Abstract
Objective: The ALSFRS-R is limited by multidimensionality, which originates from the summation of various subscales. This prevents a direct comparison between patients with identical total scores. We aim to evaluate how multidimensionality affects the performance of the ALSFRS-R in clinical trials. Methods: We simulated clinical trial data with different treatment effects for the ALSFRS-R total score and its subscales (i.e. bulbar, fine motor, gross motor and respiratory). We considered scenarios where treatment reduced the rate of ALSFRS-R subscale decline either uniformly (i.e. all subscales respond identically to treatment) or non-uniformly (i.e. subscales respond differently to treatment). Two main analytical strategies were compared: (1) analyzing only the total score or (2) utilizing a subscale-based test (i.e. alternative strategy). For each analytical strategy, we calculated the empirical power and required sample size. Results: Both strategies are valid when there is no treatment benefit and provide adequate control of type 1 error. If all subscales respond identically to treatment, using the total score is the most powerful approach. As the differences in treatment responses between subscales increase, the more the total score becomes affected. For example, to detect a 40% reduction in the bulbar rate of decline with 80% power, the total score requires 1380 patients, whereas this is 336 when using the alternative strategy. Conclusions: Ignoring the multidimensional structure of the ALSFRS-R total score could have negative consequences for ALS clinical trials. We propose determining treatment benefit on a subscale level, prior to stating whether a treatment is generally effective.
Introduction
Regulatory approval of new drugs for ALS requires conclusive evidence of an improvement in life expectancy or a slowing in progression rate (Citation1,Citation2). In general, there are two options as primary outcome for pivotal ALS clinical trials: (1) endpoints based on survival time or (2) the revised ALS functional rating scale (ALSFRS-R). Although each of these endpoints has its own strengths and weaknesses (Citation3–5), 82% of the pivotal trials currently use the ALSFRS-R total score () (Citation6).
Table 1 Overview of currently active and planned pivotal, randomized, placebo-controlled clinical trials on ClinicalTrials.gov.
For an individual patient, the ALSFRS-R total score is an accurate reflection of disease progression, where a drop in total score indicates continued deterioration (Citation5,Citation7,Citation8). The attractiveness of the total score is its simplicity, consistent change over time and ability to assess a patient’s functional status remotely (Citation9,Citation10). Moreover, the ALSFRS-R can easily be translated to clinical disease stage (Citation11,Citation12), providing investigators with the ability to evaluate when treatments may be most effective (Citation13). Critical issues arise, however, when comparing patients, as two patients with identical ALSFRS-R total scores may not be comparable as far as disease stage or prognosis is concerned (Citation14–16). This issue, often referred to as multidimensionality (Citation14,Citation15), originates from the summation of various subscales (i.e. bulbar, motor and respiratory functioning).
Despite this well-known issue, the ALSFRS-R total score continues to be recommended as a key efficacy endpoint within current clinical trial guidelines (Citation1,Citation2,Citation17). This is not surprising given the absence of clarity regarding consequences of multidimensionality for clinical trials and the lack of suitable alternatives. A multidimensional outcome is essentially a composite endpoint and, like other composite endpoints (Citation3), treatment effects may become diluted (Citation18). This is especially true when outcomes (or subscales) do not respond uniformly to treatment.
To illustrate, at the design stage of the Nuedexta trial, existing evidence suggested enhanced bulbar functioning (Citation19). At the end of the trial, the investigators indeed concluded bulbar benefit, a conclusion that would have remained even if the ALSFRS-R bulbar subscale had been defined as primary endpoint (p = 0.003). However, had the investigators used the ALSFRS-R total score, the trial conclusion would have been futile (p = 0.25). In this example, it is obvious that only an outcome that actually measures the targeted domain should be used, as adding irrelevant endpoints or, in this case, the motor and respiratory subscales, dilutes the treatment effect.
In most clinical trials, however, it is not known a priori which subscales will benefit from treatment, or whether all subscales will benefit equally. In these settings, it remains unclear how multidimensionality of the ALSFRS-R total score, or any other multidimensional endpoint, may affect trial conclusions or how best to manage treatment uncertainty at the design stage. In this study, therefore, we assess how the ALSFRS-R total score performs in clinical trials under a variety of treatment efficacy scenarios, illustrate the pitfalls and propose a simple alternative strategy to improve its use in future studies.
Methods
Simulation study
The effect of ALSFRS-R multidimensionality on clinical trial results was assessed in a simulation study. We used the PRO-ACT database (version Dec. 2015) as real-world input for our simulations (Citation20). All patients provided written informed consent for the collection and use of their data, with each individual trial being approved by an institutional review board. All data are anonymized and identifying information has been removed so that individual studies within PRO-ACT are not traceable. We excluded individuals from whom there was no information on the ALSFRS-R total score or its subscales. Our primary aim was to simulate 12-month longitudinal patterns. We, therefore, removed all ALSFRS-R information collected after 13.5 months (allowing for a 6-week collection window). Four subscales were defined: (1) bulbar; items 1–3, (2) fine motor; items 4–6, (3) gross motor; items 7–9 and (4) respiratory functioning; items 10–12 (Citation7,Citation21).
Linear mixed effects models were used to model the longitudinal patterns over time on a subscale level, where the model included a fixed monthly rate of decline, and a random intercept and slope per individual. A shared random-effects structure was modeled per individual to account for the dependencies and correlations between subscales; the subscale correlation matrix is provided in . The final (multivariate) model was used to simulate longitudinal subscale data at monthly intervals (± 5 days, i.e. SD 0.08) over a period of 12 months. We added subscale-specific treatment effects, defined as a % reduction in rate of decline, in order to simulate different scenarios. As the total score is simply the sum of the subscales, the treatment response on the total score is defined as the sum of the subscale responses (Supplementary methods).
Figure 1 Correlation matrix of the baseline scores and longitudinal rates of decline within and between subscales. To illustrate, the rate of decline in fine motor functioning is strongly correlated to the rate of decline in gross motor functioning (Pearson’s r 0.79). Abbreviations: base = baseline value at study enrollment; slope = rate of decline during follow-up.
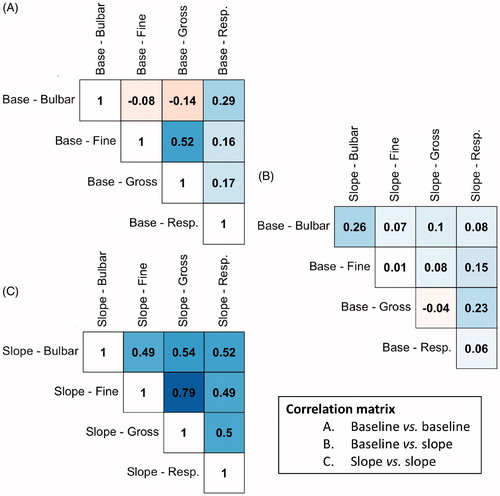
Classical and alternative trial analysis of the ALSFRS-R
For each scenario, we considered three analytical strategies, which are discussed below. We assumed that (a priori) it is not known how treatment will affect the individual subscales. All analytical strategies have the common objective of identifying a treatment effect that slows the progression rate (whether on the total score, or on any of the subscales). Standard practice in clinical trials is to determine whether the linear rate of disease progression (i.e. slope) is significantly reduced compared to a control group (e.g. placebo) (Citation17). A treatment is considered effective if the p-value for the slope difference falls below a significance threshold (e.g. p < 0.05) or, equivalently, when the confidence interval (e.g. 95%) around the difference excludes zero (i.e. no difference in slopes). This decision process is illustrated in for a hypothetical drug with an ineffective trial result.
Figure 2 Illustration of classical and alternative analytical strategies of the ALSFRS-R of the same hypothetical trial; (A) classical analysis of the mean difference in slopes between the active and placebo arm. A treatment is considered effective if p < 0.05. (B) Alternative analysis where the subscales are tested individually against an adjusted significance threshold. A treatment is considered effective if any subscale is below the adjusted significance threshold. (C) Similar to B, but with a non-inferiority boundary -Δ. A treatment is considered effective if (1) any subscale is below the adjusted one-sided significance threshold and (2) none of the lower confidence bounds cross the non-inferiority boundary.
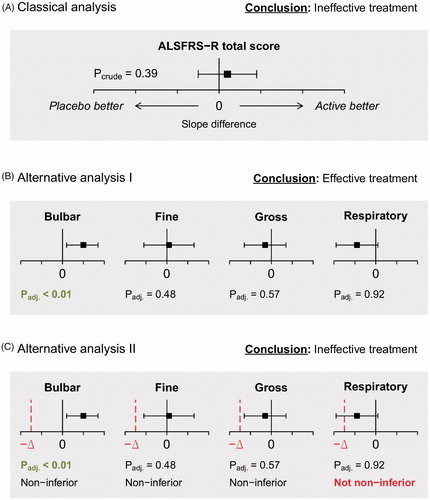
In order to address the multidimensional structure of the ALSFRS-R, a simple alternative strategy might be to evaluate the slope difference in each subscale individually rather than the difference in total score. In this framework, a treatment is considered effective if at least one of the subscales yields a statistically significant difference. This strategy requires four hypotheses tests and p-values need to be adjusted to control type I error (i.e. false-positives) (Citation22). In , employing such a strategy would consider the same trial as in as being effective due to the significant bulbar effect. Note that for this strategy there is no particular interest in any individual subscale; as long as at least one subscale yields a statistically significant difference, the treatment is considered effective.
In , however, the respiratory treatment response is slightly negative. Whether such a treatment would still be considered effective, despite the positive effect on bulbar function, depends largely on the extent of the effect. A small negative effect may still be acceptable (e.g. comparable to some minor adverse events), while a significant worsening would be unacceptable. Nonetheless, according to the decision rule in , the respiratory response can be infinitely harmful, while a treatment would still be classified as superior as long as there is a positive bulbar response. In , therefore, we extended the alternative strategy with a scalable non-inferiority boundary -Δ. If the confidence interval of the subscale treatment effect contains the non-inferiority boundary -Δ, a potentially harmful subscale effect cannot be excluded and the treatment is classified as not non-inferior (Citation23). In this case, the illustrated trial illustrated would be classified as ineffective as there is a not non-inferior respiratory effect, despite the positive bulbar response. The exact value of -Δ can be based on a priori expectations and is further detailed in the Supplementary methods (Citation23).
Comparing analytical strategies
Finally, we used the simulation model to generate clinical trial data with different treatment efficacy scenarios, where treatment effects could vary across subscales (e.g. all subscales respond identically to treatment vs. subscales respond differently to treatment). On each simulated trial, we applied the three analytical strategies (). Our primary focus was on empirical power, defined as the proportion of simulation samples in which the null hypothesis of no treatment effect was rejected. All scenarios were evaluated using a fixed sample size of 124 per arm and a 1:1 randomization ratio. The chosen sample size provides 80% power to detect a 25% total score slope reduction during a 12-month follow-up period with monthly visits and a one-sided alpha of 5% (Citation24). Each scenario was simulated 25,000 times, which provides 99% accuracy in determining the type 1 error (5%) of the analytical strategies between 4.6% and 5.4% (Citation5). In order to facilitate the translation of empirical power to trial design, we calculated sample sizes based on the empirical power using the formula provided by Healy and Schoenfeld (Citation4). A detailed description of the simulation, the model parameters and the source code can be found at http://reactive.tricals.org.
Results
In total, our simulation model was based on 26,920 ALSFRS-R scores from 3412 patients; a detailed description of the patient characteristics can be found elsewhere (Citation20). The observed rates of decline for each subscale and the total score are given in . If used in isolation, the total score would require the smallest sample size or achieve the highest power compared to its subscales. In we provide the empirical power for various treatment efficacy scenarios of the total score and the two alternative analytical strategies (i.e. with or without a non-inferiority boundary). Scenario I reflects a situation when treatment has no effect and provides the type I error of each endpoint; all strategies adequately control type I error.
Table 2 Observed longitudinal model parameters of the subscales and total score.
Table 3 Empirical power of the total score and two alternative analytical strategies.
The value of the non-inferiority boundary is illustrated in scenario II, which reflects a situation with a motor and respiratory benefit of treatment, but where treatment is harmful for bulbar functioning. The alternative analytical strategy, without non-inferiority boundary, would classify this treatment in 73.0% of the simulations as effective despite its potential harmful side-effects. An important consideration is that in this scenario, on average, there is an overall beneficial effect (i.e. a 10.6% slowing of the total score slope). As a consequence, the total score considers this treatment as effective, whereas the alternative analytical strategy with non-inferiority boundary classifies it as futile. Whether such a treatment is truly (in)effective might be debatable and such discussions could help define values for -Δ at the design stage.
In terms of treatment benefit, a uniform scenario, where treatment reduces all subscale slopes identically by 25%, is best detected by the total score (, scenario V). Nevertheless, the gain in empirical power as compared to the alternative analytical strategies is less than 5%. In case of non-uniform subscale-specific treatment effects, utilizing the alternative strategy may be a more powerful approach. In scenario VI, for example, all subscales respond, but one subscale responds more than the others. Employing the alternative strategy increases empirical power from 80.9% to 91.7%. In terms of sample size, this means that a trial based on the total score requires a sample size of 244 patients to detect the treatment effect with 80% power, whereas the alternative strategy requires only 174 patients (–28.7%). Similarly, for a scenario based on the recent trial with sodium phenylbutyrate–taurursodiol, where treatment benefit was largest on the fine motor subscale (Citation25), power increases from 83.8% to 86.0%. In general, as the differences in treatment responses between subscales increase, the more the ALSFRS-R total score becomes affected (e.g. in scenario III the total score requires 1380 patients vs. 336 (–75.7%) when utilizing the alternative strategy). In the Supplementary results we illustrate the mechanism that drives the loss of power of the total score for non-uniform treatment scenarios.
Discussion
In this simulation study, we have evaluated the performance of the ALSFRS-R total score for a variety of treatment efficacy scenarios. Our results highlight the potential consequences of ignoring the multidimensional structure of the total score in clinical trials for ALS. The ALSFRS-R total score may be insensitive to detecting treatment benefit when a treatment affects only some of its subscales, or benefits subscales in varying degrees, resulting in potentially higher false-negative rates and an increased risk of missing important treatment clues. Implementing an alternative analytical strategy that first assesses the subscale-specific effects, prior to making a decision about whether a treatment is generally effective, may circumvent the pitfalls of the total score.
These results may not only have important consequences for the design and analysis of future trials, but may also question past observations. One could hypothesize, for example, that the absent riluzole effect on the ALSFRS-R total score (Citation26,Citation27), despite the clear survival benefit (Citation28), may be driven by a non-uniform treatment effect on one of the subscales (Citation29). Unfortunately, subscale-specific treatment effects are rarely reported in ALS clinical trials and it is not known how treatments have affected the ALSFRS-R subscales in the past. The clinical trials with sodium phenylbutyrate–taurursodiol (Citation25), Nuedexta and Reldesemtiv (Citation19,Citation30), however, provide important evidence that these non-uniform treatments do exist and may dilute the treatment effect estimate when quantified by the ALSFRS-R total score.
Given our results and the potential for non-uniform treatment effects in ALS clinical trials, we recommend that the ALSFRS-R should no longer only be reported as a total score. To address the issues highlighted, it is necessary to account appropriately for the multidimensional structure of the ALSFRS-R prior to making a definite statement about treatment benefit. We evaluated a simple, alternative testing strategy that can easily be implemented in any statistical software package and which will not affect the general conduct of a trial. The non-inferiority boundary can be fine-tuned for each subscale individually, or used more conservatively when there is prior evidence of a potential harmful side-effect. Moreover, it is important to consider at the design stage which treatment effects would still be considered effective. For example, is a treatment that improves the total score, but has detrimental consequences for one of the subscales, still a valid treatment option (, scenario II)? Or is a treatment that minimally improves the total score, but has some beneficial effects on its subscales (, scenario III) worthwhile? Answering these questions is important in optimizing the proposed analytical strategies and may require consensus discussions with clinicians and patients.
In terms of trial design, if there is no a priori knowledge of the treatment effect, sample size calculation is straightforward and could be done by conservatively assuming a uniform scenario. Increasing the estimated sample size for the ALSFRS-R total score by 12.5% provides identical power for the alternative analytical strategy under a uniform treatment scenario () (Citation4), while having sufficient power to detect non-uniform treatment effects. A minimum value for –Δ can subsequently be calculated for each subscale individually using the estimated sample size and desired type 1 error level.
Our study has a few limitations that should be considered. The multidimensional structure of the ALSFRS-R makes the total score essentially a composite endpoint (Citation18). We evaluated a relatively simple, assumption-free analytical strategy that corrects solely for multiple testing. Nevertheless, more complex alternatives could be considered, such as defining a prospective testing hierarchy of the subscales, applying a weighting scheme or using multivariate mixed effects models (Citation18,Citation31–33). These alternatives may further optimize operational characteristics, but could increase the complexity of the design or interpretation of future clinical trials. Furthermore, there are several definitions of ALSFRS-R subscales reported in literature (Citation7,Citation9,Citation15,Citation16,Citation34), where fine and gross motor function are either combined or taken separately. This may affect the operational characteristics of the alternative analytical strategy as using a combined motor subscale would reduce the number of tests and may improve statistical power. Given the strong correlation between the fine and motor subscales () (Citation34), our power estimate of the alternative analytical strategy might be too conservative. More importantly, the operational characteristics of the analytical strategies are primarily driven by the ability of the subscales to detect the treatment effect. Thus, improving the sensitivity of the individual subscales may be an important target for future research. For example, replacing the bulbar subscale by the Center for Neurological Study Bulbar Function Scale (CNS-BFS) (Citation35), or the motor items by the Rasch-Built Overall ALS Disability Scale (ROADS) (Citation36), may further increase the likelihood of detecting effective treatments. In addition, new scales may reduce the occurrence of plateaus and reversals due to a more linear measurement scale and improved consistency in scoring (e.g. preventing a false “reversal” of ALSFRS-R item 2 when treating sialorrhea) (Citation36–38).
Finally, combining survival time with the ALSFRS-R has been shown to increase precision or reduce sample size (Citation3,Citation5). It would be of interest to extend the joint modeling framework, or similar strategies, to a multivariable model in which each subscale is modeled as covariate. Such a strategy could potentially lead to additional efficiency gains, but its application may be restricted to relatively long studies that have sufficient information on survival time. This limitation may be ameliorated by making better use of adaptive strategies such as seamless phase II to III designs. In these settings, a decision could be based initially on accruing ALSFRS-R subscale information, and, if there is sufficient survival data, the decision process may be shifted to the joint modeling framework. A recent example of such an approach is the STAMPEDE trial (Citation39). Additional simulation studies will be required to evaluate when such approaches are indicated, with development of appropriate methodology (Citation40). This simultaneously underscores the need to continuously update open-source databases such as PRO-ACT in order to obtain representative simulation tools for future studies.
In conclusion, in this simulation study, we show that ignoring the multidimensional structure of the ALSFRS-R total score could have potentially negative consequences for ALS clinical trials. We propose determining treatment benefit on a subscale level, prior to stating whether a treatment is generally effective. This strategy circumvents the pitfalls of the total score and may increase the likelihood of finding an effective treatment for this debilitating disease.
Supplemental Material
Download MS Word (630.6 KB)Declaration of interest
The authors report no conflicts of interest. The authors alone are responsible for the content and writing of this article.
Availability of data and materials
The dataset supporting the conclusions of this article is available in the PRO-ACT repository, https://nctu.partners.org/ProACT. The code that was used in this article is available at http://reactive.tricals.org.
Additional information
Funding
References
- European Medicines Agency. Guideline on clinical investigation of medicinal products for the treatment of amyotrophic lateral sclerosis. https://www.ema.europa.eu/documents/scientific-guideline/guideline-clinical-investigation-medicinal-products-treatment-amyotrophic-lateral-sclerosis_en.pdf, 2016, Accessed 19 Nov 2020.
- Food Drug Administration Center for Drugs Evaluation Research. Guidance for industry: amyotrophic lateral sclerosis: developing drugs for treatment. https://www.fda.gov/downloads/Drugs/GuidanceComplianceRegulatoryInformation/Guidances/UCM596718.pdf, 2019. Accessed 19 Nov 2020.
- Berry JD, Miller R, Moore DH, Cudkowicz ME, van den Berg LH, Kerr DA, et al. The Combined Assessment of Function and Survival (CAFS): a new endpoint for ALS clinical trials. Amyotroph Lateral Scler Frontotemporal Degener. 2013;14:162–8.
- Healy BC, Schoenfeld D. Comparison of analysis approaches for phase III clinical trials in amyotrophic lateral sclerosis. Muscle Nerve. 2012;46:506–11.
- van Eijk RPA, Eijkemans MJC, Rizopoulos D, van den Berg LH, Nikolakopoulos S. Comparing methods to combine functional loss and mortality in clinical trials for amyotrophic lateral sclerosis. Clin Epidemiol. 2018;10:333–41.
- van Eijk RPA, Kliest T, van den Berg LH. Current trends in the clinical trial landscape for amyotrophic lateral sclerosis. Curr Opin Neurol. 2020;33:655–61.
- Cedarbaum JM, Stambler N, Malta E, Fuller C, Hilt D, Thurmond B, et al. The ALSFRS-R: a revised ALS functional rating scale that incorporates assessments of respiratory function. BDNF ALS Study Group (Phase III). J Neurol Sci.1999;169:13–21.
- Kimura F, Fujimura C, Ishida S, Nakajima H, Furutama D, Uehara H, et al. Progression rate of ALSFRS-R at time of diagnosis predicts survival time in ALS. Neurology 2006;66:265–7.
- Bakker LA, Schröder CD, Tan HHG, Vugts SMAG, van Eijk RPA, van Es MA, et al. Development and assessment of the inter-rater and intra-rater reproducibility of a self-administration version of the ALSFRS-R. J Neurol Neurosurg Psychiatry. 2020;91:75–81.
- Rutkove SB. Clinical measures of disease progression in amyotrophic lateral sclerosis. Neurotherapeutics. 2015;12:384–93.
- Balendra R, Jones A, Jivraj N, Knights C, Ellis CM, Burman R, et al. Estimating clinical stage of amyotrophic lateral sclerosis from the ALS Functional Rating Scale. Amyotroph Lateral Scler Frontotemporal Degener. 2014;15:279–84.
- Chiò A, Hammond ER, Mora G, Bonito V, Filippini G. Development and evaluation of a clinical staging system for amyotrophic lateral sclerosis. J Neurol Neurosurg Psychiatry. 2015;86:38–44.
- de Jongh AD, van Eijk RPA, van den Berg LH. Evidence for a multimodal effect of riluzole in patients with ALS? J Neurol Neurosurg Psychiatry. 2019;90:1183–4.
- Franchignoni F, Mandrioli J, Giordano A, Ferro S. A further Rasch study confirms that ALSFRS-R does not conform to fundamental measurement requirements. Amyotroph Lateral Scler Frontotemporal Degener. 2015;16:331–7.
- Franchignoni F, Mora G, Giordano A, Volanti P, Chiò A. Evidence of multidimensionality in the ALSFRS-R Scale: a critical appraisal on its measurement properties using Rasch analysis. J Neurol Neurosurg Psychiatry. 2013;84:1340–5.
- Rooney J, Burke T, Vajda A, Heverin M, Hardiman O. What does the ALSFRS-R really measure? A longitudinal and survival analysis of functional dimension subscores in amyotrophic lateral sclerosis. J Neurol Neurosurg Psychiatry. 2017;88:381–5.
- van den Berg LH, Sorenson E, Gronseth G, Macklin EA, Andrews J, Baloh RH, et al. Revised Airlie House consensus guidelines for design and implementation of ALS clinical trials. Neurology 2019;92:e1610–e1623.
- Song M-K, Lin F-C, Ward SE, Fine JP. Composite variables: when and how. Nurs Res. 2013;62:45–9.
- Smith R, Pioro E, Myers K, Sirdofsky M, Goslin K, Meekins G, et al. Enhanced Bulbar function in amyotrophic lateral sclerosis: the Nuedexta Treatment Trial. Neurotherapeutics 2017;14:762–72.
- Atassi N, Berry J, Shui A, Zach N, Sherman A, Sinani E, et al. The PRO-ACT database: design, initial analyses, and predictive features. Neurology 2014;83:1719–25.
- Thakore NJ, Lapin BR, Kinzy TG, Pioro EP. Deconstructing progression of amyotrophic lateral sclerosis in stages: a Markov modeling approach. Amyotroph Lateral Scler Frontotemporal Degener. 2018;19:483–94.
- Hommel G. A stagewise rejective multiple test procedure based on a modified Bonferroni test. Biometrika 1988;75:383–6.
- Schumi J, Wittes JT. Through the looking glass: understanding non-inferiority. Trials 2011;12:106.
- van Eijk RPA. Frequent self-assessments in ALS Clinical Trials: worthwhile or an unnecessary burden for patients? Ann Clin Transl Neurol. 2020;7:2074–5.
- Paganoni S, Macklin EA, Hendrix S, Berry JD, Elliott MA, Maiser S, et al. Trial of Sodium Phenylbutyrate-Taurursodiol for Amyotrophic Lateral Sclerosis. N Engl J Med. 2020;383:919–30.
- Ong ML, Tan PF, Holbrook JD. Predicting functional decline and survival in amyotrophic lateral sclerosis. PLoS One. 2017;12:e0174925.
- Watanabe H, Atsuta N, Nakamura R, Hirakawa A, Watanabe H, Ito M, et al. Factors affecting longitudinal functional decline and survival in amyotrophic lateral sclerosis patients. Amyotroph Lateral Scler Frontotemporal Degener.2015;16:230–6.
- Lacomblez L, Bensimon G, Leigh PN, Guillet P, Meininger V. Dose-ranging study of riluzole in amyotrophic lateral sclerosis. Lancet. 1996;347:1425–31.
- Fang T, Al Khleifat A, Meurgey J-H, Jones A, Leigh PN, Bensimon G, et al. Stage at which riluzole treatment prolongs survival in patients with amyotrophic lateral sclerosis: a retrospective analysis of data from a dose-ranging study. Lancet Neurol. 2018;17:416–22.
- Shefner JM, Andrews JA, Genge A, et al. A phase 2, double-blind, randomized, dose-ranging trial of reldesemtiv in patients with als. Amyotroph Lateral Scler Frontotemporal Degener 2020;1–13.
- Ristl R, Urach S, Rosenkranz G, Posch M. Methods for the analysis of multiple endpoints in small populations: a review. J Biopharm Stat. 2019;29:1–29.
- Rizopoulos D. Joint models for longitudinal and time-to-event data: with applications in R. Boca Raton: CRC Press, 2012: p. xiv, 261.
- Belitskaya-Lévy I, Wang H, Shih M-C, Tian L, Doros G, Lew RA, et al. A new overall-subgroup simultaneous test for optimal inference in biomarker-targeted confirmatory trials. Stat Biosci. 2018;10:297–323.
- Thakore NJ, Lapin BR, Pioro EP. Trajectories of impairment in amyotrophic lateral sclerosis: insights from the pooled resource open-access ALS. Muscle Nerve. 2018;57:937–45.
- Smith RA, Macklin EA, Myers KJ, Pattee GL, Goslin KL, Meekins GD, et al. Assessment of bulbar function in amyotrophic lateral sclerosis: validation of a self-report scale (Center for Neurologic Study Bulbar Function Scale). Eur J Neurol. 2018;25:907–e66.
- Fournier CN, Bedlack R, Quinn C, Russell J, Beckwith D, Kaminski KH, et al. Development and validation of the Rasch-Built Overall Amyotrophic Lateral Sclerosis Disability Scale (ROADS). JAMA Neurol. 2020;77:480–8.
- Bedlack RS, Vaughan T, Wicks P, Heywood J, Sinani E, Selsov R, et al. How common are ALS plateaus and reversals? Neurology 2016;86:808–12.
- Vasta R, D’Ovidio F, Canosa A, Manera U, Torrieri MC, Grassano M, et al. Plateaus in amyotrophic lateral sclerosis progression: results from a population-based cohort. Eur J Neurol. 2020;27:1397–404.
- Sydes MR, Parmar MKB, James ND, Clarke NW, Dearnaley DP, Mason MD, et al. Issues in applying multi-arm multi-stage methodology to a clinical trial in prostate cancer: the MRC STAMPEDE trial. Trials 2009;10:39.
- Hu J, Blatchford PJ, Goldenberg NA, Kittelson JM. Group sequential designs for clinical trials with bivariate endpoints. Stat Med. 2020;39:3823–39.