Abstract
In the context of land use planning, Maryland has attracted significant attention at the national scale for innovative planning efforts. In 1997, Maryland passed a package of legislation collectively referred to as ‘smart growth’. Distinct from earlier regulatory state level attempts to alter development patterns in states like Oregon and Florida, this approach relied on incentives to influence development patterns. This innovative ‘inside/outside’ approach to managing growth relies on targeting state funding to encourage growth and investment in existing urbanized areas and areas planned for development (Priority Funding Areas [PFAs]) while discouraging growth and encouraging the preservation of rural areas (Rural Legacy Areas). Transportation funding for new projects or increased capacity must be directed into PFAs, constituting 85% of total funding on average from 1999 to 2008. Though the statute restricts the expenditure of state funds for transportation outside areas targeted for development, the statutes offer little explicit guidance regarding integrating transportation and land use policies. This article proposes that Smart Growth efforts could be even stronger if land-use policies were integrated more closely with transportation policies. The Maryland Statewide Transportation Model is applied to test the impact of various Smart Growth policies and transportation policies. The paper concludes that Smart Growth could have a bigger impact if transportation policies played a larger role.
1. Introduction
Maryland emerged as a leader in state land use policy early in the twentieth century, establishing the first statewide planning commission in the U.S. in 1933. Though the statewide planning commission was eventually dissolved, Maryland remained on the forefront of state level planning in subsequent decades. The state has a state-level Department of Planning and strong requirements for local government plans that are reviewed by the state. The declining health of the Chesapeake Bay, the largest brackish water estuary in the U.S. and an important ecosystem for the region, has been instrumental in pushing land use and environmental policy in Maryland since the 1980s (Knaap & Lewis, Citation2009). The state strengthened local planning statutes in 1992 prior to passing the package of legislation collectively known as Smart Growth in 1997.
State level legislation in Maryland transcends most U.S. states and the approach to smart growth in Maryland was innovative in using incentives rather than regulations to influence the location of development. By using the state’s spending for infrastructure as an incentive, the state sought to encourage growth in certain locally designated and state reviewed areas called Priority Funding Areas (PFAs), while discouraging growth outside of these areas. The intent of the program was not limiting or restricting development, but influencing the location of that development. Though the smart growth program in Maryland has received considerable acclaim, research shows that the legislation has not shown its intended effects on development patterns (Lewis, Knaap, & Sohn, Citation2009). While it may be too soon to tell whether smart growth has influenced the location of development in Maryland, in a state expected to add almost one million people, or 15%, by 2030,Footnote1 it is important to consider what policies might have an impact on reducing auto travel in the long term. In this paper, we use a statewide transportation model for Maryland to examine the impacts of transportation and land use policies in Maryland. We proceed as follows: In Section 2, we describe the smart growth program in Maryland and discuss how the legislative program in Maryland relates to the Smart Growth Network’s ten principles of Smart Growth. In Section 3, we describe the scenario analysis, and in Section 4, we conclude with the proposal that the Smart Growth program in Maryland could be further strengthened by integrating transportation policies.
2. Smart growth in Maryland
In 1992, the legislature passed the Economic Growth Resource Protection and Planning Act, requiring that local governments adopt comprehensive plans before adopting a zoning ordinance or subdivision ordinance. As a result of the 1992 Act, local governments must address eight elements and submit plans to the Maryland Department of Planning for review every six years. The Department of Planning reviews local plans for consistency with twelve (originally seven) visionsFootnote2 as defined by the 1992 Act. Although the 1992 Act strengthened requirements for local planning, it did not fundamentally alter land use governance; instead it offered a platform for broader land use reform five years later (Knaap & Frece, Citation2007; Knaap & Lewis, Citation2009).
Maryland emerged as a leader in statewide planning in 1997 with a package of legislation and budgetary initiatives collectively referred to as ‘smart growth’. Governor Parris N. Glendening introduced the notion of smart growth in 1996, supporting a commitment to a comprehensive package of legislation to strengthen the state’s capacity to alter growth, preservation, and revitalization decisions (Cohen, Citation2002). Underlying proposed legislation was the presumption that the state should not subsidize sprawl development and resources should be targeted into areas with existing infrastructure (Cohen, Citation2002). Rather than relying on regulations like stringent urban growth boundaries or state approved comprehensive plans, the state would rely on an incentive-based approach which uses the state’s purse to influence development, redevelopment and preservation decisions. The smart growth package of legislation included five programs, which address development, redevelopment and preservation (Lewis, Citation2011):
• | The Smart Growth Areas Act (or PFAs): State funding for new water and sewer infrastructure, new roads, and some housing, and economic development programs are limited to developed areas or areas planned for growth (PFAs). | ||||
• | Rural Legacy Program: State funding for preservation is targeted to Rural Legacy Areas, which are areas with high agricultural, forestry, natural and cultural value and threatened by development. | ||||
• | Brownfields Voluntary Cleanup and Revitalization Program: Provides financial incentives and technical assistance for redevelopment and clean up of abandoned or underutilized properties. | ||||
• | Job Creation Tax Credit Act: Provides tax credits to businesses that create over 25 jobs within PFAs. | ||||
• | Live Near Your Work Program: Provides incentives for employees buying homes in proximity to their workplace |
For the purposes of this article, we focus our discussion on the centerpiece of the 1997 smart growth package of legislation: The Smart Growth Areas Act. Under the Act, state spending for ‘growth-related’ programs is restricted to locally designated and state reviewed areas called PFAs. The state established specific criteria for constructing PFAs. Some areas were included automatically including existing municipalities, heavily developed areas inside the circumferential highways around Baltimore and the Maryland suburbs of Washington, DC, designated neighborhoods, enterprise zones, and heritage areas. Local governments were able to designate additional areas meeting certain criteria, based on existing and planned densities and infrastructure (Lewis, Citation2011). A map of PFAs is shown in Figure . As noted, ‘growth-related’ spending under certain state programs was restricted to PFAs. ‘Growth-related’ spending includes certain categories of spending for roads, housing programs, water and sewer infrastructure, state buildings, and certain economic development incentives, as defined by statute (Lewis, Citation2011). Spending from five agencies is included: transportation, housing and community development, environment, and business and economic development. Because we examine transportation and land use scenarios in Section 3, it is imperative to further discuss how the Smart Growth Areas Act addresses transportation spending. Generally, the law requires that all major projects in the construction program occur within PFAs. However, certain projects are specifically exempt from the law, including projects administered by the Maryland Transportation Authority, which administers all toll facilities. Further, PFA status does not need to be considered for system preservation, minor projects, and projects in the development and evaluation phase. Additionally, certain exemptions can be granted by the Board of Public Works when a project connects PFAs, maintains the current transportation system without increasing capacity, has the purpose of giving the Maryland Department of Transportation control or access along an existing corridor, or operational characteristics require that the project be located outside of a PFA (Lewis, Citation2011). Thus, the Smart Growth Areas Act is most applicable to new roads or expansions in capacity. By preventing state investment in new roads, the state hopes to prevent development from occurring outside PFAs. As Lewis et al. (Citation2009) reported, nearly 85% of ‘growth-related’ funds from 1999 to 2008 were transportation related.
Figure 1. Priority Funding Areas in Maryland. Data source: Maryland Department of Planning; U.S. Census (Source: Authors).
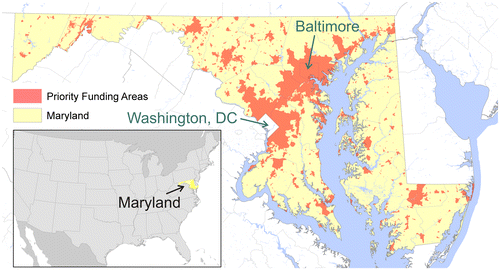
In the United States, there have been ‘three waves’ of growth management since the 1960s. The first wave relied on growth controls and was primarily related to environmental concerns and include regulatory approaches like Oregon. The second wave related to efficient provision of infrastructure, and includes states like Florida. The third wave included ‘smart growth’ and incentive-based approaches to growth management and includes states like Maryland. (Chapin, Citation2012). Thus, the Maryland approach to statewide planning is unique from most states in relying on an incentive based approach, which lies between the conventional American approach of planning at the local level and the less common regulatory statewide approach found in Oregon, Florida and other states. Other states followed suit, including Delaware, Georgia, New Jersey, New York, Pennsylvania and Wisconsin (American Planning Association [APA], Citation2002). There has been little research on the effectiveness of other smart growth programs beyond Maryland.
Though Maryland received widespread acclaim and won several awards for these innovative policies, retrospective evaluative research on the Maryland program has shown that the Smart Growth in Maryland has fallen short of its expectations (Avin et al., Citation2014; Hanlon, Howland, & McGuire, Citation2010; Lewis & Knaap, Citation2012; Lewis et al., Citation2009; Sohn & Knaap, Citation2005). Lewis et al., Citation2009 found that PFAs had little discernable impact on development patterns after the Act went into effect. However, Hanlon, Howland, and McGuire (Citation2010), found that parcels inside PFAs were more likely to develop than parcels outside PFAs. Towe, Lewis, and Lynch (Citation2014) used propensity score matching to show that the incentive based PFA policy shifted development away from areas outside PFAs with similar characteristics as areas inside PFAs. A 2011 report by the National Center for Smart Growth Research and Education at the University of Maryland studied a broad array of indicators including population, economy, housing, and natural resources outside of state programs. The report concluded: ‘The evidence assembled in this report did not find a compelling level of change in the variables chosen to represent the goals of Maryland’s Smart Growth Program’. Further, ‘If the indicators here are leaning in any direction, it is that Maryland has not made substantial progress toward improving its performance in many of the areas it says it cares about’ (National Center for Smart Growth Research and Education, Citation2011).
In summary, the general consensus of previous work on Maryland’s Smart Growth program has shown little discernable impact on development patterns. However, much of the previous work has been retrospective and looked at the impact of PFAs on development patterns after PFAs went into effect. Historically, the timing and nature of the development process means that land use policies take time to show impacts and PFAs are no exception. In this paper, we use scenarios and modeling to examine long-term future impacts.
The Maryland approach to smart growth relies on using the state’s budget to influence development, revitalization and redevelopment at the local level. It is important to note that Maryland’s state level smart growth program is distinguished from the ten principles of smart growth offered by advocacy organizations like the Smart Growth Network. Those ten principles of smart growth address mixed use, compact development, housing opportunities, walkability, sense of place, preservation, directing development to existing communities, providing transportation options, making development decisions predictable, and encouraging public participation.Footnote3 The ten principles of smart growth are reported in Table . Though it is possible that directing state funding for infrastructure into specific areas may achieve many of these objectives, the statutes do not directly address the form of development or consider many of these details. Further, smart growth in Maryland explicitly avoids limiting the amount of growth, but instead addresses the location of future development. Maryland’s approach relies on targeting state spending in order to incentivize development in some areas (PFAs) while discouraging development in other areas (Rural Legacy Areas).
Table 1. Ten principles of smart growth – Smart Growth Network.
3. Modeling the impact of smart growth strategies
Smart Growth in Maryland is a rather young concept, with the most important policies introduced in the late1990’s and early 2000’s. Given that that most urban landscape today has been developed before Smart Growth was introduced some 20 years ago, it cannot be expected that this new planning paradigm has substantially changed urban form, land cover or transportation demand (Knaap, Citation2005).
Understanding the impact of Smart Growth strategies helps to reach planning goals, to avoid unintended side effects, and to allocate infrastructure investments most efficiently. Rather than waiting a few more decades and hoping that Smart Growth strategies fulfill their promises, mathematical models can be used to assess the impact of various planning approaches. Thus, this section deviates from a traditional empirical approach, because Smart Growth has only been in place for a limited number of years. This approach allows projecting impacts for a longer time period into the future. First, a base scenario is modeled which assumes that growth does not follow the Smart Growth paradigm but rather continues suburban growth with little emphasis on density and protection of open land. Then, several scenarios are implemented that test selected Smart Growth policies, and in particular PFAs, on land consumption and travel behavior. Analyzing the difference between the base scenario and various policy scenarios allows assessing the likely impact of the Smart Growth planning paradigm.
The Maryland Statewide Transportation Model (MSTM) is used to analyze these scenarios. Since 2006, the Maryland State Highway Administration (MD SHA) and the National Center for Smart Growth Education and Research at the University of Maryland have developed a model that represents travel behavior in a two-layer approach: A national model covers long-distance travel of both autos and trucks, and a statewide layer adds local traffic within Maryland and a buffer ring around the state (Figure ). The statewide model follows the traditional four-step model design (trip generation, destination choice, mode choice and assignment). A nationwide model adds long-distance auto trips using the National Estimate of Long-Distance Travel (NELDT) (Moeckel & Donnelly, Citation2011) and long-distance truck trips using a national freight model (Mishra, Iseki, & Moeckel, Citation2014; Moeckel & Donnelly, Citation2016). The model has been calibrated using local household travel and on-board surveys and validated against traffic counts and estimates of vehicle-miles traveled (VMT). The model base year is 2007 and the future year is 2030. The model base year was constrained by data inputs. This timespan of modeling is common in transportation and urban planning, as observing change beyond 20–30 years is generally seen as more unpredictable. The model was reviewed by an independent Peer Review Panel of the U.S. Federal Highway Association and accepted as representing observed travel behavior reasonably well. The model has been documented in detail by SHA (Citation2013).
The MSTM includes non-motorized travel in trip generation but removes non-motorized trips before destination choice. Non-motorized trips are estimated based on population and employment densities but unaffected by transport policies. While it would be desirable to test the impact of smart growth policies on non-motorized travel, the spatial resolution of the model does not allow accounting explicitly for walking and biking. On the flip side, the coarser resolution allows covering a larger study area, which was necessary to capture statewide smart growth policies in Maryland.
Several Smart Growth-related scenarios were implemented and tested with the MSTM. They relate to land-use policies and transportation policies shown in Table .
Table 2. Implemented scenarios to analyze the effects of Smart Growth policies.
3.1. Business as Usual
The Business as Usual (BUA) scenario models the most likely future if no additional policies are introduced. The population and employment forecasts are based on the National Capital Region’s Financially Constrained Long-Range Transportation Plan (CLRP), a forecast that is negotiated among various local planning agencies and generally accepted as a plausible future distribution of population and employment. This forecasts includes development outside of PFAs.
3.2. Priority Funding Areas
In 1997, the state of Maryland defined PFA, directing State funding to these designated areas for development (see Section 2). Most PFAs were not drawn until around 2000 (Lewis et al., Citation2009). Figure shows PFA are primarily located in and near denser urban centers. While this policy does not restrict development outside of PFA, the program is meant to encourage development particularly in these areas. This scenario assumes that PFA are extraordinarily successful and that all development happens within PFA exclusively.Footnote4 While in reality there is some development happening outside of PFA today, this scenario explores the potential upper limit of the impact of this policy.
3.3. Transit-Oriented Development
Transit-Oriented Development (TOD) is a planning concept that focuses urban development near transit stations, with the intent of encouraging residents to use transit once they live near rail stations (Dittmar, Belzer, & Autler, Citation2004). Likewise, allocating businesses to transit-accessible locations shall support transit ridership for work and shopping trips. This TOD scenario allocates the forecasted growth to zones with rail access only (see note 4). Figure identifies zones within 1 mile (or 1.6 km) of rail stations (including commuter rail, light rail and subway stations). New development outside these rail corridors is not permitted in this scenario.
3.4. AOC25
Auto-operating costs (AOC) include costs for the purchase of a vehicle, insurance, maintenance, and gasoline. The base scenario sets AOC to 9.9 cents/mile. To represent a rise in fuel taxes, this scenario increases AOC by 25% to 12.375 cents/mile (in constant dollars).
3.5. AOC50
This scenario increases AOC by 50% to 14.85 cents/mile.
3.6. 2xPARK
Parking costs in the MSTM are estimated as a function of density, with dense urban areas having the highest parking costs and regions below an activity density of 14 units/acreFootnote5 offer free parking. In this scenario, all parking costs have been doubled.
The PFA and TOD scenarios change the population and employment distribution. While the total growth is given by the CLRP baseline forecast, these scenarios reallocate growth to selected zones. This change may be quantified by comparing the Gini Coefficients (Gini, Citation1909/1997), which measures the distribution of values. A Gini coefficient of 0 means that all zones have the same densities, and a Gini coefficient of 1 would result if all households or employment were located in one single zone. Figure shows the Lorenz curves of household distributions in three scenarios in 2030, showing more concentration of households in the PFA and TOD scenarios. The right side of Figure shows that employment has a higher Gini coefficient than population across all scenarios, displaying that employment is more concentrated than population (even in the base scenario). The Gini coefficient for population increases for both the PFA and the TOD scenario by approximately 8%. This is not a dramatic shift, but it should be kept in mind that only growth was reallocated to PFA and TOD areas. Residential areas existing in the base year were not affected. The Gini coefficients for employment remain almost unchanged, indicating that employment is very concentrated already and not significantly affected by land use restrictions.
Figure 4. Lorenz curve of household distributions (left) and Gini coefficients of households and employment distributions (right) in 2030.
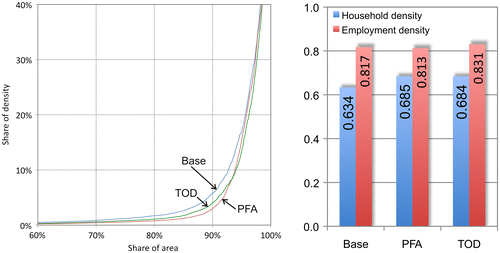
It should be noted that the density distribution shown in Figure is based on exogenous assumptions of alternative development patterns. In reality, it is possible that some households or employers would leave the study area and move to other regions that allow more traditional suburban development.
In addition to evaluating concentration of population and employment, the MSTM allows analyzing these scenarios in their impact on transportation. Average trip length, transit share and VMT are used to evaluate the success of these smart growth strategies. While Smart Growth aims at more than just reducing auto travel, these performance measures are helpful indicators to assess the success of Smart Growth strategies.
Figure shows the average trip length across all scenarios in 2030. To emphasize the relatively small difference between scenarios, the scale of the y-axis starts at 8 miles. All scenarios led to shorter trip lengths than the base scenario. The PFAs and doubling the auto-operating costs (AOC50) are the most influential scenarios. The 2xPARK scenario affects the average trip length the least.
Figure compares the share of transit trips across all scenarios. The lower end of the y-axis is set to five to emphasize differences between scenarios. In this respect, the TOD scenario is most successful. Allocating growth in zones within 1 mile of rail stations increases transit ridership by 1%. While the impact may appear rather small, it should be noted that many residential areas without rail access are in existence in the base year already and are not affected by this policy. The PFA scenario has little impact on transit ridership, as PFA cover large areas that are not transit accessible. Increasing AOC or parking costs increases transit ridership by a small degree as well.
Finally, Figure compares VMT across all scenarios. Interestingly, VMT increases for both the PFA and TOD scenarios. As counter-intuitive as this may sound, there is an easy explanation. Further analysis showed that VMT increases in the densest parts of the study area, while more rural parts reduce VMT. Figure shows most growth in the vicinity of Washington, DC and Baltimore. In both the PFA and the TOD scenario, population and employment growth is reallocated to very dense neighborhoods that are already seriously congested in the base scenario. With this additional growth, travel times in these areas become very long, and those who are able to circumvent this congestion are willing to drive fairly large distances in the network assignment step. As a consequence, the PFA and TOD scenarios lead to higher VMT overall, even though they give relief to some rural areas. The shorter trip length shown in Figure represented the ‘theoretical’ trip length, i.e. people could have traveled shorter distances if there was no congestion. But given the high level of congestion in the Washington and Baltimore area with traffic volumes that often exceed the capacity, autos driving larger detours generate an increase in VMT in the PFA and TOD scenarios.
Figure 8. Difference in Vehicle-Miles Traveled (VMT) per Acre between the BAU and the PFA scenario (Source: Authors).
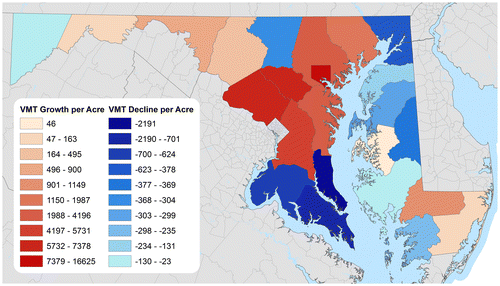
This behavior is confirmed with a similar increase in vehicle-hours traveled in urban areas, where auto travelers spent more time on the road due to lower average speeds trying to avoid the highly congested portions of the study area. The TOD scenario generates less VMT than the PFA scenario, which is due to the modeled higher transit share of the TOD scenario. Scenarios that increase the costs for transportation, on the other hand, consistently generate lower VMT.
The analysis of scenarios shows that evaluating such policies is not a one-dimensional exercise but requires comparing various indicator variables. While the PFA and TOD scenarios increase densities as anticipated by the State of Maryland, the impact on auto travel turns out to be the opposite of what Smart Growth intends.
Note that the TOD scenario assumed that the number and location of rail stations does not change. One could imagine a scenario in which massive investments in transit would increase ridership and mitigate highway congestion. Other scenario analyses (not presented here) of the Red Line in Baltimore, the Purple Line in Montgomery and Prince Georges County in MD, and the Silver Line to Dulles International Airport in Virginia showed noteworthy but limited impact on transit ridership. Cervero and Guerra (Citation2011) concluded that at least 30 people per acre need to live around transit stations to make light rail feasible. In this study area, this threshold applies to only 4% of the population. In other words, the current urban fabric is not well-suited for massive light rail expansions. Only a combination of transit expansions with TOD development has the potential to shift mode shares towards transit.
In addition to individual scenarios, integrated land-use/transport scenarios were tested. Specifically, the combinations TOD + AOC50, PFA + AOC50 and 2xPark + AOC50 were analyzed. For most parts, the effects of the combined scenarios were the simple addition of the effects of individual scenarios. For example, the TOD scenario increased the transit share by 1.0% points, and the AOC50 scenario increased the transit share by 0.3% points. TOD + AOC50 led to an increase in transit ridership by 1.3% points. While the model results do not suggest hidden benefits from combining land use with transport scenarios, combining land use policies with transport policies may increase the overall effect.
It is worth noting that the model setup simplifies the land use transport feedback cycle as described by Wegener and Fürst (Citation1999) and Conder and Lawton (Citation2002). For example, increases in congestion as shown in the PFA scenario would in reality likely lead to household relocation with some households attempting to move to housing locations that reduce their need for travel. Such feedback may be represented with integrated land use/transport models (Hunt, Kriger, & Miller, Citation2005; Timmermans, Citation2007; Wegener, Citation2014). While such modeling system is under development for the state of Maryland (Dawkins & Moeckel, Citation2016), this study focuses on the direct effects and does not attempt to reveal subtle second order effects.
4. Future direction of smart growth in Maryland
The Smart Growth efforts in Maryland are significant and more ambitious than in most other states across the U.S. It is remarkable that Maryland has continued this strategy even during an economic downturn from 2008 to 2012, when many states, such as Florida, retreated to less state level input and more developer-friendly and economic growth-centered policies. However, the implementation of Smart Growth in Maryland has fallen short of its expectations to date.
The implementation of PFA legislation ensures that public investments are focused on areas that are most suitable for future growth. This is desirable, but the concept falls short of restricting development outside the PFA.
The TOD scenario has a noteworthy impact on transit ridership, but it might be unrealistic to assume that all future growth happens in dense neighborhoods around rail stations. Too many households continue to prefer suburban development. If Maryland implemented this policy alone, some households may move to neighboring states that allow suburban development. Only a coordinated implementation of this plan across the entire Washington Megaregion could lead to successful TOD implementations. As such cooperation is unlikely at this point, TOD probably remains a planning paradigm that is popular among planners but implemented in a very few locations only with limited impact on overall travel demand.
Pricing auto travel was most successful in reducing VMT, and thereby, curbing Greenhouse Gas Emissions. In 2013, Maryland increased the tax on gasoline from 23.5 to 27 cents per gallon (≈5.2 Euro cent per liter, in addition to a federal gasoline tax of 18.4 cents per gallon or approximately 3.5 Euro cents per liter). According to model results, pricing of the private automobile effectively reduces VMT and in addition provides funding for needed infrastructure investments, though it is readily admitted that such tax increases are politically challenging at best, and maybe unattainable in the foreseeable future.
Most importantly, the PFA and TOD scenarios did not reduce congestion by the degree often claimed. Instead, adding new development to the most congested parts of the region is expected to increase congestion as severely that resulting detours generate more VMT than the base scenario. From a transportation point of view, the model results do not favor PFA or TOD policies.
Nevertheless, the Smart Growth planning paradigm remains an attractive planning vision for a number of reasons that are not as easy to measure as trip length or transit share. The model is unable to represent many aspects of urban development, though they are equally important when evaluating planning policies:
• | Smart Growth may help reduce auto dependency (Handy, Citation2005), which may reduce Greenhouse Gas Emissions in the long run. Many economists agree that fuel prices are likely to rise in the future due to a limited supply, and a denser and more walkable environment may help mitigating the effects of higher transportation costs. | ||||
• | Protecting open land may increase bio-diversity, provide space for outdoor activities, support clean air, and help sequestrating CO2 emission. | ||||
• | Improving walkability may not have an immediate impact on VMT, but even moderate walking has important health benefits (Mueller et al., Citation2015). An aging population that is less able to drive further benefits from improved walkability. | ||||
• | The urban design of Smart Growth development projects is popular at least for selected portions of the population. Smart Growth also improves accessibility to people and jobs, which creates economic opportunities, social interaction and potentially shorter travel. | ||||
• | Smart Growth is a long-term vision rather than a quick fix. Given that most parts of the city in 2030 are built already today, and thereby, are not affected by Smart Growth, true impacts may only be seen in the long run. |
Disclosure statement
No potential conflict of interest was reported by the authors.
Acknowledgement
This work was supported by the German Research Foundation (DFG) and the Technical University of Munich within the funding programme Open Access Publishing.
Notes
1. Estimates as of October 2009 from Maryland State Data Center, Department of Planning.
2. The seven visions were replaced by twelve new visions crafted by the Task Force on the Future of Growth and Development in 2009 (Maryland Department of Planning, Citation2008).
3. https://www.epa.gov/smartgrowth/about-smart-growth, accessed on 6 October 2016.
4. The region’s total growth was allocated proportionally to the forecasted growth of zones with PFA/TOD, ensuring that reasonable densities were not exceeded. Zones outside of PFA/TOD received no growth.
5. Activity density is calculated as [(Households + Retail_Employment + Total_Employment)/Area]. Retail employment enters this calculation twice (as it is also included in total employment) to emphasize higher parking costs in shopping district.
References
- American Planning Association. (2002). Planning for smart growth: 2002 state of the states. Chicago, IL: Author.
- Avin, U., Welch, T. F., Knaap, G., Ducca, F., Mishra, S., Cui, Y., & Erdogan, S. (2014). Even smarter growth? Land use, transportation, and greenhouse gas in Maryland. Proceedings of the 93rd Annual Meeting of the Transportation Research Board, Washington, DC.
- Cervero, R., & Guerra, E. (2011). Urban densities and transit: A multi-dimensional perspective. Berkeley, CA. Retrieved from http://www.its.berkeley.edu/sites/default/files/publications/UCB/2011/VWP/UCB-ITS-VWP-2011-6.pdf
- Chapin, T. (2012). From growth controls, to comprehensive planning, to smart growth: Planning’s emerging fourth wave. Journal of the American Planning Association, 78, 5–15.10.1080/01944363.2011.645273
- Cohen, J. R. (2002). Maryland’s “smart growth”: Using incentives to combat sprawl. In G. D. Squires (Ed.), Urban sprawl: Causes, consequences, and policy responses. 293–324. Washington, DC: Urban Institute.
- Conder, S., & Lawton, K. (2002). Alternative futures for integrated transportation and land-use models contrasted with Trend-Delphi models. Transportation Research Record: Journal of the Transportation Research Board, 1805, 99–107.10.3141/1805-12
- Dawkins, C., & Moeckel, R. (2016). Transit-induced gentrification: Who will stay, and who will go? Housing Policy Debate, 26, 801–818. doi:10.1080/10511482.2016.1138986
- Dittmar, H., Belzer, D., & Autler, G. (2004). An introduction towards transit-oriented development. In H. Dittmar & G. Ohland (Eds.), The New Transit town: Best practices in transit-oriented development. 1–18. Washington, DC: Island Press.
- Gini, C. (1909/1997). Concentration and dependency ratios. Rivista di Politica Economica, 87, 769–792. Translation of Gini’s article Variabilità e mutabilità from 1909.
- Handy, S. (2005). Smart growth and the transportation-land use connection: What does the research tell us? International Regional Science Review, 28, 146–167.10.1177/0160017604273626
- Hanlon, B., Howland, M., & McGuire, M. (2010). Hotspots for growth: Land use change in a transitional county in the US. University of Maryland. Unpublished manuscript.
- Hunt, J. D., Kriger, D. S., & Miller, E. J. (2005). Current operational urban land-use-transport modelling frameworks: A review. Transport Reviews, 25, 329–376.10.1080/0144164052000336470
- Knaap, G. J., & Frece, J. (2007). Smart growth in Maryland: Looking forward and looking back. Idaho Law Review, 43 (2), 445–473.
- Knaap, G.-J. (2005). A requiem for smart growth? In D. R. Mandelker (Ed.), Planning reform in the new century. 103–128. Chicago, IL: American Planning Association.
- Knaap, G. J., & Lewis, R. (2007). State agency spending under Maryland’s Smart Growth Areas Act: who’s tracking, who’s spending, how much, and where? Report released by the national center for smart growth research and education, College Park, MD.
- Knaap, G. J. & Lewis, R. (2009). Maryland case study. In G. K. Ingram, A. Carbonell, Y-H. Hong, & A. Flint (Eds.), Smart growth policies: an evaluation of programs and outcomes. Cambridge, MA: Lincoln Institute for Land Policy.
- Lewis, R. (2011). Do smart growth instruments in Maryland make a difference? ( PhD dissertation). University of Maryland, College Park, MD.
- Lewis, R., & Knaap, G.-J. (2012). Targeting spending for land conservation: An evaluation of Maryland’s rural legacy program. Journal of the American Planning Association, 78, 34–52.10.1080/01944363.2011.645275
- Lewis, R., Knaap, G.-J., & Sohn, J. (2009). Managing growth with priority funding areas: A good idea whose time has yet to come. Journal of the American Planning Association, 75, 457–478.
- Maryland Department of Planning. (2008). Where do we grow from here? A report of the task force on the future for growth and development in Maryland. Baltimore, MD: Maryland Department of Planning.
- Mishra, S., Iseki, H., & Moeckel, R. (2014). Multi entity perspective freight demand modeling technique: Varying objectives and outcomes. Transport Policy, 35, 176–185.
- Moeckel, R., & Donnelly, R. (2011). Nationwide estimate of long-distance travel (NELDT). Generating external trips for local travel demand models. Conference Proceedings of the Annual Meeting of the Transportation Research Forum. Long Beach, CA.
- Moeckel, R., & Donnelly, R. (2016). A model for national freight flows, distribution centers, empty trucks and urban truck movements. Transportation Planning and Technology, 39, 693–711.10.1080/03081060.2016.1204091
- Mueller, N., Rojas-Rueda, D., Cole-Hunter, T., de Nazelle, A., Dons, E., Gerike, R., & Nieuwenhuijsen, M. (2015). Health impact assessment of active transportation: A systematic review. Preventive Medicine, 76, 103–114.10.1016/j.ypmed.2015.04.010
- National Center for Smart Growth. (2011). Indicators of smart growth in Maryland. College Park, MD: National Center for Smart Growth. Retrieved from http://smartgrowth.umd.edu/assets/documents/indicators/2011_smart_growth_indicators_report.pdf
- SHA. (2013). The Maryland statewide transportation model. Model Documentation (version 1.0). Baltimore, MD. 187 p.
- Sohn, J., & Knaap, G. J. (2005). Does job creation tax credit program in Maryland help concentrate employment growth? Economic Development Quarterly, 19, 313–326.10.1177/0891242405278183
- Timmermans, H. (2007). The saga of integrated land use-transport modeling: How many more dreams before we wake up? In K. W. Axhausen (Ed.), Moving through nets: The physical and social dimensions of travel (pp. 219–248). Amsterdam: Elsevier.
- Towe, C., Lewis, R., & Lynch, L. (2014). Using quasi-experimental methods to evaluate land policies: Application to Maryland’s priority funding legislation. In J. Duke & J. Wu (Eds.), The Oxford handbook of land economics. 452–480. Oxford: Oxford University Press.
- Wegener, M. (2014). Land-use transport interaction models. In M. Fischer & P. Nijkamp (Eds.), Handbook of regional science (pp. 741–758). Berlin, Heidelberg: Springer.10.1007/978-3-642-23430-9
- Wegener, M., & Fürst, F. (1999). Land-use transport interaction: State of the art ( Working Paper 46). University of Dortmund. Retrieved from https://eldorado.tu-dortmund.de/bitstream/2003/26574/1/46.pdf