ABSTRACT
Several analysis methods exist to identify hotspots regarding resource efficiency in industry and consecutively find measures to reduce environmental impact. Still, many companies do not use systematic methods to analyse and improve their resource efficiency, partly because they do not have the competencies to apply them. In this context, learning factories support by providing a realistic environment to teach methodological competencies. This paper systematically derives criteria and compares hotspot analysis methods for the identification of resource efficiency hotspots. Based on these results, an approach is developed to derive needed competencies and a list of required technical infrastructure for learning factories. The developed tool includes the general ratings of the methods depending on prerequisites as well as the possibility to weight the criteria. Learning factory operators and trainers can use this tool to identify suitable hotspot analysis methods and get guidance on how to implement the methods in their learning environment.
1. Introduction
Since sustainability and mitigation of climate change gain increasing importance, the industry as the largest contributor to global greenhouse gas emissions, including emissions regarding energy demand, needs to become active (Ritchie & Roser, Citation2020). Hotspot analysis methods can be used to identify critical parts in industrial production sites regarding their environmental impact. Learning factories provide suitable learning environments to teach these methodological competencies – as shown in a recent study about existing topics regarding energy and resource efficiency in learning factories (Weyand et al., Citation2022). However, because of the large variety of existing hotspot analysis methods, learning factory operators need to choose the best ones for their target group and their respective requirements. Although advantages and disadvantages can be found for each method separately, guidance is missing for learning factory operators about which methods and respective comparison criteria to consider when approaching the topic of resource efficiency. Therefore, this paper presents a systematic comparison approach for hotspot analysis methods regarding resource efficiency by first defining criteria and second rating the methods based on the application results at the ETA Learning Factory at TU Darmstadt. When the hotspot analysis methods are chosen, the paper furthermore presents an approach to consequentially derive needed competencies and respective technical requirements for the learning factory.
2. Basics
2.1. Extension of learning factories
To understand the benefits of extending learning factories for new topics, first a learning factory has to be defined. According to the International Association of Learning Factories, a learning factory is defined as ‘[…] a learning environment where processes and technologies are based on a real industrial site […] Learning factories are based on a didactical concept emphasizing experimental and problem-based learning. The continuous improvement philosophy is facilitated by own actions and interactive involvement of the participants.’ (International Association of Learning Factories, Citation2021). The concept can be divided into learning factories in the narrow and broader sense. Learning factories in the narrow sense have a real value chain, where a physical product is manufactured, and communication of learning content is on-site. Learning factories in a broader sense, on the other hand, include for example virtual value chains (Riemann & Metternich, Citation2022) and hybrid workshops (B. Thiede et al., Citation2022). To design a learning factory, Tisch et al. (Citation2015) defined three levels, starting with the Macro level, addressing the learning factory with production environment and infrastructure. The Meso level is addressing the design of the learning modules, followed by the Micro level focussing on specific learning situations. The scope of the paper is focussing on the Macro level to support learning factory operators to remodel or extend their learning factory infrastructure according to the topic of resource efficiency.
During the last years, different design approaches were presented for learning factories. While most design approaches focus on the initial development of a learning factory, only a limited number tackles the learning factory life cycle phase of remodelling (Enke, Citation2019; Tisch & Metternich, Citation2017). In , design approaches focusing on or including steps also relevant for remodelling a learning factory are presented and compared. The approach by Tisch et al. is included as well since it is the most used design approach so far (Kreß et al., Citation2022). Remodelling approaches found in the literature that do not include an explicit design approach such as in (Gräßler et al., Citation2016; S. Thiede et al., Citation2016; Schuhmacher & Hummel, Citation2016) were not considered in the table. It is noticeable that all found remodelling approaches focus on the extension regarding digitalisation technologies (Enke, Citation2019), although some already include the context of resource efficiency (Böhner et al., Citation2018). Despite the different number and wording of steps, the four compared design approaches also differ in the arrangement of the steps. While the approaches by Tisch et al. (Citation2015) and Karre et al. (Citation2017) are depicted as linear with start and end points, the design approach by Plorin et al. (Citation2015) is structured as a circle. Doch et al. (Citation2015) also depicts a linear approach, but included continuous adjustment, hence it could be interpreted as a circle as well.
Table 1. Comparison of relevant design approaches and respective steps.
In all examined approaches, no focus was given to the answer of how exactly to determine the needed technical infrastructure in relation to the intended competencies. The focus of the previous design approach is on the detailed steps to develop the learning modules (like steps 1–6 in Plorin et al. or steps 1 and 3 in Karre et al.), but the implications given by the infrastructure are hardly considered. Therefore, based on the comparison of the existing design approaches and previous project experience in the extension of a learning factory and the chosen approach there (Weyand, Schmitt, et al., Citation2021), the approach depicted in was derived. It includes the requirement analysis as a first step, focusing on the analysis of the existing infrastructure, the organizational targets pursued with the extension as well as the target group that is either already addressed in the learning factory or the target group to be addressed by the process result. The second step is the derivation of intended competencies, followed by the deduction of resulting requirements for the learning environment. As mentioned before, these two steps are presented in the literature already for the topics of digitalisation technologies and industry 4.0 but are missing so far for the topic of resource efficiency. Hence, the scope of the paper is to provide guidance, especially in these two steps.
After the requirements for the learning environment are deducted, the needed changes can be defined by comparing the current with the target state. The missing components are implemented in the fifth step then and the learning modules are developed. It has to be noted that this development of learning modules is dependent on the intended infrastructure, which is why it can only start as soon as the pursued changes are defined.
2.2. Resource efficiency and hotspot analysis methods
According to Bergmann et al. (Citation2017), resource efficiency is defined as the needed amount of resources in relation to the generated output. Following the definition given in VDI 4800 (Citation2016), resources in the scope of the paper are understood as natural resources, including, for example, material and energy, but excluding financial or human resources. When addressing resource efficiency, one of the main competencies for employees is the ability to identify relevant spots in production, so-called hotspots, where resources might be wasted, or where the efficient use of resources might be increaseable. Based on the identified hotspots, measures can be determined and implemented to improve resource efficiency. This general process was published in a previous paper and is shown in (Weyand, Rommel, et al., Citation2021). Learning factories specifically can support the second step by building methodological competencies in realistic environments for trainees to test and get familiar with certain methods (Tisch & Metternich, Citation2017). Regarding resource efficiency, many different methods exist to perform the hotspot analysis in this step to optimise resource efficiency within a production facility. The existing methods range from complex ones, with many necessary measurements and impact categories analysed, to simpler ones with a low effort needed for the application. Due to this variety, the intended competencies and resulting requirements for the learning environment, as depicted in , depend on the chosen method for the hotspot analysis in the case of resource efficiency. Therefore, guidance is first needed for learning factory operators to choose which hotspot analysis methods to teach to their targets and target group. In the following sections, possible hotspot analysis methods are described, and a systematic comparison of these methods is developed.
Figure 2. The considered methods in the framework for increasing resource efficiency by Weyand, Rommel, et al. (Citation2021).
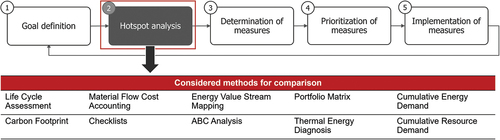
The selection of methods presented in this paper is based on a catalogue of methods compiled by the Association of German Engineers in the centre for resource efficiency (VDI ZRE) (Citation2021). The considered methods are chosen based on their specific focus on resource efficiency in production, which is why general lean management methods or methods for product development are not considered, although they might as well have a positive impact (Abualfaraa et al., Citation2020). Further development of the methods in the catalogue, like presented by Li et al. (Citation2017), was not considered but can also be included in the rating prospectively. The methods are briefly described in to make the later rating more comprehensible.
Table 2. Description of the considered methods.
3. Rating of the hotspot analysis methods
A systematic approach to rate the different hotspot analysis methods is depicted in . In the first step, general aspects of the rating system are defined. In the next step, categories for the rating system are systematically derived, based on literature analysis, analysis of the methods and involvement of stakeholders. In the third step, the scale division is defined based on a use case in a learning factory. After that is concluded, the topic of weighting the different criteria is discussed, and the methods are rated based on the defined scale. While the first three steps are the same for each learning factory, the adjustment of weighting factors and the final rating of methods is influenced by the requirement analysis (see ). The steps as well as the links towards the requirement analysis are described in detail in the following sections.
3.1. Definition of basic rating principles
The rating system aims at giving guidance to learning factory operators to choose a suitable hotspot analysis method to teach in their learning factory. Therefore, they need to consider various requirements from their respective target groups. Multiple characteristics influence if a method is considered to be suitable for a company, respectively, a possible target group for learning factories. To depict these, a multidimensional analysis with multiple criteria is chosen as a rating system. Another requirement for the rating system is a transferable rating, applicable to various production sites. A normalized scale, the use of weighting factors and visualization with Harvey balls is considered a suitable solution to this requirement.
3.2. Determination of rating criteria
The rating criteria are derived in three substeps. First, a literature analysis is conducted to find rating criteria used in other rating systems. Existing approaches for comparison of resource-related methods focus on energy-related methods (e.g. Li et al., Citation2017) or depict broader concepts (e.g. C. Schmidt et al., Citation2015). They are usually briefly described and used as a basis to point out research gaps. A systematic comparison as well as a general rating system for methods regarding resource efficiency is missing. However, the existing approaches are still useful to collect possible criteria like effort or considered resources, which are considered to be suitable also for the target of the paper. The second step to derive criteria is the detailed breakdown of the hotspot analysis methods regarding their advantages and disadvantages. The methods differ, for example, in the considered environmental impacts or life cycle phases. In the third step, the first set of criteria is discussed with experts and stakeholdersfor example, people in the industry as well as researchers in the field of resource efficiency, but also learning factory operators. Most of the drafted criteria were confirmed, but also minor remarks were made. The consulted learning factory operators for instance came up with the criterion of time needed to acquire the skills to perform each method since it can be a relevant factor for them in establishing a curriculum for their learning factories. The revised criteria are described further in the following.
In general, the criteria are divided into effort-related and benefit-related criteria. The effort can be quantified regarding time and cost, and both can also be subdivided. Time effort occurs on the one hand for the execution of the method, meaning the time needed to apply the method in a company. On the other hand, time is also needed for the initial skill adaption training in a learning factory. Here, first variations in the later rating can occur, depending on the initial competencies that the target group already has. One example is the Energy Value Stream Mapping (EVSM). Since it is based on the general Value Stream Mapping (VSM), workshop participants already familiar with this method will need a considerably less amount of time to be able to perform an EVSM. The same accounts for the methods where detailed resource measurements are needed (LCA, CF, EVSM, CED and CMD, see ). If the target group is not familiar with doing energy measurements, for example, time should be scheduled to teach competencies in this field when choosing to teach one of these methods.
The cost effort as a further criterion can be quantified regarding the costs needed for data acquisition. Included in this criterion are costs for sensors and related equipment needed to get sensor data, but also equipment like a thermal imaging camera which is needed for the Thermal Energy Diagnosis (TED). The second criterion related to costs is for evaluation and visualisation. This includes software for the evaluation of resource consumption regarding related CO2 emissionsfor example, databases to get the carbon footprint of raw materials, but also software for more complex calculations like they are needed for the MFCA. In the cost criteria, the same accounts for the variations depending on pre-analysed requirements; if it can be assumed that the respective target group already has sensors installed, at least partially, the respective additional costs for data acquisition will reduce. In case software like an LCIA database is already available for a target group, here again the costs will drop for the applicable methods. Basic calculation software like MS Excel© is assumed to be available at industrial companies and is therefore not considered in general. In detail, the different variations are described in the next section. To address these variations, a questionnaire for the requirement analysis is developed, presented in more detail in section 5.
The benefits, on the other hand, can be divided into four different categories. First, the benefits of the methods can be compared according to the life cycle phases (Cao & Folan, Citation2012) they consider. Most methods focus on the production phase, but some assess the complete life cycle including phases like product development, recycling or use phase. Since the result of the product development determines the needed raw material in production, the examined methods are considered to address the product development phase if they consider the needed raw material. Another benefit category is the considered resource. The term is meant in the sense of natural resources (VDI, Citation2016), this includes mainly energy (electrical and thermal) and materials (raw material, but also operating materials) in production sites. The results of the methods can be quantified in various ways, therefore, different quantification units are further categories. Besides the energetic and mass-related units, also CO2-equivalents or other environmental impact indicators like fossil depletion or human toxicity can be considered. In general, the possibility to quantify resource consumption can be a highly relevant criterion, since after the detection of hotspots with the methods, measures to improve resource efficiency at these hotspots need to be found and quantified regarding their potential. Hotspot analysis methods that already include measurements and quantification of the current state in a certain unit provide a benefit at this point since that is not needed afterwards. Another benefit category is the consideration of financial aspects, meaning if costs are also directly analysed with the method.
3.3. Definition of scale division
After the criteria are determined, the scale division needs to be defined to perform the rating of the methods. Therefore, each method was applied in the ETA Learning Factory at TU Darmstadt (Citation2016). The production line in the learning factory, producing a gear box, consists of five process steps including a turning machine, heat treatment, transportation by robots as well as a mounting station. Furthermore, the supply systems such as the compressors for the compressed air are also considered. In total, nine measurement points for electricity and three for compressed air were identified. To get the rating of each method in the categories, the methods were applied to the production line and all efforts needed to apply the methods well as benefits for the respective methods were noted.
The results regarding the benefits depend on the methods themselves, whereas the results in the effort criteria additionally can vary in other production lines. To include possible changes in the rating of the methods due to prerequisites that might be fulfilled in other production sites or learning factories, alternatives can occur. In the following, the assumed default system as well as the alternatives in all categories are described and the respective changes in the rating results are explained. Based on the results, a tool was developed, in which the alternatives are covered by a questionnaire given to the learning factory operators before, to identify the relevant prerequisites and chose the suitable alternative(s) varying from the default set-up.
3.3.1. Time effort for execution
In the default scenario, it is assumed that the sensors that are needed for some methods are not installed. For the ETA learning factory, that meant an additional time effort of approximately 0.5–1 month, depending on the number of sensors needed (only electricity or additionally also sensors for compressed air). In this time frame, the selection, ordering, instalment, and implementation of the sensors in an appropriate monitoring system are considered. In case sensors are implemented already, the time needed for execution reduces accordingly for the methods where measurements are required.
Alternatives occur for LCA (− 1 month), CF (− 1 month), MFCA (−1 month), EVSM (- 0,5 months, only electricity measurements), CED (- 0,5 months, only electricity measurements), CMD (− 1 month) if sensors are already installed.
3.3.2. Time effort for initial skill adoption training
In this category, the existing competencies of the target group can have a major impact on the rating results. For the default scenario in the ETA learning factory, the target group was set as employees from the industry, familiar with production but not necessarily with resource efficiency. As mentioned in , the EVSM is based on the VSM, which is why participants already familiar with the normal VSM will need less time to adopt the competencies needed for the EVSM. In the default scenario, the target group is not familiar with the VSM, thus needing approximately 2 days to learn the EVSM. Another point is the competencies needed to perform resource measurements. If the target group does not have these competencies but would be responsible for the measurements themselves, an additional day is assumed to teach them.
Alternatives occur for LCA, CF, MFCA, EVSM, CED and CMD (each+1 day) to teach competencies regarding resource measurement, and for EVSM (− 1 day) if VSM is already known.
3.3.3. Costs for data acquisition
Similar to the time effort for execution, the default scenario for the data acquisition costs is also set to be with no sensors installed. The price to implement sensors surely varies depending on the vendor and the number of needed sensors. Next to the sensors themselves, additional equipment such as bus couplers and measuring boards might be needed to connect the sensor data with the respective monitoring system. The personal costs for employees installing the sensors are not considered. Two alternative scenarios are set in the rating system next to the default one without any installed sensors. In the first, energy measurement equipment is already installed, but other resources are not measured with sensors. In the second alternative scenario, all needed sensors are already installed; hence, no additional costs are needed to get the needed data for these sensor-based methods. For thermal energy diagnosis, a thermal imaging camera is needed – if available already, costs here are set to zero as well, respectively.
Alternatives occur for LCA, CF, MFCA, EVSM, TED, CED and CMD (costs are reduced or set to zero) if equipment for measurements is already installed/available.
3.3.4. Costs for evaluation and visualisation
Depending on the method, software might be needed to perform the calculations and quantification of resource hotspots. In the case of the ETA learning factory, this is the case for the LCA, the CF, and the MFCA, where the program Umberto Efficiency+ (Institut für Umweltinformatik, Citation2022) is used. Furthermore, the LCIA database ecoinvent (full license for LCA and license with only GWP factors for CF) (ecoinvent, 2021) is used to get the respective impact factors for the LCA and the CF. The costs assumed for the rating are based on offers gathered in 2021. For both, the calculation as well as the database, tools free of charge are available, that might not have the same functional range or are more complex to operate but can be used as well. In this case, there do not occur any costs for the evaluation and visualisation.
Alternatives occur for LCA, CF and MFCA (costs are reduced or set to zero) if a calculation and visualisation tool is available, and for LCA and CF (costs are reduced or set to zero) if an LCIA database is available for the needed resources.
Despite the mentioned alternatives, it is assumed that the relation between the effort of the different methods per category stays the same regarding other size settings in other production sites. No matter if the examined production site is smaller or bigger than the production site studied here, the LCA, for example, will always need a higher time effort for execution than the ABC analysis due to the needed measurements and higher amount of data needed. The collected results and the respective alternatives for the methods applied to the ETA learning factory are depicted in . The gathered information in can be seen as an orientation to get the relation between the methods. Harvey balls instead of fixed numbers are used in the later derived rating system to focus on this relation. Since low efforts are considered positive, the highest value for each effort category is given zero points, respectively, an empty circle (○) and the lowest value one point, respectively, a full-filled circle (●). For the benefit categories, it is the opposite since high values are considered positive here. The range in between was distributed equally in all categories. This linear distribution can be changed to a logarithmic one if it proves to be more suitable for certain categories in future applications. It is assumed to be the case for the costs for evaluation and visualisation, where the range is quite high between the lowest and the highest value. The results for the scale division can be seen in .
Table 3. Matrix of efforts and benefits for each method, applied to the ETA Learning Factory at TU Darmstadt (in case of alternatives, default scenario is marked bold).
Table 4. Resulting scale division for each rating criterion.
3.4. Development of weighting scale
Since the importance of the different rating criteria presented in section 3.2 can vary depending on the target group, a manually adaptable weighting is included in the rating system. The weighting should be discussed with different stakeholders of the learning factory to become clear about the goal and identify conflicting requirements regarding the hotspot analysis methods. A possible option to derive the weighting systematically is the paired comparison (David, Citation1963). There, each criterion is compared with the others and rated if more relevant (indicated with 2), equally relevant (indicated with 1) or less relevant (indicated with 0). The resulting numbers are summed up for each criterion and can also be normalized by dividing through the overall sum to get the final weighting factors. In , an example of the paired comparison adapted to the presented rating criteria is presented. In this example, the stakeholders said, for example, that it is more relevant for the target group to have lower costs in data acquisition than to consider other life cycle phases besides the production – hence the numbers in .
Figure 4. Examples of different options to get the weighting scale: paired comparison (A) and direct numbers (B).
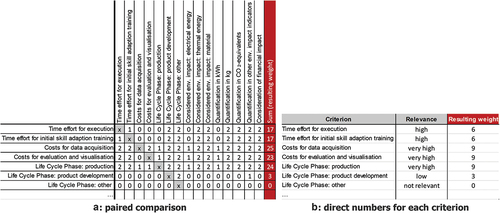
Another option to get the weighting is by directly assigning a number for each criterion. In , an example of this option is presented, choosing the number zero for ‘not relevant’, up to the number nine for ‘highly relevant’. Since the final score is normalized with the overall sum, it does not matter what scale (for example, from zero to nine or from zero to 100) is used for the weighting factors, since the share and therefore the influence of the criterion will stay the same.
3.5. Rating of hotspot analysis methods
Using the defined scale division and the results conducted in the ETA learning factory, the final rating of the considered hotspot analysis methods can be derived. To conclude the rating of the different criteria, the single points per criterion (see ) is summed up and normalized for each method. Since the effort is rated only in four criteria, whereas the benefit is rated in 11 criteria, the points are first summed up and normalized separately for effort and benefit, respectively. As mentioned in chapter 3.2, the multidimensional rating was chosen to consider the different advantages and disadvantages of the methods. Assuming that all criteria are equally important for a user in the default state, the weighting factors are set to 1 for all criteria. The weighting factors can be easily changed since they are part of the questionnaire filled out before the rating is done (see chapter 5). The derived rating and the scores in the different criteria should be considered as a basis for further discussions about the final choice, narrowing the field of suitable methods, instead of presenting one method as the only perfect one to be implemented.
4. Deriving target competencies and requirements for the learning environment
Based on the chosen hotspot analysis method, the resulting intended competencies can be derived with the competence transformation based on Tisch et al. (Tisch et al., Citation2013). This was done based on a detailed description of the methods in expert discussions. The derived competencies were further divided into sub-competencies and formulated with the taxonomy given by Anderson et al. (Citation2001). Competency matrices were developed for each described hotspot analysis method accordingly. Following the approach previously presented by Weyand, Schmitt, et al. (Citation2021), respective technical requirements were formulated in these matrices for each sub-competence. Examples of these matrices are depicted in .
Table 5. Extract of the competency matrices including respective requirements, developed for each hotspot analysis method.
5. Approach for learning factory operators
To apply the previously described aspects to implement suitable resource efficiency methods in learning factories, the approach depicted in is developed.
First, a questionnaire is given to learning factory operators to examine different prerequisites such as if the VSM is already known to the target group or if sensors are already implemented. As described in section 3.3, these aspects influence the rating of the method. The same accounts for the weighting factors that are also ascertained in the questionnaire. Therefore, learning factory operators are asked to rate the different criteria according to relevance to perform a direct rating or a paired comparison to get the weighting factors (see ). These two aspects, the determination of weighting factors and the adjustment of rating based on the prerequisites are then used to get the rating results and a suggestion for the best suitable hotspot analysis methods for the respective learning factory. Based on the choices made then by the learning factory operators, the respective intended competencies as well as the resulting requirements for the chosen hotspot analysis method(s) can be deduced from the lists described in section 4. These lists can be discussed and adjusted in stakeholder sessions to make sure all necessary requirements are listed. When this list is derived, steps 4 and 5 of the process to implement new topics in learning factories can be approached (see ).
To ease the application of the approach presented, a software tool was developed for the rating of hotspot analysis methods in learning factories. The tool is extended with a detailed description of each method as well as the questionnaire, helping users to identify the weighting factors and prerequisites best representing their individual requirements.
6. Application in learning factories
The tool mentioned in section 5 was applied at a learning factory not dealing with resource efficiency yet, namely the CiP learning factory at TU Darmstadt (PTW TU Darmstadt, Citation2022). First, the most important criteria for the learning factory operators were analysed and the respective weighting factors were determined. The scale for the weighting factors was set according to the direct rating presented in from 0 to 9. Since the CiP did not have any equipment or learning modules for resource efficiency yet, the representatives decided to start with the consideration of energy in production first. This resulted in the weighting factor 0 for the other life cycle phases besides production as well as for other resources, meaning these possible advantages were not relevant and therefore not considered. The consideration of electrical energy was ranked highly relevant, whereas thermal energy was ranked as neglectable in the first round due to no heat treatment in the CiP production line. The quantification in CO2-equivalents or other environmental impact indicators was also considered neglectable for the start, the exception being the quantification in kWh, which was considered highly relevant. Since the CiP partners visiting the learning factory are partly small and medium-sized companies with limited time and budgets, the effort criteria were considered very important, therefore weighted with factor 9. However, the criterion of considered financial impact was decided to be of low importance for a workshop focusing on resource efficiency and therefore weighted by a factor of 3. As prerequisites, it was noted that there were no sensors for resource consumption installed so far, making it necessary to take these additional efforts into account. However, since the target group was not considered to be responsible for the instalment of sensors, the time needed to teach competencies related to this topic was not considered. Furthermore, the CiP learning factory, originally focused on lean management topics, mostly welcomed workshop participants already familiar with the VSM – reducing the potential time to understand the EVSM. Based on these prerequisites and the weighting factors, the rating was performed and the results can be seen in .
Figure 6. Rating of the methods with adjusted weighting according to the identified requirements and prerequisites in the CiP Learning Factory.
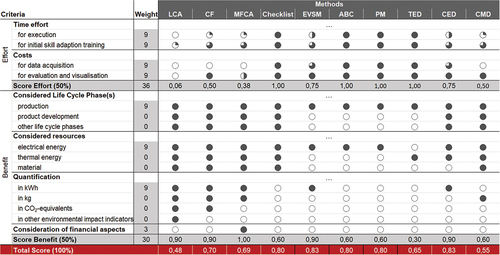
For this case, the EVSM as well as the CED with 0.83 points each got the highest scoring, followed by the Checklist, the ABC analysis and the PM with 0.80 points. The scoring was used as a basis for further discussion, resulting in the learning factory operators choosing the EVSM as the method to be implemented. Besides its very high score, the operators also valued the advantages of the EVSM in the approach familiar to their target group since similar to the VSM. Furthermore, discussions are ongoing to also implement one of the methods with a low teaching effort, such as the ABC or PM, since they might be an easy addition to address small companies with no budgets for energy measurements.
7. Discussion, conclusion, and outlook
In this paper, a systematic approach to implement resource efficiency methods in learning factories is presented. The core of the concept is a comparison approach regarding hotspot analysis methods in the context of resource efficiency. It is based on a rating system with various criteria, considering benefits as well as related efforts regarding the analysed methods. A study was done to actually quantify the benefits of the methods in relation to the number of measures found based on the results of the methods. It was found that the number and type of measures derived are highly dependent on the production line and the results cannot be generalized. Therefore, criteria were defined that raise the chance of finding the most suitable measures, like the number of considered resources. Nevertheless, it cannot be said that, just because a method only considers electrical energy or is based on type plates instead of measurements, the measures derived are less effective in general. The rating system can be used by learning factory operators as a starting point to find suitable hotspot analysis methods for their target groups. The developed tool further allows the adjustment of the rating depending on prerequisites and weighting factors. This can be necessary if, for example, sensors are already implemented in a learning factory and therefore the effort of data acquisition potentially decreases. The tool can also be used by industry if the weighting would be adjusted, respectively, according to the requirements. Nevertheless, the rating could be changed for industrial cases since it is, in some cases, dependent on the competencies available in a company. That is the case, for example, for the category of time effort for initial skill adoption training – if competencies for certain methods are already available in a company, this time effort will reduce as far as to zero. To consider the existing competencies, a category or part in the questionnaire could be included for industrial cases.
So far, the tool has to be adjusted manually. An automated adjustment based on the answers to the questionnaire in the tool could be implemented prospectively. Based on the chosen hotspot analysis method, an approach to create a learning module about resource efficiency for a learning factory was developed. This includes the determination of intended competencies and, respectively, needed technical adjustments in the learning factory infrastructure. Further research needs to be done to combine the implemented hotspot analysis method with the process of improvement measure identification.
Acknowledgments
The authors are grateful to the EU and the state Hessen for funding the basis of this work in the Reoptify project as well as to the Deutsche Bundesstiftung Umwelt for funding the further extension of the approach and tool development in the project Kompetenzen für die Klimaneutrale Produktion.
Disclosure statement
No potential conflict of interest was reported by the authors.
Additional information
Funding
References
- Abele, E. (2016). Learning factory. In L. Laperrière & G. Reinhart (Eds.), The internationalInternational Academy for production engineering (Vol. 32, pp. 1–20). Springer. https://doi.org/10.1007/978-3-642-35950-7_16828-1
- Abualfaraa, W., Salonitis, K., Al-Ashaab, A., & Ala’raj, M. (2020). Lean-green manufacturing practices and their link with sustainability: A critical review. Sustainability, 12(3), 981. https://doi.org/10.3390/su12030981
- Anderson, L. W., Krathwohl, D. R., & Airasian, P. W. (Eds.). (2001). A taxonomy for learning, teaching, and assessing: A revision of bloom’s taxonomy of educational objectives (Complete ed.). Longman.
- Bergmann, A., Günther, E., & Kara, S. (2017). Resource efficiency and an integral framework for performance measurement. Sustainable Development, 25(2), 150–165. https://doi.org/10.1002/sd.1669
- Böhner, J., Scholz, M., Franke, J., & Sauer, A. (2018). Integrating digitization technologies into resource efficiency driven industrial learning environments. Procedia Manufacturing, 23, 39–44. https://doi.org/10.1016/j.promfg.2018.03.158
- Cao, H., & Folan, P. (2012). Product life cycle: The evolution of a paradigm and literature review from 1950–2009. Production Planning & Control, 23(8), 641–662. https://doi.org/10.1080/09537287.2011.577460
- David, H. A. (1963) The method of paired comparisons Kendall, M. G. In Griffin’s statistical monographs and courses. 2. ed rev Vol. 41 Oxford Univ. Press; Griffin 1–124.
- Deutsches Institut für Normung e.V. (DIN). (2011). Environmental management – material flow cost accounting – general framework. Beuth Verlag GmbH. DIN EN ISO 14051.
- Deutsches Institut für Normung e.V. (DIN). (2019a-). 02 Treibhausgase - Carbon Footprint von Produkten - Anforderungen an und Leitlinien für Quantifizierung DIN EN ISO 14067Beuth Verlag GmbH https://www.beuth.de/de/norm/din-en-iso-14067/289443505.
- Deutsches Institut für Normung e.V. (DIN). (2019b-). 06 Treibhausgase - Teil 1: Spezifikation mit Anleitung zur quantitativen Bestimmung und Berichterstattung von Treibhausgasemissionen und Entzug von Treibhausgasen auf Organisationsebene DIN EN ISO 14064-1Beuth Verlag GmbH https://www.beuth.de/de/norm/din-en-iso-14064-1/291289049.
- Deutsches Institut für Normung e.V. (DIN). (2021a). Umweltmanagement – Ökobilanz – Anforderungen und Anleitungen. Beuth Verlag. DIN EN ISO 14044.
- Deutsches Institut für Normung e.V. (DIN). (2021b). Umweltmanagement – Ökobilanz – Grundsätze und Rahmenbedingungen. Beuth Verlag. DIN EN ISO 14040.
- Doch, S., Merker, S., GmbH Berlin, I. T. C. L., Straube, F., & Roy, D. (2015). Aufbau und Umsetzung einer Lernfabrik : Produktionsnahe Lean-Weiterbildung in der Prozess- und Pharmaindustrie. Industrie 4.0 Management, 26–30.ecoinvent. (2021, December 12). ecoinvent Database. https://ecoinvent.org/the-ecoinvent-database/
- Enke, J. (2019). Methodik zur multidimensionalen reifegradbasierten Entwicklung von Lernfabriken für die Produktion [ Dissertation]. TU Darmstadt,
- Erlach, K. (2013). Energy value stream: Increasing energy efficiency in production. In G. Schuh, R. Neugebauer, & E. Uhlmann (Eds.), Future trends in production engineering (pp. 343–349). Springer. https://doi.org/10.1007/978-3-642-24491-9_34
- Gräßler, I., Pöhler, A., & Pottebaum, J. (2016). Creation of a learning factory for cyber physical production systems. Procedia CIRP, 54, 107–112. https://doi.org/10.1016/j.procir.2016.05.063
- Institut für Umweltinformatik. (2022). Umberto: Die Ressourceneffizienz-Software Umberto Efficiency+. https://www.ifu.com/de/umberto/oekobilanz-software/
- International Association of Learning Factories. (2021). Definition of “learning factory”. https://ialf-online.net
- Karre, H., Hammer, M., Kleindienst, M., & Ramsauer, C. (2017). Transition towards an industry 4.0 state of the LeanLab at Graz University of Technology. Procedia Manufacturing, 9, 206–213. https://doi.org/10.1016/j.promfg.2017.04.006
- Katunsky, D., Korjenic, A., Katunska, J., Lopusniak, M., Korjenic, S., & Doroudiani, S. (2013). Analysis of thermal energy demand and saving in industrial buildings: A case study in Slovakia. Building and Environment, 67, 138–146. https://doi.org/10.1016/j.buildenv.2013.05.014
- Kishita, Y., Low, B. H., Fukushige, S., Umeda, Y., Suzuki, A., & Kawabe, T. (2010). Checklist-based assessment methodology for sustainable design. Journal of Mechanical Design, 132(9). Article 091011 https://doi.org/10.1115/1.4002130.
- Kreß, A., Wuchterl, S., & Metternich, J. (2022). Design approaches for learning factories – review and evaluation. Social Science Research Network Electronic Journal. https://doi.org/10.2139/ssrn.3857880
- Li, W., Thiede, S., Kara, S., & Herrmann, C. (2017). A generic sankey tool for evaluating energy value stream in manufacturing systems. Procedia CIRP, 61, 475–480. https://doi.org/10.1016/j.procir.2016.11.174
- Plorin, D., Jentsch, D., Hopf, H., & Müller, E. (2015). Advanced Learning Factory (aLF) – Method, Implementation and Evaluation. Procedia CIRP, 32, 13–18. https://doi.org/10.1016/j.procir.2015.02.115
- Posselt, G. (2016). Towards Energy Transparent Factories. Springer International Publishing. https://doi.org/10.1007/978-3-319-20869-5
- PTW TU Darmstadt. (2022). Prozesslernfabrik CiP - Center für industrielle Produktivität. https://www.prozesslernfabrik.de/
- Riemann, T., & Metternich, J. (2022). Virtual reality supported trainings for lean education: Conceptualization, design and evaluation of competency-oriented teaching-learning environments. International Journal of Lean Six Sigma. Advance online publication. https://doi.org/10.1108/IJLSS-04-2022-0095
- Ritchie, H., & Roser, M. (2020). CO₂ and Greenhouse Gas Emissions. https://ourworldindata.org/emissions-by-sector
- Schmidt, C., Li, W., Thiede, S., Kara, S., & Herrmann, C. (2015). A methodology for customized prediction of energy consumption in manufacturing industries. International Journal of Precision Engineering and Manufacturing-Green Technology, 2(2), 163–172. https://doi.org/10.1007/s40684-015-0021-z
- Schmidt, M., & Nakajima, M. (2013). Material flow cost accounting as an approach to improve resource efficiency in manufacturing companies. Resources, 2(3), 358–369. https://doi.org/10.3390/resources2030358
- Schuhmacher, J., & Hummel, V. (2016). Decentralized control of logistic processes in cyber-physical production systems at the example of esb logistics learning factory. Procedia CIRP, 54, 19–24. https://doi.org/10.1016/j.procir.2016.04.095
- Scriven, M. (2000). The logic and methodology of checklists. Western Michigan University.
- Smith, B. (2004). The greenhouse gas protocol: A corporate accounting and reporting standard (Rev. ed.). World Resources Institute; World Business Council for Sustainable Development.
- Thiede, S. (2012). Energy efficiency in manufacturing systems. In Sustainable Production, life cycle engineering and management ser (1st ed.). Springer Berlin/Heidelberg. https://ebookcentral.proquest.com/lib/kxp/detail.action?docID=973962
- Thiede, S., Juraschek, M., & Herrmann, C. (2016). Implementing cyber-physical production systems in learning factories. Procedia CIRP, 54, 7–12. https://doi.org/10.1016/j.procir.2016.04.098
- Thiede, B., Mindt, N., Mennenga, M., & Herrmann, C. (2022). Creating a hybrid multi-user learning experience by enhancing learning factories using interactive 3d-environments. Social Science Research Network Electronic Journal. Advance online publication. https://doi.org/10.2139/ssrn.4074712
- Tisch, M., Hertle, C., Abele, E., Metternich, J., & Tenberg, R. (2015). Learning factory design: A competency-oriented approach integrating three design levels. International Journal of Computer Integrated Manufacturing, 29(12), 1355–1375. https://doi.org/10.1080/0951192X.2015.1033017
- Tisch, M., Hertle, C., Cachay, J., Abele, E., Metternich, J., & Tenberg, R. (2013). A Systematic Approach on Developing Action-oriented, Competency-based Learning Factories. Procedia CIRP, 7, 580–585. https://doi.org/10.1016/j.procir.2013.06.036
- Tisch, M., & Metternich, J. (2017). Potentials and Limits of Learning Factories in Research, Innovation Transfer, Education, and Training. Procedia Manufacturing, 9, 89–96. https://doi.org/10.1016/j.promfg.2017.04.027
- Umweltbundesamt (Ed.). (2001) . Handbuch Umweltcontrolling. Erw. Auflage). Vahlen.
- VDI Zentrum Ressourceneffizienz. (2021, October 26). Instrumente VDI 4801. https://www.ressource-deutschland.de/instrumente/instrumente-vdi-4801/
- Verein Deutscher Ingenieure (VDI). (2012). VDI 4600 - Cumulative energy demand (KEA): Terms, definitions, methods of calculation. Beuth Verlag GmbH.
- Verein Deutscher Ingenieure (VDI). 02. 2016 VDI 4800 Part 1 - Resource efficiency: Methodological principles and strategies (VDI 4800 Blatt 1) Beuth Verlag GmbH
- Verein Deutscher Ingenieure (VDI). (2018). VDI 4800 - Resource efficiency: Evaluation of raw material demand. Beuth Verlag GmbH.
- Weyand, A., Rommel, C., Zeulner, J., Sossenheimer, J., Weigold, M., & Abele, E. (2021). Method to increase resource efficiency in production with the use of MFCA. Procedia CIRP, 98, 264–269. https://doi.org/10.1016/j.procir.2021.01.101
- Weyand, A., Schmitt, S., Petruschke, L., Elserafi, G., & Weigold, M. (2021). Approach for Implementing New Topics in Learning Factories – Application of Product-specific Carbon Footprint Analysis. SSRN Journal (SSRN Electronic Journal), Proceedings of the Conference on Learning Factories (CLF). Advance online publication. https://doi.org/10.2139/ssrn.3863447
- Weyand, A., Thiede, S., Mangers, J., Plapper, P., Ketenci, A., Wolf, M., Panagiotopoulou, V. C., Stavropoulos, P., Köppe, G., Gries, T., & Weigold, M. (2022). Sustainability and Circular Economy in Learning Factories – Case Studies. Social Science Research Network Electronic Journal. Advance online publication. https://doi.org/10.2139/ssrn.4080162
- Wiedmann, T., & Minx, J. C. (2008). A Definition of ‘Carbon Footprint' Pertsova, C. C. In C. C. Pertsova, Ecological Economics Research Trends: Chapter 1 (pp. 1–11). Nova Science Publishers.