Abstract
The liver is an amazing organ due to its powerful regenerative capacity. Although many studies on liver regeneration have been documented, the detailed mechanisms remain unclear. Two-third partial hepatectomy (PH) in rodents plays a crucial role in the study of liver regeneration. In this study, the time series data of gene expression during liver regeneration in mouse were analyzed using the gene set numbered GSE6998 in GEO. A variety of bioinformatics methods, including masigPro, Weighted Gene Co-expression Network Analysis (WGCNA), spatial analysis of functional enrichment (SAFE) and ingenuity canonical pathway analysis (IPA) were used to identify and compare the significantly changed pathways, potential upstream regulators and key genes during liver regeneration. Our study showed that liver regeneration in the mouse is a coordinated process, which cell-cycle-related progress are at the centre of the interaction network involved in liver regeneration. Several candidate upstream regulators including PPARA, NFE2L2, MAD1 and CNR1 and some key genes such as Cdk1, Plk1, Cdc20, Aurka, Racgap1, Cenpa, Rrm1, Rrm2 were identified. In conclusion, these findings could contribute to revealing the molecular mechanism of liver regeneration after PH, which could provide new ideas and treatment methods for regenerative medicine, oncological drug development and oncological treatment.
Introduction
The liver plays a major role in maintaining the body’s metabolic stability including carbohydrate, lipids and protein. Its most amazing feature is its powerful regenerative capacity. In rodent liver regeneration studies, the most effective surgical model is a 2/3 partial hepatectomy [Citation1]. After hepatectomy, the liver regains its original weight within two weeks through a process called liver regeneration. Once the remaining liver reaches the functional needs of the organ, the regeneration process stops. This process takes about two rounds of the cell cycle. There have been many studies on the molecular mechanisms of liver regeneration, and many cytokines and growth factors have been confirmed to be involved, but the specific molecular mechanisms remain unclear [Citation2–5].
Most of the previous studies focused on differentially expressed genes at a certain time point or certain stage during liver regeneration [Citation6,Citation7]. In our study, the dynamic changes in gene expression profile were explored during the liver regeneration in the mouse. At the same time, the candidate pathways associated with liver regeneration were explored using a series of bioinformatics methods. To the best of our knowledge, this is the first report describing the dynamic changes in gene expression during LR in the mouse.
In this study, the DEGs were identified using maSigPro software [Citation8], and the gene co-expression network was constructed using WGCNA [Citation9,Citation10]. Next, the canonical pathway analysis was performed by ingenuity pathway analysis (IPA) on the above modules identified by WGCNA [Citation11]. Spatial analysis of functional enrichment (SAFE) was used to identify the potential key pathways during LR [Citation12,Citation13]. The identification of Hub–Bottlenecks genes was performed by CytoHubba plugin in Cytoscape [Citation14–16].
Materials and methods
Data processing
The raw data in the .CEL format of GSE6998 provided by Out HH et al. is available for download at GEO (https://www.ncbi.nlm.nih.gov/geo/) [Citation17]. The chip used in this experimental series is the Genome 430 2.0 ARRay. A total of 20 tissue samples were divided into 10 consecutive time points. Each experimental time point is represented by two separate samples consisting of at least 3 pooled tissues from different mice. The downloaded .CEL file is converted to expression data using the Robust Muliti-array Average Algorithm (RMA).
The identification of differentially expressed genes during liver regeneration
Differentially expressed genes (DEG) during dynamic changes in liver regeneration at different time points in mice were identified using maSigPro software [Citation8,Citation18,Citation19]. Genes with significant changes in expression between the experimental and control groups were identified by dummy variables, which was defined by maSigPro as a two-regression step method. In our study, a four-element regression model was defined for analysis, and this model has enough power to calculate the number of time points of reduction. The stepwise regression method was taken with the parameters set as follows, step.method = "two.ways.backward", α = .05), and the R2 cut-off value was fitted to .7 (rsq = .7).
Construction of weighted gene co-expression networks
Weighted Gene Co-Expression Network Analysis (WGCNA) has been widely used to analyze gene expression data, such as the discovery of modules of interest and hub genes within modules [Citation9,Citation10,Citation20]. In our study, the DEGs obtained by maSigPro were used to construct a co-expression network.
Ingenuity pathway analysis (IPA) of the genes in each module from WGCNA
The ingenuity pathway analysis (IPA) was used to explore and compare the relationship among the enrichment results from genes within each module [Citation21,Citation22]. At the dataset uploading, the kME of each gene in every module was set to “Expr log Ratio” option. So the genes which positively and negatively correlated with the outer trait were positive and negative value respectively.
In order to compare the similarity and difference among the enriched pathways and upstream regulator, the comparison analysis was performed using the comparison analysis function in IPA. The heat map was allowed to visualize the canonical pathways and upstream regulators correlate with multiple analysis simultaneously which allow us to identify pathways and upstream regulators whose activity appears to increase or decrease in our interesting modules.
The identification of key modules
Genes with similar expression patterns tend to share the same biological process or signalling pathway, meaning that these genes have specific biological implications, and this relationship between genes can be defined in biological networks. Spatial analysis of functional enrichment (SAFE) can help us understand how biological networks are functionally organized and can annotate biological networks and examine functional organization [Citation12,Citation13,Citation23]. STRING is used to construct a network of protein–protein interactions, with closely related genes, be close together. The node in the network represents all known LR-related genes downloaded from NCBI and all DEGs obtained from maSigPro. An edge represents the relationship between genes [Citation24]. The distribution of genes in the network is shown by applying an edge-weighted spring embedding layout style in Cytoscape, and all nodes are located according to their co-occurrence [Citation15,Citation25]. The genes in the four modules obtained from WGCNA are each overlapped into the network. Key modules were identified based on the location distribution of these genes and the overlap with known LR-related genes. In addition, the MCODE plugin is used to identify densely connected areas in the above network [Citation26].
The identification of hub–bottlenecks genes
In gene co-expression networks, high-connectivity genes are critical to the maintenance of overall network stability, and these genes are called hub genes (high degrees). In addition to the degree, intermediation is also one of the important topological properties of the network. It represents the number of shortest paths through a node, and these nodes with high mediation are called bottlenecks. In WGCNA, intra-module connectivity and correlation with module eigengenes were used to select hub genes without any statistical criteria [Citation20]. According to Han [Citation27], the genes for both hub and bottlenecks play a more important role in the PPI network [Citation28]. In our study, the degree and bottleneck of each gene in the above-constructed network were calculated by the CytoHubba plugin. At the same time, the top 5% of the genes both in degree and bottlenecks are defined as the Hub–Bottlenecks genes.
Results
Differentially expressed genes during liver regeneration in mouse using maSigPro
Raw data in .cel format for 20 tissue samples from GSE13149 were downloaded from NCBI and were converted to expression data using the Robust Multi-array Average (RMA) algorithm based on R language including background correction, normalization, and summarization. A total of 1128 DEGs were screened using masigpro software according to the details described in the materials and methods section.
Construction of weighted gene co-expression networks and identification of modules associated with liver regeneration
The co-expression network was constructed from the DEGs obtained from maSigPro during LR with 7 modules were identified including the grey module. The soft threshold power 10 was chosen to define the adjacency matrix based on the criterion of approximate scale-free topology (). Other parameters were set as follows: the minimum module size was set to 30, the module detection sensitivity deepSplit was 2 and cut height for merging of modules was 0.2 which meant that the modules whose eigengenes were correlated above 0.8 will be merged (). The modules associated with different time point during LR were identified according to the module-trait relationships (). The black, blue, brown and cyan module was positively correlated with the priming stage of LR. The blue module is correlated with 0–2 h, 6–18 h, 24–48 h and 72 h after PH, respectively. The relationship between module eigengenes was analyzed ().
Figure 1. Weighted gene co-expression network analysis. (A) Network topology for different soft-thresholding powers. Numbers in the plots indicate the corresponding soft thresholding powers. (B) Gene dendrogram obtained by clustering the dissimilarity based on consensus Topological Overlap with the corresponding module colours indicated by the colour row. Each coloured row represents a colour-coded module which contains a group of highly connected genes. (C) Relationships of consensus module eigengenes and different time point during liver regeneration. Numbers in the table report the correlations of the corresponding module eigengenes and stage, with the p-values printed below the correlations in parentheses. Intensity and direction of correlations are indicated on the right side of the heatmap (red, positively correlated; green, negatively correlated). (D) Correlation of eigengenes of each module in WGCNA. The lower left triangle is the correlation coefficient of the eigengenes between any two modules, and the right upper triangle is the corresponding fitting curve.
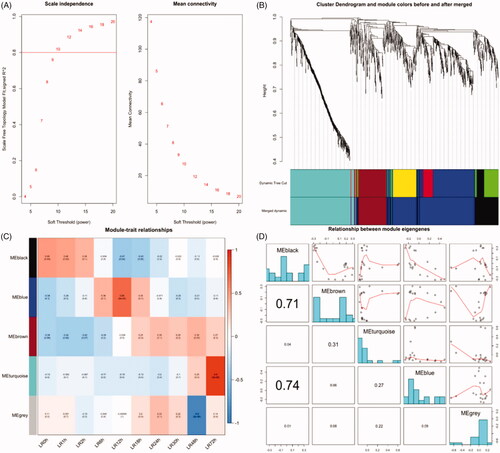
Comparison analysis of canonical pathway using IPA
The enriched pathways across four modules were shown in according to the IPA comparison analysis results. It can be seen that the genes in the turquoise module primarily related to cell cycle progression and DNA damage-related pathways. The genes in the black module enriched in acute phase response signalling and IL-6 pathway. The blue and turquoise module associated with p53 signalling mainly. The visualization of pathway activity analysis was shown in . Through the activity analysis function, we can find that cell-cycle-related progress correlated negatively with 72 h after PH.
Figure 2. Canonical pathway and comparison analysis for genes in each module using IPA. (A) The heat map of the canonical pathway across different modules displaying the Fisher’s exact test p-value. (B) The heat map displays the z-score from pathway activated analysis (orange and blue rectangles represent activation and suppression, respectively). (C) The visualization of genes which are positively or negatively correlated with acute phase response signalling using the gene level heat map. (D) The visualization of genes which are positively or negatively correlated with IL-6 Signaling using the gene level heat map.
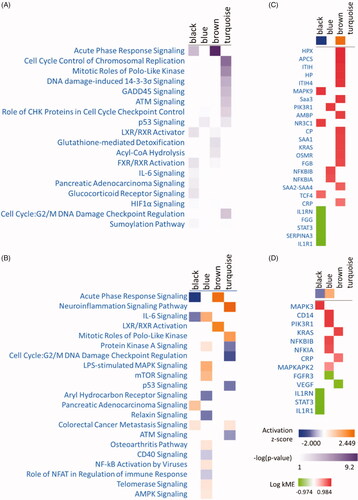
Some pathways positively or negatively correlated with different time points () were identified. The genes that are positively or negatively correlated with acute phase response and IL-6 signalling in four modules were visualized using the gene level heat map ( and )).
Comparison analysis of upstream regulator using IPA
The potential upstream regulators of the genes in four modules were identified using IPA upstream analysis. The potential upstream regulators including PPARA, NFE2L2, IL6, MED1, MYC, NR1H4 etc. (). There were no predicted upstream regulators in the black module. PPARA and NFE2L2 were predicted regulators which positively correlated with a blue module which were consistent with previous results (). At the same time, they were the only two regulators which enriched across two modules which showed that they played their roles in a different pattern. According to previous studies, PPARA play a vital role during liver regeneration in rat and mouse [Citation29–33]. As an important regulator of the antioxidant defence, elevated NFE2L2 expression impairs liver regeneration by inducing apoptosis and delaying proliferation of hepatocytes [Citation34–36].
Figure 3. Comparison analysis heat map for upstream regulators in each module using a comparison analysis function in IPA. (A) The heat map displays the z-score from Upstream Regulator Analysis (orange and blue rectangles represent activation and suppression, respectively). (B) The relevant network of PPARA in the blue module. (C) The relevant network of PPARA in the brown module. (D) The relevant network of NFE2L2 in blue module. (E) The relevant network of NFE2L2 in the brown module.
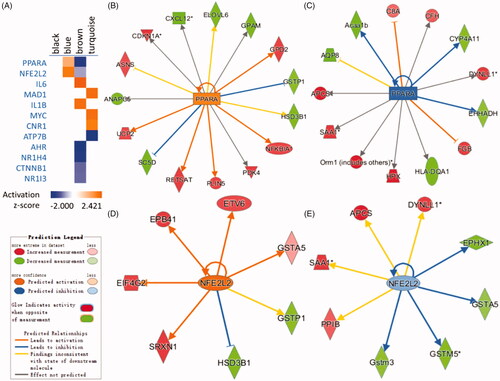
Key candidate modules and genes identification using protein–protein network (PPI) and spatial analysis of function enrichment (SAFE)
The protein–protein interaction network for LR containing 993 nodes and 7177 edges was constructed by connecting the genes which included 219 known LR-related genes downloaded from National Center for Biotechnology Information (NCBI) and the 1128 DEGs during LR in the mouse. The network was visualized by applying the spring-embedded layout algorithm in Cytoscape as shown in . Next, the genes sharing the similar expression profiles in each module from WGCNA were overlapped into the network with different colours corresponding to different modules as shown in . It can be found that genes in the turquoise module arranged closely. The known LR-related genes (the yellow dot pointed by the arrow) were overlapped into the network as shown in . Notably, the known genes related to liver regeneration were overlapped mostly into the blue and turquoise module which represented p53 signalling and cell-cycle progress respectively. It can be inferred turquoise and the blue module may play vital roles during liver regeneration. Top 5 significant modules were identified which concluded more than 10 nodes (). The genes in the top 2 significant modules were overlapped into blue and turquoise modules mostly (). Module 1, which consisted of 76 nodes and 1147 edges (), mainly associated with cell-cycle progress and p53 signalling. Module 2, which consisted of 50 nodes and 379 edges, mainly associated with DNA replication.
Figure 4. Distribution of known LR-related genes and DEGs in the spatial protein–protein interaction network. A map of the network was generated in Cytoscape. (A) A protein–protein interaction network was constructed by connecting all the known LR-related genes and DEGs in our study. (B) All the DEGs from 4 modules were coloured in different colours as shown in the figure. (C) All known LR-related genes were mapped to the PPI network shown in yellow. These genes are overlapped mostly with the blue model.
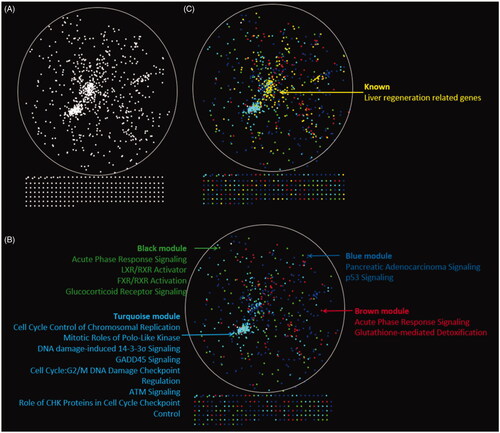
Figure 5. Top 5 significant modules consisting of more than 10 10 nodes identified by MCODE and the distribution in PPI network. (A–E) Five modules identified by MCODE plugin. (F) The distribution of Module 1, which are mainly associated with cell-cycle progress and p53 signalling. (G) The distribution of Module 2, which mainly associated with DNA replication.
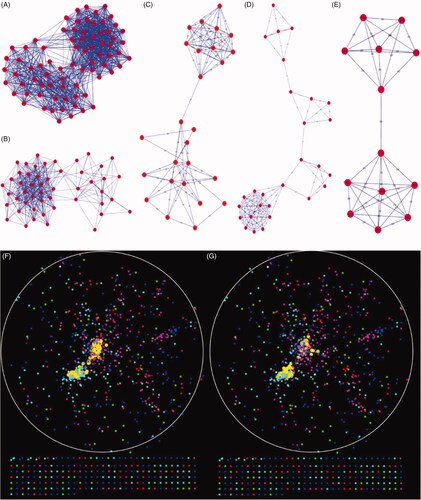
The degree and betweenness of every gene in the network was calculated respectively using cytohubba plugin. Eight Hub–Bottlenecks genes including Cdk1, Plk1, Cdc20, Aurka, Racgap1, Cenpa, Rrm1, Rrm2 were identified. Interesting, all the Hub–Bottlenecks genes were all in the turquoise module, which showed the importance of the module.
Discussions
Regenerative biology and regenerative medicine aim to explore the mechanisms of regeneration and apply them to the clinic. People’s interest in liver regeneration research has increased dramatically, and a lot interesting things have been found both in biological science and clinical perspective [Citation37–39]. The complexity of liver regeneration exceeds people’s imagination. Liver regeneration is a unique model that can simultaneously study signal transduction and cell-cycle events in vivo. From a clinical point of view, understanding the mechanisms of liver regeneration can lead to the development of new therapies for proper management and for some important diseases such as acute liver failure and cirrhosis.
The main purpose of our study was to explore the biological processes or signalling pathways, key genes and upstream regulatory factors that regulate differentially expressed genes that have undergone significant changes during liver regeneration. The 1183 DEGs obtained from MASIGPRO were divided into 4 modules by WGCNA according to their expression patterns. The genetic changes within each module are strongly time-dependent, which also validates the orderliness of liver regeneration. Different modules have significant correlations with different time points. It can be found that, the black module represented by the acute phase response signalling and IL-6 signalling positively correlated with the priming stage mainly which consistent with previous studies [Citation40]. PPARA, NFE2L2 were identified upstream regulators which potentially regulate DEGs in two modules. The role of PPARA and NFE2L2 in liver regeneration has been reported [Citation29–32,Citation35,Citation41]. Blue and turquoise module lie at the centre of interaction network, which indicated that p53 signalling and cell-cycle progress play vital roles in liver regeneration. Eight Hub–Bottlenecks genes including Cdk1, Plk1, Cdc20, Aurka, Racgap1, Cenpa, Rrm1, Rrm2 were identified. It is worth noting that all the above genes belong to the turquoise module, which verifies the importance of turquoise module. According to the previous study, CDK1, CDC20 and CENPA overexpressed in lung adenocarcinoma cancer tissue with co-expression patterns, which showed that they might serve as a novel cluster of prognostic biomarkers [Citation42]. In gastric cancer, AURKA is directly associated with the expression of RACGAP1 [Citation43]. ARUKA transgenic mouse studies have shown that the overexpressed AURAKA could induce some phenotypic abnormalities including early entry into S phase which occurs at the priming stage during liver regeneration and cytokinesis failure [Citation44]. As one of the hub-bottlenecks genes, the role of AURKA in liver regeneration deserves further study. Next, we will screen some of the hub-bottlenecks genes and regulators for the validation and analysis of their mechanisms.
Conclusion
In conclusion, our study showed that liver regeneration in the mouse is a coordinated process and that the cell-cycle-related progress was at the heart of the interaction network involved in liver regeneration. Several candidate upstream regulators including PPARA, NFE2L2, MAD1 and CNR1 etc. were identified and some key genes such as Cdk1, Plk1, Cdc20, Aurka, Racgap1, Cenpa, Rrm1, Rrm2 were identified. These findings could contribute to revealing the molecular mechanism of liver regeneration after PH, which could provide new ideas and treatment methods for regenerative medicine, oncological drug development and oncological treatment.
Disclosure statement
The authors have declared that no competing interest exists.
Additional information
Funding
References
- Wei W, Dirsch O, McLean AL, et al. Rodent models and imaging techniques to study liver regeneration. Eur Surg Res. 2015;54:97–113.
- Alison MR, Lin WR. Regenerating the liver: not so simple after all?. F1000Res. 2016;5.
- Forbes SJ, Newsome PN. Liver regeneration - mechanisms and models to clinical application. Nat Rev Gastroenterol Hepatol. 2016;13:473–485.
- Michalopoulos GK. Liver regeneration after partial hepatectomy: critical analysis of mechanistic dilemmas. Am J Pathol. 2010;176:2–13.
- Tao Y, Wang M, Chen E, et al. Liver regeneration: analysis of the main relevant signaling molecules. Mediators Inflamm. 2017;2017:1.
- Jia C. Advances in the regulation of liver regeneration. Expert Rev Gastroenterol Hepatol. 2011;5:105–121.
- Su AI, Guidotti LG, Pezacki JP, et al. Gene expression during the priming phase of liver regeneration after partial hepatectomy in mice. Proc Natl Acad Sci USA. 2002;99:11181–11186.
- Conesa A, Nueda MJ, Ferrer A, et al. Masigpro: a method to identify significantly differential expression profiles in time-course microarray experiments. Bioinformatics. 2006;22:1096–1102.
- Giulietti M, Occhipinti G, Principato G, et al. Weighted gene co-expression network analysis reveals key genes involved in pancreatic ductal adenocarcinoma development. Cell Oncol (Dordr). 2016;39:379–388.
- Langfelder P, Horvath S. Wgcna: an R package for weighted correlation network analysis. BMC Bioinformatics. 2008;9:559.
- Presson AP, Sobel EM, Papp JC, et al. Integrated weighted gene co-expression network analysis with an application to chronic fatigue syndrome. BMC Syst Biol. 2008;2:95.
- Baryshnikova A. Systematic functional annotation and visualization of biological networks. Cell Syst. 2016;2:412–421.
- Costanzo M, VanderSluis B, Koch EN, et al. A global genetic interaction network maps a wiring diagram of cellular function. Science. 2016;353:6306.
- Chin CH, Chen SH, Wu HH, et al. Cytohubba: identifying hub objects and sub-networks from complex interactome. BMC Syst Biol. 2014;8:S11.
- Killcoyne S, Carter GW, Smith J, et al. Cytoscape: a community-based framework for network modeling. Methods Mol Biol. 2009;563:219–239.
- Yin L, Chang C, Xu C. G2/M checkpoint plays a vital role at the early stage of hcc by analysis of key pathways and genes. Oncotarget. 2017;8:76305–76317.
- Otu HH, Naxerova K, Ho K, et al. Restoration of liver mass after injury requires proliferative and not embryonic transcriptional patterns. J Biol Chem. 2007;282:11197–11204.
- Nueda MJ, Martorell-Marugan J, Marti C, et al. Identification and visualization of differential isoform expression in Rna-Seq time series. Bioinformatics 2018;34:524–526.
- Nueda MJ, Tarazona S, Conesa A. Next Masigpro: updating Masigpro bioconductor package for RNA-Seq time series. Bioinformatics. 2014;30:2598–2602.
- Bi D, Ning H, Liu S, et al. Gene expression patterns combined with network analysis identify hub genes associated with bladder cancer. Comput Biol Chem. 2015;56:71–83.
- Gao C, Devarajan K, Zhou Y, et al. Identifying breast cancer risk loci by global differential allele-specific expression (dase) analysis in mammary epithelial transcriptome. BMC Genomics. 2012;13:570.
- Kramer A, Green J, Pollard J, Jr, et al. Causal analysis approaches in ingenuity pathway analysis. Bioinformatics. 2014;30:523–530.
- Costanzo M, Baryshnikova A, Bellay J, et al. The genetic landscape of a cell Science. 2010;327:425–431.
- Szklarczyk D, Franceschini A, Wyder S, et al. V10: protein-protein interaction networks, integrated over the tree of life. Nucleic Acids Res. 2015;43:D447–D452.
- Shannon P, Markiel A, Ozier O, et al. Cytoscape: a software environment for integrated models of biomolecular interaction networks. Genome Res. 2003;13:2498–2504.
- Bader GD, Hogue CW. An automated method for finding molecular complexes in large protein interaction networks. BMC Bioinformatics. 2003;4:2.
- Han JD, Bertin N, Hao T, et al. Evidence for dynamically organized modularity in the yeast protein-protein interaction network. Nature. 2004;430:88–93.
- Yu H, Kim PM, Sprecher E, et al. The importance of bottlenecks in protein networks: correlation with gene essentiality and expression dynamics. PLoS Comput Biol. 2007;3:e59.
- Anderson SP, Yoon L, Richard EB, et al. Delayed liver regeneration in peroxisome proliferator-activated receptor-alpha-null mice. Hepatology. 2002;36:544–554.
- Gazit V, Huang J, Weymann A, et al. Analysis of the role of hepatic ppargamma expression during mouse liver regeneration. Hepatology. 2012;56:1489–1498.
- Liu HX, Fang Y, Hu Y, et al. PPARβ regulates liver regeneration by modulating Akt and E2f signaling. PLoS One. 2013;8:e65644.
- Yamamoto Y, Ono T, Dhar DK, et al. Role of peroxisome proliferator-activated receptor-gamma (ppargamma) during liver regeneration in rats. J Gastroenterol Hepatol. 2008;23:930–937.
- Yuan X, Yan S, Zhao J, et al. Lipid metabolism and peroxisome proliferator-activated receptor signaling pathways participate in late-phase liver regeneration. J Proteome Res. 2011;10:1179–1190.
- Kohler UA, Kurinna S, Schwitter D, et al. Activated Nrf2 impairs liver regeneration in mice by activation of genes involved in cell-cycle control and apoptosis. Hepatology. 2014;60:670–678.
- Zou Y, Hu M, Lee J, et al. Nrf2 is essential for timely M phase entry of replicating hepatocytes during liver regeneration. Am J Physiol Gastrointest Liver Physiol. 2015;308:G262–G268.
- Zou Y, Lee J, Nambiar SM, et al. Nrf2 is involved in maintaining hepatocyte identity during liver regeneration. PLoS One. 2014;9(9):e107423.
- Yi PS, Zhang M, Xu MQ. Role of microrna in liver regeneration. Hbpd Int. 2016;15:141–146.
- Sato Y, Katoh Y, Matsumoto M, et al. Regulatory signatures of liver regeneration distilled by integrative analysis of Mrna, histone methylation, and proteomics. J Biol Chem. 2017;292:8019–8037.
- Kiroplastis K, Fouzas I, Katsiki E, et al. The effect of sorafenib on liver regeneration and angiogenesis after partial hepatectomy in rats. Hippokratia 2015;19:249–255.
- Su AI, Guidotti LG, Pezacki JP, et al. Gene expression during the priming phase of liver regeneration after partial hepatectomy in mice. Proc Natl Acad Sci. 2002;99:11181–11186.
- Dayoub R, Vogel A, Schuett J, et al. Nrf2 activates augmenter of liver regeneration (alr) via antioxidant response element and links oxidative stress to liver regeneration. Mol Med. 2013;19:237–244.
- Liu WT, Wang Y, Zhang J, et al. A novel strategy of integrated microarray analysis identifies Cenpa, Cdk1 and Cdc20 as a cluster of diagnostic biomarkers in lung adenocarcinoma. Cancer Lett. 2018;425:43–53.
- Bornschein J, Nielitz J, Drozdov I, et al. Expression of aurora kinase A correlates with the Wnt-modulator RACGAP1 in gastric cancer Cancer Med. 2016;5:516–526.
- Li CC, Chu HY, Yang CW, et al. Aurora-a overexpression in mouse liver causes P53-dependent premitotic arrest during liver regeneration. Mol Cancer Res. 2009;7:678–688.