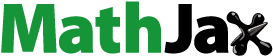
Abstract
We examined how both horizontal and vertical aspects of land-cover diversity influence patterns of avian species-richness across North America. Using count data from Breeding Bird Survey routes within the conterminous USA and land-cover data from the National Land Cover Data-set, we analyzed relationships between species-richness estimates, vegetative strata, landscape diversity and elevation and geographic position using both linear-regression models and a classification and regression tree. We found that latitude, the diversity of land-cover classes present, and the proportion of the landscape containing cover-classes representing 3 vegetative strata had the strongest influence on species richness. This illustrates that, while broad-scale biodiversity trends are strongly influenced by dominant regional factors, they are also sensitive to the structure of the intermediate-level landscape. Thus, factors at multiple scales must be considered when modeling spatial patterns of biodiversity such as avian species-richness.
Public Interest Statement
Explaining the complex patterns of biodiversity at different spatial scales remains a significant challenge for scientists, while the growing numbers of species eliminated or adversely affected by human activities makes the task increasingly urgent. We compared levels of bird diversity estimated from the National Breeding Bird Survey in 1992 to land-cover and geographic factors to determine how useful landscape-level information is to inferring habitat-level attributes, and how important these factors are when compared to regional-level factors across the contiguous USA. Although the regional-level factors explained much of the variations in the diversity of bird species across the USA, the more local-level factors of landscape diversity and habitat complexity were also important, meaning that factors at all levels need to be integrated to satisfactorily explain large-scale patterns of species diversity. Thus, efforts to model biodiversity patterns for conservation or to predict the effects of environmental changes must consider factors at multiple scales.
1. Introduction
The spatial variation in biological communities is a major research topic in applied geography, landscape ecology, biogeography, and community ecology (Costanza, Moody, & Peet, Citation2011; Elton, Citation2001; de Roos & Sabelis, Citation1995; Wilson, Citation2000), and spatial variation is believed to be as important as temporal variation to the regulation of ecosystem processes (Ma, Zuckerberg, Porter, & Zhang, Citation2012; Turner & Cardille, Citation2007). Knowledge of the spatial variability of biodiversity is also fundamental to the identification of priority areas for conservation interventions, such as the designation and delineation of “protected areas” (Carroll, Noss, Paquet, & Schumaker, Citation2003; del Carmen Sabatini, Verdiell, Rodríguez Iglesias, & Vidal, Citation2007; Reid, Citation1998; Soulé, Citation1991), the identification of “gaps” in existing conservation arrangements (McKendry & Machlis, Citation1993), and in shifting the focus in conservation from the preservation of individual species and habitats to that of maintaining functional ecosystems (Frost, Campbell, Medina, & Usongo, Citation2006; Naveh, Citation1994; Wilson, Citation2002). Over the last 10,000 years, humans have emerged as a dominant force driving the evolution of terrestrial landscapes, with the rate of transformation increasing dramatically in the last half-century (Foley et al., Citation2005; Turner, Gardner, & O’Neill, Citation2001). This widespread and complex transformation of terrestrial landscapes—particularly rapid in the cases of agricultural expansion and urbanization (Pijanowski & Robinson, Citation2011; Seto, Güneralp, & Hutyra, Citation2012; Tilman et al., Citation2001)—is believed to be one of the most significant factors contributing to contemporary, accelerated rates of biodiversity loss (Sala et al., Citation2000; UNCBD, Citation2010), but biodiversity forecasts focused on the anticipated effects of climate change outnumber those focused projected land-change scenarios (Titeux et al., Citation2016). Given the dominant role humans now play in shaping the planet’s terrestrial landscapes and the immediate and profound implications of land change for biodiversity, a more thorough understanding of how local landscape features influence regional biodiversity patterns should be an important research priority (Sala et al., Citation2000; USNRC, Citation2001).
1.1. Avian species-richness as a measure of biodiversity
Avian species-richness, typically considered an α-level variable in the traditional biodiversity hierarchy (MacArthur, Citation2004; Whittaker, Citation1972; Whittaker, Willis, & Field, Citation2001), is commonly used in studies of biodiversity patterns over macro-scales (Gaston & Williams, Citation1993) because it tracks well with other dominant biodiversity factors at these scales (Gaston & Blackburn, Citation1995). However, it does not capture sub-species variations—which may be declining at a rate three orders of magnitude greater than that of species extinctions (Rands et al., Citation2010)—and is neutral in regards to species composition and attributes (e.g. evenness, rarity, endemism) that may be important in other studies (e.g. trophic structures, sink-source delineation, predator-prey models) and to conservation and management (Storch, Marquet, & Brown, Citation2007; Wilson, Citation2000). The advantage to this neutrality, however, is that species-richness estimates tend to be more robust and readily corrected for differences in detection probabilities, and therefore more accurately reflect one aspect of biodiversity at larger scales and in sampling situations—such as the North American Breeding Bird Survey (BBS)—where the requisite corrections for detection and sampling bias (i.e. each species, each route, each observer) would pose significant logistic difficulties (Gaston & Spicer, Citation1998; Ibáñez et al., Citation2006). Thus, species richness is best considered as a widely used, basic indicator of biodiversity that is, however, necessarily incomplete (Lévêque & Mounolou, Citation2003).
More generally, the dominant drivers of species richness tend to be highly scale-dependent (Böhning-Gaese, Citation1997). Studies of species richness at or near the habitat level tend to identify factors related to habitat complexity, resource quality, and community interactions as dominant drivers (Goetz, Sun, Zolkos, Hansen, & Dubayah, Citation2014; Harris, Milligan, & Fewless, Citation1983; Karr & Roth, Citation1971), although which variables best reflect these factors tends to vary with the type of habitat considered, and different groups of species tend to respond differently to each variable (e.g. Coppedge, Engle, Masters, & Gregory, Citation2001; Donovan & Flather, Citation2002; Flather & Sauer, Citation1996). Explanations of regional patterns of species richness tend to polarize into positions maintaining that either such patterns can ultimately be explained by one (i.e. climate) or no more than a few variables, or that the ensemble of determinant variables depends on the region considered and must be established empirically (Currie, Citation2007).
1.2. Hypothesized effects of horizontal landscape diversity and vertical stratification on avian species-richness
Rather than mutually exclusive categories, these two positions can be reconciled by positing that both habitat-level and regional-level explanations provide important insights into broad-scale biodiversity patterns, and cross-scale integration of such variables could be valuable to modeling and regional analysis (Brown & Sax, Citation2004; Storch et al., Citation2007). Therefore, we chose to focus on landscape diversity (as indicated by a Shannon index of the number of different land-cover classes within 5 km of each BBS route) and vertical stratification (as indicated by the proportion of land-cover classes reflecting differing numbers of vegetative strata) as landscape- or meso-level variables, but to use them to infer general information about habitat-level diversity, and to use geographic coordinates and topography (mean elevation and standard deviation) as surrogates to regional-level variables to determine whether and how landscape variables influence regional species-richness trends alongside stronger, regional-level climatic and topographic influences. We hypothesized that, insofar as landscape diversity and vertical stratification reflect increased habitat diversity within the landscape, higher values of either variable will generally correspond to higher levels of species richness. We also stipulated, however, that the exact form of this relationship may be non-linear, as landscape-diversity values beyond a given threshold may begin to reflect habitat fragmentation rather than richness, while a similar threshold for any one strata class may conversely indicate a lower degree of overall landscape diversity.
MacArthur (Citation1964) demonstrates that vertical habitat features are more important where overall land-cover diversity is low, as the presence of multiple patches of different land-cover types introduces a corresponding number of different vertical profiles into the landscape. Accordingly, we hypothesized that a model including both horizontal land-cover diversity and vertical stratification (i.e. vegetation class) would indicate that land-cover diversity is more important than strata class where the value of the former is high, and that strata class would become more important where land-cover diversity is low.
Attempts to characterize the relative diversity of a landscape commonly employ metrics derived from information theory such as diversity or richness indices, but frequently with a focus on horizontal heterogeneity that does not reflect potentially important heterogeneity in the vertical plane (Farina, Citation1998; Turner et al., Citation2001). At finer scales, a series of recent studies explicitly include vertical habitat structure through the use of LiDAR (e.g. Davies & Asner, Citation2014; Pekin & Pijanowski, Citation2012; Vierling, Vierling, Gould, Martinuzzi, & Clawges, Citation2008), but the 0.5–3 m resolution of LiDAR data (Storch et al., Citation2007) reflects a much finer scale representation than land-cover data derived from 30 m resolution imagery, and LiDAR coverage is sparse compared to land-cover data (Goetz et al., Citation2014). This study is original in that it uses land-cover variables to indirectly infer habitat-level factors and geographic position and topography to indirectly represent regional factors, and then compares the influence of both sets of factors on species-richness estimates sampled across the conterminous USA.
2. Data
Data were synthesized from three complementary national data-sets: (1) avian species-richness estimates from BBS routes sampled across the conterminous USA, (2) elevation data from the United States National Aeronautics and Space Administration (NASA) Shuttle Radar Topographic Mission’s (SRTM) 90-meter Digital Elevation Model (DEM) and (3) land-cover characteristics from the 1992 National Land-Cover Data-set (NLCD) [The data-sets used in the statistical analyses are available in the supplementary material].
2.1. BBS sample data
The BBS is a macro-scale, long-term inventory of avian species trends, and is one of the longest-running species surveys at the subcontinental scale. Started in 1966 by researchers at the USGS’s Patuxent Wildlife Research Center, it is used to monitor species trends throughout the conterminous USA and includes 3,000 survey routes that are sampled annually during the spring/summer breeding season. Survey routes are pseudo-randomly situated within one-degree blocks of latitude and longitude along roadways, and each survey route is 40 km (24.5 miles) long. The observer stops every 0.8 km (0.5 miles) along the route for three minutes and records the number and species of every bird seen or heard within 0.4 km.
Beyond the above-mentioned pseudo-random situation, BBS routes are not distributed evenly or randomly throughout the USA, and tend to over-represent deciduous forests in the north-eastern region and under represent higher elevations in drier regions (Lawler & O’Connor, Citation2004). Although a higher number of survey routes in a particular region may increase the likelihood of a given rare species being detected along any one of the survey routes, this should not influence measures of species richness made along each route, as each route is sampled—and species-richness estimates are calculated—independently. The fact that survey routes are situated along roads may have a significant effect on species-richness estimates, as proximity to road has been shown to influence the species richness of vegetation (Marcantonio, Rocchini, Geri, Bacaro, & Amici, Citation2013), habitat composition and change (Harris & Haskell, Citation2007), and composition of avian communities (Wellicome, Kardynal, Franken, & Gillies, Citation2014). The risk of these factors introducing systematic biases in this study, however, was minimized insofar as their effects should be randomly distributed across all survey routes.
Observer skill presents another potential bias in the survey: although volunteers undergo training prior to participating in the survey, accumulated life experience introduces a potentially significant detection bias that is difficult to account for Gu and Swihart (Citation2004), Link and Sauer (Citation1998) and Urban and Swihart (Citation2009). To some extent, such bias is unavoidable in any data-set based on long-term volunteer surveys, and BBS organizers account for observer experience when examining population trends over time (Sauer, Peterjohn, & Link, Citation1994).
We attempted to further mitigate the possibility of this bias in two ways: (1) by estimating species-richness values from only one survey-year, we minimized within-observer effects (Kendall, Peterjohn, & Sauer, Citation1996), and (2) using species richness rather than population factors, species misidentification—insofar as it did not change the number of species detected—posed less of a threat to the validity of the results.
Survey results from 1966 to present are publicly available from the USGS. To optimize computational resources, we used data aggregated into 10-stop summaries of the 2,087 routes that were sampled in 1992 and situated within the portion of the conterminous US covered by the NLCD to estimate route-level species-richness in this study.
2.2. SRTM DEM
BBS meta-data specifies latitude and longitude coordinates for each survey route, but does not mention elevation or topography. To include these factors, we integrated data from the NASA SRTM’s global, 90 m DEMs (Jarvis, Reuter, Nelson, & Guevara, Citation2008). We were primarily interested in the overall altitude of each route, which we estimated by calculating the mean elevation value for all cells within a 5 km buffer (see Section 3.2) around each survey route. We also calculated the standard deviation within each route-buffer, which we included in the statistical model as an indicator of the sample area’s topographic heterogeneity (Rahbek et al., Citation2007).
The use of latitude, longitude, and mean elevation as moderate-scale surrogates to more specific climatic and other regional variables (solar radiation, precipitation, temperature, distance to surface water, etc. HilleRisLambers, Harsch, Ettinger, Ford, and Theobald (Citation2013) was based on previous studies that use these factors to account for climatic variability (e.g. Russell, Domke, Woodall, & D’Amato, Citation2015; Rweyongeza, Barnhardt, & Hansen, Citation2011; Shore, Safranyik, & Lemieux, Citation2000). This use of geographic variables as indirect indicators of climatic factors allowed us to capture the effects of the effects of climatic variability, including the longitudinal moisture/climatic gradient across the conterminous USA (Gaston & Spicer, Citation1998; Whittaker, Citation1975), while avoiding the dilemma of either inflating the potential error of the statistical models by including all necessary climatic variables to which different avian species are sensitive (in sensu Jetz, Wilcove, & Dobson, Citation2007) or excluding biologically important variables from the model.
2.3. 1992 NLCD
The 1992 NLCD, also developed by the USGS, is one of the earliest and most widely used land-cover data-sets for the USA (USEPA, Citation2014a). We selected the 1992 NLCD over more recent data-sets because of this widespread use, and because it has been shown to provide an effective baseline against which subsequent land-change can be identified, although its accuracy is somewhat less than that of a neural-network based classification (Boyd, Foody, & Ripple, Citation2002; Kolios & Stylios, Citation2013).
The NLCD’s land-cover types are classified from Landsat Thematic Mapper imagery into 21 classes analogous to Anderson, Hardy, Roach, and Witmer (Citation1976) levels I and II (as modified by Vierling et al. (Citation2008)) at 30 m spatial resolution. Whereas minimum accuracy at level I is estimated to be 70%, level II accuracy is lower (minimum < 40%) and more widely variable (Stehman, Wickham, Smith, & Yang, Citation2003; Wickham, Stehman, Smith, & Yang, Citation2004).
Despite the higher error rate (Thogmartin, Gallant, Knutson, Fox, & Suárez, Citation2004), we used level II classes to estimate horizontal diversity with the Shannon index (Shannon, Citation1948) on the grounds that we were more interested in the number of different classes than their designations, but reverted to level I classes to assign vertical-stratification categories.
At the landscape scale, vertical habitat-structure can be generalized following MacArthur and Wilson’s (Citation1967) and Whittaker (Citation1975) characterization of vegetative stratification in different vegetation types. In this context, forests represent the most structurally complex landscapes with at least three different strata available to most bird species: (1) the upper canopy, (2) shrubs and small trees and (3) herbs and other vegetation at or near ground-level. Woodlands and similar brush habitats contain only the latter two strata, and grasslands only the last stratum. Like land-cover classification, such stratigraphic characterization may not reflect how birds actually perceive the landscape, but is a useful analytical tool to classify and compare landscapes in terms of the horizontal and vertical diversity of habitat types (MacArthur & MacArthur, Citation1961). Using the NLCD land-cover classification criteria (USEPA, Citation2014b; Vogelmann, Sohl, Campbell, & Shaw, Citation1998), we re-classified land-cover classes into one of four strata classes. Classes 1–3 refer to the number of strata associated with the land-cover classes in those categories, and class 0 contains land-cover classes with little or no vegetation present and those with an indeterminate degree of stratification (see Table ).
Table 1. Classification of land-cover classes based on landscape vegetative strata
3. Analytical methods
To test the hypothesized relationship between avian species-richness and landscape factors using diversity and vegetative strata as indirect indicators of habitat-level diversity, we focused on two principal questions in our statistical analysis: (1) Do increasing proportions of multi-strata vegetation correspond to higher levels of species richness in a manner analogous to the effects of increasing land-cover diversity? and (2) Does the importance of vegetation strata decrease as overall landscape diversity increases? To determine the importance of these landscape-level variables and the habitat-level factors they indirectly reflect at the regional scale, we asked whether their influence could be detected across the study region and remain statistically significant even when modeled against regional factors.
The outer boundary of the study region in this analysis was determined by the US national borders, but the region was otherwise open and unbounded, and species richness was treated as an emergent property of landscape diversity, vegetative strata, latitude, longitude, and mean elevation (sensu Brown, Ernest, Parody, & Haskell, Citation2001). The translation of the BBS, geographic, and land-cover data into a meaningful analysis of species richness took place in three general phases: (1) species-richness estimation, (2) derivation of spatial attributes, and (3) analysis of relationships between species richness and spatial attributes.
3.1. Species-richness estimation
The total number of species detected along each survey route was adjusted with a first-order jackknife estimator to adjust for heterogeneous detection probabilities [9]. Of the eight models reviewed by Boulinier, Nichols, Sauer, Hines, and Pollock (Citation1998), Mh—in which detection probability varies with individual species—is robust to deviations from model assumptions, performs well if the average detection probability is large enough, and is selected the most frequently as the best estimator of species richness in their study of 317 survey route-years. This is consistent with other analyses indicating that the first-order jackknife yields optimal results with BBS data (Cam, Nichols, Sauer, Hines, & Flather, Citation2000a, Citation2000b; Nichols, Boulinier, Hines, Pollock, & Sauer, Citation1998).
The first-order jackknife successively resamples each survey route based on the incidences of species occurring at only one stop, as indicated by Equation (1). The variable Ŝ represents the estimated species richness, So represents the number of species observed, α1 is the number of species observed at only one stop, and N is the number of sampling occasions. To reduce the computational load of the analysis, the USGS provides the species accounts of all 50 stops along each survey route aggregated into 10-stop summaries (i.e. the species counts of ten consecutive stops are combined into a single species account). This effectively reduces the number of sample points from 50 to 5, meaning N = 5 instead of N = 50. Because the jackknife uses instances where a given species is only detected at one sample point to calculate the detection-probability coefficient, the term is adjusted down from 0.98 to 0.8 in Equation (1):
(1)
(1)
The implication of this adjustment is that the species-richness estimates derived from the 10-stop summaries was slightly lower than those derived from the full 50-stop accounts, but the effect was common to all 2,087 routes. Because this study examined richness levels relative to one-another across all routes, rather than absolute richness levels for any particular route, estimates based on the 10-stop data do not bias the statistical analyses.
Subspecies were resolved to the 10th supplement (Chesser et al., Citation2010) to the 7th American Ornithologist’s Union check-list of bird species (AOU, Citation1998), and unidentified species were filtered from the data-set. We opted to include shorebirds, nocturnal species, and other species that are not always well-recorded in the BBS because we expected that the large number of routes included in the analysis would offset any effects that such rare sightings may have on the alpha term of the jackknife estimate. We used the “vegan” library (Oksanen, Kindt, Legendre, O’Hara, & Stevens, Citation2009) for the R Statistical Language (R Development Core Team, Citation2008) to estimate species richness independently for each route sampled in 1992 (Figure ).
Figure 1. Map of 1992 North American breeding bird survey routes color-coded by species-richness estimates, with values divided into five groups at natural breaks.
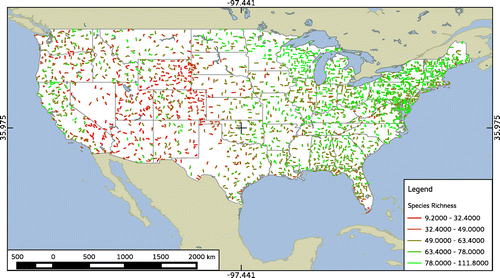
3.2. Derivation of geographic and land-cover variables
Latitude and longitude values were acquired from meta-data associated with the vector map of BBS route paths used to overlay routes onto the GTOPO30 and NLCD grids. To obtain elevation values, we projected a 5 km buffer onto the GTOPO30 DEM around each BBS route and calculated the mean elevation value within said buffer. The 5 km buffer, which was the maximum buffer size the computer system could process at the time of the analysis, was selected as a more inclusive buffer after it was determined that the results did not differ significantly to 1 km and 500 m buffers (unpublished data).
BBS routes are not straight transects, so the number of cells within each 5 km buffer is not constant. Because the NLCD does not cover Canada or Mexico, adjacency to national borders and coastlines also affects the total number of cells within each buffer. To account for these variable buffer sizes, we used the proportion of cells in each strata class rather than cell counts as the determinate variables.
3.3. Analysis of relationships between species richness and spatial attributes
Two types of regression analyses were employed to address the research questions: (1) a series of formal multiple-regression models to estimate regression coefficients and compare their explanatory power, and (2) a classification and regression tree (CART, hereafter regression tree) to identify key thresholds when explanatory variables are arranged hierarchically.
Land-cover diversity and proportions of each of the four strata classes were all calculated from the same set of land-cover values, and therefore highly collinear. In the latter case, the collinearity was so extreme that any attempt to generate a model containing all four variables simultaneously produced a singularity. Because both the predictive power of the individual variables and the signs of the regression coefficients—the two factors most strongly affected by multicollinearity (Hair, Tatham, Anderson, & Black, Citation1998)—were precisely what we were interested in determining, we separated the collinear variables into different models and generated Akaike Information Criteria (AIC; Akaike, Citation1974) scores to compare the different models’ explanatory power. To more explicitly determine whether geographic and topographic variables, serving as surrogates to regional factors, eclipsed land-cover factors at our study scale, we generated a model containing only geographic variables (latitude, longitude, and elevation) to serve as a “null” model against which the AIC scores of the models containing land-cover variables could be compared. Because horizontal land-cover diversity and strata proportions were calculated (albeit differently) from the same data, we first examined these variables separately in their own models (i.e. Equation (2), where the species-richness estimate of route i is a function of latitude, longitude, elevation, and either land-cover diversity or the proportion of strata-class 0, 1, 2, or 3 (land) with an error term).(2)
(2)
We then generated pairwise models for each of the strata classes that contained the same geographic variables with both land-cover diversity and the proportion of that strata class as explanatory variables (Equation (3), where the species-richness estimate of route i is a function of latitude, longitude, elevation, land-cover diversity (lcd) and the proportion of strata-class j (strata) with an error term). This use of separate models allowed us to determine whether collinearity between land-cover diversity and strata class affected the sign of the regression coefficients.
(3)
(3)
When assessing the significance of the regression coefficients, we used a Bonferroni inequality to reduce the inflated probability of a Type I error induced by multiple hypothesis-tests (Ott & Longnecker, Citation2001; Sokal & Rohlf, Citation1981), which adjusted the significance threshold down from α = 0.05–0.005 (i.e. α/10 hypothesis-tests; Zuur, Ieno, & Smith, Citation2007).
In terms of our first research question, we anticipated that lower AIC scores for models containing land-cover data (diversity, strata class, or both) would indicate that landscape-level variables are indeed important explanatory factors at our study scale. Based on the expectation that higher levels of both horizontal land-cover diversity and vertical vegetative stratification indicate a larger diversity of available habitats, we anticipated that the estimated coefficients for land-cover diversity and the proportion of strata-class 3 would be positive, whereas those for strata class 1 would be negative. The signs of the coefficients for classes 0 and 2 were more difficult to anticipate, as class 0 represents indeterminate degrees of vegetative stratification and the sign of the coefficient for class 2 would depend largely on whether its increase was at the cost of class 1 (positive) or class 3 (negative).
To determine whether changes in land-cover diversity affect the relative importance of vertical stratification and under what conditions this occurs, we used a regression-tree analysis to situate both geographic and landscape-scale variables hierarchically. Because regression-tree analysis is robust to interactions between independent variables (De’ath & Fabricius, Citation2000; Zuur et al., Citation2007), we were able to include all variables in a single model despite the aforementioned problem of multicollinearity. Optimally homogeneous splitting-points in the data were identified by binary recursive partitioning of the independent variables, and the number of terminal nodes (leaves) was determined by a cross-validation algorithm that successively “prunes” the tree according to a range of cp (complexity parameter) values until the mean of the cross-validations is less than the mean values of the cross-validation errors plus the standard deviation of the cross-validations on convergence. We used the “tree” library for R (Ripley, Citation2009) to generate this model.
If the explanatory power of vertical stratification decreases with increasing land-cover diversity, then we anticipated that when strata class appeared in the regression tree it would be situated below land-cover diversity and on the lower side of the splitting point.
4. Results
The results here are divided into two groups: (1) those from preliminary data assessment and (2) those produced by the formal hypothesis tests.
4.1. Preliminary data-assessment
Species-richness estimates ranged from 9.2 to 111.8 with a mean of 64.0 ± 17.4 SD and a Gaussian distribution across the study region. To ensure the validity of our statistical models, we used the Lattice tool-set for R (Sarkar, Citation2008) to examine the distributions of species-richness estimates against the eight explanatory variables and the extent to which these distributions exhibited pairwise linear relationships (Hair et al., Citation1998; Maindonald & Braum, Citation2003).
Our initial postulation that detection of relationships between proportions of strata-class 2 and species richness would be more problematic than with other explanatory variables was reinforced by the widespread absence of land-cover types in strata-class 2 from the route buffers. Whereas classes 0 and 1 were present in all 2,087 route-buffers (albeit with minimum proportions of 0.001%, but maximums of 95.1 and 99.9%, respectively) and class 3 was only absent from 22 route buffers, class 2 was entirely absent from nearly 50% (i.e. 1,031) buffers. This disparity in the representation of class 2 may be an important factor in the strong negative relationship to species richness indicated by the formal analyses (see below) and the preliminary data visualization (see Figure ).
Figure 2. Scatter plots of species-richness estimates against latitude, longitude, mean elevation, standard deviation of elevation, land-cover diversity, and proportions of the four strata classes present within 5 km buffers around each BBS route.
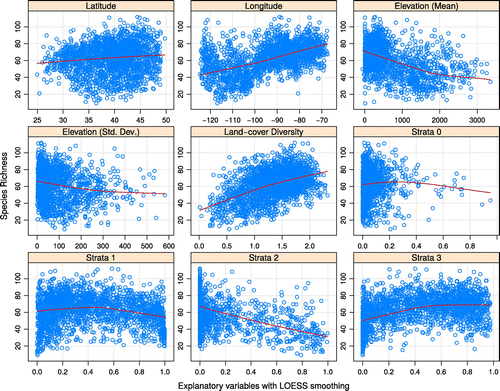
As discussed above, strata class 0 contains land-cover classes without vegetation and those with otherwise indeterminate stratification. Although a negative relationship to species richness may reasonably be postulated for at least the barren cover-types, the clustering of the majority of species-richness estimates at low values of strata proportion (indicated in Figure ) made it difficult to determine whether a statistically significant correlation–coefficient reflected a biologically significant relationship or simply that the proportion of cells in strata-class 0 was greater than 50% in less than 15 of the 2,087 route-buffers.
The general distribution of species-richness estimates with respect to land-cover diversity, and proportions of cells in strata-classes 1 and 3, confirmed our initial stipulation that these relationships may be non-linear. The locally weighted regression curves (LOWESS; Cleveland, Citation1981) in Figure depict these curvilinear relationships more clearly, suggesting that they may be modeled more precisely as curvilinear regressions rather than by straight lines. However, the position of the start and end points of the LOWESS curves at different points along the y-axis and the overall shapes of the curves indicated that a linear model should capture the basic trends in the data sufficiently enough to indicate the overall directions of the relationships between variables. As we were primarily concerned with the slopes of the coefficients rather than the closeness of fit between model curves and data points (Zuur et al., Citation2007), we used linear models for formal hypothesis-testing.
4.2. Results of formal hypothesis-testing
4.2.1. Multiple-regression models
Of the 10 multiple-regression models examined, the model containing both land-cover diversity and proportion of strata-class 3 yielded an AIC value substantially lower than the others (16,621, vs. the next lowest value of 16,667, Table ), and was the only model in which the confidence intervals for both land-cover diversity and proportion of strata class were both consistently positive and statistically significant.
Table 2. Confidence intervals (95% on α=0.05) for coefficient estimates of listed variables, significance levels of the same, and the last three digits (all numbers began with 16) of model-wise AIC values for each of the multiple-regression models examined (10 models total)
The confidence intervals of the coefficients for latitude, longitude, standard deviation of elevation, land-cover diversity, and proportion of strata-class 3 were all positive, with the slopes for both mean and standard deviation of elevation statistically significant but close to zero. Where statistically significant, on the other hand, the slopes of the coefficients for strata-classes 0–2 were all negative (although the upper limit of the interval of strata-class 2 is positive where it is modeled without land-cover diversity; Table ). Only in the model containing the proportion of cells in strata-class 0 with geographic variables and that containing both land-cover diversity and the proportion of cells in strata-class 1 was the null hypothesis for the slope of the coefficient for strata class not rejected, and this only after adjusting the significance test for multiple comparisons (using the Bonferroni adjustment, as discussed above).
4.2.2. Regression-tree analysis
The CART analysis yielded a tree with 6 splitting points using longitude, latitude, land-cover diversity, and proportion of strata-class 3, and with 7 terminal nodes (leaves; Table ). The division on longitude <−97.4 was responsible for the greatest amount of deviation reduction, but was seconded by landscape factors on both sides of the division. The node with the highest mean species-richness identified routes situated east of longitude -97.4, with proportion of forested land >22%, and north of latitude 38.95, and that with the lowest mean identified routes west of longitude −97.4, horizontal land-cover diversity <0.96, and proportion of forested land <0.8% (Figure ).
Table 3. Tabular summary of regression-tree output depicting each node with the value of the splitting point, the number of data points on its right-hand (n), and their deviance and mean
Figure 3. Plot of the regression tree generated using all geographic and land-cover variables together.
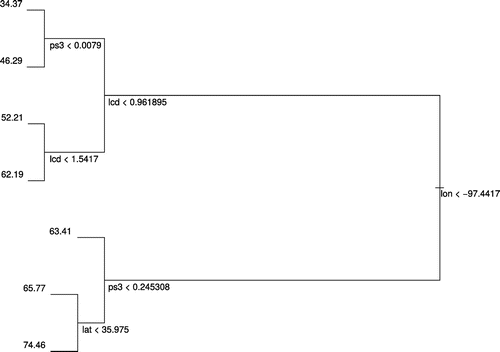
5. Discussion
The positive coefficient estimates for land-cover diversity in all models was consistent with the expectation of our initial hypothesis that more structurally diverse landscapes hold more species. Similarly, the positive coefficient estimates for the proportion of strata-class 3 was consistent with the expectation—and previous studies—indicating that landscapes containing larger proportions of forested area also tend to host more species (Rojas, Pino, Basnou, & Vivanco, Citation2013). Negative coefficients for proportion of strata-class 1, on the other hand, suggested that species-richness levels decrease as the landscape is increasingly dominated by simpler vertical vegetation structure such as that associated with grasslands and agriculture. That the slope of the coefficient for strata-class 1 was not estimated to be significantly different to strata-class 0 when land-cover diversity was included in the model is consistent with the extremely shallow slope indicated by the scatter plots, and suggests that the negative effects of this strata type on species richness is largely counteracted by the overall diversity of the landscape.
The importance of longitude in the regression tree was consistent with the east–west climatic moisture gradient of the conterminous USA (Whittaker, Citation1975). Insofar as they indicated higher species-richness levels on the eastern side of the USA, the results of the regression-tree analysis differed to predictions based on Cook’s (Citation1969) range-based isopleth map of species richness, but were consistent with O’Connor et al.’s (Citation1996) analysis that compared similarly derived species-richness estimates to land-cover features estimated from alternate sources and from specific meteorological variables.
Land-cover diversity was only included in the regression tree where longitude <−97.4, but was situated above the node based on strata-class 3 in this portion of the hierarchy. This suggests that land-cover diversity was more important than strata-class here, and that the proportion of land covered by multi-strata vegetation was only important where diversity <0.96, which is consistent with the expectation that strata class is more important when land cover is more homogeneous.
Where latitude appeared in the regression tree, higher levels of species richness were found north of the dividing line, which was opposite to the hemispheric tropical-arctic species-diversity gradient (Fischer, Citation2004) but consistent with sub-global tendencies indicated in Cook’s (Citation1969) map. The extent to which these results converge logically with those of previous studies reinforces the validity of the variables selected. Divergences, conversely, suggest further investigation of broader- or finer scale factors to refine our understanding of these regional biodiversity trends.
6. Conclusions
The findings in this study indicating that landscape factors remain statistically significant even alongside regional factors across the conterminous USA are consistent with other regional studies (e.g. Culbert et al., Citation2013; Pekin & Pijanowski, Citation2012; Rahbek et al., Citation2007) demonstrating that models that purport to explain spatial patterns of species-richness using only one or a few regional factors (Currie (Citation2007) “strong constraints family” of explanation in biogeography) are necessarily incomplete. Although they were derived from different land-cover datasets and survey years, the findings in this study regarding the positive contributions of land-cover diversity and forests (i.e. strata class 3) to species richness in particularly are consistent with those reported in a similar study by O’Connor et al. (Citation1996), and the factors included in the regression tree here were also identified in their analysis.
We used the 1992 NLCD in place of more recent land-cover data-sets with the hopes that the analytical approach and statistical models used in this study could serve as a basis for subsequent studies that incorporate temporal trajectories of land-cover and species-richness trends and their interactions (Whittaker, Citation1972), as well as compounding factors such as climate change (Stephens et al., Citation2016; Storch et al., Citation2007) and the spread of West Nile virus to North America (Cruz-Pacheco, Esteva, & Vargas, Citation2012). Because the BBS is repeated annually, one of the most straightforward ways to build on the results reported here would be to repeat the analyses we performed using data from other years for which national land-cover data are available. Repeating the regression-tree model with data from other years could be particularly interesting, as it would indicate whether the dominant drivers of species richness remain constant or shift over time.
To be useful to biodiversity conservation, the information regarding species richness provided in this study would need to be complemented by studies of specific species assemblages, and factors such as the endemism and rarity of individual species would need to be considered (Hanberry, Citation2014; Lévêque & Mounolou, Citation2003; Rands et al., Citation2010). The approach described here, however, could be used to relate these additional response variables to the explanatory factors, and the species-richness data itself could help to provide useful preliminary indications of zones or locations with relatively higher levels of overall species-diversity (Gaston & Spicer, Citation1998).
The use of land-cover classes to infer vertical stratification is an important and novel contribution of this study, as it suggests a straightforward research question for further examination—i.e. do more vertically complex landscapes reflect more structurally complex habitats?—while the consistency of the results with our initial hypotheses suggests that these factors may be at least partially extrapolated from land-cover data. As LiDAR (Goetz et al., Citation2014; Tattoni, Rizzolli, & Pedrini, Citation2012) and other forms of higher resolution data—including drone-based aerial imagery (Paneque-Gálvez, McCall, Napoletano, Wich, & Koh, Citation2014)—become available over increasingly larger extents, the multi-scale relationships between habitat and vegetation structure, and landscape diversity, and geo-climatic can be examined more directly. In forecast models calibrated to include future land change (Titeux et al., Citation2016), legacy data-sets, and other instances where newer high-resolution data remain unavailable (Priego-Santander, Campos, Bocco, & Ramírez-Sánchez, Citation2013) our findings indicate that land-cover data can be utilized to partially infer the influence of habitat-level attributes on regional biodiversity trends.
Funding
The authors received no direct funding for this research.
Supplementary material
Supplementary material for this article can be accessed here http://dx.doi.org/10.1080/23311843.2017.1296604.
OAES_1296604_Supplementary_Material.zip
Download Zip (39.6 MB)Acknowledgements
The authors thank Keith Pardieck, Dave Ziolkowski, and other USGS personnel responsible for the BBS, as well as the volunteers who participate in the survey. They also thank the Department of Forestry and Natural Resources of Purdue University for the resources to undertake the investigation described above, and Jeff Holland and Bernie Krause, who served on the first author’s Doctoral Committee with the other authors, for their critical input.
Declaration of interests
None of the authors has any financial interest or other conflict of interest in this study to disclose.
Additional information
Notes on contributors
Brian M. Napoletano
Dr Brian M. Napoletano’s research interests are primarily in the complex interactions between human systems and biophysical patterns and processes, particularly in the context of land change and its underlying politico-economic drivers. Bryan Pijanowski has a PhD in Zoology from Michigan State University. His work focusses on the relationship between land use/cover, biodiversity of animals, hydrology and food security at landscape to continental scales. He employs spatial technologies such as GIS, remote sensing and spatial statistics to understand these relationships. His work has taken him all across North America, Central America, South America, Africa and Asia. Dr Dunning studies the impact of habitat change across large landscapes on native wildlife species. He has used field surveys, simulation modeling and restoration projects (as a form of large-scale experiment) to record the impact of anthropogenic change of birds of forest, grasslands and wetlands.
References
- Akaike, H. (1974). A new look at the statistical model identification. IEEE Transactions on Automatic Control, 19, 716–723.10.1109/TAC.1974.1100705
- Anderson, J., Hardy, E., Roach, J., & Witmer, R. (1976). A land use and land cover classification system for use with remote sensor data (Professional Paper 964). Reston, VA: United States Geological Survey.
- AOU. (1998). The A.O.U. check-list of North American birds (Technical Report 7th Edition). Farmington, NM: American Ornithologists’ Union, Committee on Classification and Nomenclature of North and Middle American Birds. Includes through 48th Supplement.
- Böhning-Gaese, K. (1997). Determinants of avian species richness at different spatial scales. Journal of Biogeography, 24, 49–60.10.1111/jbi.1997.24.issue-1
- Boulinier, T., Nichols, J. D., Sauer, J. R., Hines, J. E., & Pollock, K. H. (1998). Estimating species richness: The importance of heterogeneity in species detectability. Ecology, 79, 1018–1028.10.1890/0012-9658(1998)079[1018:ESRTIO]2.0.CO;2
- Boyd, D., Foody, G., & Ripple, W. (2002). Evaluation of approaches for forest cover estimation in the Pacific Northwest, USA, using remote sensing. Applied Geography, 22, 375–392.10.1016/S0143-6228(02)00048-6
- Brown, J. H., Ernest, S. K. M., Parody, J. M., & Haskell, J. P. (2001). Regulation of diversity: Maintenance of species richness in changing environments. Oecologia, 126, 321–332.10.1007/s004420000536
- Brown, J. H., & Sax, D. F. (2004). Gradients in species diversity: Why are there so many species in the tropics? In M. V. Lomolino, D. F. Sax, & J. H. Brown (Eds.), Foundations of biogeography—Classic papers with commentaries (pp. 1145–1154). Chicago, IL: University of Chicago Press.
- Cam, E., Nichols, J. D., Sauer, J. R., Hines, J. E., & Flather, C. H. (2000a). Relative species richness and community completeness: Birds and urbanization in the Mid-Atlantic States. Ecological Applications, 10, 1196–1210.10.1890/1051-0761(2000)010[1196:RSRACC]2.0.CO;2
- Cam, E., Sauer, J. R., Nichols, J. D., Hines, J. E., & Flather, C. H. (2000b). Geographic analysis of species richness and community attributes of forest birds from survey data in the Mid-Atlantic Integrated Assessment Region. Environmental Monitoring and Assessment, 63, 81–94.10.1023/A:1006473804874
- Carroll, C., Noss, R. F., Paquet, P. C., & Schumaker, N. H. (2003). Use of population viability analysis and reserve selection algorithms in regional conservation plans. Ecological Applications, 13, 1773–1789.10.1890/02-5195
- Chesser, R. T., Banks, R. C., Barker, F. K., Cicero, C., Dunn, J. L., Kratter, A. W., … Lovette, I. J. (2010). Fifty-first supplement to the American ornithologists' union check-list of North American birds. The Auk, 127, 726–744.10.1525/auk.2010.127.3.726
- Cleveland, W. S. (1981). LOWESS: A program for smoothing scatterplots by robust locally weighted regression. The American Statistician, 35, 54–54.10.2307/2683591
- Cook, R. E. (1969). Variation in species density of North American birds. Systematic Zoology, 18, 63–84.10.2307/2412411
- Coppedge, B. R., Engle, D. M., Masters, R. E., & Gregory, M. S. (2001). Avian response to landscape change in fragmented southern Great Plains grasslands. Ecological Applications, 11, 47–59.10.1890/1051-0761(2001)011[0047:ARTLCI]2.0.CO;2
- Costanza, J., Moody, A., & Peet, R. (2011). Multi-scale environmental heterogeneity as a predictor of plant species richness. Landscape Ecology, 26, 851–864.10.1007/s10980-011-9613-3
- Cruz-Pacheco, G., Esteva, L., & Vargas, C. (2012). Multi-species interactions in West Nile virus infection. Journal of Biological Dynamics, 6, 281–298.10.1080/17513758.2011.571721
- Culbert, P. D., Radeloff, V. C., Flather, C. H., Kellndorfer, J. M., Rittenhouse, C. D., & Pidgeon, A. M. (2013). The influence of vertical and horizontal habitat structure on nationwide patterns of avian biodiversity. The Auk, 130, 656–665.10.1525/auk.2013.13007
- Currie, D. J. (2007). Regional-to-global patterns of biodiversity, and what they have to say about mechanisms. In D. Storch, P. Marquet, & J. Brown (Eds.), Scaling biodiversity (pp. 258–282). Cambridge: Cambridge University Press.
- Davies, A. B., & Asner, G. P. (2014). Advances in animal ecology from 3D-LiDAR ecosystem mapping. Trends in Ecology and Evolution, 29, 681–691.10.1016/j.tree.2014.10.005
- De’ath, G., & Fabricius, K. E. (2000). Classification and regression trees: A powerful yet simple technique for ecological data analysis. Ecology, 81, 3178–3192.10.1890/0012-9658(2000)081[3178:CARTAP]2.0.CO;2
- de Roos, A. M., & Sabelis, M. W. (1995). Why does space matter? In a spatial world it is hard to see the forest before the trees. Oikos, 74, 347–348.
- del Carmen Sabatini, M., Verdiell, A., Rodríguez Iglesias, R. M., & Vidal, M. (2007). A quantitative method for zoning of protected areas and its spatial ecological implications. Journal of Environmental Management, 83, 198–206.10.1016/j.jenvman.2006.02.005
- Donovan, T. M., & Flather, C. H. (2002). Relationships among North American songbird trends, habitat fragmentation, and landscape occupancy. Ecological Applications, 12, 364–374.
- Elton, C. (2001). Animal ecology. Chicago, IL: University of Chicago Press.
- Farina, A. (1998). Principles and methods in landscape ecology: Towards a science of landscape, volume 3 of landscape series (2nd ed.). Dordrecht: Springer.10.1007/978-94-015-8984-0
- Fischer, A. G. (2004). Latitudinal gradients in organic diversity. In M. V. Lomolino, D. F. Sax, & J. H. Brown (Eds.), Foundations of biogeography—Classic papers with commentaries (pp. 1168–1185). Chicago, IL: University of Chicago Press.
- Flather, C. H., & Sauer, J. R. (1996). Using landscape ecology to test hypotheses about large-scale abundance patterns in migratory birds. Ecology, 77, 28–35.10.2307/2265651
- Foley, J. A., DeFries, R., Asner, G. P., Barford, C., Bonan, G., Carpenter, S. R., … Snyder, P. K. (2005). Global consequences of land use. Science, 309, 570–574.10.1126/science.1111772
- Frost, P., Campbell, B., Medina, G., and Usongo, L. (2006). Landscape-scale approaches for integrated natural resource management in tropical forest landscapes. Ecology and Society, 11(2), 30.10.5751/ES-01932-110230
- Gaston, K. J., & Blackburn, T. M. (1995). mapping biodiversity using surrogates for species richness: Macro-scales and new world birds. Proceedings of the Royal Society B: Biological Sciences, 262, 335–341.10.1098/rspb.1995.0214
- Gaston, K. J., & Spicer, J. I. (1998). Conservation science and action. Oxford: Blackwell Science.10.1002/9781444313499
- Gaston, K. J., & Williams, P. H. (1993). Mapping the world's species-the higher Taxon approach. Biodiversity Letters, 1, 2–8.10.2307/2999642
- Goetz, S. J., Sun, M., Zolkos, S., Hansen, A., & Dubayah, R. (2014). The relative importance of climate and vegetation properties on patterns of North American breeding bird species richness. Environmental Research Letters, 9, 18 pp., 034013.10.1088/1748-9326/9/3/034013
- Gu, W., & Swihart, R. K. (2004). Absent or undetected? Effects of non-detection of species occurrence on wildlife-habitat models. Biological Conservation, 116, 195–203.10.1016/S0006-3207(03)00190-3
- Hair, J. F., Tatham, R. L., Anderson, R. E., & Black, W. (1998). Multivariate data analysis (5th ed.). Upper Saddle River, NJ: Pearson Education.
- Hanberry, B. B. (2014). Compositional changes in selected forest ecosystems of the western United States. Applied Geography, 52, 90–98.10.1016/j.apgeog.2014.05.004
- Harris, H. J., Milligan, M. S., & Fewless, G. A. (1983). Diversity: Quantification and ecological evaluation in freshwater marshes. Biological Conservation, 27, 99–110.10.1016/0006-3207(83)90082-4
- Harris, J. B. C., & Haskell, D. G. (2007). Land cover sampling biases associated with roadside bird surveys. Avian Conservation and Ecology, 2(2), 1–19.
- HilleRisLambers, J., Harsch, M. A., Ettinger, A. K., Ford, K. R., & Theobald, E. J. (2013). How will biotic interactions influence climate change–induced range shifts? Annals of the New York Academy of Sciences, 1297, 112–125.
- Ibáñez, I., Clark, J. S., Dietze, M. C., Feeley, K., Hersh, M., LaDeau, S., … Wolosin, M. S. (2006). Predicting biodiversity change: Outside the climate envelope, beyond the species-area curve. Ecology, 87, 1896–1906.
- Jarvis, A., Reuter, H., Nelson, A., and Guevara, E. (2008). Hole-filled SRTM for the globe [Version 4, CGIAR-CSI SRTM 90m Database]. Washington, DC: Consortium for Spatial Information.
- Jetz, W., Wilcove, D. S., & Dobson, A. P. (2007). Projected impacts of climate and land-use change on the global diversity of birds. Public Library of Science, Biology, 5(6), e157.
- Karr, J. R., & Roth, R. R. (1971). Vegetation structure and avian diversity in several new world areas. The American Naturalist, 105, 423–435.10.1086/282735
- Kendall, W. L., Peterjohn, B. G., & Sauer, J. R. (1996). First-time observer effects in the North American breeding bird survey. The Auk, 113, 823–829.10.2307/4088860
- Kolios, S., & Stylios, C. D. (2013). Identification of land cover/land use changes in the greater area of the Preveza peninsula in Greece using Landsat satellite data. Applied Geography, 40, 150–160.10.1016/j.apgeog.2013.02.005
- Lawler, J. J., & O’Connor, R. J. (2004). How well do consistently monitored Breeding Bird Survey routes represent the environments of the counterminous United States? The Condor, 106, 801–814.10.1650/7472
- Lévêque, C., & Mounolou, J.-C. (2003). Biodiversity (English ed.). West Sussex: Wiley.
- Link, W. A., & Sauer, J. R. (1998). Estimating population change from count data: Application to the North American breeding bird survey. Ecological Applications, 8, 258–268.10.1890/1051-0761(1998)008[0258:EPCFCD]2.0.CO;2
- Ma, Z., Zuckerberg, B., Porter, W. F., & Zhang, L. (2012). Use of localized descriptive statistics for exploring the spatial pattern changes of bird species richness at multiple scales. Applied Geography, 32, 185–194.10.1016/j.apgeog.2011.05.005
- MacArthur, R. H. (1964). Environmental factors affecting bird species diversity. The American Naturalist, 98, 387–397.10.1086/282334
- MacArthur, R. H. (2004). Geographical ecology: Patterns in the distribution of species. In M. V. Lomolino, D. F. Sax, & J. H. Brown (Eds.), Geographical ecology: Patterns in the distributions of species (Chapters 7, 8, pp. 1217–1253). New York, NY: Harper & Row.
- MacArthur, R. H., & MacArthur, J. W. (1961). On bird species diversity. Ecology, 42, 594–598.10.2307/1932254
- MacArthur, R. H., & Wilson, E. O. (1967). The theory of island biogeography, volume 1 of monographs in population biology (paperback ed.). Princeton, NJ: Princeton University Press.
- Maindonald, J., & Braum, J. (2003). Data analysis and graphics using R: An example-based approach. Cambridge Series in Statistical and Probabilistic Mathematics (1st ed.). Cambridge: Cambridge University Press.
- Marcantonio, M., Rocchini, D., Geri, F., Bacaro, G., & Amici, V. (2013). Biodiversity, roads, and landscape fragmentation: Two Mediterranean cases. Applied Geography, 42, 63–72.10.1016/j.apgeog.2013.05.001
- McKendry, J. E., & Machlis, G. E. (1993). The role of geography in extending biodiversity gap analaysis. Applied Geography, 13, 135–152.10.1016/0143-6228(93)90055-6
- Naveh, Z. (1994). From biodiversity to ecodiversity: A landscape-ecology approach to conservation and restoration. Restoration Ecology, 2, 180–189.10.1111/rec.1994.2.issue-3
- Nichols, J. D., Boulinier, T., Hines, J. E., Pollock, K. H., & Sauer, J. R. (1998). Inference methods for spatial variation in species richness and community composition when not all species are detected. Conservation Biology, 12, 1390–1398.10.1111/j.1523-1739.1998.97331.x
- O’Connor, R. J., Jones, M. T., White, D., Hunsaker, C., Loveland, T., Jones, B., & Preston, E. (1996). Spatial partitioning of environmental correlates of avian biodiversity in the conterminous United States. Biodiversity Letters, 3, 97–110.10.2307/2999723
- Oksanen, J., Kindt, R., Legendre, P., O’Hara, B., & Stevens, M. H. H. (2009). The vegan package: Community ecology package [Package ‘vegan’]. Vienna: R Foundation for Statistical Computing.
- Ott, R. L., & Longnecker, M. (2001). An introduction to statistical methods and data analysis (5th ed.). Duxbury, Pacific Grove, CA: Thomson Learning.
- Paneque-Gálvez, J., McCall, M. K., Napoletano, B. M., Wich, S. A., & Koh, L. P. (2014). Small drones for community-based forest monitoring: An assessment of their feasibility and potential in tropical areas. Forests, 5, 1481–1507.10.3390/f5061481
- Pekin, B. K., & Pijanowski, B. C. (2012). Global land use intensity and the endangerment status of mammal species. Diversity and Distributions, 18, 909–918.10.1111/j.1472-4642.2012.00928.x
- Pijanowski, B. C., & Robinson, K. D. (2011). Rates and patterns of land use change in the Upper Great Lakes States, USA: A framework for spatial temporal analysis. Landscape and Urban Planning, 102, 102–116.10.1016/j.landurbplan.2011.03.014
- Priego-Santander, Á. G., Campos, M., Bocco, G., & Ramírez-Sánchez, L. G. (2013). Relationship between landscape heterogeneity and plant species richness on the Mexican Pacific coast. Applied Geography, 40, 171–178.10.1016/j.apgeog.2013.02.013
- Rahbek, C., Gotelli, N. J., Colwell, R. K., Entsminger, G. L., Rangel, T. F. L., & Graves, G. R. (2007). Predicting continental-scale patterns of bird species richness with spatially explicit models. Proceedings of the Royal Society B: Biological Sciences, 274, 165–174.10.1098/rspb.2006.3700
- Rands, M. R. W., Adams, W. M., Bennun, L., Butchart, S. H. M., Clements, A., Coomes, D., … Entwistle, A. (2010). Biodiversity conservation: challenges beyond 2010. Science, 329, 1298–1303.10.1126/science.1189138
- R Development Core Team. (2008). R: A language and environment for statistical computing. Vienna: The R Foundation.
- Reid, W. V. (1998). Biodiversity hotspots. Trends in Ecology and Evolution, 13, 275–280.10.1016/S0169-5347(98)01363-9
- Ripley, B. (2009). Classification and regression trees (Package ‘tree’). R Foundation for Statistical Computing. Vienna.
- Rojas, C., Pino, J., Basnou, C., & Vivanco, M. (2013). Assessing land-use and -cover changes in relation to geographic factors and urban planning in the metropolitan area of Concepción (Chile). Implications for biodiversity conservation. pplied Geography, 39, 93–103.
- Russell, M. B., Domke, G. M., Woodall, C. W., & D’Amato, A. W. (2015). Comparisons of allometric and climate-derived estimates of tree coarse root carbon stocks in forests of the United States. Carbon Balance and Management, 10, 20.10.1186/s13021-015-0032-7
- Rweyongeza, D. M., Barnhardt, L. K., & Hansen, C. (2011). Patterns of optimal growth for white spruce provenances in Alberta (Technical Report Ref. T/255). Alberta: Government of Alberta Sustainable Resource Center.
- Sala, O. E., Chapin, F. S., III, Armesto, J. J., Berlow, E., Bloomfield, J., Dirzo, R., … Wall, D. H. (2000). Global biodiversity scenarios for the year 2100. Science, 287, 1770–1774.10.1126/science.287.5459.1770
- Sarkar, D. (2008). Lattice: Multivariate data visualization with R. Use R!. London: Springer.10.1007/978-0-387-75969-2
- Sauer, J. R., Peterjohn, B. G., & Link, W. A. (1994). Observer differences in the North American breeding bird survey. The Auk, 111, 50–62.10.2307/4088504
- Seto, K. C., Güneralp, B., & Hutyra, L. R. (2012). Global forecasts of urban expansion to 2030 and direct impacts on biodiversity and carbon pools. Proceedings of the National Academy of Sciences of the United States of America, 109, 16083–16088.
- Shannon, C. (1948). A mathematical theory of communication. Bell System Technical Journal, 27, 623–656.10.1002/bltj.1948.27.issue-4
- Shore, T. L., Safranyik, L., & Lemieux, J. P. (2000). Susceptibility of lodgepole pine stands to the mountain pine beetle: testing of a rating system. Canadian Journal of Forest Research, 30, 44–49.10.1139/x99-182
- Sokal, R. R., & Rohlf, F. J. (1981). Biometry (2nd ed.). San Francisco, CA: W. H. Freeman and Company.
- Soulé, M. E. (1991). Conservation: Tactics for a constant crisis. Science, 253, 744–750.10.1126/science.253.5021.744
- Stehman, S., Wickham, J., Smith, J., & Yang, L. (2003). Thematic accuracy of the 1992 national land-cover data for the eastern United States: Statistical methodology and regional results. Remote Sensing of Environment, 86, 500–516.10.1016/S0034-4257(03)00128-7
- Stephens, P. A., Mason, L. R., Green, R. E., Gregory, R. D., Sauer, J. R., Alison, J., … Willis, S. G. (2016). Consistent response of bird populations to climate change on two continents. Science, 352, 84–87.10.1126/science.aac4858
- Storch, D., Marquet, P. A., & Brown, J. H. (2007). Introduction: Scaling biodiversity — what is the problem? In D. Storch, P. Marquet, & J. Brown (Eds.), Scaling biodiversity (pp. 1–11). Cambridge: Cambridge University Press.
- Tattoni, C., Rizzolli, F., & Pedrini, P. (2012). Can LiDAR data improve bird habitat suitability models? Ecological Modelling, 245, 103–110.10.1016/j.ecolmodel.2012.03.020
- Thogmartin, W. E., Gallant, A. L., Knutson, M. G., Fox, T. J., & Suárez, M. J. (2004). A cautionary tale regarding use of the national land cover dataset 1992. Wildlife Society Bulletin, 32, 970–978.10.2193/0091-7648(2004)032[0970:CACTRU]2.0.CO;2
- Tilman, D., Fargione, J., Wolff, B., D’Antonio, C., Dobson, A., Howarth, R., … Swackhamer, D. (2001). Forecasting agriculturally driven global environmental change. Science, 292, 281–284.10.1126/science.1057544
- Titeux, N., Henle, K., Mihoub, J.-B., Regos, A., Geijzendorffer, I. R., Cramer, W., … Brotons, L. (2016). Biodiversity scenarios neglect future land-use changes. Global Change Biology, 22, 2505–2515.10.1111/gcb.13272
- Turner, M. G., & Cardille, J. A. (2007). Spatial heterogeneity and ecosystem processes. In J. Wu & R. Hobbs (Eds.), Cambridge studies in landscape ecology (pp. 62–77). Cambridge: Cambridge University Press.
- Turner, M. G., Gardner, R. H., & O’Neill, R. V. (2001). Landscape ecology in theory and practice: Pattern and process. New York, NY: Springer-Verlag.
- UNCBD (United Nations Convention on Biological Diversity). (2010). Global biodiversity outlook 3 (Report). Montreal: Author.
- Urban, N. A., & Swihart, R. K. (2009). Multiscale perspectives on occupancy of meadow jumping mice in landscapes dominated by agriculture. Journal of Mammalogy, 90, 1431–1439.10.1644/08-MAMM-A-393R.1
- USEPA. (2014a). 1992 National land-cover dataset. Retrieved from http://www.epa.gov/mrlc/nlcd.html
- USEPA. (2014b). 1992 National land-cover dataset: Definitions. Retrieved from http://www.epa.gov/mrlc/definitions.html
- USNRC. (2001). Grand challenges in environmental sciences. Washington, DC: National Academies Press.
- Vierling, K. T., Vierling, L. A., Gould, W. A., Martinuzzi, S., & Clawges, R. M. (2008). Lidar: shedding new light on habitat characterization and modeling. Frontiers in Ecology and the Environment, 6, 90–98.10.1890/070001
- Vogelmann, J., Sohl, T., Campbell, P., & Shaw, D. (1998). Regional land cover characterization using Landsat Thematic Mapper data and ancillary data sources. Environmental Monitoring and Assessment, 51, 415–428.10.1023/A:1005996900217
- Wellicome, T. I., Kardynal, K. J., Franken, R. J., & Gillies, C. S. (2014). Off-road sampling reveals a different grassland bird community than roadside sampling: Implications for survey design and estimates to guide conservation. Avian Conservation and Ecology, 9(1), 1–13.
- Whittaker, R. H. (1972). Evolution and measurement of species diversity. Taxon, 21, 213–251.10.2307/1218190
- Whittaker, R. H. (1975). Communities and ecosystems (2nd ed.). New York, NY: Macmillan.
- Whittaker, R. J., Willis, K. J., & Field, R. (2001). Scale and species richness: Towards a general, hierarchical theory of species diversity. Journal of Biogeography, 28, 453–470.10.1046/j.1365-2699.2001.00563.x
- Wickham, J., Stehman, S., Smith, J., & Yang, L. (2004). Thematic accuracy of the 1992 national land-cover data for the western United States. Remote Sensing of Environment, 91, 452–468.10.1016/j.rse.2004.04.002
- Wilson, E. O. (2000). A global biodiversity map. Science, 289, 2279.
- Wilson, E. O. (2002). The future of life. New York, NY: Alfred A. Knopf.
- Zuur, A. F., Ieno, E. N., & Smith, G. M. (2007). Analysing ecological data. New York, NY: Springer.10.1007/978-0-387-45972-1