleanu TE, Pluzanski A, Lee JS, Otterson G, Audigier-Valette C, Minenza E, Linardou H, Burgers S, Salman P, et al. Nivolumab (nivo) + ipilimumab (ipi) vs platinum-doublet chemotherapy (PT-DC) as first-line (1L) treatment (tx) for advanced non-small cell lung cancer (NSCLC): initial results from CheckMate 227. Cancer Res. 2018;78:CT077–CT.
- Horn L, Mansfield AS, Szczesna A, Havel L, Krzakowski M, Hochmair MJ, Huemer F, Losonczy G, Johnson ML, Nishio M, et al. First-line atezolizumab plus chemotherapy in extensive-stage small-cell lung cancer. N Engl J Med. 2018. doi:10.1056/NEJMoa1809064.
- Bonta L, Isac JF, Meiri E, Bonta D, Rich P. Correlation between tumor mutation burden and response to immunotherapy. J Clin Oncol. 2017;35(15_suppl). doi:10.1200/JCO.2017.35.15_suppl.e14579.
- Roszik J, Haydu LE, Hess KR, Oba J, Joon AY, Siroy AE, Karpinets TV, Stingo FC, Baladandayuthapani V, Tetzlaff MT, et al. Novel algorithmic approach predicts tumor mutation load and correlates with immunotherapy clinical outcomes using a defined gene mutation set. BMC Med. 2016;14:168. doi:10.1186/s12916-016-0705-4.
- Jiang T, Li X, Wang J, Su C, Han W, Zhao C, Wu F, Gao G, Li W, Chen X, et al. Mutational landscape of cfDNA identifies distinct molecular features associated with therapeutic response to first-line platinum-based doublet chemotherapy in patients with advanced NSCLC. Theranostics. 2017;7:4753–4762. doi:10.7150/thno.21687.
- Johnson DB, Frampton GM, Rioth MJ, Yusko E, Xu Y, Guo X, Ennis RC, Fabrizio D, Chalmers ZR, Greenbowe J, et al. Targeted next generation sequencing identifies markers of response to PD-1 blockade. Cancer Immunol Res. 2016;4:959–967. doi:10.1158/2326-6066.CIR-16-0143.
- Kazmi SM. A retrospective analysis of response to checkpoint inhibitors and its correlation to both PD-L1 score and tumor mutation burden in patients with solid tumor malignancies. Annals of Oncol. 2017;28:xi10. doi:10.1093/annonc/mdx075.
- Khagi Y, Goodman AM, Daniels GA, Patel SP, Sacco AG, Randall JM, Bazhenova LA, Kurzrock R. Hypermutated circulating tumor DNA: correlation with response to checkpoint inhibitor-based immunotherapy. Clin Cancer Res: off J Am Assoc Cancer Res. 2017;23:5729–5736. doi:10.1158/1078-0432.CCR-17-1439.
- Kowanetz M, Zou W, Shames D, Cummings C, Rizvi N, Spira A, Frampton G, Leveque V, Flynn S, Mocci S, et al. Tumor mutation burden (TMB) is associated with improved efficacy of atezolizumab in 1l and 2l+ NSCLC patients. J Thoracic Oncol. 2017;12:S321–S2. doi:10.1016/j.jtho.2016.11.343.
- Le DT, Uram JN, Wang H, Bartlett BR, Kemberling H, Eyring AD, Skora AD, Luber BS, Azad NS, Laheru D, et al. PD-1 blockade in tumors with mismatch-repair deficiency. N Engl J Med. 2015;372:2509–2520. doi:10.1056/NEJMoa1500596.
- Madore J, Strbenac D, Vilain R, Menzies AM, Yang JY, Thompson JF, Long GV, Mann GJ, Scolyer RA, Wilmott JS. PD-L1 negative status is associated with lower mutation burden, differential expression of immune-related genes, and worse survival in stage III melanoma. Clin Cancer Res: off J Am Assoc Cancer Res. 2016;22:3915–3923. doi:10.1158/1078-0432.CCR-15-1714.
- Miller A, Asmann Y, Cattaneo L, Braggio E, Keats J, Auclair D, Lonial S, Network MC, Russell SJ, Stewart AK. High somatic mutation and neoantigen burden are correlated with decreased progression-free survival in multiple myeloma. Blood Cancer J. 2017;7:e612. doi:10.1038/bcj.2017.94.
- Nathanson T, Ahuja A, Rubinsteyn A, Aksoy BA, Hellmann MD, Miao D, Van Allen E, Merghoub T, Wolchok JD, Snyder A, et al. Somatic mutations and neoepitope homology in melanomas treated with CTLA-4 blockade. Cancer Immunol Res. 2017;5:84–91. doi:10.1158/2326-6066.CIR-16-0019.
- Offin M, Rizvi H, Tenet M, Ni A, Sanchez-Vega F, Li BT, Drilon A, Kris MG, Rudin CM, Schultz N, et al. Tumor mutation burden and efficacy of EGFR-tyrosine kinase inhibitors in patients with EGFR-mutant lung cancers. C Cancer Res: off J Am Assoc Cancer Res. 2019;25(3):1063–1069. doi:10.1158/1078-0432.CCR-18-1102.
- Owada-Ozaki Y, Muto S, Takagi H, Inoue T, Watanabe Y, Fukuhara M, Yamaura T, Okabe N, Matsumura Y, Hasegawa T, et al. Prognostic impact of tumor mutation burden in patients with completely resected non-small cell lung cancer: brief report. J Thoracic Oncol: off Publ Int Assoc Study Lung Cancer. 2018;13:1217–1221. doi:10.1016/j.jtho.2018.04.003.
- Pai SG, Carneiro BA, Chae YK, Costa RL, Kalyan A, Shah HA, Helenowski I, Rademaker AW, Mahalingam D, Giles FJ. Correlation of tumor mutational burden and treatment outcomes in patients with colorectal cancer. J Gastrointestinal Oncol. 2017;8:858–866. doi:10.21037/jgo.2017.06.20.
- Peters S, Creelan B, Hellmann MD, Socinski MA, Reck M, Bhagavatheeswaran P, Chang H, Geese WJ, Paz-Ares L, Carbone DP. Impact of tumor mutation burden on the efficacy of first-line nivolumab in stage iv or recurrent non-small cell lung cancer: an exploratory analysis of CheckMate 026. Cancer Res. 2017;77:CT082–CT.
- Rizvi H, Sanchez-Vega F, La K, Chatila W, Jonsson P, Halpenny D, Plodkowski A, Long N, Sauter JL, Rekhtman N, et al. Molecular determinants of response to anti-programmed cell death (PD)-1 and anti-programmed death-ligand 1 (PD-L1) blockade in patients with non-small-cell lung cancer profiled with targeted next-generation sequencing. J Clin Oncol: off J Am Soc Clin Oncol. 2018;36:633–641. doi:10.1200/JCO.2017.75.3384.
- Rosenberg JE, Hoffman-Censits J, Powles T, van der Heijden MS, Balar AV, Necchi A, Dawson N, O’Donnell PH, Balmanoukian A, Loriot Y, et al. Atezolizumab in patients with locally advanced and metastatic urothelial carcinoma who have progressed following treatment with platinum-based chemotherapy: a single-arm, multicentre, phase 2 trial. Lancet (London, England). 2016;387:1909–1920. doi:10.1016/S0140-6736(16)00561-4.
- Shrestha R, Prithviraj P, Anaka M, Bridle KR, Crawford DHG, Dhungel B, Steel JC, Jayachandran A. Monitoring immune checkpoint regulators as predictive biomarkers in hepatocellular carcinoma. Frontiers in Oncol. 2018;8:269. doi:10.3389/fonc.2018.00269.
- Devarakonda S, Rotolo F, Tsao MS, Lanc I, Brambilla E, Masood A, Olaussen KA, Fulton R, Sakashita S, McLeer-Florin A, et al. Tumor mutation burden as a biomarker in resected non-small-cell lung cancer. J Clin Oncol: off J Am Soc Clin Oncol. 2018;36(30):2995–3006. doi:10.1200/JCO.2018.78.1963.
- Simpson D, FergusonR, Martinez CN, Kazlow E, Moran U, Heguy A, Hanniford D, Hernando E, Osman I, Kirchhoff T. Mutation burden as a potential prognostic marker of melanoma progression and survival. J Clin Oncol. 2017;35(15_suppl):9567–9567. doi:10.1200/JCO.2017.35.15_suppl.9567.
- Singal G, Miller PG, Agarwala V, Li G, Gossai A, Albacker LA, Goldberg ME, He J, Frank S, Bourque D, et al. Analyzing biomarkers of cancer immunotherapy (CIT) response using a real-world clinico-genomic database. Annals of Oncol. 2017;28:v404–v5. doi:10.1093/annonc/mdx376.005.
- Snyder A, Nathanson T, Funt SA, Ahuja A, Buros Novik J, Hellmann MD, Chang E, Aksoy BA, Al-Ahmadie H, Yusko E, et al. Contribution of systemic and somatic factors to clinical response and resistance to PD-L1 blockade in urothelial cancer: an exploratory multi-omic analysis. PLoS Med. 2017;14:e1002309. doi:10.1371/journal.pmed.1002230.
- Goodman AM, Piccioni D, Kato S, Boichard A, Wang HY, Frampton G, Lippman SM, Connelly C, Fabrizio D, Miller V, et al. Prevalence of PDL1 amplification and preliminary response to immune checkpoint blockade in solid tumors. JAMA Oncol. 2018;4:1237–1244. doi:10.1001/jamaoncol.2018.1701.
- Xiao D, Pan H, Li F, Wu K, Zhang X, He J. Analysis of ultra-deep targeted sequencing reveals mutation burden is associated with gender and clinical outcome in lung adenocarcinoma. Oncotarget. 2016;7:22857–22864. doi:10.18632/oncotarget.8213.
- Xu J, Guo X, Jing M, Sun T. Prediction of tumor mutation burden in breast cancer based on the expression of ER, PR, HER-2, and Ki-67. OncoTargets Ther. 2018;11:2269–2275. doi:10.2147/OTT.S159830.
- Hodges TR, Ott M, Xiu J, Gatalica Z, Swensen J, Zhou S, Huse JT, de Groot J, Li S, Overwijk WW, et al. Mutational burden, immune checkpoint expression, and mismatch repair in glioma: implications for immune checkpoint immunotherapy. Neuro-Oncology. 2017;19:1047–1057. doi:10.1093/neuonc/nox026.
- Postow MA, Sidlow R, Hellmann MD. Immune-related adverse events associated with immune checkpoint blockade. N Engl J Med. 2018;378:158–168. doi:10.1056/NEJMra1703481.
- Khunger M, Rakshit S, Schalper KA, Elson P, Pennell NA, Stevenson J, Velcheti V. Meta-analysis of tumor PD-L1 expression as a predictive biomarker of benefit from PD-1/PD-L1 axis inhibitors in solid tumors. J Clin Oncol. 2016;34:11603. doi:10.1200/JCO.2016.34.15_suppl.11603.
- Cao D, Xu H, Xu X, Guo T, Ge W. A reliable and feasible way to predict the benefits of Nivolumab in patients with non-small cell lung cancer: a pooled analysis of 14 retrospective studies. OncoImmunology. 2018;7(11):e1507262. doi:10.1080/2162402X.2018.1507262.
- Le DT, Durham JN, Smith KN, Wang H, Bartlett BR, Aulakh LK, Lu S, Kemberling H, Wilt C, Luber BS, et al. Mismatch repair deficiency predicts response of solid tumors to PD-1 blockade. Science (New York, NY). 2017;357:409–413. doi:10.1126/science.aan6733.
- Haratani K, Hayashi H, Chiba Y, Kudo K, Yonesaka K, Kato R, Kaneda H, Hasegawa Y, Tanaka K, Takeda M, et al. Association of immune-related adverse events with nivolumab efficacy in non–small-cell lung cancer. JAMA Oncol. 2018;4:374–378. doi:10.1001/jamaoncol.2017.2925.
- Higgins J, Green S. Cochrane handbook for systematic reviews of interventions Version 5.1. 0. The cochrane collaboration. Confidence Intervals. 2011. p. 93–429.
- Wells G, Shea B, O’connell D, Peterson J, Welch V, Losos M, Tugwell P. The Newcastle-Ottawa Scale (NOS) for assessing the quality of nonrandomised studies in meta-analyses. Accessed March 2016. Ottawa (ON): Ottawa Hospital Research Institute; 2009.
- Douxfils J, Haguet H, Mullier F, Chatelain C, Graux C, Dogné J. Association between bcr-abl tyrosine kinase inhibitors for chronic myeloid leukemia and cardiovascular events, major molecular response, and overall survival: A systematic review and meta-analysis. JAMA Oncol. 2016;2:625–632. doi:10.1001/jamaoncol.2015.5932.
- Parmar MK, Torri V, Stewart L. Extracting summary statistics to perform meta-analyses of the published literature for survival endpoints. Stat Med. 1998;17:2815–2834.
- Williamson PR, Smith CT, Hutton JL, Marson AG. Aggregate data meta-analysis with time-to-event outcomes. Stat Med. 2002;21:3337–3351. doi:10.1002/sim.1303.