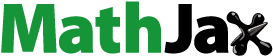
Abstract
The use of data to inform instructional and educational decisions is an increasingly important facet of teachers’ professional practice. However, little is presently known about the best teacher learning mechanisms by which to promote data use. This study (N = 329) examined the nature and distribution of both Illinois public teacher data use practices and teacher learning for data use and how learning opportunities and other factors previously identified in the literature (e.g., leadership and teacher beliefs) relate to data use practices. Our study replicates the importance of specific teacher beliefs for data use practices, and contributes new evidence for the role of course-based learning opportunities.
Public Interest Statement
Teachers need to make many decisions in order to provide high-quality learning environments for students. Many have argued that such decisions should be made on the basis of data, such as test score data on students’ prior knowledge. However, little is known about how to promote data use practices among schoolteachers. In response, this study surveyed Illinois teachers about their data use practices as well as factors that might promote such practices. The study found that teachers who frequently use data to inform decision-making are more likely to hold particular beliefs, such as the belief that “assessment improves teaching.” In addition, this study found that teachers who have taken particular undergraduate and graduate courses more frequently use data to inform decisions. Understanding the factors that relate to teacher data use practices can provide important targets for efforts intended to increase the implementation of those practices.
1. Introduction
Over the past decade, professional and political will within the education field has increasingly championed teachers’ use of assessment data for instructional and educational decision-making. The underlying theory is that using data to inform decisions concerning curricular and instructional goals, methods, and time allocation, teachers can better target learning environments to students, ultimately resulting in higher levels of student achievement (Greenberg & Walsh, Citation2012; Hamilton et al., Citation2009; Means, Padilla, DeBarger, & Bakia, Citation2009). Indeed, rigorous evidence shows that data use initiatives can improve student achievement (e.g. Carlson, Borman, & Robinson, Citation2011).
That data can help classroom teachers support teaching and learning is not an entirely new concept. Formative assessment—student assessment conducted with the purpose of improving teaching and learning—has long been viewed as a key element of effective teaching (Summers, Reeves, Schwartz, & Walker, Citation2015a). In fact, there is a robust literature indicating non-trivial effects of teacher formative assessment practices on student achievement (Black & Wiliam, Citation1998; Herman, Osmundson, Dai, Ringstaff, & Timms, Citation2015). While data use clearly has roots in formative uses of classroom assessment data, the current educational accountability environment inundates teachers today with more and diverse kinds of data from various sources (e.g. scale scores from interim/benchmark and curriculum-based external assessments). Correspondingly, increasing attention has been directed toward teachers’ capacity to use data broadly.
Despite the long-standing interest in teacher data use internationally (Avramides, Hunter, Oliver, & Luckin, Citation2014; Schildkamp, Karbautzki, & Vanhoof, Citation2013; Vanhoof & Schildkamp, Citation2014), however, research shows teacher data use practices still vary widely (Banilower et al., Citation2013; Farley-Ripple & Buttram, Citation2014; Goertz, Oláh, & Riggan, Citation2009). Consequently, scholars and practitioners have directed much attention to factors that support or constrain effective teacher data use. For example, prior research has addressed the roles of student data system infrastructure (Lachat & Smith, Citation2005; Wayman, Jimerson, & Cho, Citation2012), teacher beliefs (Dunn, Airola, Lo, & Garrison, Citation2013), and principal leadership (Wayman, Cho, & Johnston, Citation2007), in supporting data use.
A key constraint on efforts to promote teacher implementation of data-driven decision-making is teachers’ lack of expertise in doing so. Research indicates that the analysis, interpretation, and use of data prove challenging for teachers (e.g. DeLuca & Bellara, Citation2013; Farley-Ripple & Buttram, Citation2014; Means et al., Citation2009), suggesting a need for teacher training and support surrounding data use. Teacher education has therefore received a fair amount of attention in the data use literature as well (Jacobs, Gregory, Hoppey, & Yendol-Hoppey, Citation2009; Kerr, Marsh, Ikemoto, Darilek, & Barney, Citation2006; Mandinach & Gummer, Citation2013a, Citation2013b). Yet, other research estimates minimal and/or uneven opportunities for teachers to learn about and practice data use at the pre-service stage (Reeves & Honig, Citation2015; Greenberg & Walsh, Citation2012; Mandinach, Friedman, & Gummer, Citation2015; Mann & Simon, Citation2010, July).
While scholarship on data use by teachers and its antecedents has burgeoned over the past decade, this body of literature has a number of limitations. First, many studies are of a small-scale nature, basing conclusions on small numbers of participants in a particular school/district context. Second, evidence concerning the frequency and correlates of data use practices (e.g. teacher beliefs and/or leadership) is often derived in a piecemeal fashion from diverse individual studies, making it difficult to draw comparisons, conclusions across studies, and assess the relative contributions of various factors to data use practices. Arguably, findings from the current literature are hard to integrate, given that they are conducted with diverse participants in diverse contexts. Finally, given that reforms aimed at promoting data use have been underway for some time, earlier findings concerning how teachers use data may no longer apply.
In particular, absent from the literature are studies which compare the relative influences of different teacher education mechanisms on data use practices (Marsh, Citation2012). Thus, while there is consensus that teacher data use practices are desirable, less is known about the best formal and informal teacher learning mechanisms—at the pre-service and in-service stages—by which to promote them (Mandinach & Gummer, Citation2013a, Citation2013b). The present literature also lacks studies which consider how teacher education mechanisms relate to data use practices at the same time as other factors such as leadership beliefs.
In the present paper, we seek to address these limitations through a study of Illinois public school teacher data use in 2015. This study re-examines the nature and distribution of teachers’ data use via collection of data from teachers in many districts and schools in the state. We also aim to replicate in the context of a single study, and using different operations of key variables, the importance of several factors implicated in prior scholarship as related to data use (e.g. leadership and/or teacher beliefs). Furthermore, we seek to extend the teacher data use literature by examining more closely the role of teacher learning opportunities, both pre-service and in-service, in promoting data use practices. In doing so, we sought to replicate findings from, update, and extend the literature base related to teacher data use. Our study sought to address the following research questions:
(1) | To what extent and in what ways do Illinois public school teachers use data at the classroom level? | ||||
(2) | What is the distribution of pre-service (e.g. coursework) and in-service (e.g. workshops and/or data teams) learning opportunities for data use in Illinois? | ||||
(3) | What is the relationship between pre-service and in-service learning opportunities and data use, net of contextual factors (i.e. school level, locale, and leadership), and teacher characteristics (e.g. data-driven decision-making self-efficacy beliefs) in Illinois? |
Up-to-date, research-based answers to these questions are critical for both in-school stakeholders, such as leaders, as well as teacher educators. For instance, identification of the specific ways in which teachers use data can help inform implementation of mechanisms to promote the full breadth of teacher data use practices. At the same time, identification of potentially malleable factors related to data use can inform constructs to target (e.g. attitudes toward the value of instructional value of assessment and/or educator leadership for data use) during preparation of both teachers and leaders. This present study also serves to identify the specific types of learning opportunities that might best be implemented to support teacher data use practices. Notably, the present study examined many factors simultaneously in a single study to assess the relative import of these various factors and unify this body of literature.
2. Theoretical framework and literature review
2.1. The nature of data use
Data use—popularly “data-driven decision-making”—has been theorized as a process (Coburn & Turner, Citation2011; Hamilton et al., Citation2009; Means et al., Citation2009) in which an actor (1) accesses or collects data, (2) filters, organizes, or analyzes data into information, (3) combines information with expertise and understanding to build knowledge, (4) knows how to respond and takes action or adjusts one’s practice, and (5) assesses the effectiveness of these actions or outcomes that result (Marsh, Citation2012). In terms of specific data use practices, scholars have differentiated between analysis-oriented and action-oriented tasks (Cosner, Citation2011; Marsh, Pane, & Hamilton, Citation2006). Analysis-oriented tasks include (for example) examining student work products for patterns such as errors and misconceptions, or filtering a data-set in order to disaggregate results by student sub-group. Action-oriented tasks include providing feedback to students (e.g. Hattie & Timperley, Citation2007); selecting students/content on which to focus or instructional method(s) (Mandinach & Gummer, Citation2013a); identifying performance targets, given baseline student data; and deriving student learning objectives (SLO) (Summers, Reeves, Schwartz, & Walker, Citation2015b).
2.2. The distribution of data use practices
Not all data use practices are distributed equally within and across schools. Evidence from several studies suggests variation in the frequencies with which teachers, or groups of teachers, engage in particular data use practices (Banilower et al., Citation2013; Goertz et al., Citation2009). For example, Farley-Ripple and Buttram (Citation2014) found that action-oriented tasks were often more frequent than analysis-oriented tasks among teachers engaged in professional learning communities. While, on average, teachers in their study did engage in each of five analysis-oriented and four action-oriented tasks, the most common data use practice was setting curricular or instructional priorities (an action-oriented task). Other prior research showed that action-oriented data use is typically focused on: modifying instruction for students who are struggling, determining whether to re-teach, and grouping students (see Datnow & Hubbard, Citation2015).
Literature also sheds light on those data use practices that occur among educators with less regularity. For instance, work suggests that teachers less commonly use data to identify reasons for student performance (Nelson, Slavit, & Deuel, Citation2012); identify promising instructional practices (Pashler et al., Citation2007); and inform changes to the specific instructional method one has used (beyond just re-teaching in the same fashion or providing additional support/s; Marsh, Bertrand, & Huguet, Citation2015). In a multi-year study of school-wide reform, Cosner (Citation2011) also found that the nature of data use practices evolved over time, with groups of teachers first focusing on using data to identify instructional objectives, then to group students, and finally to evaluate instructional effectiveness. Unfortunately, findings reviewed in this section are derived from diverse studies conducted with different populations, making direct comparisons difficult. At the same time, altogether these studies are limited in that they examined a relatively small number of specific data use practices. In response, the present study revisits the relative frequencies of a larger breadth of data use practices (27) in a single group of participants.
2.3. The factors related to data use
Much has been written about individual- and organizational-level factors related to teacher data use (e.g. Young & Kim, Citation2010). While the emphasis of the present study was on pre- and in-service teacher educational factors, the literature also highlights the roles of other factors as well. For example, work has addressed data use practices in relation to organizational factors such as principal leadership and individual teacher characteristics such as beliefs. These key factors are discussed in depth in the sections that follow.
2.3.1. Teacher education
Researchers have consistently demonstrated that teachers find practices such as asking questions of, analyzing and interpreting, and identifying specific instructional practices based on data to be challenging (Piro, Dunlap, & Shutt, Citation2014; Roehrig, Duggar, Moats, Glover, & Mincey, Citation2008; Wayman & Jimerson, Citation2014). That teachers find this lattermost data use practice (i.e. selecting particular instructional practices) difficult is perhaps not surprising, given oft-insufficient research on the optimality of a particular instructional method in certain circumstances. Nonetheless, such teacher difficulties with data use have led to calls in policy and practice for enhanced pre- and in-service teacher learning opportunities on this front (Data Quality Campaign, Citation2014; Kerr et al., Citation2006; Mandinach & Gummer, Citation2013a, Citation2013b).
2.3.1.1. Pre-service teacher education
Pre-service teacher preparation programs are designed to promote changes in teacher candidates’ knowledge, skills, attitudes, and beliefs; graduates then practice accordingly in P-12 classroom settings, and these practices positively affect P-12 student learning (Diez, Citation2010). As such, an emphasis on data use during initial teacher preparation could arguably equip teachers with the knowledge and skills (or beliefs and attitudes for that matter), requisite for eventual and effectual data use practices upon field entry (Data Quality Campaign, Citation2014; Mandinach et al., Citation2015).
Some work has attempted to describe broadly the status of pre-service teacher preparation for data use. Large, national survey studies conducted in the US have estimated minimal elementary and secondary pre-service teacher opportunities to learn about and practice data use (Data Quality Campaign, Citation2013; Mandinach et al., Citation2015). In particular, opportunities to work collaboratively around, and analyze, interpret, and use standardized test data are insufficiently represented in pre-service curricula (Greenberg, Walsh, & McKee, Citation2015). There is also some evidence that clinical experiences can provide opportunities for teacher learning concerning data use (Athanases, Bennett, & Wahleithner, Citation2013; Reeves, Citation2016).
A handful of other prior studies reported on course-based pre-service experiences aimed at promoting teacher education students’ capacity to use data. For instance, Piro and colleagues’ “Data Chat” involved undergraduate pre-service teachers in the collaborative analysis of assessment data. During that intervention study, participants exhibited pretest–posttest increases in self-efficacy and knowledge related to data analysis and interpretation (Piro et al., Citation2014). Similarly, Reeves and Honig (Citation2015) reported pretest–posttest belief and objective knowledge gains during a course-based classroom assessment data literacy intervention for pre-service teachers.
The aforementioned literature on pre-service teacher preparation for data use largely consists of small-scale studies of course-based interventions intended for undergraduate students. While these studies have suggested effects of such programming on knowledge and beliefs (Athanases et al., Citation2013; Reeves & Honig, Citation2015), less is known about relationships between pre-service preparation and teachers’ ultimate data use practices in the classroom. There is also a paucity of work on the distribution of different types of undergraduate teacher education courses completed by teachers (for example, stand-alone courses in data use/data-driven decision-making; Mandinach et al., Citation2015). Even less is known as it relates to the distribution of graduate-level courses intended to promote data use practices, and relationships between prior coursework and in-service teachers’ data use practices.
Unsparingly, the status of the knowledge base related to pre-service education (conceived to include both undergraduate and graduate coursework) has resulted in calls for future research. In particular, scholars have argued for the need to address outstanding questions concerning the nature, distribution, and impact of such teacher learning opportunities (Arrington & Lu, Citation2015; DeLuca & Bellara, Citation2013; Greenberg & Walsh, Citation2012; Hamilton et al., Citation2009; Mandinach & Gummer, Citation2013a, Citation2013b; Mandinach, Gummer, & Muller, Citation2011). Thus, in the present study, one aim was to examine how prior participation in a variety of formal courses, both undergraduate and graduate (e.g. assessment and/or teacher inquiry/teacher research/action research), relates to teachers’ data use practices.
2.3.1.2. In-service teacher education
Another mechanism by which to promote to teacher practice around data use is in-service teacher education broadly. Most efforts intended to equip teachers for data use, at least of late, fall within this category. For example, the literature contains evidence for the effects of in-service teacher data use interventions (or interventions containing data use training components) on (1) teacher practices such as analyzing data, setting instructional goals, and providing feedback to students (Gearhart & Osmundson, Citation2009; Mertler, Citation2009) and (2) student achievement (Carlson et al., Citation2011; Gearhart and Osmundson, Citation2009; Marsh, Citation2012; McDougall, Saunders, & Goldenberg, Citation2007; Wayman & Jimerson, Citation2014; Young & Kim, Citation2010). Relative to pre-service teacher education, in-service teacher learning mechanisms might better promote data use, given that they are embedded in authentic contexts (Bocala & Boudett, Citation2015).
However, other literature evidences uneven or limited effects of some in-service data use interventions (Carlson et al., Citation2011; Hamilton et al., Citation2009; Kerr et al., Citation2006; Marsh, Citation2012; Turner & Coburn, Citation2012). Some studies report considerable post-intervention variation among in-service teachers in data use practices such as examining student data, setting curricular or instructional priorities, selecting instructional methods to address specific patterns in data (e.g. student errors or misconceptions; Farley-Ripple & Buttram, Citation2014; Goertz et al., Citation2009), and progress monitoring (e.g. Roehrig et al., Citation2008). No doubt, many data use practices are arduous, requiring the integration of data-based interpretations with both content and pedagogical knowledge (Coburn & Turner, Citation2011; Young & Kim, Citation2010).
In-school teacher education mechanisms by which to promote data use are various (Coburn & Turner, Citation2011). Such mechanisms comprise traditional in-service workshops, as well as more reformed mechanisms for teacher learning such as data teams, data coaching, and professional learning communities (Marsh, Citation2012). There is some evidence that particular forms of in-service workshops can be effective in terms of promoting data use practices among both in-service (Carlson et al., Citation2011; Murnane, Sharkey, & Boudett, Citation2005) and student-teachers (Reeves, Citation2016). On the other hand, professional learning communities, and particularly data teams and data coaching, are newer and have less evidentiary support (Hamilton et al., Citation2009). Each of these latter in-service methods of promoting data use are grounded in the assumption that teacher collaboration is a key condition for data use (Lachat & Smith, Citation2005; Wayman et al., Citation2012) and are discussed next in turn.
2.3.1.2.1. Data teams
Data teams are an increasingly common mechanism by which to promote data use. Data teams bring together diverse within-school stakeholders such as teachers, subject matter specialists, and administrators to support data use (Cosner, Citation2011; Farley-Ripple & Buttram, Citation2014). These often grade- or subject-specific teams meet periodically to engage collaboratively in and model for other teachers’ data analysis, interpretation, and use of data within a particular school context (Hamilton et al., Citation2009; Lachat & Smith, Citation2005; Marsh, Citation2012). While there is, at present, little direct evidence for the effects of data teams per se on student achievement (Hamilton et al., Citation2009), evidence does suggest potential effects on in-service teacher beliefs and practices (Gallimore, Ermeling, Saunders, & Goldenberg, Citation2009).
2.3.1.2.2. Data coaching
Data coaching initiatives use internal personnel or external consultants to facilitate data-driven decision-making among teachers (Hamilton et al., Citation2009; Marsh et al., Citation2015). In practice, data coaching is often combined with other reform mechanisms such as data teams wherein the coach supports the work of the data team in analyzing, interpreting data, and assists with problem-solving (Lachat & Smith, Citation2005). There is some evidence for the effects of in-service interventions involving data coaching (Hamilton et al., Citation2009), and other research is suggestive of a data coach’s key role in dialoguing with teachers and mediating their data use (e.g. Lachat & Smith, Citation2005; Marsh et al., Citation2015).
2.3.1.2.3. Professional learning communities
A professional learning community (PLC) is another strategy by which to support teacher learning and capacity building vis-à-vis data use. The hallmark features of PLCs are teacher collaboration and interaction concerning their practice and student data (Achinstein, Citation2002; Eaker, DuFour, & Burnette, Citation2002; Fullan, Citation2001). Having been around for about two decades, PLCs and constitutive collegial teacher interactions have been linked to self-reported instructional efficacy, instructional practices (including data use practices), and student achievement measures (e.g. Goddard, Goddard, & Tschannen-Moran, Citation2007; Marsh et al., Citation2015; Mason, Citation2003; Nelson et al., Citation2012).
Much recent attention has been given to the development of teachers’ capacity to use data through different in-service teacher education mechanisms (Hamilton et al., Citation2009; Jacobs et al., Citation2009; Kerr et al., Citation2006). This is especially the case for mechanisms involving teacher collaboration such as professional learning communities and data teams (Wayman et al., Citation2012). In total, the scholarship related to in-service teacher learning for data use suggests that diverse approaches (e.g. data teams and/or in-service workshops) can be effectual. Unfortunately, the fragmented nature of the data use literature precludes easy comparisons across studies; rarely have approaches to in-service teacher learning been contrasted with one another (and to mechanisms such as undergraduate and graduate coursework). Given the many ways to conduct in-service training for data use, the present study attempts to shed light on the (relatively) best mechanisms for in-service teacher learning.
2.3.2. Organizational factors
Research also indicates a number of organizational contextual factors that can facilitate and/or constrain teacher data use, such as principal leadership (e.g. Wayman et al., Citation2012), discussed in this section.
2.3.2.1. School level and locale
Increased emphasis on teacher data use notwithstanding, the cross-school distribution of data use is non-uniform. In particular, several studies observed differences in data use practices by school level (i.e. elementary, middle and/or high). In a large-scale study conducted in Wyoming, Wayman et al. (Citation2007) found that elementary schools featured a culture more facilitative of data use as well as more data use by teachers. Similarly, Means, Gallagher, and Padilla (Citation2007) found that in-service teachers’ likelihood of using data systems for various purposes (e.g. identify knowledge gaps and/or pace instruction) varied as a function of their school level, again favoring elementary-level teachers. As is the case with reforms more generally, locale (e.g. urban, rural and/or suburban) is another important factor in understanding teacher data use. For instance, expertise to promote teacher data use might not be available in rural areas. At the same time, greater resources might be available in suburban districts to build capacity for such practices relative to rural and urban ones (e.g. Provasnik et al., Citation2007).
2.3.2.2. Leadership
A large body of scholarship also implicates the role of leadership in supporting teacher data use practices (Farley-Ripple & Buttram, Citation2014; Lachat & Smith, Citation2005; Wayman et al., Citation2007, Citation2012). In particular, this research highlights the role of school-level administrators, namely principals (Cosner, Citation2011; Gerzon, Citation2015; Kerr et al., Citation2006). Theoretically, principals and other leaders can advance data use practices in a variety of ways. For example, leaders can make the implementation of data use practices a priority and communicate expectations concerning the use of such practices. Leaders can also ensure that teachers have access to a high-quality data system (Kerr et al., Citation2006; Lachat & Smith, Citation2005; Wayman & Jimerson, Citation2014; Wayman et al., Citation2012) and sufficient time to use and collaborate around data (Data Quality Campaign, Citation2013; Greenberg & Walsh, Citation2012; Hamilton et al., Citation2009; Young & Kim, Citation2010).
2.3.3. Teacher characteristics
Another category of variables examined previously with respect to data use (and assessment more generally) are teacher characteristics, namely: teacher beliefs and anxiety (Coburn & Turner, Citation2011; Dunn et al., Citation2013; Kerr et al., Citation2006). In general, beliefs are consequential cognitive constructs because they drive teaching behaviors (Pajares, Citation1992). The natures and strengths of teacher beliefs about assessment and data use can, then, also facilitate or constrain data use practices (e.g. Brown & Remesal, Citation2012). For instance, in Kerr et al.’s (Citation2006) study of data use in three districts undergoing reform, less data use was associated with beliefs that assessment data are invalid. Nelson et al.’s (Citation2012) work also highlighted the role of teacher beliefs in the context of professional learning communities.
While teacher beliefs are various (Brown, Citation2006; Datnow & Hubbard, Citation2015), an especially critical category of beliefs is teachers’ self-efficacy beliefs (Dunn et al., Citation2013)—“beliefs in one’s capabilities to organize and execute courses of action required to produce given attainments” (Bandura, Citation1997, p. 3). Self-efficacy theory posits that beliefs about one’s ability to do something—such as access, analyze, and instructionally use data—affect his or her behavior and effectiveness in performing that behavior (or set of behaviors). Indeed, research has found that measures of teacher self-efficacy are related to both teaching practice and student achievement (Tschannen-Moran, Hoy, & Hoy, Citation1998). As such, successful data use by teachers necessitates self-efficacy with respect to several facets of the data use process, such as identifying and accessing data, analyzing and interpreting data, and deriving instructional implications from data (Dunn et al., Citation2013). Finally, while beliefs in one’s capacity to engage in data-driven decision-making processes can promote those processes, anxiety concerning those processes can impede them (Airola, Dunn, & Garrison, Citation2011).
Given the roles of these constructs, recent arguments have been advanced to change not only teacher knowledge and skills but also beliefs (e.g. beliefs in the utility of data to improve instruction) in order to promote data use practices (Data Quality Campaign, Citation2014). Along these lines, there is evidence that pre-service teachers can change their beliefs in favorable ways concomitant with participation in interventions designed to promote their facility with data (e.g. Reeves & Honig, Citation2015). Given this prior research, this study re-interrogates teacher data use practices in the context of several specific beliefs (namely beliefs about assessment in general and data use self-efficacy beliefs) as well as anxiety, among other variables.
3. Method
3.1. Participants and procedures
Recruitment procedures involved contacting Illinois public school district principals with a request to distribute an electronic survey to their teachers. The analytic sample comprised 329 Illinois public school teachers—from at least 71 schools across at least 54 districts—who served in an instructional role at the time of study participation and provided informed consent.Footnote1 Overall, about 79% of the sample was female, 98% were white, and 3% were Hispanic/Latino. The mean participant age was 39.80 (SD = 11.26). All individual pre-K-12 grade levels were represented in the data; about 31% of the respondents served at the elementary level (grades K-5), about 43% at the middle school level (grades 6–8), about 22% at the high school level (grades 9–12), and about 4% served in roles that spanned levels (e.g. grades 6–12).
3.2. Instrumentation
An online survey was administered via Qualtrics (Citation2015)-elicited data concerning the key variables in the theoretical framework and our research questions. Embedded within the survey were several researcher-developed and existing instruments.
3.2.1. Data use practices
The first block of survey questions represented a researcher-developed instrument intended to provide evidence concerning classroom teacher data use practices. The term “data” was defined at the beginning of the survey as follows:
Data are pieces of information, and include assessment data (e.g. state or district benchmark test scores, student performance on classroom-based formative and summative assessments such as running records, and student work) as well as other types of data such as student attendance and demographics.
Then, respondents were asked the frequency with which they engaged in particular data use practices on a five-point frequency scale (see Table ). Respondents were asked, “How often do you do each of the following?” for each of 27 data use behaviors codified in US professional teaching standards (e.g. Interstate Teacher Assessment & Support Consortium, Citation2011, National Board Standards, and Illinois Professional Teaching Standards). Example behaviors included: “use data to identify student strengths and weaknesses;” “use data to select which content to teach;” and “use data to evaluate the effectiveness of your instruction (e.g. lessons, units).” The items represented individual, sub-group, and overall class data use practices, but were limited to practices that might reasonably be performed in classroom contexts (cf. analyzing achievement trends over multiple years and/or planning long-term district goals). Exploratory common factor analysis (principal axis factoring)Footnote2 revealed a single dominant latent factor underlying these 27 items; the factor had an eigenvalue of 13.41 and explained 49.65% of the common item variance. Extracted communalities ranged from .12 to .69 and factor loadings ranged from .35 (for using data to assign grades) to .83 (for using data to identify student learning needs). Internal consistency reliability (α) was .96.
Table 1. Sample descriptive statistics for teacher data use practices (N = 329)
3.2.2. Leadership
Another researcher-developed instrument was designed to gather evidence concerning school-level leadership for teacher data use. Nineteen items comprised key data use leadership facets defined in the literature: prioritization (e.g. “The administrators in my school make data use a priority”); provision of time and opportunities for teacher data use (e.g. “The administrators in my school have built time for teachers to discuss data into the school schedule”); data use expertise (e.g. “The administrators in my school understand how to use student data to drive instruction”); modeling (“The administrators in my school model student data use practices”); and facilitation of data use (e.g. “The administrators in my school lead discussions about student data”).
Preceded by the prompt, “Please indicate the extent to which you agree with the following statements about your school and your school administration (e.g. principals, assistant principals, department chairs, etc.),” the response format for the items was: 1 = strongly disagree, 2 = disagree, 3 = neither agree nor disagree, 4 = agree, and 5 = strongly agree. Exploratory common factor analysis (principal axis factoring)Footnote3 revealed a single dominant latent factor underlying these 19 items; the factor had an eigenvalue of 11.20 and explained 58.95% of the common item variance. Extracted communalities ranged from .24 to .73 and factor loadings ranged from .49 (for “The administrators in my school expect teachers to use student data to drive instruction”) to .86 (for “The administrators in my school understand how to use student data to drive instruction”). Score internal consistency (α) was .96.
3.2.3. Teacher beliefs and anxiety
Two existing instruments, the Conceptions of Assessment—III Abridged scale (COA-III; Brown, Citation2006) and the Data-Driven Decision-Making Efficacy and Anxiety inventory (3D-MEA; Dunn et al., Citation2013), were, respectively, embedded in the survey to elicit evidence of teacher beliefs related to assessment, and self-efficacy and anxiety related to data-driven decision-making. The COA-III’s nine sub-scales were intended to measure nine distinct beliefs about assessment (e.g. Assessment is Valid, Assessment Makes Students Accountable, and/or Assessment is Bad). Each of the nine COA-III sub-scales had three items and the response format was a six-point rating scale: 1 = strongly disagree; 2 = mostly disagree; 3 = slightly agree; 4 = moderately agree; 5 = mostly agree; and 6 = strongly agree. Reliability estimates for the nine COA-III scales ranged from .32 (for the Assessment Makes Students Accountable sub-scale) to .83 (and was .74 for the Assessment Improves Teaching sub-scale significantly related to data use in the statistical model described later).
The Data-Driven Decision-Making Efficacy and Anxiety (3D-MEA; Dunn et al., Citation2013) inventory’s 20 items assessed four dimensions of data-driven decision-making self-efficacy (i.e. self-efficacy for data identification and access, self-efficacy for data technology use, self-efficacy for data analysis and interpretation, and self-efficacy for application of data to instruction) and data-driven decision-making anxiety. The response format for the 20 3D-MEA items was a five-point rating scale: 1 = strongly disagree; 2 = disagree; 3 = neither agree nor disagree; 4 = agree; and 5 = strongly agree. Internal consistency reliabilities (αs) for the five 3D-MEA sub-scales ranged from .84 to .92
3.2.4. Teacher learning mechanisms
Another set of questions asked respondents about their preparation and training for data use, both pre-service and in-service. Specifically, participants reported the number of each of the following pre-service teacher education courses, undergraduate and graduate, he or she had completed: assessment; data-driven decision-making/data use; response to intervention/progress monitoring; and teacher inquiry/teacher research/action research. Also, participants reported whether they had ever participated in any of the following activities: in-service workshop(s) about assessment; in-service workshop(s) about data-driven decision-making/data use; data teams; data coaching; and professional learning communities focused on assessment/data.
3.2.5. Other data
The final section of the survey collected information about the participants’ professional experience (i.e. years of experience) and work context (e.g. primary position, grade level, subject area, school, and/or district). On the basis of these data, we constructed variables reflecting both school level and school locale. The school-level variable was derived from self-reported data provided by participants concerning the primary grade level in which they taught, and for respondents who did not answer this particular question, information concerning the school in which the participant taught (e.g. “Applewood Elementary School” (a pseudonym) was manually verified to be a K-5 school). Finally, we obtained data on district locale (recoded into four categories: urban, town, suburban, and rural) from the most recently available Common Core of Data (Keaton, Citation2014) using unique National Center for Education Statistics school identifiers.
3.3. Analytic approach
Descriptive statistical analyses were used to understand item response distributions, the scope of missing data, and address the first two research questions. After removing non-consenting and/or otherwise ineligible respondents, missing data were minimal. Missing data ranged between 0 and 1.2% across the 27 data use practice items, between 1.5 and 3.0% across the 19 leadership items, between .9 and 1.8% across the 20 3D-MEA items, and between .9 and 2.7% across the 27 COA-III items. Item-level mean substitution was used to handle these small amounts of missing data for scale item variables prior to conducting the psychometric analyses reported earlier; thus, data were complete for all of these variables for all participants. Missing data for all other variables (e.g. school level and/or pre-service course taking) were also minimal, ranging between .3 and 3.6%; thus, listwise deletion was used for other analyses. For each respondent, composite scores were constructed to represent each of the constructs (i.e. classroom teacher data use practices, leadership, teacher beliefs, and anxiety) by taking the means of their respective items.
Preliminary unconditional multilevel models estimated with available school- and district-level data showed non-significant intercept variance in data use practices by school [ = .03,
, p = .19], and district [
= .02,
, p = .19], and indicated that multilevel modeling was not necessary. Ordinary least squares (OLS) regression analysis was then used to address research question three. In particular, we estimated a series of OLS models in which different categories of variables were either forced into the model on the basis of research and theory or entered based on their empirical properties (i.e. forward stepwise variable entry). The blocks (categories) of variables were: (1) school contextual characteristics (e.g. level, locale, and/or leadership), (2) general assessment beliefs (nine COA-III sub-scales), (3) data-driven decision-making self-efficacy beliefs and anxiety (five 3D-MEA sub-scales), (4) undergraduate and graduate coursework, and (5) in-service teacher learning opportunities (two types of workshops, data coaching, data teams, and professional learning communities). Block one variables were force entered into the model, whereas variables in the other blocks were selected empirically.Footnote4 Unreported analyses found no evidence of differences in data use practices by other school/district contextual factors (e.g. size and/or demographic composition) or participant characteristics of sex, race, and ethnicity. Thus, these data were not included in the model to address possible confounding.
The dependent variable for the third research question was the composite data use practice score and was standardized before regression analysis. All categorical regressor variables were dummy coded. For school level, elementary school was the reference group with dummy variables created for pre-K, middle, high, and other levels. For school locale, suburban school was the reference group with dummy variables created for urban, town, and rural schools. For analytic purposes, the pre-service coursework variables were re-coded such that 0 = no course and 1 = one or more courses. In the final multiple regression analysis, with N = 296 and 13 predictors, statistical power was very high, .99, for two-tailed detection of a medium-sized fixed effect (f2 = .15). Tolerance and variance inflation factor (VIF) indices did not suggest collinearity issues in the final model (Min. tolerance was .58 and Max. VIF was 1.73).
4. Results
4.1. Teacher data use practices
Table presents descriptive statistics for the 27 data use practice items used to address our first research question. Means for these items ranged between 2.61 (between “Once a month or less” and “A few times per month”) and 3.72 (between “A few times per month” and “Once a week”). The most frequently reported in-service teacher data use practices in the sample involved using data to: determine students’ level of achievement after instruction; identify next steps for instruction (e.g. move on and re-teach); identify patterns in student thinking (e.g. errors and/or misconceptions); evaluate the effectiveness of one’s instruction (e.g. lessons and/or units); and modify instruction or lesson plans for current students (e.g. activities, representations, and/or materials). The least frequently reported data use practices were using data to: identify students for acceleration/enrichment; select which assessments to administer; select which content to teach; identify students for more intensive intervention; and communicate student performance to parents. Sample respondents indicating that they “never” used data in particular ways ranged from 1.2 (for identifying next steps for instruction) to 18.8% (for selecting which content to teach).
Also notable are the data use item scores with the largest dispersions, which included using data to: assign grades; select scaffolds to provide; identify patterns in student thinking (e.g. errors and/or misconceptions); and select which content to teach. The most (relatively) homogeneous response patterns pertained to using data to: determine students’ level of achievement after instruction; communicate student performance to parents; identify reasons for poor student performance; identify students for more intensive intervention; and identify gaps in student knowledge.
4.2. Teacher data use learning opportunities
Table presents sample-reported frequencies for participation in particular undergraduate and graduate courses and in-school learning opportunities related to data use (research question two). More of the sample reported having completed undergraduate assessment-focused coursework compared to coursework in other specific areas. About 63% reported having taken at least one assessment course. The percentages of respondents indicating they had completed one undergraduate course (or more) in data-driven decision-making/data use, response to intervention/progress monitoring, and teacher inquiry/teacher research/action research were less than that seen for assessment, ranging from about 37 to 49. While the percent of respondents indicating they took assessment coursework at the graduate level was similar to that seen at the undergraduate level, percentages for the other graduate courses were higher than at the undergraduate level.
Table 2. Sample response distributions for undergraduate and graduate coursework and in-school learning opportunities related to data use (percentages)
Participants’ most commonly reported in-school learning opportunities were in-service workshops focused on assessment (about 89%) and data-driven decision-making/data use (about 77%). Sizable majorities of participants also experienced professional learning communities (about 68%) and data teams (about 63 percent). On the other hand, data coaching was much less common, with only about 24% of respondents indicating having participated in such initiatives.
4.3. Factors related to teacher data use
Table presents descriptive statistics for the multiple regression analytic sample (N = 296) and Table summarizes results from the successively estimated OLS regression models. The reader will recall that variables were entered into the model in blocks. Initially, organizational contextual factors (locale, level, and leadership) were force entered into the model (Block 1). Then, a stepwise forward entry procedure was used to add additional variables from several categories to the model: first, general assessment-related beliefs (Block 2), second data-driven decision-making-related self-efficacy beliefs and anxiety (Block 3), third undergraduate and graduate coursework (Block 4), and fifth in-service teacher learning opportunities (Block 5).
Table 3. Descriptive statistics for multiple regression analytic sample (N = 296)
Table 4. Successive multiple regression analysis model’s results and coefficients (N = 296)
Upon initially entering only the organizational context factors into the model, two variables were significant. Teachers in middle-level schools (compared to teachers in elementary schools) and schools with higher data use leadership had higher data use practices. The general assessment beliefs block (2) introduced one variable, the belief that “assessment informs teaching,” at which point the leadership variable was no longer significant. The data-driven decision-making beliefs and anxiety block (3) introduced one variable as well, the self-efficacy belief concerning one’s ability to apply data to instruction. Block four introduced three coursework variables, first an undergraduate course in data use/data-based decision-making, second a graduate course in response to intervention/progress monitoring, and third a graduate assessment course. With the introduction of undergraduate coursework in data use/data-based decision-making to the model, the middle school variable was no longer significant. No in-school learning opportunity variables (Block 5) were entered into the model.
The final statistical model was statistically significant, F (13, 282) = 11.53, p < .001, and explained about 32% () of the variance in data use practices. In this final model, only the two belief variables and three course variables were statistically significant. In all cases, these variables were positively related to data use practices, with the exception of the graduate assessment course. All effects were small to medium in magnitude. For example, teacher data use was approximately four-tenths of a standard deviation higher for teachers who had completed an undergraduate data use course (after partialing out variance associated with the other variables in the model).
5. Discussion
In the current era of educational accountability, data are ubiquitous. Teachers, as key actors in the education system, are increasingly charged with analyzing, interpreting, and using data, particularly assessment data, to inform practice. Despite increasing international professional interest in teachers anchoring their decisions in data (Avramides et al., Citation2014; Schildkamp et al., Citation2013; Vanhoof & Schildkamp, Citation2014), many in-service teachers struggle with such practices. Correspondingly, there is wide variation among teachers in terms of their data use practices (e.g. Farley-Ripple & Buttram, Citation2014; Goertz et al., Citation2009; Kerr et al., Citation2006). Given this context, it is not surprising that researchers have given much recent attention to data use, including how teachers use data and the antecedents of data use (Datnow & Hubbard, Citation2015).
5.1. Teacher data use practices
Our first research question pertained to the nature and distribution of specific data use practices. As noted earlier, one limitation of the current literature is that such findings are derived from diverse studies in a piecemeal fashion, making comparisons about the relative frequencies of particular practices tenuous. This study’s results imply that on average, Illinois teachers are engaging in each of the 27 observed data use practices codified in US professional standards. However, there is much variation in the frequency with which they implement each of these practices. In what follows, we discuss our findings concerning the frequency of various data use practices and then contextualize them within the prior literature.
Most frequently, data were used to: determine students’ level of achievement after instruction; identify next steps for instruction (e.g. move on and/or re-teach); identify patterns in student thinking (e.g. errors and/or misconceptions); evaluate the effectiveness of one’s instruction (e.g. lessons and/or units); and modify instruction or lesson plans for current students (e.g. activities, representations, and/or materials). These findings are perhaps not surprising, given that these are presumably essential formative and summative assessment purposes from the perspective of classroom teachers. Also among the most common uses of data was evaluating the effectiveness of one’s instruction, which is sensible in light of increasing use of student achievement-based evidence in teacher evaluation systems (Summers et al., Citation2015b).
Data were used least frequently to identify students for acceleration/enrichment; select which assessments to administer; select which content to teach; identify students for more intensive intervention; and communicate student performance to parents. In terms of selecting content to teach and assessments to administer based on data, these practices may occur less frequently because of prescriptive curriculum scope and sequence and assessment policies in some contexts and/or use of off-the-shelf curriculum and assessment materials. In terms of the relatively less frequent teacher use of data to identify students for acceleration/enrichment, this might be explained on account of a dearth of accelerated/enrichment programming in some contexts. The fact that using data to identify students for more intensive intervention was relatively infrequent is interesting, given mandates in Illinois for response to intervention. However, the infrequency of this practice may simply reflect the rate at which students are evaluated for tier reassignment. Alternatively, such practices may be conducted by special educators rather than classroom teachers.
The data use item scores with the largest dispersions included using data to: assign grades; select scaffolds to provide; identify patterns in student thinking (e.g. errors and/or misconceptions); and select which content to teach. Variation in the use of data to assign grades might be explained on account of demonstrated differences in teacher grading practices (e.g. McMillan, Myran, & Workman, Citation2002). Individual teacher differences in using data to identify patterns in student thinking might be a reflection of differences in teacher pedagogical content knowledge (e.g. Hill, Rowan, & Ball, Citation2005). Variation in using data to select which content to teach might reflect differentially prescriptive district/school policies concerning curriculum scope and sequence. Sample respondents indicating that they “never” used data in particular ways ranged from 1.2 (for identifying next steps for instruction) to 18.8% (for selecting which content to teach), which is problematic given that all of these data use practices are reflected in professional standards.
Our findings feature both points of convergence and divergence with prior work on the specific ways in which teachers use data (Banilower et al., Citation2013; Farley-Ripple & Buttram, Citation2014; Goertz et al., Citation2009). For example, work has shown that data use is typically focused on modifying instruction, specifically for students who are struggling, and determining whether to re-teach (see Datnow & Hubbard, Citation2015). In our study, using data to modify instruction and to determine whether to re-teach was also among the most frequent practices. In addition, Farley-Ripple and Buttram (Citation2014) and Cosner (Citation2011) found the most common data use practices were setting curricular or instructional priorities, which is consistent with the relative frequency of using data to plan/design lessons in our study (a key aspect of planning is specifying instructional objectives). Similarly, our work suggests, like prior research (Nelson et al., Citation2012), that teachers less commonly use data to identify reasons for student performance. On the other hand, there are points of divergence between our findings and those of other studies. For example, using data to group students was relatively less common in our study (see Datnow & Hubbard, Citation2015).
The sample-observed variation, and the lack of some teachers’ experience with some practices, might warrant concern among in-school stakeholders (e.g. principals) and teacher educators. For example, about 8% of respondents indicated that they never used data to set student performance goals or targets. This is assumedly an important data use practice in general, but is also requisite for the use of SLO, an approach to incorporating student achievement in teacher evaluation (Summers et., Citation2015b). Indeed, some of these findings probably reflect contextual differences related to the student population, the availability of data, and teacher or organizational readiness for data use.
5.2. Teacher data use learning opportunities
Our next research question concerned teachers’ opportunities to learn how to use data through both undergraduate and graduate coursework and in-school formal and informal learning opportunities. As noted earlier, such opportunities are key mechanisms by which to promote teacher data use and implementation of data use reforms (e.g. Carlson et al., Citation2011; McDougall et al., Citation2007). Our data certainly suggest that teacher education systems, at least in Illinois, are responding to mandates through curricular means. In the present study, this is evidenced by the sheer percentages of teachers reporting having taken various relevant courses at the undergraduate and graduate levels. The most commonly experienced undergraduate and graduate coursework was assessment coursework.
In addition, at the graduate level, we found that teachers reported more often completing other data use-relevant coursework, namely courses in data use/data-driven decision-making, response to intervention/progress monitoring, (and especially) teacher inquiry/teacher research/action research. Even so, 37.2% of teachers or more in our sample had not taken at least one of each of the undergraduate and graduate courses assessed, which suggests a need for in-school learning opportunities as well. These findings are consistent with prior scholarship, suggesting the need for enhanced teacher education for teacher data use (e.g. Mandinach et al., Citation2015).
Relative to in-school experiences, our findings suggest that workshops focused on assessment and data use/data-based decision-making are most commonly experienced by Illinois teachers. Somewhat fewer, but still sizable numbers of teachers, also reported involvement in professional learning communities and data teams. On the other hand, experience with data coaching was less common, a finding also observed in a study with pre-service student-teachers completing a clinical experience (Reeves, Citation2016). Data coaching may be pursued less frequently in schools, given that it requires unique expertise (e.g. external consultants) that may be resource intensive (e.g. Gerzon, Citation2015).
5.3. Factors related to teacher data use
This study also investigated whether and the degree to which various factors account for the level of teacher data use practices. In particular, we investigated the role of teacher learning mechanisms—undergraduate and graduate coursework and in-school experiences—as well as school organizational contextual factors (i.e. level, locale, and leadership) and teacher characteristics. We found that two specific beliefs, i.e. beliefs that assessment improves teaching and that one has the capacity to use data to change instruction, were related to data use practices. This comports with prior research on the role of teacher beliefs in data use (e.g. Coburn & Turner, Citation2011; Kerr et al., Citation2006) and instructional practice more generally (Pajares, Citation1992).
With respect to organizational contextual characteristics, our study fails to replicate findings from earlier research. First, while leadership was statistically significant upon initial model entry, it was not once other variables were accounted for by the model—specifically, the belief variables. This change in significance with model re-specification might be interpreted to mean that leadership for data use is important to the extent that it promotes favorable beliefs toward data. This explanation is plausible given the nature of our leadership variable, which comprised diverse facets of leaderships such as articulating a vision for data use. Such leadership might promote favorable teacher beliefs, which in turn promote data use practices.
To test this hypothesis, we conducted supplemental simple mediation analyses using the Preacher and Hayes (Citation2008) method. We separately tested whether the relationship between leadership and data use practices is mediated by each of the two beliefs that were statistically significant in our final regression model. This analysis confirmed a statistically significant indirect path ab from leadership to data use practices, mediated by the belief that assessment improves teaching (point estimate = .17; 95% bias-corrected confidence interval ranged from .11 to .24). Similarly, the second simple mediation analysis showed a statistically significant indirect path from leadership to data use practices, mediated by the self-efficacy for application of data to instruction (point estimate = .22; 95% bias-corrected confidence interval ranged from .14 to .32). Thus, these findings are suggestive that leadership might promote indirect effects on data use practices through teacher beliefs.
While school locale was unrelated to data use practices in all models, there was a statistically significant mean difference between middle schools and elementary schools in the initial models (i.e. more frequent data use in elementary schools than middle schools). Similar findings have been observed in prior research (Means et al., Citation2007; Wayman et al., Citation2007). However, upon entry of the undergraduate data use/data-based decision-making coursework variable into the model, the school-level difference was no longer significant. This suggested that school-level differences in data use practices may be explained on account of differences in the distribution of course taking across teachers in these school contexts. However, a supplemental chi-square test of association indicated that this was not the case, = .11, p = .74.
In terms of undergraduate and graduate coursework as mechanisms for teacher learning, three course variables were entered into the model. First, having taken undergraduate coursework in data use/data-driven decision-making and graduate coursework in response to intervention (RtI)/progress monitoring was each associated with increased data use practices. Given the fact that RtI/progress monitoring often falls within the purview of special education (SPED) teachers, a sub-set of our sample, supplemental analyses were conducted to rule out that this relationship was spurious (i.e. capturing SPED–non-SPED teacher differences in data use practices). In a sub-sample for whom SPED teacher status was known, we confirmed that special education teachers were indeed more likely to take a graduate RtI/progress monitoring course, = 19.93, p < .01. But, with a SPED teacher dummy variable also included in the regression model, the RtI/progress monitoring course variable was still significant (whereas the SPED variable was not). Second, participation in graduate assessment coursework was negatively associated with data use practices. This finding is curious, and warrants explanation through follow-up research and collection of qualitative data.
We found that undergraduate courses in assessment, response to intervention/progress monitoring, and teacher inquiry/teacher research/action research, and graduate courses in data use/data-driven decision-making and teacher inquiry/teacher research/action research, were unrelated to data use practices, when accounting for other variables in the model. Interestingly, in an earlier study with pre-service student-teachers, participants were more likely to use data if they had taken a teacher inquiry/teacher research/action research course (Reeves, Citation2016). One reason for this discrepancy could be that there is less of a temporal gap between completion of a teacher inquiry course and a student teaching experience, compared to completion of a teacher inquiry course and bonafide service as a teacher. These findings could also be interpreted to mean that such courses do not matter, at least in terms of the frequency with which one engages in data use practices. However, participation in such coursework may indeed be related to the effectiveness of those practices in terms of promoting teaching and learning, or may exert an effect indirectly (e.g. through beliefs and attitudes).
This study offers no evidence that each of five in-school formal and informal teacher learning experiences relates to data use practices. In terms of traditional professional development workshops, this is inconsistent with earlier evidence that traditional professional development might promote data use practices (e.g. Reeves, Citation2016; Murnane et al., Citation2005). Teacher participation in other learning mechanisms, specifically more active, reformed mechanisms (i.e. data teams, data coaching, and professional learning communities), was also unrelated to data use practices. These findings are somewhat surprising, given some prior evidence for their promise in supporting teacher data use (e.g. Lachat & Smith, Citation2005; Marsh et al., Citation2015). It is possible that the quality of such learning opportunities as experienced by our study participants relative to those reported in earlier published work might explain this discrepancy. As these methods all rely on teacher collaboration, challenges related to teacher collaboration might have undermined their effectiveness (Wayman & Jimerson, Citation2014), for example. Effects might alternatively be limited due to insufficient dosage and/or lack of support (Achinstein, Citation2002; Kerr et al., Citation2006; Marsh, Citation2012; Wayman & Jimerson, Citation2014). However, the absence of qualitative data on the character of the opportunities as experienced by our sample precludes explication of these null findings.
5.4. Implications, limitations, and future directions
This study contributes research-based knowledge that can, when interpreted in the context of other research as well as theory, potentially inform the development of teacher data use capacity. In the context of a single sample, this study revisited the specific ways in which teachers use data, which should prove helpful for both researchers and other education stakeholders as they target data use reforms in light of the status of data use in 2015. This study also estimates considerable teacher-to-teacher variation in specific data use practices, suggesting a need for mechanisms to better equip and/or support all teachers with respect to these practices. This study also replicates the roles of beliefs in teacher data use practices, which affirms these are worthy targets for leadership, interventions, and reforms aimed at promoting data use (Data Quality Campaign, Citation2014).
The present study also extends this body of literature in being the first to highlight the roles of particular undergraduate and graduate coursework in promoting teacher data use practices. Such data may have implications for policy and practice concerning teacher education program design. While we did not find evidence for the roles of professional learning communities, data teams, and data coaching in supporting data use; such mechanisms have been deemed key components in the promotion of data use in prior research (e.g. Roehrig et al., Citation2008; Wayman & Jimerson, Citation2014). In the context of supporting data use by all teachers, including existing teachers, teacher education for data use will likely necessitate a multi-pronged strategy involving both pre- and in-service teacher learning. In turn, systemic improvements to these learning opportunities should enhance the quality of classroom practice related to data use and in doing so K-12 student achievement.
Nonetheless, these findings should be interpreted in light of this study’s key methodological limitations, which should be addressed through follow-up research. First, the study’s non-probability sampling approach and inclusion of only (primarily white) teachers from Illinois, US, precludes generalization to all teachers, and warrants additional work conducted with more diverse teachers in diverse local and national contexts. Replication of these findings is critical, given the relatively low response rate and potential for sampling error. Second, the study’s statistical models incorporate only a select number of variables as possible predictors of teacher data use and there remains a sizable share of unexplained variance in the final regression model. While one strength of this study is that it compared the relative influences of a variety of factors (contextual, beliefs, and teacher learning mechanisms) using the same sample, other variables deserve exploration as well.
Third, while the score properties of the employed researcher-developed data use and leadership instruments were favorable, additional reliability and validity evidence is required. Especially important is the collection of observational data to provide an external perspective on respondent’s self-reporting of their data use practices. Fourth, in terms of the course-taking variables, as knowledge and skills related to data use may be integrated across pre-service courses, future research might attempt to more directly measure such learning opportunities within teacher preparation programs instead of relying on coursework as proxies for such opportunities. Indeed, some of the course-taking estimates in this study were unintuitively high, possibly due to ideosyncratic teacher interpretation of survey questions (e.g. Wilhelm & Andrews-Larson, Citation2016).
Finally, this study only examined factors related to data use practices in general, and as such does not tease apart factors related to data use for particular purposes. Subsequent studies should examine factors related to particular data use practices (e.g. using data to plan/design lessons or modify instruction or lesson plans). Relatedly, it would also be interesting to understand the nature of the data employed during teachers’ implementation of data-driven decision-making practices. Another possible extension to this body of literature would be to examine nuances in data use-relevant course-taking by teacher type (e.g. elementary, secondary, and/or special education).
Funding
The authors received no direct funding for this research.
Acknowledgments
The authors are most grateful to all of the in-service teachers who participated in the study, those administrators who assisted with recruitment efforts, and three external reviewers for their critical and constructive feedback.
Additional information
Notes on contributors
Todd D. Reeves
Todd D. Reeves, PhD, is an assistant professor of Educational Research and Evaluation at Northern Illinois University. His research addresses problems related to assessment, teacher education and development and educational technology, and problems at the intersections of these domains.
Kelly H. Summers
Kelly H. Summers, PhD, is an assistant professor in the Department of Leadership, Educational Psychology, and Foundations at Northern Illinois University. Her research interests include assessment literacy among school leaders, public school finance, and bullying intervention and prevention in public school settings.
Evan Grove
Evan Grove is an undergraduate student at the University of Minnesota—Twin Cities. He is interested in electrical engineering and robotics.
Notes
1. School was indeterminable for 25 respondents (7.6%). One district was a charter-school district. The sample represented teachers from approximately 2% of Illinois public schools and 6% of Illinois public school districts.
2. The Kaiser-Meyer-Olkin measure of sampling adequacy was .95, and Barlett’s sphericity test was significant (p < .001).
3. The Kaiser–Meyer–Olkin measure of sampling adequacy was .96, and Barlett’s sphericity test was significant (p < .001).
4. While research suggests the role of beliefs in data use, the specific beliefs which support data use practices are less clear, and thus we used empirical model entry selection for these variables.
References
- Achinstein, B. (2002). Conflict amid community: The micropolitics of teacher collaboration. Teachers College Record, 104, 421–455.10.1111/tcre.2002.104.issue-3
- Airola, D., Dunn, K. E., & Garrison, M. (2011). 3D-ME: The validation of the data driven decision-making efficacy questionnaire. Washington, DC: Poster presented at the American Psychological Association Convention.
- Arrington, N. M., & Lu, H. L. (2015). Assessing our students assessing their students: Support and impact of preservice teachers on P-5 student learning. The Teacher Educator, 50, 9–30.10.1080/08878730.2014.976105
- Athanases, S. Z., Bennett, L. H., & Wahleithner, J. M. (2013). Fostering data literacy through preservice teacher inquiry in English language arts. The Teacher Educator, 48, 8–28.10.1080/08878730.2012.740151
- Avramides, K., Hunter, J., Oliver, M., & Luckin, R. (2014). A method for teacher inquiry in cross-curricular projects: Lessons from a case study. British Journal of Educational Technology, doi:10.1111/bjet.12233
- Bandura, A. (1997). Self-efficacy: The exercise of control. New York, NY: Freeman.
- Banilower, E. R., Smith, P. S., Weiss, I. R., Malzahn, K. A., Campbell, K. M., & Weis, A. M. (2013). Report of the 2012 national survey of Science and Mathematics education. Chapel Hill, NC: Horizon Research, Inc.
- Black, P., & Wiliam, D. (1998). Inside the black box: Raising standards through classroom assessment. London: Granada Learning.
- Bocala, C., & Boudett, K. P. (2015). Teacher educators habits of mind for using data wisely. Teachers College Record, 117(4), 1–20.
- Brown, G. T. L. (2006). Teachers’ conceptions of assessment: Validation of an abridged instrument. Psychological Reports, 99, 166–170.
- Brown, G. T., & Remesal, A. (2012). Prospective teachers' conceptions of assessment: A cross-cultural comparison. The Spanish Journal of Psychology, 15, 75–89.10.5209/rev_SJOP.2012.v15.n1.37286
- Carlson, D., Borman, G., & Robinson, M. (2011). A multistate district-level cluster randomized trial of the impact of data-driven reform on reading and mathematics achievement. Educational Evaluation and Policy Analysis, 33, 378–398.10.3102/0162373711412765
- Coburn, C. E., & Turner, E. O. (2011). Research on data use: A framework and analysis. Measurement: Interdisciplinary Research & Perspectives, 9, 173–206.
- Cosner, S. (2011). Teacher learning, instructional considerations and principal communication: Lessons from a longitudinal study of collaborative data use by teachers. Educational Management Administration & Leadership, 39, 568–589.
- Data Quality Campaign. (2013). Data for action 2013. Retrieved from http://www.dataqualitycampaign.org/files/DataForAction2013.pdf
- Data Quality Campaign. (2014). Teacher data literacy: It’s about time. Washington, DC: Author.
- Datnow, A., & Hubbard, L. (2015). Teachers’ use of data to inform instruction: Lessons from the past, prospects for the future. Teachers College Record, 117(4), 1–26.
- DeLuca, C., & Bellara, A. (2013). The current state of assessment education: Aligning policy, standards, and teacher education curriculum. Journal of Teacher Education, 64, 356–372.10.1177/0022487113488144
- Diez, M. E. (2010). It is complicated: Unpacking the flow of teacher education's impact on student learning. Journal of Teacher Education, 61, 441–450.10.1177/0022487110372927
- Dunn, K. E., Airola, D. T., Lo, W. J., & Garrison, M. (2013). What teachers think about what they can do with data: Development and validation of the data driven decision-making efficacy and anxiety inventory. Contemporary Educational Psychology, 38, 87–98.10.1016/j.cedpsych.2012.11.002
- Eaker, R., DuFour, R., & Burnette, R. (2002). Getting started: Reculturing schools to become professional learning communities. Bloomington, IN: National Educational Service.
- Farley-Ripple, E. N., & Buttram, J. L. (2014). Developing collaborative data use through professional learning communities: Early lessons from Delaware. Studies in Educational Evaluation, 42, 41–53.10.1016/j.stueduc.2013.09.006
- Fullan, M. (2001). The new meaning of educational change. New York, NY: Teachers College Press.
- Gallimore, R., Ermeling, B. A.Saunders, W. M., & Goldenberg, C. (2009). Moving the learning of teaching closer to practice: Teacher education implications of school‐based inquiry teams. The Elementary School Journal, 109, 537– 553.
- Gearhart, M., & Osmundson, E. (2009). Assessment portfolios as opportunities for teacher learning. Educational Assessment, 14(1), 1–24.10.1080/10627190902816108
- Gerzon, N. (2015). Structuring professional learning to develop a culture of data use: Aligning knowledge from the field and research findings. Teachers College Record, 117(4), 1–28.
- Goddard, Y., Goddard, R., & Tschannen-Moran, M. (2007). A theoretical and empirical investigation of teacher collaboration for school improvement and student achievement in public elementary schools. Teachers College Record, 109, 877–896.
- Goertz, M. E., Oláh, L. N., & Riggan, M. (2009). From testing to teaching: The use of interim assessments in classroom instruction. Philadelphia, PA: Consortium for Policy Research in Education.
- Greenberg, J., & Walsh, K. (2012). What teacher preparation programs teach about K-12 assessment: A review. New York, NY: National Council on Teacher Quality.
- Greenberg, J., Walsh, K., & McKee, A. (2015). 2014 teacher prep review: A review of the nation’s teacher preparation programs. Washington, DC: National Council on Teacher Quality.
- Hamilton, L., Halverson, R., Jackson, S., Mandinach, E., Supovitz, J., & Wayman, J. (2009). Using student achievement data to support instructional decision making (NCEE 2009-4067). Washington, DC: National Center for Education Evaluation and Regional Assistance, Institute of Education Sciences, U.S. Department of Education.
- Hattie, J., & Timperley, H. (2007). The power of feedback. Review of Educational Research, 77, 81–112.10.3102/003465430298487
- Herman, J., Osmundson, E., Dai, Y., Ringstaff, K., & Timms, M. (2015). Investigating the dynamics of formative assessment: Relationships between teacher knowledge, assessment practice and learning. Assessment in Education: Principles, Policy & Practice, 22, 344–367.
- Hill, H. C., Rowan, B., & Ball, D. L. (2005). Effects of teachers' mathematical knowledge for teaching on student achievement. American Educational Research Journal, 42, 371–406.10.3102/00028312042002371
- Interstate Teacher Assessment and Support Consortium. (2011). InTASC model core teaching standards. Retrieved from http://www.ccsso.org/Resources/Publications/InTASC_Model_Core_Teaching_Standards_2011_MS_Word_Version.html
- Jacobs, J., Gregory, A., Hoppey, D., & Yendol-Hoppey, D. (2009). Data literacy: Understanding teachers' data use in a context of accountability and response to intervention. Action in Teacher Education, 31, 41–55.10.1080/01626620.2009.10463527
- Keaton, P. (2014). Documentation to the NCES common core of data local education agency universe survey: School year 2012–13 provisional version 1a (NCES 2015-008). Washington, DC: National Center for Education Statistics, Institute of Education Sciences, U.S. Department of Education.
- Kerr, K. A., Marsh, J. A., Ikemoto, G. S., Darilek, H., & Barney, H. (2006). Strategies to promote data use for instructional improvement: Actions, outcomes, and lessons from three urban districts. American Journal of Education, 112, 496–520.10.1086/505057
- Lachat, M. A., & Smith, S. (2005). Practices that support data use in urban high schools. Journal of Education for Students Placed at Risk (JESPAR), 10, 333–349.10.1207/s15327671espr1003_7
- Mandinach, E. B., & Gummer, E. S. (2013a). A systemic view of implementing data literacy in educator preparation. Educational Researcher, 42, 30–37.10.3102/0013189X12459803
- Mandinach, E. B., & Gummer, E. S. (2013b). Building educators’ data literacy: Differing perspectives. The Journal of Educational Research & Policy Studies, 13(2), 1–5.
- Mandinach, E. B., Gummer, E. S., & Muller, R. D. (2011). The complexities of integrating data-driven decision making into professional preparation in schools of education: It’s harder than you think. Alexandria, VA, Portland, OR, and Washington, DC: CNA Education, Education Northwest, and WestEd.
- Mandinach, E. B., Friedman, J. M., & Gummer, E. S. (2015). How can schools of education help to build educators’ capacity to use data: A systemic view of the issue. Teachers College Record, 117(4), 1–50.
- Mann, B., & Simon, T. (2010, July). Teaching teachers to use data. Presentation at the NCES STATS-DC 2010 Data Conference, Bethesda, MD.
- Marsh, J. A. (2012). Interventions promoting educators’ use of data: Research insights and gaps. Teachers College Record, 114(11), 1–48.
- Marsh, J. A., Pane, J. F., & Hamilton, L. (2006). Making sense of data-driven decision making in education. Santa Monica, CA: Rand Corporation.
- Marsh, J. A., Bertrand, M., & Huguet, A. (2015). Using data to alter instructional practice: The mediating role of coaches and professional learning communities. Teachers College Record, 117(4), 1–40.
- Mason, S. A. (2003). Learning from data: The role of professional learning communities. Retrieved from http://files.eric.ed.gov/fulltext/ED476852.pdf
- McDougall, D., Saunders, W. M., & Goldenberg, C. (2007). Inside the black box of school reform: Explaining the how and why of change at getting results schools. International Journal of Disability, Development and Education, 54, 51–89.10.1080/10349120601149755
- McMillan, J. H., Myran, S., & Workman, D. (2002). Elementary teachers' classroom assessment and grading practices. The Journal of Educational Research, 95, 203–213.10.1080/00220670209596593
- Means, B., Gallagher, L., & Padilla, C. (2007). Teachers’ use of student data systems to improve instruction. Retrieved from http://files.eric.ed.gov/fulltext/ED501547.pdf
- Means, B., Padilla, C., DeBarger, A., & Bakia, M. (2009). Implementing data-informed decision making in schools: Teacher access, supports and use. Washington, DC: U.S. Department of Education.
- Mertler, C. A. (2009). Teachers’ assessment knowledge and their perceptions of the impact of classroom assessment professional development. Improving Schools, 12, 101–113.10.1177/1365480209105575
- Murnane, R. J., Sharkey, N. S., & Boudett, K. P. (2005). Using student-assessment results to improve instruction: Lessons from a workshop. Journal of Education for Students Placed at Risk (JESPAR), 10, 269–280.10.1207/s15327671espr1003_3
- Nelson, T. H., Slavit, D., & Deuel, A. (2012). Two dimensions of an inquiry stance toward student-learning data. Teachers College Record, 114(8), 1–42.
- Pajares, M. F. (1992). Teachers’ beliefs and educational research: Cleaning up a messy construct. Review of Educational Research, 62, 307–332.10.3102/00346543062003307
- Pashler, H., Bain, P., Bottge, B., Graesser, A., Koedinger, K., McDaniel, M., & Metcalfe, J. (2007). Organizing instruction and study to improve student learning (NCER 2007–2004). Washington, DC: National Center for Education Research, Institute of Education Sciences, U.S. Department of Education.
- Piro, J. S., Dunlap, K., & Shutt, T. (2014). A collaborative Data Chat: Teaching summative assessment data use in pre-service teacher education. Cogent Education, 1(1).
- Preacher, K. J., & Hayes, A. F. (2008). Asymptotic and resampling strategies for assessing and comparing indirect effects in multiple mediator models. Behavior Research Methods, 40, 879–891.10.3758/BRM.40.3.879
- Provasnik, S., KewalRamani, A., Coleman, M. M., Gilbertson, L., Herring, W., & Xie, Q. (2007). Status of education in rural America (NCES 2007–040). Washington, DC: National Center for Education Statistics, Institute of Education Sciences, U.S. Department of Education.
- Qualtrics. (2015). Qualtrics [computer software]. Provo, UT: Author.
- Reeves, T. D., & Honig, S. L. (2015). A classroom assessment data literacy intervention for pre-service teachers. Teaching and Teacher Education, 50, 90–101.
- Reeves, T. D. (2016). Pre-service teachers’ data use opportunities during student teaching. Unpublished manuscript.
- Roehrig, A. D., Duggar, S. W., Moats, L., Glover, M., & Mincey, B. (2008). When teachers work to use progress monitoring data to inform literacy instruction: Identifying potential supports and challenges. Remedial and Special Education, 29, 364–382.10.1177/0741932507314021
- Schildkamp, K., Karbautzki, L., & Vanhoof, J. (2013). Exploring data use practices around Europe: Identifying enablers and barriers. Studies in Educational Evaluation, 42, 15–24.
- Summers, K. H., Reeves, T. D., Schwartz, J., & Walker, D. A. (2015a). Professional development for educational leaders in the era of performance evaluation. School Leadership Review, 10, 33–43.
- Summers, K. H., Reeves, T. D., Schwartz, J., & Walker, D. A. (2015b). Understanding student growth measures: A primer for school business managers. Journal of School Business Management, 27, 22–29.
- Tschannen-Moran, M., Hoy, A., & Hoy, W. K. (1998). Teacher efficacy: Its meaning and measure. Review of Educational Research, 68, 202–248.10.3102/00346543068002202
- Turner, E. O., & Coburn, C. E. (2012). Interventions to promote data use: An introduction. Teachers College Record, 114(11), 1–13.
- Vanhoof, J., & Schildkamp, K. (2014). From ‘professional development for data use’ to ‘data use for professional development’. Studies in Educational Evaluation, 42, 1–4.10.1016/j.stueduc.2014.05.001
- Wayman, J. C., & Jimerson, J. B. (2014). Teacher needs for data-related professional learning. Studies in Educational Evaluation, 42, 25–34.10.1016/j.stueduc.2013.11.001
- Wayman, J. C., Cho, V., & Johnston, M. T. (2007). The data-informed district: A district-wide evaluation of data use in the Natrona County School District. Retrieved from http://edadmin.edb.utexas.edu/datause/Wayman_data_use_evaluation.pdf
- Wayman, J. C., Jimerson, J. B., & Cho, V. (2012). Organizational considerations in establishing the Data-Informed District. School Effectiveness and School Improvement, 23, 159–178.10.1080/09243453.2011.652124
- Wilhelm, A. G., & Andrews-Larson, C. (2016). Why don’t teachers understand our questions? Reconceptualizing teachers’ “misinterpretation” of survey items. AERA Open, 2(2).
- Young, V. M., & Kim, D. H. (2010). Using assessments for instructional improvement: A literature review. Education Policy Analysis Archives, 18(19).