Abstract
Academic self-efficacy is mostly construed as specific; task-specific, course-specific or domain-specific. Previous research in the Danish university context has shown that the self-efficacy subscale in the Motivated Strategies for Leaning Questionnaire is not a single scale, but consists of two separate course- and activity-specific scales; the Specific Academic Learning Self-Efficacy Scale (SAL-SE) and the Specific Academic Exam Self-efficacy scale (SAE-SE). The SAL-SE and the SAE-SE subscales have previously been found to fit the Rasch model, have excellent reliability, and initial evidence of criterion validity has been established. The aim of this study was to conduct a new validity study of the SAL-SE and SAE-SE scales in the Danish university context. Specifically, whether the original findings of fit to the Rasch model, as well as the other psychometric properties of the scales could be replicated for a sample enrolled in another course context, as well as additional criterion validity. The sample consisted of 341 Psychology students enrolled in a first-semester statistics course. Results showed that the SAL-SE scale fit the Rasch model, while the SAE-SE scale did not as two items were locally dependent, reliability was excellent, and both SAL-SE and SAE-SE levels were positively related to final statistics grades.
PUBLIC INTEREST STATEMENT
The research reports further evidence of the construct and criterion validity of the Specific Academic Learning Self-Efficacy Scale (SAL-SE) and the Specific Academic Exam Self-efficacy scale (SAE-SE) for use in the higher education context. Analyses were conducted within the framework of item response theory using Rasch measurement models. These models were chosen for their adherence to strict standards for measurement quality. The results confirm that the SAL-SE and SAE-SE scales are indeed two separate scales, thus confirming the measurement properties, including excellent reliability, of the SAL-SE and SAE-SE scales from the original validity study. Furthermore, the results extend the previous criterion validity evidence as the scales are related to grades in the expected manner. Both scales are of very good quality measurement-wise even though they are short, and their use in contemporary higher education research and practice is warranted.
1. Introduction
Self-efficacy refers to the individual’s belief in his or her own capability to plan and perform actions necessary to attain a certain outcome (Bandura, Citation1997). The impact of self-efficacy on academic outcome has been linked to the attainment of academic outcomes (e.g. Ferla et al., Citation2009; Luszczynska et al., Citation2005; Richardson et al., Citation2012; Zimmerman et al., Citation1992). Meta-analyses have concluded that self-efficacy is the strongest predictor of grade point average (GPA) in tertiary education, even above personality traits, motivation and various learning strategies (Bartimote-Aufflick et al., Citation2016; Richardson et al., Citation2012). Furthermore, students who feel efficacious when learning or performing educational tasks (i.e. are high in academic self-efficacy), have been found to participate more readily, work harder, persist longer when they encounter difficulties, and to achieve at a higher level of academic performance (Schunk & Pajares, Citation2002).
Self-efficacy, and thus academic self-efficacy, is situated within Bandura’s social cognitive theoretical framework, which poses that human achievement depends upon interactions between the person’s behaviours, personal factors such as abilities, beliefs, motivation, and environmental conditions (Bandura, Citation1997). Thus, situational factors are central for the person’s feelings of academic self-efficaciousness, and a person can be more or less efficacious in relation to very specific tasks, particular situations or domains of academic functioning (Bandura, Citation1977; Citation1997; Richardson et al., Citation2012). In addition, it has been argued that the various and numerous cases of failure or success experienced over time in different academic domains facilitates assessment of a general sense of self-efficacy, which refers to a global ability to master challenges (e.g. Scholz et al., Citation2002; Schwarzer & Jerusalem, Citation1995), while more specific self-efficacy beliefs have been found to account for the connections between general efficacy beliefs and particular performance (e.g. Agarwal et al., Citation2000; Bandura, Citation1997; Pond & Hay, Citation1989). Thus, in the field there appears to be agreement that the varying demands through education imply that the individual’s notion of academic self-efficacy will vary depending on the specific educational context and the point in time in the course of education, whether academic self-efficacy is defined as task-specific, domain-specific or somewhere in between. Thus, a student might be very efficacious at one time-point during the course of education, but less so at a later time-point, or a student might feel more efficacious in relation to specific courses or tasks within courses, but not towards other courses or tasks.
Many academic self-efficacy scales and subscales in larger instruments, which are specific in various ways have been developed; e.g. the domain-specific Internet self-efficacy scale (Chuang et al., Citation2015), the somewhat task-specific self-efficacy in problem-solving and communication scale (Aguirre et al., Citation2012), the course-specific self-efficacy scale in the Motivated Strategies for Learning Questionnaire (MSLQ; Pintrich et al., Citation1991), and the both course- and task-specific learning exam self-efficacy subscales derived from the MSLQ self-efficacy scale (Nielsen et al., Citation2017).
Nielsen et al. (Citation2017) proposed the Specific Academic Learning Self-efficacy (SAL-SE) and the Specific Academic Exam Self-Efficacy (SAE-SE) scales as two separate scales derived from the self-efficacy scale of the MSLQ (Pintrich et al., Citation1991). The SAL-SE and the SAE-SE subscales each consisted of four items from the MSLQ self-efficacy scale, with a slightly modified response scale. Nielsen et al. (Citation2017) showed that both the SAL-SE and the SAE-SE scale each fit a unidimensional Rasch model, while the total eight items did not make up a unidimensional scale, and both of the scales were measurement invariant (i.e. free of DIF) relative to age, gender, course targeted, university and admission criteria. Furthermore, they showed that both the SAL-SE and the SAE-SE scale had excellent reliability, i.e. high enough for individual assessment, as was the intention of the MSLQ. Finally, they showed good criterion validity, as differences in students’ SAL-SE and SAE-SE scores varied in somewhat expected and certainly in explainable ways dependent on the criteria that the students had been admitted to university on. The original validation study of Nielsen et al. (Citation2017) used three samples of psychology students enrolled in three different psychology subject courses (i.e. biological psychology, personality psychology, and industrial & organisational psychology) from two Danish universities. A first sample was used for construct and criterion validity (i.e. item analysis by Rasch models and differences in scores), while a second and third sample was used to replicate the criterion validity analysis. Thus, while the initial validation study demonstrated excellent psychometric properties of the SAL-SE and the SAE-SE scales with one sample of students, this is not in itself sufficient evidence of construct validity, and further studies are needed.
1.1. Building evidence of validity through replication and further studies
Neither validity nor reliability are universal attributes of measurement scales. A key feature of reliability is that it is inherently sample dependent, and should thus be assessed in every study employing the scales. For example, it might be that reliability of the SAL-SE and the SAE-SE scales is very good for one student sample as in Nielsen et al. (Citation2017) study due to the variability between students introduced by the three different psychology subject courses targeted in that study, but that reliability is less good when a sample is made up of students attending more similar courses or a single course and so on. A key feature of validity is that it is purpose dependent, and thus validity, or rather the building of evidence of the validity of scales, is an ongoing process, where evidence of or against validity is continuously collected with different target groups and purposes, with different criterion variables and so on (within the scope of the instrument, naturally). For example, even if the original validity study by Nielsen et al. (Citation2017) showed that the SAL-SE and SAE-SE scale were separate and unidimensional constructs and that criterion validity was good as differences in these scores were dependent on admission method and university, this is not sufficient evidence of the validity of the SAL-SE and SAE-SE constructs. Construct validity should be investigated for other groups of higher education students, and criterion validity should be investigated using other criteria.
1.2. The current study
The aims of the study were: 1) to conduct a second investigation of construct validity and psychometric properties of the Danish Specific Academic Learning Self-Efficacy (SAL-SE) and the Specific Academic Exam Self-Efficacy (SAE-SE) scales, using Rasch measurement models to evaluate whether the results from the initial validation study (Nielsen et al., Citation2017) could be replicated with a sample of psychology students assessing their self-efficacy in relation to statistics as a tool subject. 2) To investigate further the criterion validity of the SAL-SE and SAE-SE scales by investigating their relationship with grade outcome. To fulfil these aims, the following research questions were investigated:
RQ1: Are the SAL-SE and SAE-SE scales measurement invariant (i.e. free of DIF) across student subgroups defined by gender, age, year cohort, perceived adequacy of mathematics ability, and expectation of future work including statistics?
RQ2: Do the SAL-SE and SAE-SE scales each fit a Rasch measurement model?
RQ3: Are the SAL-SE and the SAE-SE scales well-targeted for the study population, and is reliability sufficient for individual assessment?
RQ4: Are the SAL-SE and SAE-SE scales separate unidimensional scales as proposed by Nielsen et al. (Citation2017) or a single overarching self-efficacy scale as proposed by Pintrich et al. (Citation1991)?
RQ5: Are the students’ sense of learning and exam self-efficaciousness in relation to statistics at the start of the first semester positively related to their statistics grades obtained after two semesters of classes?
2. Methods
2.1. Participants and data collection
Data were collected in one Danish university in statistics classes for students enrolled in the Bachelor of psychology degree program. Data were collected in the fifth statistics lecture with paper-pencil completed questionnaires in the first semester (i.e. 1 month into the BA psychology program) in 2-year cohorts of psychology students. Students had been informed of the data collection by the statistics lecturer beforehand, and the lecturer had allowed time for this within the lecture. In the lecture students were informed in detail of the purpose of the data collection, how data would be utilized, that participation was voluntary, and they were provided with an information sheet which included contact information for the responsible researcher. In the questionnaire students actively consented to use of their data for research and were given the opportunity to also allow their data to be utilized by the statistics lecturer for exercises after proper anonymization and publication of research.
From the two cohorts 169 and 172 students chose to participate, this corresponded to 76,8 percent and 78,9 percent of the two cohorts, respectively. The distribution of gender and age is provided for each cohort and the total study sample in Table .
Table 1. Gender and age distributions in the two cohorts of the study sample
All SAL-SE and SAE-SE items were administered in the same relative order as in the original MSLQ (Pintrich et al., Citation1991), but mixed in with items from the Intrinsic Motivation and Extrinsic Motivation scales of the MSLQ, which are not utilized in the current study.
Across the two cohorts 253 students gave permission to obtain their grades from the university administration and supplied their students ID for this purpose.
2.2. Instruments
The Specific Academic Learning Self-efficacy (SAL-SE) and the Specific Exam Self-efficacy (SAE-SE) scales were adapted from the self-efficacy scale in the Motivated Strategies of Learning Questionnaire (MSLQ; Pintrich et al., Citation1991) by Nielsen et al. (Citation2017). The SAL-SE and the SAE-SE scale each consist of four items (See Nielsen et al., Citation2017), and are intended to measure course-specific academic learning and exam self-efficacy, respectively. Nielsen et al. (Citation2017) showed the SAL-SE and SAE-SE subscales, with an adapted response scale, to have excellent measurement properties, as they each fit Rasch models for samples of Danish Psychology students, and their reliabilities were very close to what is required for the individual assessment intended with the MSLQ (i.e. 0.87 and 0.89). The adapted response scale had five response categories, all with meaning anchors, as opposed to the seven partially anchored categories in the original MSLQ (Nielsen et al., Citation2017; Pintrich et al., Citation1991)
2.3. Rasch measurement models
The Rasch model (RM; Rasch, Citation1960) is a measurement model, within the Item Response Theory (IRT) framework, with particularly desirable properties (Fischer & Molenaar, Citation1995). It is in mathematical terms parsimonious, while at the same time allowing independent investigation of the item and person parameters, as well as the relationship between these parameters (Kreiner, Citation2007). If a scale fits the RM, the sum score is a sufficient statistic for the person parameter estimates from the model (i.e. all necessary information is obtained with the sum score), a property unique to scales fitting the RM (Kreiner, Citation2013). Sufficiency is an attractive property, particularly so with scales were the sum score is used for research and assessment, as it is the case with the SAL-SE and the SAE-SE scales. The requirements for fit to the Rasch model are (Kreiner, Citation2013):
Unidimensionality: The items of the scale assess one single underlying latent construct. In this case; the SAL-SE scale assesses one construct and the SAE-SE scale assessed another.
Monotonicity: The expected item scores increase with increasing values of the latent variable. In this case; the probability of any of the statements in the items providing a good description will increase with increasing self-efficacy scores.
Local independence of items (no local dependence; no LD): The response to a single item should be conditionally independent from the response to another item of the scale given the latent variable. In this case; responses to any one self-efficacy item should only depend on the level of self-efficacy, and not also on responses to the other items.
Absence of differential item functioning (no DIF): Items and exogenous (i.e. background variables) should be conditionally independent given the latent variable. In this case; responses to any one self-efficacy item should only depend on the level of self-efficacy, and not also on subgroup membership as for example,, gender or the university students are enrolled in.
Homogeneity: The rank order of the item parameters (i.e. the item difficulties) should be the same across all persons regardless of their level on the latent variable. In this case; the item that requires the least self-efficacy to be endorsed should be the same for all students no matter if they are little or very self-efficacious, and the same for the item requiring the second-lowest self-efficacy, and so on for all items.
The first four requirements adhere to all IRT models and provide criterion-related construct validity as defined by Rosenbaum (Citation1989), while homogeneity is only a requirement of the Rasch model.
Departures from the RM in the form of DIF or LD are often found in psychological non-ability measurement scales. Thus, even though the Danish version of the SAL-SE and the SAE-SE scales were found to fit Rasch models, this would not necessarily be the case with the student sample in the present study. If the only departures from the RM in a scale were in the form of uniform LD and/or DIF (i.e. the same at all levels of the latent variable), I employed graphical loglinear Rasch Models (GLLRM; Kreiner & Christensen, Citation2002; Citation2004; Citation2007) to overcome this. In the GLLRM the LD and DIF terms are added as interactions terms in the model, and the same test of fit as for the RM can then be used to test fit to this more complex model. GLLRMs retain most of the desirable properties of the RM once the departures of LD or DIF are taken into account, and the sum score will remain a sufficient statistic if the score is appropriately adjusted for any DIF included in the model (Kreiner & Christensen, Citation2007).
In the present study, the Partial Credit model (PCM; Masters, Citation1982) was used, as it is a generalization of the dichotomous Rasch model to take ordinal items, it provides the same measurement properties as the dichotomous Rasch model (Mesbah & Kreiner, Citation2013), and it extends to graphical loglinear Rasch models as well (Kreiner, Citation2007).
2.3.1. Item analysis by Rasch models
In order to test each of the scales rigorously against the Rasch model requirements (RQ1-RQ4), I included the following in the analysis. As the analysis is an iterative process of attempting to discover evidence against the model, these are not ordered steps and some are conducted more than once:
Overall test of homogeneity of item parameters across low and high scoring groups.
Overall tests of no differential item functioning (no DIF) in relation to Cohort (1, 2), Perceived adequacy of mathematics ability (more than adequate, adequate, less than adequate), Expectation to work with statistics in the future (yes, maybe, no), Gender (male, female), Age group (20 years and younger, 21 years, 22 years and older).
Tests of no DIF for all single items relative to the background variables listed above.
Tests of local independence for all item pairs.
Fit of the individual items to the RM.
In the case of evidence of local dependence between items or DIF, interaction terms were added to the model and the resulting GLLRM was tested with the above tests.
After resolving the final model for each of the scales, the following steps were taken:
Assessment of targeting and reliability of each scale.
Test of unidimensionality across the two resulting scales.
The fit of individual items to the RM was tested by comparing the observed item-rest-score correlations with the expected item-restscore correlations under the model (Kreiner & Christensen, Citation2004). Overall tests of fit to the RM (i.e. tests of global homogeneity by comparison of item parameters in low and high scoring groups, and global tests of no DIF) were conducted using Andersen conditional likelihood ratio test (CLR; Andersen, Citation1973). The local independence of items and absence of DIF was tested using Kelderman’s (Citation1984) likelihood-ratio test, and if evidence against these assumptions were discovered the magnitude of the local dependence of items and/or DIF was informed by partial Goodman-Kruskal gamma coefficients conditional on the restscores (Kreiner & Christensen, Citation2004).
Reliability was calculated with Hamon and Mesbah (Citation2002) Monte Carlo method, which takes into account any local dependence between items in a GLLRM. Targeting was assessed numerically with two indices (Kreiner & Christensen, Citation2013): the test information target index (the mean test information divided by the maximum test information) and the root mean squared error target index (the minimum standard error of measurement divided by the mean standard error of measurement). Both indices should have a value close to one. I also estimated the target of the observed score and the standard error of measurement of the observed score. Lastly, to provide a graphical illustration of targeting and test information, I plotted item maps showing the distribution of the person locations against the item locations, with the inclusion of the information curve. Person location were plotted as weighted maximum likelihood estimates of the person parameters (i.e. the latent scores) and person parameter estimates assuming a normal distribution (i.e. the theoretical distribution). Item locations were plotted as item thresholds.
Unidimensionality across the SAL-SE and SAE-SE scales was tested by comparing the observed gamma (γ) correlation of the scales with the expected γ correlation of the scales under the unidimensional model (Horton et al., Citation2013). Two scales measuring different constructs will be significantly weaker correlated than what is expected under the common unidimensional model. This was done after having established fit to the RM and GLLRM, respectively, as unrecognized local dependence between items within the scales could result in spurious evidence against unidimensionality across the two scales.
All the test statistics effectively tests whether item response data comply with the expectations of the model; thus, the results are all evaluated in the same manner; significant p-values signify evidence against the model. In line with the recommendations by Cox et al. (Citation1977), we evaluated p-values as a continuous measure of evidence against the null, distinguishing between weak (p < 0.05), moderate (p < 0.01), and strong (p < 0.001) evidence against the model, rather than applying a deterministic critical limit of 5% for p-values. Furthermore, I used the Benjamini and Hochberg (Citation1995) procedure to adjust for false discovery rate (FDR) due to multiple testing, in order to reduce false evidence against the model created by the many tests conducted (i.e. reduce type I errors), whenever appropriate.
The full sample of 341 students was used for all item analyses by Rasch models.
2.4. Criterion validity
To investigate whether the students’ sense of learning and exam self-efficacy in relation statistics were positively related to obtained grades (RQ5) the SAL-SE and the SAE-SE scores were each categorized into low (4–9), medium (10–14) and high (15–20) scores. The mean grades obtained by students in the three score groups (SAL-SE as well as SAE-SE) were then compared using t-tests, again with an adjustment of critical values for multiple testing (Benjamini & Hochberg, Citation1995). The reduced sample of 253 students with information on grades was used for this part of the study.
2.5. Software
All item analysis was conducted with the Digram software package (Kreiner, Citation2003; Kreiner & Nielsen, Citation2013), while the item maps were plotted in R. Criterion validity analysis was conducted in SPSS version 26.
3. Results
The Specific Academic Learning Self-Efficacy (SAL-SE) scale fitted the RM, while the Specific Academic Exam Self-Efficacy (SAE-SE) scale fitted a GLLRM with two locally dependent items. Thus, for the SAL-SE scale, there was no evidence against homogeneity of the item parameters for high and low scorers, no global evidence of DIF relative to student cohort, students’ perceived adequacy of mathematics ability, students’ expectation to work with statistics in the future, gender or age (Table , SAL-SE column), nor any evidence of DIF when testing at the single item level (Supplemental file Table S3, SAL-SE part). There was also no evidence against fit of the single SAL-SE items to the RM, as the observed item-restscore correlations did not differ from the correlations expected under the model (Table , SAL-SE column), nor any evidence against local independence of the SAL-SE items (Supplemental file Table S4, SAL-SE part).
Table 2. Global tests of homogeneity and differential item function for the Specific Academic Learning Self-Efficacy under the Rasch model and the Specific Academic Exam Self-Efficacy scales under the graphical loglinear Rasch model
Table 3. Item fit statistics for the Specific Academic Learning Self-Efficacy under the Rasch model and the Specific Academic Exam Self-Efficacy subscales under the graphical loglinear Rasch model
Initially there was also no evidence against fit to the RM of the SAE-SE items, as again there was no evidence against homogeneity or any global evidence of DIF (Supplemental file Table S1), nor any evidence against item fit (Supplemental file Table S2), and finally no evidence of DIF when testing at the item level (Supplemental file Table S3, SAE-SE part). There was, however, weak evidence against local independence of SAE-SE items 2 and 3 (Supplemental file Table 4, SAE-SE part). When an interaction term between these two SAE-SE items was included in the model, effectively turning it into a GLLRM without DIF, no further evidence against the model was discovered; thus, Tables and (SAE-SE parts) relay the fit statistics for the final SAE-SE model.
3.1. Item difficulties
The item difficulties (i.e. the likelihood to endorse items) for the individual items of the SAL-SE and the SAE-SE scales are both within a range of just about four logits on the respective latent scales; from approximately −2.5 to just be 2.0 (Figure ). Thus, both of the two scales contained items, which were easy to endorse (i.e. demanded a low level of self-efficacy) or quite difficult to endorse (i.e. demanded a high level of self-efficacy).
In the SAE-SE scale item 1 (I believe I will receive an excellent grade in this class) was the hardest to endorse in terms of level of specific academic exam self-efficacy, while item 4 (Considering the difficulty of this course, the teacher, and my skills, I think I will do well in this class) was the easiest in terms of specific academic exam self-efficacy.
For the SAL-SE scale, the hardest to endorse was 1 (I’m certain I can understand the most difficult material presented in the readings for this course), while the easiest to endorse was item 2 (I’m confident I can understand the basic concepts taught in this course).
3.2. Targeting and reliability
The targeting of both the SAE-SE and the SAL-SE scale was good, with between 72% and 97% of the maximum information obtained on average (Table ). The item maps, in Figure , illustrate that both person estimates and item thresholds are spread out along quite wide intervals of the respective latent scales. It is also illustrated that even though the point of maximum information is located towards the lower end of both scales, information is nearly as high in the interval where most persons are located (± 5). The reliability of both the SAL-SE and the SAE-SE scale was excellent; .88 and .92, respectively.
Table 4. Targeting and reliability of the Specific Academic Learning Self-Efficacy and the Specific Academic Exam Self-Efficacy scales
Figure 2. Item maps for the Specific Academic Learning Self-Efficacy (left) and the Specific Academic Exam Self-Efficacy (right) scales
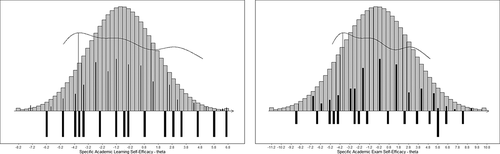
3.3. Unidimensionality
I formally tested whether the SAL-SE and SAE-SE scales made up a single unidimensional self-efficacy scale. The observed correlation between the SAL-SE and the SAE-SE scales was weaker than the expected correlation under a unidimensional model (γobserved = 0.708, γexpected = 0.791, SE = 0.019, p < 0.0001); thus, unidimensionality across the SAL-SE and the SAE-SE scale were clearly rejected, and they are to be regarded as two separate scales measuring qualitatively different self-efficacy constructs.
3.4. Criterion validity
As expected, there was a positive relationship between the SAL-SE and SAE-SE scores at the start of the first semester of statistics classes and the mean grades obtained after two semesters of statistics classes (Table ). Thus, the mean grades for students who were low, medium and high in SAL-SE or SAE-SE were not only significantly different, but also displaying the expected rising pattern in grades with rising levels of self-efficacy. Table S5 in the supplemental file contains a description of the Danish grading scale.
Table 5. Mean statistics grades for student with different levels of Specific Academic Learning Self-Efficacy and Specific Academic Exam Self-Efficacy (n = 253)
4. Discussion, implications and future research
The aim of the study was to conduct a second investigation of construct validity and psychometric properties of the Danish Specific Academic Learning Self-Efficacy (SAL-SE) and the Specific Academic Exam Self-Efficacy (SAE-SE) scales, using Rasch measurement models to evaluate whether the results from the initial validation study (Nielsen et al., Citation2017) could be replicated with a sample of psychology students assessing their self-efficacy in relation to statistics as a tool subject. Nielsen et al. (Citation2017), who proposed the scales, showed that these each fitted Rasch models with several two separate samples of Danish students in three different psychology subject courses. The replication of results was successful, as the present study found the SAL-SE scale to also fit the Rasch model, while weak evidence of moderate local dependence between two items was found for the SAE-SE scale, which subsequently was found to fit a graphical loglinear Rasch model taking this local dependence into account.
4.1. Local dependence between items
The local dependence between two of the SAE-SE items was moderate in magnitude (γ = 0.18), and the statistical evidence of its presence was weak (p just below .05). As this is the only discrepancy in the current findings when comparing them to the original validity study by Nielsen et al. (Citation2017), it has to be taken into account that one of the two studies might include a statistical error. When a lot of tests are conducted, errors will occur, and even though a strong method was used to adjust p-values for false discovery rate in both studies, it might very well be that an error is present in one of the studies. However, it is not possible to determine whether it was the finding that the SAE-SE items were locally independent in the original validity study or the finding that two of the SAE-SE items were not locally independent in the current study might be the erroneous result, if one of them are. What is beyond discussion though, is that the effect of the local dependence on the reliability is non-existent. The reliability estimated with the method taking into account local dependencies between items in the SAE-SE scale was 0.92. Cronbach’s alpha, which assumes that items are locally independent (i.e. this would in this case equal fit to the RM as the local dependence was the only departure), was also 0.92. Furthermore, this is not substantially different from the reliability of 0.89 reported by Nielsen et al. (Citation2017) for the SAE-SE scale fitting the RM.
4.2. Reliability and targeting
Reliability of both the SAL-SE and the SAE-SE scale was adequate for individual assessment for this study sample of psychology students in statistics courses within the psychology Bachelor program. This is congruent with the findings in the original validation study of the SAL-SE and SAE-SE scales by Nielsen et al. (Citation2017) for their sample of bachelor psychology students in three different psychology subject courses, where reliabilities were also around 0.90. Furthermore, both the present study and Nielsen et al.’s previous study thus report reliabilities very close to the reliability of 0.93 reported by Pintrich et al. (Citation1991), when they developed the 8-item self-efficacy scale, which was shown to consist of the two separate scale; SAL-SE and SAE-SE by Nielsen et al. It is noteworthy that even with half the number of items in each scale and five rather than seven response categories the same level of reliability is reached for the separate SAL-SE and SAE-SE scales, and this is now reported in two separate studies for psychology students in subject-wise very different courses.
Targeting (i.e. the degree to which items provide information in the area of the scale where the sample population is located) was good for both scales in the present study; with the best targeting found for the SAL-SE scale. Thus, the targeting of both scales were better for the sample of psychology students in statistics classes in the present study compared to the sample of psychology students in psychology subject classes reported by Nielsen et al. (Citation2017), where the targeting of the SAL-SE scale was good and the targeting of the SAE-SE scale moderate. The current results concerning targeting are not comparable to additional studies, as targeting of the SAL-SE and SAE-SE scales has previously only been investigated by Nielsen et al. (Citation2017).
Further validity studies of the SAL-SE and SAE-SE scales utilizing samples of students from additional academic disciplines and other courses would add substantially to the knowledge of how well these scales are targeted to other student groups and whether the level of precision required for individual assessment is also present for other student groups.
4.3. Item difficulties
A noteworthy finding in the present study is the rank order of the item difficulties (i.e. the likelihood to endorse an item in terms of level on the latent construct) of the SAL-SE and the SAE-SE items. This was identical to the rank order of item difficulties in the original study by Nielsen et al. (Citation2017) for both scales’ items. This is noteworthy, because the study by Nielsen et al. included psychology students rating their self-efficacy in relation to personality psychology, biological psychology, and industrial psychology, while the present study exclusively included psychology students rating their self-efficacy in relation to statistics classes. The magnitude of the individual item difficulties of the present study differed slightly from those reported by Nielsen et al. (Citation2017). The biggest difference was due to SAE-SE items 2 & 3 being locally dependent in the present study and thus having a single common difficulty. In both scales the hardest item to endorse (i.e. SAE-SE1: I believe I will receive an excellent grade in this class and SAL-SE1: I’m certain I can understand the most difficult material presented in the readings for this course) was harder to endorse for the students in statistics classes in the present study than they each were for students in the mixed psychology-oriented classes in the study by Nielsen et al. in 2017. With regard to the items that were the easiest to endorse, the picture was slightly more complex. Thus, the SAE-SE4 item (Considering the difficulty of this course, the teacher, and my skills, I think I will do well in this class) was equally easy to endorse for the psychology students in statistics classes in the present study and for the sample of psychology students in various psychology courses in the 2017 study (Nielsen et al., Citation2017). The SAL-SE2 item (I’m confident I can understand the basic concepts taught in this course) was comparably harder to endorse for the students in statistics classes in the present study than for the students in the subject courses in the 2017 study (to endorse for the students in the 2017 study (Nielsen et al., Citation2017). Considering that Danish psychology students in general find statistics a difficult subject in which only a few have special interest, these findings are not surprising.
It thus appears that the sense of which parts of learning and exam related activities psychology students feel most efficacious towards might be the same (i.e. universal) across subject courses, even if there is some variation in the magnitude of efficaciousness needed to endorse the various activities across subject courses (i.e. context bound). This is certainly a matter for future studies to explore further, for example, by including psychology students from a wider selection of subject courses.
4.4. Criterion validity
In the current study, the expected pattern of higher statistics grades at the end of two semesters of statistics classes with higher levels of both SAL-SE and SAE-SE at the start of the first semester was confirmed. This is in line with previous research, which has shown that self-efficacy is positively related to academic achievement (e.g. Bartimote-Aufflick et al., Citation2016; Richardson et al., Citation2012; Schunk & Pajares, Citation2002). Criterion validity of the SAL-SE and SAE-SE scales had only previously been investigated for psychology students in the original validity study by Nielsen et al. (Citation2017). They found that the difference in SAL-SE and SAE-SE scores of students in relation to various subject classes within the psychology program varied with the criteria on which the students had been admitted to the program. Thus, with the current study, the SAL-SE and SAE-SE constructs have been shown to be related both to the admission criteria and to specific course outcome. In order to investigate these relationships further, an obvious suggestion is thus to conduct studies on the longitudinal relationship between admission criteria/methods, SAL-SE and SAE-SE in courses at different timepoint in a degree program, and the obtained grades in these programs.
5. Conclusion
The present work strengthens the evidence of both construct and criterion validity of the Specific Academic Learning Self-efficacy and the Specific Academic Exam Self-Efficacy scales, by confirming fit to Rasch model with a new student sample, and by expanding the criterion validity evidence. Furthermore, the excellent reliability reported in the original validity study is also confirmed in the current study. The research contributes to existing self-efficacy research and educational practice as it confirms that the self-efficacy scale from the motivated strategies of learning questionnaire should not be used as a single scale, but as the two scales mentioned. Thus, the present research has the capacity to change how self-efficacy is measured, both for higher education research and in educational practice for individual assessment and feedback.
Supplemental Material
Download PDF (219.7 KB)Acknowledgements
I would like to thank all the students for participating and their teacher for providing the opportunities for data collection. I also want to acknowledge that the work was mostly done at the University of Copenhagen.
Supplementary material
Supplemental data for this article can be accessed here.
Additional information
Funding
Notes on contributors
Tine Nielsen
Tine Nielsen is an Associate Professor at the Department of Applied Research in Education and Social Sciences, UCL University College, Odense, Denmark. Her research is focused within the fields of higher education psychology, applied educational research, and psychometrics applied to instruments with relevance for education. The present study is one in a series of validity studies aimed at providing the Danish educational research community with brief valid and reliable measures, which can also be used cross-culturally. She is an active member of several education as well as psychometric societies and network groups. For example: the European Association for Research in Learning and Instruction (EARLI), The European Rasch Research and Teaching Groups (ERRTG), and the Danish Measurement network (DM) which she founded in 2016. She is a subject editor (higher education and psychometrics) at the Scandinavian Journal of Educational Research.
References
- Agarwal, R., Sambamurthy, V., & Stair, R. M. (2000). Research report: The evolving relationship between general and specific computer self-efficacy – An empirical assessment. Information Systems Research, 11(4), 418–15. https://doi.org/10.1287/isre.11.4.418.11876
- Aguirre, J. F., Muñoz, F., De Rueda, B., & Blanco, J. R. (2012). Composición factorial de una escala de autoeficacia en la solución de problemas y comunicación en universitarios de ciencias sociales. Formación Universitaria, 5(5), 27–38. https://doi.org/10.4067/S0718-50062012000500004
- Andersen, E. B. (1973). A goodness of fit test for the Rasch model. Psychometrika, 38(1), 123–140. https://doi.org/10.1007/BF02291180
- Bandura, A. (1977). Self-efficacy: Toward a unifying theory of behavioral change. Psychological Review, 84(2), 191–215. https://doi.org/10.1037/0033-295X.84.2.191
- Bandura, A. (1997). Self-efficacy. The exercise of control. Freeman. https://doi.org/10.5860/CHOICE.35-1826
- Bartimote-Aufflick, K., Bridgeman, A., Walker, R., Sharma, M., & Smith, L. (2016). The study, evaluation, and improvement of university student self-efficacy. Studies in Higher Education, 41(11), 1918–1942. https://doi.org/10.1080/03075079.2014.999319
- Benjamini, Y., & Hochberg, Y. (1995). Controlling the false discovery rate: A practical and powerful approach to multiple testing. Journal of the Royal Statistical Society. Series B (Methodological), 57(1), 289–300. http://www.jstor.org/stable/2346101
- Chuang, S.-C., Lin, F.-M., & Tsai, -C.-C. (2015). An exploration of the relationship between Internet self-efficacy and sources of Internet self-efficacy among Taiwanese university students. Computers in Human Behavior, 48, 147–155. https://doi.org/10.1016/j.chb.2015.01.044
- Cox, D. R., Spjøtvoll, E., Johansen, S., van Zwet, W. R., Bithell, J. F., Barndorff-Nielsen, O., & Keuls, M. (1977). The role of significance tests [with discussion and reply]. Scandinavian Journal of Statistics, 4(2), 49–70. http://www.jstor.org/stable/4615652
- Ferla, J., Valcke, M., & Cai, Y. (2009). Academic self-efficacy and academic self-concept: Reconsidering structural relationships. Learning and Individual Differences, 19(4), 499–505. https://doi.org/10.1016/j.lindif.2009.05.004
- Fischer, G. H., & Molenaar, I. W. (Eds.) (1995). Rasch models – Foundations, recent developments, and applications. Springer-Verlag.
- Hamon, A., & Mesbah, M. (2002). Questionnaire reliability under the Rasch Model. In M. Mesbah, B. F. Cole, & M. L. T. Lee (Eds.), Statistical methods for quality of life studies. Design, measurement and analysis (pp. 155–168). Kluwer Academic Publishers. https://doi.org/10.1007/978-1-4757-36250_13
- Horton, M., Marais, I., & Christensen, K. B. (2013). Dimensionality. In K. B. Christensen, S. Kreiner, & M. Mesbah (Eds.), Rasch models health (pp. 137–158). ISTE and John Wiley & Sons, Inc. https://doi.org/10.1002/9781118574454.ch9
- Kelderman, H. (1984). Loglinear Rasch model tests. Psychometrika, 49(2), 223–245. https://doi.org/10.1007/BF02294174
- Kreiner, S. (2003). Introduction to DIGRAM. Department of Biostatistics, University of Copenhagen.
- Kreiner, S. (2007). Validity and objectivity: Reflections on the role and nature of Rasch models. Nordic Psychology, 59(3), 268–298. https://doi.org/10.1027/1901-2276.59.3.268
- Kreiner, S. (2013). The Rasch model for dichotomous items. In K. B. Christensen, S. Kreiner, & M. Mesbah (Eds.), Rasch models health (pp. 5–26). ISTE and John Wiley & Sons, Inc. https://doi.org/10.1002/9781118574454.ch1
- Kreiner, S., & Christensen, K. B. (2002). Graphical Rasch models. In M. Mesbah, B. F. Cole, & M.-L. T. Lee (Eds.), Statistical methods for quality of life studies: Design, measurements and analysis (pp. 187–203). Springer US. https://doi.org/10.1007/978-1-4757-3625-0_15
- Kreiner, S., & Christensen, K. B. (2004). Analysis of local dependence and multidimensionality in graphical loglinear Rasch models. Communication in Statistics –theory and Methods, 33(6), 1239–1276. https://doi.org/10.1081/sta-120030148
- Kreiner, S., & Christensen, K. B. (2007). Validity and objectivity in health-related scales: Analysis by graphical loglinear Rasch models. In von Davier & Carstensen (Eds.), Multivariate and mixture distribution Rasch models (pp. 329–346). Springer. https://doi.org/10.1007/978-0-387-49839-3_21
- Kreiner, S., & Christensen, K. B. (2013). Person parameter estimation and measurement in Rasch models. In K. B. Christensen, S. Kreiner, & M. Mesbah (Eds.), Rasch models health (pp. 63–78). ISTE and John Wiley & Sons, Inc. https://doi.org/10.1002/9781118574454.ch4
- Kreiner, S., & Nielsen, T. (2013). Item analysis in DIGRAM 3.04. Part I: Guided tours (Research report 2013/06). University of Copenhagen, Department of Public Health.
- Luszczynska, A., Gutiérrez-Doña, B., & Schwarzer, R. (2005). General self-efficacy in various domains of human functioning: Evidence from five countries. International Journal of Psychology, 40(2), 80–89. https://doi.org/10.1080/00207590444000041
- Masters, G. N. (1982). A Rasch model for partial credit scoring. Psychometrika, 47(2), 149–174. https://doi.org/10.1007/BF02296272
- Mesbah, M., & Kreiner, S. (2013). The Rasch model for ordered polytomous items. In K. B. Christensen, S. Kreiner, & M. Mesbah (Eds.), Rasch models health (pp. 27–42). ISTE and John Wiley & Sons, Inc. https://doi.org/10.1002/9781118574454.ch2
- Nielsen, T., Makransky, G., Vang, M. L., & Dammeyer, J. (2017). How specific is specific self-efficacy? A construct validity study using Rasch measurement models. Studies in Educational Evaluation, 57, 87–97. https://doi.org/10.1016/j.stueduc.2017.04.003
- Pintrich, P. R., Smith, D. A. F., Garcia, T., & McKeachie, W. J. (1991). A manual for the use of the Motivated Strategies for Learning Questionnaire (MSLQ) (Technical Report No. 91-8-004). The Regents of the University of Michigan.
- Pond, S. B., & Hay, M. S. (1989). The impact of task preview information as a function of recipient self-efficacy. Journal of Vocational Behavior, 35(1), 17–29. https://doi.org/10.1016/0001-8791(89)90045-6
- Rasch, G. (1960). Probabilistic models for some intelligence and attainment tests. Danish Institute for Educational Research.
- Richardson, M., Abraham, C., & Bond, R. (2012). Psychological correlates of university students’ academic performance: A systematic review and meta-analysis. Psychological Bulletin, 138(2), 353. https://doi.org/10.1037/a0026838
- Rosenbaum, P. R. (1989). Criterion-related construct validity. Psychometrika, 54(4), 625–633. https://doi.org/10.1007/BF02296400
- Scholz, U., Doña, B. G., Sud, S., & Schwarzer, R. (2002). Is general self-efficacy a universal construct?1. European Journal of Psychological Assessment, 18(3), 242. https://doi.org/10.1027//1015-5759.18.3.242
- Schunk, D., & Pajares, F. (2002). The development of academic self-efficacy. In A. Wigfield & J. Eccles (Eds.), Development of achievement motivation (pp. 15–31). Academic Press. https://doi.org/10.1016/b978-012750053-9/50003-6
- Schwarzer, R., & Jerusalem, M. (1995). Generalized self-efficacy scale. Measures in health psychology: A user’s portfolio. Causal and Control Beliefs, 1, 35–37.
- Zimmerman, B. J., Bandura, A., & Martinez-Pons, M. (1992). Self-motivation for academic attainment: The role of self-efficacy beliefs and personal goal setting. American Educational Research Journal, 29(3), 663–676. https://doi.org/10.3102/00028312029003663