Abstract
Prior findings highlight school students’ low motivation to study science, while 21st-century education aims to achieve their key competencies: scientific literacy and autonomous problem-solving to be prepared for smooth adaption to quickly changing circumstances. Real-life learning scenarios can meet these shortfalls to engage the learners by the knowledge-in-use perspective. This, however, requires that learners have high-level abilities of complex learning. We conducted a case study of outdoor learning with 8th–graders (age 14–15) to foster their scientific thinking addressing a real-life problem hands-on. Although the results showed that students’ conceptual knowledge increased, they also indicate participants’ cognitive taxonomy difficulties. An insight into the cognitive load aspects is given as it may appear in different autonomous learning situations. This work highlights the aspects little discussed so far (1) school students’ varying cognitive skills; (2) difficulties arising from the high cognitive load caused by multitasking, accompanied by the complex learning approach. This raises the questions of how to conduct motivating science lessons, where to practice the multi-component learning skills; and how to foster autonomous learning, where to gain the relevant knowledge without overburdening novice learners.
PUBLIC INTEREST STATEMENT
Constructing one’s conceptual knowledge in complex SDL) directly necessitates advanced higher-order skills to integrate one s pre-existing and new knowledge, which is being learned, flexibly. While SDL means synthesizing a relevant conclusion by (comprehending the task demand, and planning respective activities)combining the needed items into a new whole that necessitates keeping several learning elements in the focus of the human limited working memory at once for adequate analysis (choosing the best model). This, however, adds significantly greater cognitive demands on the young learners’ cognitive systems compared to traditional learning methods (orchestrated by the teacher, and thus the cognitive load is lower for the learner). In this, one’s metacognitive executive attention is responsible for maintaining the relevant information, through resisting interference by irrelevant elements, in order to make correct decisions in sub-task to reach the final right conceptualization. Yet, executive functions’ development is prolonged in children, hence, the hightened cognitive demand accompanying SDL may cause one’s cognitive overload that hampers (a) memorization and (b) controlling of one’s learning at the same time. As a result, the learning outcome might be poor. Therefore, SDL needs to be studied particularly in the K-12 context which lacks empirical evidence with school students.
1. Introduction
In quickly changed global circumstances, it is increasingly important to think and conceptualize independently (Tekkol & Demirel, Citation2018). This demands enhancing the scientific literacy of the young generation while practicing a hands-on approach (Aziz et al., Citation2014), and training them to regulate their own learning (Dörrenbächer & Perels, Citation2016) to be prepared to reorient smoothly in the 21st century (Lee et al., Citation2014). However, this kind of complex learning can be challenging especially for young students to reach their proper conclusions (De Bruin & van Merriënboer, Citation2017; Schwaighofer et al., Citation2017) in information-rich environments (Arghode et al., Citation2013; Garner, Citation2009). It is because complex learning requires the learner to maintain a pile of information items concurrently in the focus of the human limited working memory (Cowan, Citation2014; Engle, Citation2018; Du Toit-Brits, Citation2018) to “highlight” some part of the information to be kept in mind when trying to keep track of the goal, and to memorize at the same time—which is difficult if confronted with too much concurrent information (Butcher & Sumner, Citation2011; Kirschner et al., Citation2006). Especially this aspect is difficult for school students due to their developmental immaturity at the cognitive capability level yet (Rutherford et al., Citation2018).
In this regard, a critical range is the adolescence period, when students’ aspirations concerning science are formed (DeWitt & Archer, Citation2015), while learners in this age often lack motivation (Archer et al., Citation2017). As a contradiction: complex science learning, especially hands-on, is not possible without the individual motivation to contribute (1) determining the problem to solve (by factual knowledge); (2) finding resources to use (by prior learning skills) while (3) constantly adjusting one’s own attention (Francom, Citation2010. p 31).
The problem is that making choices, solving problems, and organizing one’s own learning (Hematian et al., Citation2017) creates a much higher cognitive load than in conventional methods, where the teacher organizes most of the learning material (De Bruin & van Merriënboer, Citation2017; Lee et al., Citation2014) with considerably lower cognitive demand for the learner (Gredler & Garavalia, Citation2000). While self-directed learning, in order to plan, and search for learning items (Rutherford et al., Citation2018) necessitates also the sub-conclusions to be kept in mind (Garner, Citation2009; Nęcka & Lulewicz, Citation2016; Sweller et al., Citation1998, Citation2019; Zepeda et al., Citation2015). The latter may cause severe working memory pre-occupation, which, due to one’s cognitive overload, challenges reaching the proper new knowledge construction (Schwaighofer et al., Citation2017. p. 60).
Developmental neuroscience shows that the maturing of human cognitive abilities (to cope with the kind of heightened cognitive load that accompanies complex learning) advances gradually and with a prolonged path (Lee et al., Citation2013). Hence, significant individual differences exist in school students’ cognitive capacities because of their varying maturing rhythm of being developed (Best & Miller, Citation2010; OECD, Citation2018). It is because the frontal cortex—which facilitates higher-order processes—matures as the last area of the brain in late adolescence, and continues improvement even in early adulthood (Anderson, Citation2002; Reio & Davis, Citation2005).
Based on the aforementioned findings, this paper examines two important aspects from the young learners’ perspective (1) individual cognitive skills that facilitate complex learning, and (2) the components of autonomous construction of knowledge—to be prepared for its proper usage in everyday situations (Duit et al., Citation2008), where one’s skills to evaluate arguments based on evidence are particularly important (Millar, Citation2006; Stöckert & Bogner, Citation2020). Prior work shows that many students (especially at adolescence age) seriously lack autonomous learning skills (Millar, Citation2006; Reio & Davis, Citation2005). The latter may be related to that while following subtasks, processing information, and integrating new knowledge at the same time, the learner’s cognitive overload arising by the multitasking severely hampers their processing (Kirschner et al., Citation2006). Thus, the interplay of working memory and executive attention to resist distractions during a cognitive constructive activity becomes especially evident (Schunk, Citation1989). It is because when too many items enter the limited cognitive scope, it hampers efficient integration of elements for proper conceptualization (Schwaighofer et al., Citation2017. p. 62). Therefore, too high a cognitive load, as a result, leads to insufficient/not deep enough or superficial knowledge gain (Sweller et al., Citation2019).
This paper differs from previous work by examining in-depth the learners´ scientific concept construction, while prior studies have so far handled mainly the participants’ pre and post data, not the components related to the phenomenon of the learning progress itself (Mills et al., Citation2016).
2. Theoretical background
2.1. Hands-on learning
Self-directed hands-on learning is based on the autonomous learning approach, which means taking initiative from the external (teacher) to the individual (learner): defining one’s own learning needs; establishing learning goals; deciding on the sources for learning; choosing, applying, and carrying out appropriate learning strategies (Aziz et al., Citation2014; Tekkol & Demirel, Citation2018). This kind of multi-level contribution needs one’s proper attentional control that is fundamental in each of those steps (Dagal & Bayindir, Citation2016) and therefore requires advanced executive skills to resist distractions (Rutherford et al., Citation2018). It is because the human cognitive architecture allows to include in working memory concurrently only a small amount of elements to deal with, that is, 3 to 4 units (Cowan, Citation2014), after which the respective result (by giving meaning to this set of integrated elements) is to be saved in the long-term memory (Sweller et al., Citation1998). The working memory reflects one’s capacity to keep important information active, in which the high- and low-span learners differ greatly (Coolidge & Wynn, Citation2005. p. 8). In this regard, working memory capacity (WMC) is an important individual-difference variable (Engle, Citation2018), like an input “bottleneck” into one’s long-term memory (as a mental gating of memorization) in achieving the best output of the current mental task demand (Baddeley, Citation1996).
Nonetheless, the effectiveness of complex learning requires also other cognitive functions, such as abstraction and differentiation—a complicated psychological process that cannot simply be “taken on” or “learned” but develops gradually (Vygotsky, Citation1994. p 356). Therefore, especially in young learners (with their normal development not fully matured yet), working memory gets more easily overloaded in high load conditions and cannot be used for memorization at the same time (Kirschner et al., Citation2006). In other words, the capability to select among alternatives (i.e., to “highlight” some part of the information to be kept in mind) is complicated especially for school students when trying to keep track of the goal and memorizing at the same time, while also confronted with too much concurrent information (Kirschner et al., Citation2006; Butcher and Sumner, Citation2011). The latter, however, is particularly important in complex learning (Best & Miller, Citation2010) requiring multi-level adaptation (Dörrenbächer & Perels, Citation2016) while solving the sub-tasks and controlling one’s own learning at the same time—that creates a much higher cognitive load than conventional methods (De Bruin & van Merriënboer, Citation2017). Therefore, complex hands-on learning in particular requires one’s proper capability to resist distraction (Rutherford et al., Citation2018) to retain only the relevant information in the focus of attention (Engle, Citation2018). Thus, one’s proper executive control (to resist distracting the sequential processing) is especially crucial, defending an input between the working memory and long-term memory in memorizing information (Nęcka & Lulewicz, Citation2016; Sweller et al., Citation1998, Citation2019; Unsworth et al., Citation2014).
2.2. Group-work aspects
From the developmental perspective, grounded on Vygotskian views of higher psychological processes being socially embedded (Valsiner, Citation2015), the importance of social support, for example, collaborating learning to engage in learning is crucial (Gredler, Citation2012; Hadwin & Oshige, Citation2011). Working together with others can promote knowledge growth, where the group (a) serves “as a teacher” helping to structure the process of autonomous learning (Schweder & Raufelder, Citation2019); and (b) as a medium based on a social root in one’s cognitive development (Stöckert & Bogner, Citation2020). Also, the usage of negotiating in the learning process (Paas & Sweller, Citation2012) promotes face-to-face interaction to achieve the group’s goals as well as an assessment of the sub-achievements that improve the whole group’s effectiveness (Roger & Johnson, Citation1994. p 2). Group work helps to consider alternative views proposed by others, enlarging one’s own understanding (Roger & Johnson, Citation1994), where students cooperate rather than compete (Aziz et al., Citation2014). Also, in hands-on learning outdoors (where groups of students are physically distributed), peer students can become an important source of help (Mettis & Väljataga, Citation2019), where individuals may benefit from socially shared communication and examples given by others (Stöckert & Bogner, Citation2020).
3. Current work
Involving planning, exploring, synthesizing, and conceptual concluding (see,), we designed for the students an experimental assignment outdoors—the theory-driven approach (for understanding design-based educational innovations in practice) to foster scientific thinking in fruitful settings (Design-Based Research Collective, Citation2003). The aim of this work is to investigate adolescents’ hands-on science learning to independently draw evidence-based conclusions to make decisions about the natural world and the human-made changes directly influencing it (OECD-PISA, Citation1999). We used a group-based collaborative learning approach in an authentic environment, where participants hands-on gathered data in real-world conditions to deduce their conceptual conclusions within the given topic.
Figure 1. Research design and instruments of a study(i.e., participants’ learning) activity timeline.
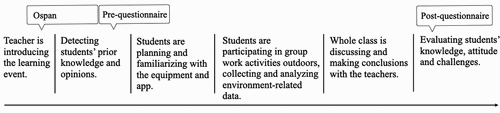
Our ultimate goal was to detect whether there appear indications to the relations between the following aspects:
individual cognitive capacity (measured in each student before starting the outdoor assignment) and the change in one’s conceptual knowledge;
the number of difficulties (by the participants’ reports they faced during the hands-on learning scenario);
the cognitive dimensions evolved from the difficulties (referring to the possible overload at the developmental sequences);
the number of additional tasks taken upon oneself in collaborative learning (to succeed working in a small group).
The participants’ assignment was to learn hands-on about a real-life problem that was currently relevant in the Estonian society, that is, “Should the new road be built on the seaside leisure region favoured by citizens or not?” and how to explain evidence-based opinion on this issue in scientific terms. This contained the following sub-task that are typical of complex learning: remembering facts; understanding the concepts (for reasoning); applying (carrying out procedures); analysing (determining how the parts interrelate); evaluating (making judgments); creating (putting elements together, and/or reorganizing) elements into a new pattern or structure (Wilson, Citation2016). The current experiment was organized as a part of a more extensive research study on innovative approaches to learning and teaching outside the classroom supported by technology.
4. Data gathering
The following tools were used:
(1) To measure the profile (high/low level) of individual working memory capacity (WMC) we used the Ospan memory task created by Unsworth et al (Citation2005). This tool was adapted into Estonian already before the present study, by which significant differences were found by Uus, Seitlinger, & Ley (Citation2020) in participants’ knowledge gain indoors within the framework of the autonomous learning paradigm. The assumption in the current study was that conditions learning outdoors were even more fruitful (compared to screen-based learning indoors), thus they can directly hamper processing while constructing new knowledge in working memory to save respective “results” in long-term memory (de Bruin & van Merriënboer, Citation2017). Moreover, young students’ working memory efficiency (Schwaighofer et al., Citation2017. p. 60) varies considerably (Nęcka & Lulewicz, Citation2016).
(2) Semi-structured questionnaires were used to measure (a) the change in one’s conceptual knowledge (comparing prior and post knowledge levels); (b) the number of difficulties; (c) the number of group-work sub-tasks taken upon oneself (following a track / measuring substances / gathered data entry); (d) retrospective reflections on group-work experiences.
We have run a collective case study that includes personalized feedback of several similar individuals about the phenomenon based on their personal experiences (Baxter & Jack, Citation2008) during the SDL outdoors. We also present some descriptive information leading to an understanding of the study phenomenon by additional information expressed visually. We follow the process theory approach, that is, evidence-based examination of specific contexts and particular individuals (Brantlinger et al., Citation2005, p 203; Maxwell, Citation2010). The present approach helps us to open the qualities of different perspectives to gain an understanding of the important aspects within the domain (Gammelgaard, Citation2017) of the phenomenon to be revealed (Baxter & Jack, Citation2008). Giving some numerical results of a visual overview, we address the main research question:
How do school students’ individual cognitive abilities, cognitive demand accompanying complex learning, and collaborative learning tasks interact during his/her scientific knowledge gain?
5. Method
Permission from the school director, then the science teacher was asked. Participation in the learning event was voluntary (and was decided by the teacher of this class). A letter describing the study and its aim, data management, and ensuring confidentiality as well as no harm caused to the students, was sent to the parents asking for their consent (to allow their children to participate in the study). Participants provided their consent upon entering the experiment (we also informed them that they could quit at any time).
First, we defined each participant’s (1) individual working memory capacity (independent variable), expressing one’s cognitive profile to process information in complex learning conditions; (2) prior knowledge level within the given topic (independent variable) i.e., what facts he/she already knows on the topic that will be learned. Second, after finishing the assignment, we considered individual (3) post-knowledge level (dependent variable) to find out whether he/she learned new knowledge as aresult of this kind of hands-on learning assignment; (4) difficulties they faced (independent variable); (5) tasks taken in the group work (independent variable); (6) retrospective experience on the group-work (independent variable).
6. Participants
The participants of the study were school students aged 14–15y from one class (Grade 8) of a municipal school in Tallinn, Estonia. Participation in the study was entirely voluntary. We chose this age group for two reasons: (1) in the age of adolescence the students often lose their motivation to learn science (Archer et al., Citation2017), thus the scenario used in this work was to attract them. (2) One’s cognitive load aspects (Rutherford et al., Citation2018), directly influencing the transfer of knowledge (Sweller et al., Citation2019; Young et al., Citation2014) to reach a proper conclusion (Du Toit-Brits, Citation2018), can open the different corners of the heightened cognitive demand accompanying the complex learning approach (described in this work).
6.1. Inclusion criteria
Although there was a larger sample size (24 participants) taking part initially, we discovered that many students had not completed all the tasks; and/or answered all the questions; and/or did not provide their correct personal identification code, which they were asked to create and use in each of the sub-tasks (e.g., letters of one´s name and numbers of the date of birth). Due to the aforementioned reasons, we included only these students’ data who had completed all the tasks, answered all the questions, and marked in all the sub-tasks the correct identification code. The final number of participants was 10.
6.2. Hands-on tasks for participants
Based on a socio-environmental problem, that is, the construction of a road at the seaside in Tallinn, a four-hour lesson was organized, where the participants formed an evidence-based scientific conclusion about the positive and negative effects of road construction. The lesson included:
Introduction to the learning event (15 minutes). Researchers (acted also as teachers in the experiment) introduced the socio-environmental problem as a context for the learning tasks and shortly demonstrated the technology used for supporting the learning tasks.
Ospan memory task (20 minutes, performed individually).
Prior knowledge of the road construction (10 minutes, performed individually)
Gathering into small groups: setting up a hypothesis (water, air, or soil quality, according to each group’s sub-topic), carrying out the outdoor measurements, that is, collecting data; coming back to the classroom where they drew conclusions, designed a poster with the main conclusion, and presented it to other groups (2 hours 20 minutes, together with one’s groupmates).
Post knowledge of the road construction, and experience about the group-work (10 minutes, individually).
7. Research instruments and data analysis
(I) Ospan (The Operation Span Task) is a validated measure of individual cognitive working memory capacity (WMC), with good internal (.78) and test–retest reliability (.83) in which to-be-remembered items are interspersed with some form of distracting activity as a dual-task that is typical in multitasking situations (Unsworth et al., Citation2014, Citation2005). In the Ospan task, the distracting activity includes math equations presented consecutively (on separate pages) that have to be judged—true or false (e.g., 3 * 7 = 22). To-be-remembered items were letters {F, H, J, K, L, N, P, Q, R, S, T, Y} appearing at the centre of the screen (one after another for 1 sec each). In every trial, the letters and equations alternated until the last letter was presented, then students were asked to recall all presented letters in the same order. A higher score (in recalling correctly) shows the participant’s more effective ability to remember important information. While a lower Ospan-score indicates that it is more difficult for this participant to keep the attention and resist “distractions”—it makes it difficult for him to concentrate only on relevant information to be remembered (Unsworth et al., Citation2014). The latter aspect is particularly important in the SDL context. We implemented this task as a web-based application of JSPsych (De Leeuw, Citation2015).
(II) Semi-structured questionnaires were used to measure (1) individual knowledge-level change (conducted before and after the outdoor-learning scenario to detect whether the students increased their initial knowledge level, that is, if they learned or not); (2) difficulties (obstacles the students encountered); (3) the number of group-work sub-tasks taken during the collaborative learning; (4) group work reflection (the cooperation efficacy between the group mates).
To analyse the results, we used a communicative product of written words (often varying in terms of perception as well as by individuals) to examine the processes that would otherwise be difficult to measure (Osgood, Citation1952. p 198). The knowledge levels were analysed based on Heddy et al. (Citation2017) operationalization of conceptual change as follows:
“1” indicating the student has an inaccurate, misconception
“2” indicating the student has a hybrid conception that mixes misconceptions with an accurate understanding;
“3” indicating an accurate but underdeveloped understanding of the concepts (1 explanation)
“4” indicating an accurate but not fully developed understanding of the concepts (2 explanations)
“5” indicating the student has a well-developed and nuanced understanding of the concept (3 or more explanations).
The difficulties evolved by the participants’ answers: conceptual; metacognitive; procedural; strategic, were analysed based on Hannafin et al. (Citation1999). We relied on Bloom’s and Krathwohl (Citation1956) which contains categories ranging from lower-order towards higher-order skills with gradually increasing complexity (Adams, Citation2015; Wilson, Citation2016). The taxonomy we used (initiated Bloom & Krathwohl, Citation1956, revised by Krathwohl, Citation2002) is the following:
The group-work experience was evaluated on a 5-point scale, from “1” not satisfied at all to “5” very satisfied. There was also an option in the questionnaire to add participants’ own words to describe their group-work experience (contribution to the group-work); satisfaction with the group members; cooperation within the group; evaluation on their group work by a presentation of their SDL results (see, , on the right).
After the deep analysis that was done by three researchers to avoid possible differences, analyses were conducted with the SPSS statistics program using descriptive statistics.
The Ospan task was conducted in the classroom, where the students were asked to find a place in the room as distanced as possible from others sitting at separate tables each equipped with a tablet. Telephones, pencil/paper, and/or other helping tools were not allowed. Participants were provided with verbal instruction and demonstration of how to generate a personal ID-code for an anonymous treatment of the data, and how to perform the Ospan-task. We (three researchers) made sure that everyone understood, completed the task individually, and stayed focused. Each student was asked to individually answer questionnaires based on his/her current knowledge about the socio-environmental problem of their hometown (described above). Dividing into small groups (consisting of 3–4 students) each group created hypotheses according to the topic they were given (e.g., air, soil, or water quality); followed a predefined path with different location points; carried out their necessary measurements (about the environmental conditions, see, , in the centre); came back into the classroom; analysed data; made their conclusion; presented their outcome to other groups ( on the right).
The learning activities were supported by the web- and location-based application Avastusrada (Discovery Track by a smartphone or a tablet, see, ) to help navigate in an unfamiliar location; the students were asked to use their own smartphones (familiar to them) to follow the track and insert data they had gathered. For the latter different sensors were used (salinity, conductivity, temperature, pH, turbidity, anemometer, dissolved oxygen, relative humidity, light sensor, soil moisture sensor, infrared thermometer; EV3 Lego robot’s brain was used for reading sensor measurements).
Figure 3. A screenshot of the application used in this work called ”Avastusrada” (”Tracking path” in English).
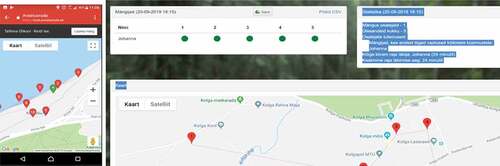
The researchers’ task was to observe the students in action remotely without direct intervention (to scaffold only if needed). The students’ movement and their location were monitored by GPS (see, on the left). The location points with questions and tasks got activated when students reached the particular location and turned to green as soon as the answer to the question or the task was submitted (, on the right). In this study, the Avastusrada app was used with four aims: to direct instructions, to provide access to content (of the experiment), to support data collection, and to enable interaction between context and learner (Suárez et al., Citation2018).
8. Results
We first give an overview of the cross-case results, while the details within each case description are provided at the end of this paper.
9. Cross-case analysis
As our data reflects participants’ experiences with the phenomenon of complex learning and their group work, the numbers (as a legitimate sort of data about the frequency, amount, or typicality of particular phenomena) are complementary to qualitative information, yet not substituting for it (Maxwell, Citation2010).
Two participants with higher Ospan memory task scores of 64 and 73 increased their post-knowledge even by four levels, whereas in cases of Ospan-score up to 60, no special dynamics (between prior and post-knowledge levels) appeared. However, with higher Ospan-scores (the memory task result from 60 upwards respectively), there was a remarkable increase in participants’ post-knowledge level. Yet, the post-knowledge of the student with the highest Ospan-score (76), in turn, somewhat decreased.
We next analysed () the conceptual change (in terms of increase of the knowledge) regarding the difficulties the participants reflected (they had faced over their SDL assignment course). The participant with the highest Ospan-score (76) reported the greatest number of difficulties i.e., six of them (the details are described in section Within-Case Analysis, p. 29: Participant 10) who did not increased but even decreased his/her initial knowledge level. Quite a similar pattern is revealed also in the case of the participant with the average Ospan-score (56) who also reported the greatest number of difficulties (i.e., six of them) and did not learned new knowledge (i.e., did not increased his post-knowledge level).
Figure 4. Dynamics in participant’s conceptual knowledge level in light of his/her cognitive capacity score (given on the right vertical) based on the number of difficulties he/she reported (given on the left).
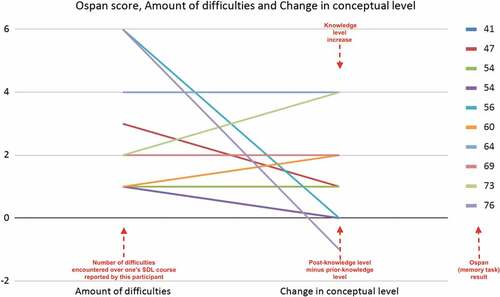
On the other hand, two students: one of them with a rather average Ospan-score (i.e., the result of 60 in memory task, increasing his/her knowledge level by 2 points), and the other with rather a high capacity (73) both increased their conceptual knowledge by 4 points—true, reflecting only a few difficulties (one in the case of the former; two by the latter participant), compared to the six difficulties described above. We next analysed the cognitive dimensions in difficulties according to Bloom’s and Krathwohl (Citation1956) cognitive taxonomy (see, ) from simple to more challenging types (Wilson, Citation2016), and from the concrete to the abstract (Krathwohl, Citation2002). We see, () that the most demanding for participants were (1) the Conceptual Knowledge (altogether mentioned 13 times) type of difficulties (i.e., relations of models and structures, compiling multi-component cognitive acts) that require quick mental interaction between Understanding/Applying/Evaluating/Creating. Also, (2) Factual Knowledge (mentioned 8 times), which includes the process of Creating that is the most difficult mental function (Krathwohl, Citation2002) because it requires that the students keep in mind the relevant items (while resisting irrelevant) especially important in high cognitive load (Kirschner et al., Citation2006; Butcher and Sumner, Citation2011), as it requires multi-level adaptation (Dörrenbächer & Perels, Citation2016). Both of these (1 and 2) directly relate to Procedural Knowledge (mentioned 6 times), which necessitates carrying out respective physical acts (i.e., to embody the mental “results”).
Table 1. Bloom’s taxonomy
Table 2. Cognitive categories evolved from the difficulties (that were reflected by the participants’ own words)
The latter findings might be related to the outcome discovered above: when the number of difficulties was higher (the students with their Ospan-score 56 and 76, who both reported 6 difficulties), the level of their conceptual knowledge did not increase (as it seems to be the case of those students who reported fewer difficulties), even in the case of the participant with the highest WMC score (76), his/her post knowledge, in turn, decreased. This pattern may indicate an overload (1) accompanying complex learning, which itself requires multi-level processing concurrently; and (2) cognitive load gets heavier when taking upon oneself too many tasks in collaborative group-work activities (Kirschner et al., Citation2006). We next analysed (based on participants’ own words) the number of sub-tasks taken on their shoulders during the group work ). We see that when taking the highest number of group-work tasks (i.e., six of them), the conceptual knowledge level in this participant (with his/her Ospan score of 54b marked by dark violet) did not increase.
Figure 5. The number of group-work tasks taken in collaborative learning and his/her conceptual knowledge change.
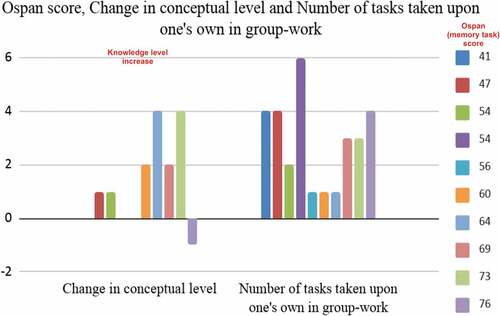
However, when taking only one group-work task (e.g., with an Ospan score of 64), then his/her conceptual knowledge increased even by four levels. Then again, when taking four tasks, this student (e.g., with a low Ospan score of 47) increased his/her conceptual knowledge only by one level. Moreover, taking four group-work tasks, yet having the lowest Ospan-score (41), this student did not increase his/her conceptual knowledge level at all.
Based on those outcomes, we see an indication that the more the participant took the group-work sub-tasks on their shoulders, the less their conceptual level increased, or, in turn, was even hampered (as it turned out in participants with the highest Ospan-score of 76). These dynamics can at least partly be related to the overall functioning of the entire group as well. The same pattern was revealed also from the within-case analysis, where not all participants were contributing equally, and therefore some students seemingly had to take too many tasks upon themselves. For example, the participant with the lowest Ospan-score (41) taking upon him/herself four group-work sub-tasks, said on his/her group work, “It did not go exactly as planned”, despite he/she reports only one difficulty “Setting up hypothesis” (that shows it was not overly difficult to learn for him).
Next, we compared participants’ conceptual knowledge change based on their average group work experience (). We see that students who rated their group-work experience lower also had lower Ospan-score as well as smaller conceptual knowledge change.
At the same time, the participant with the highest Ospan-score (76) showed even negative dynamics in his/her conceptual knowledge (i.e., decreasing it by 1 level), yet this student still enjoyed the group work a lot (as shown Within-case analysis p. 29). More precisely, this participant evaluated his/her contribution to their group-work also very highly (rating at “5” very satisfied), explaining, “We all worked equally and a lot”. He/she took upon him/herself four group-work sub-tasks and was very satisfied with the group-mates as well, saying, “We had a very good group”. However, he/she also said, “It went smoothly but my idea got stuck … ”, while at the same time reporting six difficulties.
The latter example may indicate that this student faced cognitive overload (while reflecting six difficulties), and thus got tired and as a result either dramatically decreasing his/her post-knowledge results or simply not answering correctly (the last questionnaire in this complex learning assignment).
10. Discussion
We studied adolescence-age school-students complex learning outdoors focussing on detailed contextual aspects of specific cases, using the process view that sees the actual mechanisms involved in particular events and situations (Maxwell, Citation2010). Our results show an initial trajectory: the participants with their higher cognitive capacity profile increased their conceptual post knowledge more than students with the lower cognitive capacity profile. Although higher-capacity students also reported difficulties, they, in general, learned better. In line with prior findings, this can be related to their more efficient executive attention to resist distraction during the cognitive acts along with the complex learning (Rutherford et al., Citation2018). It is because of the human cognitive architecture restrictions, allowing to include concurrently only a small number of elements in working memory to deal with (Cowan, Citation2014) to create new schemas (Sweller et al., Citation1998). Thus, when working memory is being used for searching for sub-solutions (that is typical in complex learning), it is not used to save at the same time (Kirschner et al., Citation2006). Yet, working memory and attention interaction is the most important factor of mental operations for the integration and transfer of new knowledge (Sweller et al., Citation2019; Young et al., Citation2014) to reach a proper conclusion (Du Toit-Brits, Citation2018) in order to memorize new information (Sweller et al., Citation1998). This aspect came out also from the current work difficulties by the participants’ own words (). We interpret the latter: Constructing one’s conceptual knowledge (from identifying the problem towards deducing the final solution), the learner’s cognitive mechanism triangulates with all the needed info-items (keeping in mind what?, in which sequence?, and how I should do?) directly necessitating one’s higher-order skills (Adams, Citation2015). These sequential processes can be seen through a cumulative hierarchical framework (that was needed to perform the SDL assignmnt also in the current work), where the achievement of the next (i.e., more complex) skill requires completing the previous one (Krathwohl, Citation2002. p. 218). In this, the young learner’s cognitive restrictions, directly enabling one’s mental “postage”, are especially important to acknowledge by educators in order not to overload students along with the complex learningthat imposes a heavy cognitive load on the working memory (Engle, Citation2018)
It is because, especially complex SDL necessitates the learner to maintain a pile of information elements concurrently in the focus of the human limited working memory (Cowan, Citation2014; du Toit-Brits, Citation2018) in order to “highlight” some part of the information (to be memorized i.e., kept in mind) while at the same time to keep track of the goal, and organize subsequent activities (e.g., measuring, calculating, but also communicating with group-mates), which is difficult if confronted with too much concurrent information (Kirschne et al., 2006; Butcher & Sumner, Citation2011). Particularly this aspect is found to be esepcially difficult for school-age students due to their developmental immaturity at the cognitive capability level yet (Rutherford et al., Citation2018).
In this regard, the outcome of the current work is in line with prior findings: you cannot synthesize a relevant conclusion without creating a proper hypothesis that needs (i) comprehending; (ii) planning; (iii) combining the needed info-tems, and respective skills into a new whole from Comprehension to Creating that requires keeping in the focus of one’s limited working memory several elements concurrently in order to adequately analyse (i.e., to compare alternatives to choose the best model) before properly creating (Krathwohl, Citation2002. p. 216). This relates to prior findings, saying that especially complex learning adds significantly greater cognitive demands on individual cognitive mechanisms compared to traditional learning methods (Rutherford et al., Citation2018), where executive attention is directly responsible for maintaining the present information (helping to resist interference) through a higher order control process (Coolidge & Wynn, Citation2005). According to the Cognitive Load Theory, too high a mental load hampers processing, which distracts one’s ability to construct new knowledge in long-term memory (De Bruin & van Merriënboer, Citation2017).
This aspect (e.g., applying is impossible without understanding) came out also in the current work, suggesting the avoidance of cognitive overload, and highlighting the importance of a metacognitive cometency training and practicing with school-agestudents, considering their lower cognitive skills, compared to adults (especially in learning tasks that induce heightened processing loadyet directly affecting the learner’s ability to construct new knowledge autonomously (De Bruin & van Merriënboer, Citation2017). Especially in the adolescence age, when learning science is often related to students’ low motivation (Archer et al., Citation2017). Whereas the latter (at least partly) can also be caused by the aspect of difficulty to learn complex lessons autonomously passing developmental spurts at the cognitive capability level related to the human prefrontal cortex improvement, among other developmental growing up aspects (Rutherford et al., Citation2018; Wilson, Citation2016). Therefore, to enable the young generation to practice hands-on science learning, it is highly needed to keep their optimal cognitive load to cope the kind of reciprocal mental acts accompanying the complex learning (Garner, Citation2009; Rutherford et al., Citation2018). The latter is needed to help them to create proper new knowledge and obtain scientific literacy—not getting lost, and loosing motivation, in the multiplicity of information—to gain not superficial or fake but quality knowledge, and make independent decisions in order to be prepared for conceptualizing adequately.
11. Conclusion
Based on prior work and the current results, we cannot take for granted that when implementing the complex learning approach, all participants equally cope with the necessary multi-level cognitive steps to create their new knowledge. We thus take the current results as the base for our next study with a larger sample of the same age group of learners, keeping in mind the important aspects of young learners described in this work. Moreover, autonomous learning skills are important also within the distance-learning circumstances, which is an increasingly required educational approach caused by the global pandemic situation, yet not always with superior results. Notwithstanding the small sample size (thus not generalizable results) from a negative side of our work, we call for further studies to find the strategies in science learning as well as in the complex learning framework in general to optimize learners’ cognitive load. There may also be other characteristics associated with autonomous learning, thus, future work should include additional fine-grained aspects of complex learning and its role in learners’ achievement. As educational researchers from the perspective of psychology, we, therefore, continue discussions on how to offer today’s school students optimally challenging science lessons as well as innovative age-appropriate demands to efficiently implement the complex SDL paradigm. This, on the one hand, would properly stimulate the learners’ intellect to enable them to gain relevant knowledge; and on the other hand, should avoid overburdening young learners to uphold their interest in science and motivation to learn autonomously, continuing life-long learning—to be prepared to make their own independent decisions and adequately adapt in the rapidly changing life of the 21st century.
Knowledge construction in technology-enhanced outdoor learning
The initial conceptualization of self-directed learning (SDL) is based on andragogy with adult learners’ fully refined capabilities. While from the young learners’ perspective whose cognitive capabilities are in the process of being developed, SDL lacks respective empirics.
Outdoor learning – going out of a safe classroom, often without the direct support of a teacher, which makes especially important the individual ability to regulate one’s activities – supported by technology integrated into this study is one example of the typical SDL scenario where students are supposed to carry out both: obtain quality knowledge and guide their learning autonomously.
Yet, the aspect of accompanying with complex learning such as one’'s cognitive load aspect (instanced in this work from the difficulties’ perspective reported by the students), needs to be studied further on how to efficiently integrate technology in SDL outdoors, where students can connect information from both environments (a) real-life connectable to (b) virtual, in a way not overloading young learners who are still in the stage of being developed and therefore may suffer through the overly high cognitive demand – deteriorating ”to be learned”.
Acknowledgements
This project is partially funded by the European Union’s Horizon 2020 Research and Innovation Programme, grant agreement No. 669074 and No 856954, and by the project “TU TEE - Tallinn University as a promoter of intelligent lifestyle“ (nr 2014-2020.4.01.16-0033) under activity A5 in the Tallinn University Centre of Excellence in Educational Innovation.
We are greatly thankful to all school students, and their teachers taking part in this study as well as other participants of the Ceiter research team who were helping to prepare and cary out this Outdoor Learning Project Day.
Disclosure statement
No potential conflict of interest was reported by the author(s).
Additional information
Funding
Notes on contributors
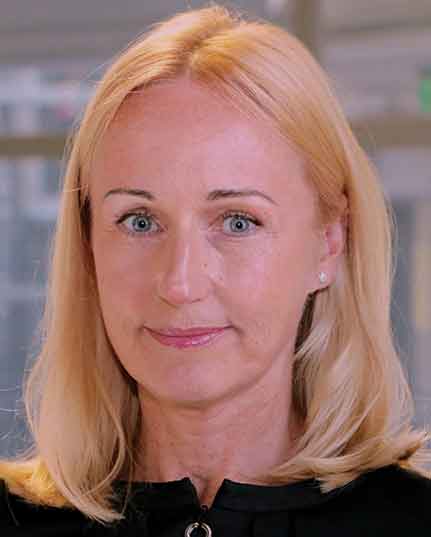
Õnne Uus
Õnne Uus Junior Research Fellow School of Educational Sciences https://www.etis.ee/CV/%C3%95nne_Uus001/est?tabId=CV_ENG [email protected]
Kadri Mettis
Terje Väljataga Associate Professor in new learning environments and technologies School of Educational Sciences https://www.etis.ee/Portal/Persons/Display/40f45cf8-d2d1-4aec-957d-8c08b88d830c?PersonVID=52888&lang=EST&tabId=CV_ENG [email protected]
Terje Väljataga
Kadri Mettis Junior Research Fellow School of Digital Technologies https://www.etis.ee/CV/Kadri_Mettis/est?tabId=CV_ENG [email protected]
References
- Adams, N. E. (2015). Bloom’s taxonomy of cognitive learning objectives. Journal of the Medical Library Association: JMLA, 103(3), 152. https://doi.org/10.3163/1536-5050.103.3.010
- Anderson, P. (2002). Assessment and development of executive function (EF) during childhood. Child Neuropsychology, 8(2), 71–20. https://doi.org/10.1076/chin.8.2.71.8724
- Archer, L., Moote, J., Francis, B., DeWitt, J., & Yeomans, L. (2017). The “exceptional” physics girl: A sociological analysis of multimethod data from young women aged 10–16 to explore gendered patterns of post-16 participation. American Educational Research Journal, 54(1), 88–126. https://doi.org/10.3102/0002831216678379
- Arghode, V., Yalvac, B., & Liew, J. (2013). Teacher empathy and science education: A collective case study. Eurasia Journal of Mathematics, Science and Technology Education, 9(2), 89–99. https://doi.org/10.12973/eurasia.2013.921a
- Aziz, M. S., Zain, A. N. M., Samsudin, M. A. B., & Saleh, S. B. (2014). The effects of problem-based learning on self-directed learning skills among physics undergraduates. International Journal of Academic Research in Progressive Education and Development, 3(1), 126–137. https://doi.org/10.6007/IJARPED/v3-i1/694
- Baddeley, A. (1996). The fractionation of working memory. Proceedings of the National Academy of Sciences, 93(24), 13468–13472. https://doi.org/10.1073/pnas.93.24.13468
- Baxter, P., & Jack, S. (2008). Qualitative case study methodology: Study design and implementation for novice researchers. The Qualitative Report, 13(4), 544–559.
- Best, J. R., & Miller, P. H. (2010). A developmental perspective on executive function. Child Development. NIH Public Access, 81(6), 1641–1660. https://doi.org/10.1111/j.1467-8624.2010.01499.x
- Bloom, B. S., & Krathwohl, D. R. (1956). Taxonomy of educational objectives: The classification of educational goals, by a committee of college and university examiners. Handbook I: Cognitive domain. Longmans, Green.
- Brantlinger, E., Jimenez, R., Klingner, J., Pugach, M., & Richardson, V. (2005). Qualitative studies in special education. Exceptional Children, 71(2), 195–207. https://doi.org/10.1177/001440290507100205
- Butcher, K. R., & Sumner, T. (2011). Self-Directed learning and the sensemaking paradox. Human–computer Interaction, 26(1–2), 123–159.
- Coolidge, F. L., & Wynn, T. (2005). Working memory, its executive functions, and the emergence of modern thinking. Cambridge Archaeological Journal, 15(1), 5–26. https://doi.org/10.1017/S0959774305000016
- Cowan, N. (2014). Working memory underpins cognitive development, learning, and education. Educational Psychology Review, 26(2), 197–223. https://doi.org/10.1007/s10648-013-9246-y
- Dagal, A. B., & Bayindir, D. (2016). The investigation of the relationship between the level of metacognitive awareness, self-directed learning readiness and academic achievement of preschool teacher candidates. Universal Journal of Educational Research, 4(11), 2533–2540. https://doi.org/10.13189/ujer.2016.041106
- De Bruin, A. B., & van Merriënboer, J. J. (2017). Bridging cognitive load and self-regulated learning research: A complementary approach to contemporary issues in educational research. Learning and Instruction, 51, 1–9. https://doi.org/10.1016/j.learninstruc.2017.06.001
- De Leeuw, J. R. (2015). jsPsych: A JavaScript library for creating behavioral experiments in a Web browser. Behavior Research Methods, 47(1), 1–12. https://doi.org/10.3758/s13428-014-0458-y
- Design-Based Research Collective. (2003). Design-based research: An emerging paradigm for educational inquiry. Educational Researcher, 32(1), 5–8. https://doi.org/10.3102/0013189X032001005
- DeWitt, J., & Archer, L. (2015). Who aspires to a science career? A comparison of survey responses from primary and secondary school students. International Journal of Science Education, 37(13), 2170–2192. https://doi.org/10.1080/09500693.2015.1071899
- Dörrenbächer, L., & Perels, F. (2016). More is more? Evaluation of interventions to foster self-regulated learning in college. International Journal of Educational Research, 78, 50–65. https://doi.org/10.1016/j.ijer.2016.05.010
- Du Toit-Brits, C. (2018). Towards a transformative and holistic continuing self-directed learning theory. South African Journal of Higher Education, 32(4), 51–65. https://doi.org/10.20853/32-4-2434
- Duit, R., Treagust, D., & Widodo, A. (2008). Teaching science for conceptual change: Theory and practice. In International handbook of research on conceptual change (pp. 629–646). Routledge.
- Engle, R. W. (2018). Working memory and executive attention: A revisit. Perspectives on Psychological Science, 13(2), 190–193. https://doi.org/10.1177/1745691617720478
- Francom, G. M. (2010). Teach me how to learn: Principles for fostering students’ self-directed learning skills. International Journal of Self-directed Learning, 7(1), 29–44. http://citeseerx.ist.psu.edu/viewdoc/download?doi=10.1.1.469.3531&rep=rep1&type=pdf#page=34
- Gammelgaard, B. (2017). Editorial: The qualitative case study. The International Journal of Logistics Management), 28(4), 910–913. https://doi.org/10.1108/IJLM-09-2017-0231
- Garner, J. K. (2009). Conceptualizing the relations between executive functions and self-regulated learning. The Journal of Psychology, 143(4), 405–426. https://doi.org/10.3200/JRLP.143.4.405-426
- Gredler, M. E., & Garavalia, L. S. (2000). Students’ perceptions of their self-regulatory and other-directed study strategies: A factor analysis. Psychological Reports, 86(1), 102–108. https://doi.org/10.2466/pr0.2000.86.1.102
- Gredler, M. E. (2012). Understanding Vygotsky for the classroom: Is it too late? Educational Psychology Review, 24(1), 113–131. https://doi.org/10.1007/s10648-011-9183-6
- Hadwin, A. F., & Oshige, M. (2011). Self-regulation, coregulation, and socially shared regulation: Exploring perspectives of social in self-regulated learning theory. Teachers College Record: The Voice of Scholarship in Education, 113(2), 240–264. https://doi.org/10.1177/016146811111300204
- Hannafin, M. J., Land, S. M., & Oliver, K. (1999). Open learning environments: Foundations, methods, and models. In C. Reigeluth (Ed.), Instructional design theories and models (Vol. II pp. 115–140). Erlbaum.
- Heddy, B. C., Danielson, R. W., Sinatra, G. M., & Graham, J. (2017). Modifying knowledge, emotions, and attitudes regarding genetically modified foods. The Journal of Experimental Education, 85(3), 513–533. https://doi.org/10.1080/00220973.2016.1260523
- Hematian, F., Rezaei, A. M., & Mohammadyfar, M. A. (2017). On the effect of goal setting on self-directed learning, achievement motivation, and academic achievement among students. Modern Applied Science, 11(1), 37–47.
- Kirschner, P. A., Sweller, J., & Clark, R. E. (2006). Why minimal guidance during instruction does not work: An analysis of the failure of constructivist, discovery, problem-based, experiential, and inquiry-based teaching. Educational Psychologist, 41(2), 75–86. https://doi.org/10.1207/s15326985ep4102_1
- Krathwohl, D. R. (2002). A revision of Bloom’s’ taxonomy: an overview. Theory into Practice, 41(4), 212–218. https://doi.org/10.1207/s15430421tip4104_2
- Lee, K., Bull, R., & Ho, R. M. (2013). Developmental changes in executive functioning. Child Development, 84(6), 1933–1953. https://doi.org/10.1111/cdev.12096
- Lee, K., Tsai, P.-S., Chai, C., & Koh, J. (2014). Students’ perceptions of self-directed learning and collaborative learning with and without technology. Journal of Computer Assisted Learning, 30(5), 425–437. https://doi.org/10.1111/jcal.12055
- Maxwell, J. A. (2010). Using numbers in qualitative research. Qualitative Inquiry, 16(6), 475–482. https://doi.org/10.1177/1077800410364740
- Mettis, K., & Väljataga, T. (2019). Mapping the challenges of outdoor learning for both students and teachers. In Digital turn in schools—research, policy, practice (pp. 51–65). Singapore: Springer.
- Millar, R. (2006). Twenty first century science: insights from the design and implementation of a scientific literacy approach in school science. International Journal Of Science Education, 28(13), 1499–1521. https://doi.org/10.1080/09500690600718344
- Mills, R., Tomas, L., & Lewthwaite, B. (2016). Learning in earth and space science: A review of conceptual change instructional approaches. International Journal of Science Education, 38(5), 767–790. https://doi.org/10.1080/09500693.2016.1154227
- Nęcka, E., & Lulewicz, A. (2016). Capacity, control, or both–which aspects of working memory contribute to children’s general fluid intelligence? Polish Psychological Bulletin, 47(1), 21–28. https://doi.org/10.1515/ppb-2016-0003
- OECD. (2018). The future of education and skills: education 2030. OECD Education Working Papers.
- OECD-PISA. (1999). Measuring student knowledge and skills: A new framework for assessment.
- Osgood, C. E. (1952). The nature and measurement of meaning. Psychological Bulletin, 49(3), 197. https://doi.org/10.1037/h0055737
- Paas, F., & Sweller, J. (2012). An evolutionary upgrade of cognitive load theory: Using the human motor system and collaboration to support the learning of complex cognitive tasks. Educational Psychology Review, 24(1), 27–45. https://doi.org/10.1007/s10648-011-9179-2
- Reio, T. G., & Davis, W. (2005). Age and gender differences in self-directed learning readiness: A developmental perspective. International Journal of Self-Directed Learning, 2(1), 40–49. https://www.oltraining.com/SDLwebsite/IJSDL/IJSDL2.1-2005.pdf#page=44
- Roger, T., & Johnson, D. W. (1994). An overview of cooperative learning. Creativity and Collaborative Learning, 1–21.
- Rutherford, T., Buschkuehl, M., Jaeggi, S. M., & Farkas, G. (2018). Links between achievement, executive functions, and self‐regulated learning. Applied Cognitive Psychology, 32(6), 763–774. https://doi.org/10.1002/acp.3462
- Schunk, D. H. (1989). Social cognitive theory and self-regulated learning. In Self-regulated learning and academic achievement (pp. 83–110). New York, NY: Springer.
- Schwaighofer, M., Bühner, M., & Fischer, F. (2017). Executive functions in the context of complex learning: Malleable moderators? Frontline Learning Research, 5(1), 58–75. https://doi.org/10.14786/flr.v5i1.268
- Schweder, S., & Raufelder, D. (2019). Positive emotions, learning behavior and teacher support in self-directed learning during adolescence: Do age and gender matter?. Journal of Adolescence, 73, 73–84. https://doi-org.ezproxy.tlu.ee/10.1016/j.adolescence.2019.04.004
- Stöckert, A., & Bogner, F. X. (2020). Cognitive learning about waste management: How relevance and interest influence long-term knowledge. Education Sciences, 10(4), 102. https://doi.org/10.3390/educsci10040102
- Suárez, A., Specht, M., Prinsen, F., Kalz, M., & Ternier, S. (2018). A review of the types of mobile activities in mobile inquiry-based learning. Computers and Education, 118, 38–55. https://doi.org/10.1016/j.compedu.2017.11.004
- Sweller, J., Van Merrienboer, J. J., & Paas, F. G. (1998). Cognitive architecture and instructional design. Educational Psychology Review, 10(3), 251–296. https://doi.org/10.1023/A:1022193728205
- Sweller, J., van Merriënboer, J. J., & Paas, F. (2019). Cognitive architecture and instructional design: 20 years later. Educational Psychology Review, 31(2), 261–292. https://doi.org/10.1007/s10648-019-09465-5
- Tekkol, İ. A., & Demirel, M. (2018). An investigation of self-directed learning skills of undergraduate students. Frontiers in Psychology, 9(NOV), 2324. https://doi.org/10.3389/fpsyg.2018.02324
- Unsworth, N., Heitz, R. P., Schrock, J. C., & Engle, R. W. (2005). An automated version of the operation span task. Behavior Research Methods, 37(3), 498–505. https://doi.org/10.3758/BF03192720
- Unsworth, N., Fukuda, K., Awh, E., & Vogel, E. K. (2014). Working memory and fluid intelligence: Capacity, attention control, and secondary memory retrieval. Cognitive Psychology, 71, 1–26. https://doi.org/10.1016/j.cogpsych.2014.01.003
- Uus, Õ., Seitlinger, P. C., & Ley, T. T. (2020). Cognitive capacity in self-directed learning: Evidence of middle school students’ executive attention to resist distraction. Acta Psychologica, 209, 103089. https://doi.org/10.1016/j.actpsy.2020.103089
- Valsiner, J. (2015). The place for synthesis: Vygotsky’s analysis of affective generalization. History of the Human Sciences, 28(2), 93–102. https://doi.org/10.1177/0952695114559530
- Vygotsky, L. S. (1994). The development of academic concepts in school aged children. In R. van der Veer & J. Valsiner (Eds .), The Vygotsky Reader, vol. 355, pp. 355–370. Oxford: Blackwell. https://www.marxists.org/archive/vygotsky/works/reader/p355.pdf
- Wilson, L. O. (2016). Anderson and Krathwohl–Bloom’s taxonomy revised. Understanding the New Version of Bloom’s Taxonomy. https://quincycollege.edu/wp-content/uploads/Anderson-and-Krathwohl_Revised-Blooms-Taxonomy.pdf
- Young, J. Q., Van Merrienboer, J., Durning, S., & Ten Cate, O. (2014). Cognitive load theory: Implications for medical education: AMEE guide no. 86. Medical Teacher, 36(5), 371–384. https://doi.org/10.3109/0142159X.2014.889290
- Zepeda, C. D., Richey, J. E., Ronevich, P., & Nokes-Malach, T. J. (2015). Direct instruction of metacognition benefits adolescent science learning, transfer, and motivation: An in vivo study. Journal of Educational Psychology, 107(4), 954.
Within-Case Analysis
Participant 1, Ospan score 41
His/her prior opinion (about the road) was negative (i.e., the road should not be built) and stayed the same after the SDL. This student had a very high conceptual understanding of the problem already before the intervention (level 5 on the scale of 1–5) and remained the same (after the assignment). The student evaluated his/her contribution to the group work very highly (5), and explained: “We divided all the tasks and all of us did their parts”. He/she was very satisfied with the group members, and explained: “It was fun”. To the question about how satisfied the student was with the group work result, the answer was neutral, and he/she explained “It did not go exactly as planned”. This student took upon him/herself four different group-work sub-tasks. She/he had one difficulty - setting up a hypothesis.
Participant 2, Ospan score 47
The pre-opinion was negative and stayed the same (after). This student had a relatively high prior knowledge level (level 4), which increased by 1 point (to level 5 after). The student evaluated his/her contribution to the group work rather highly (4), and explained “I conducted measuring, mapped the situation and listened to the presentations”. He/she had a neutral evaluation about the group-mates (3), explaining “One of our group members left, one didn’t contribute and also the third one was rather inactive, it was rather a pair work than group work”. About the group work result, the answer was very positive (5), and he/she explained: “We could answer all the questions and the poster came out very nice”. This student took upon him/herself four different sub-tasks during the group work activities. He/she had three difficulties - setting up robots and sensors; analyzing the collected data and making conclusions; preparing the presentation.
Participant 3, Ospan score 54a
The pre-opinion about the road was negative, but it changed into neutral (after). He/she had some conceptual knowledge about the issue (level 3), which increased by 1 level (after). The student evaluated his/her contribution to the group work very highly (5), and explained: “We solved the tasks in turns, and the cooperation was smooth”. He/she was very satisfied with the group members, explaining “We all were hardworking people”, also with the group work result, explaining “Everything was very well divided between us”; and with the cooperation in the group. He/she took upon him/herself two different tasks (in the group-work). He/she had one difficulty - setting up robots and sensors.
Participant 4, Ospan score 54b
The pre-opinion about the road was negative, and it stayed the same (after). He/she had some conceptual knowledge (level 3) about the issue and this level remained the same (after). The student evaluated his/her contribution to the group work rather highly (4), and explained: “I was active”. He/she was rather satisfied with the group members (4), explaining “group members helped to solve the tasks”. About the group work result, the answer was neutral (3), explaining “We didn’t have enough time to prepare”. He/she was rather satisfied with the cooperation, explaining “We all had our roles in the group and everyone filled their tasks”. He/she took upon him/herself six different tasks (in the group-work). He/she had one difficulty - understanding the instructions.
Participant 5, Ospan score 56
The pre-opinion about the road was negative and stayed the same (after). He/she had some conceptual knowledge (level 3) about the issue and this level remained the same (after). The student evaluated his/her contribution to the group work at a medium level (3), explaining “We all were actively involved”. He/she was very satisfied with the group members, explaining “We were successful at fulfilling our tasks”; also with the groupwork result, and with the cooperation in the group, explaining “Everything worked well in the group”. This student took upon him/herself one task (in the group work). This student had six difficulties - understanding the instructions; setting up a hypothesis; finding the location points; setting up robots and sensors; following the track in Avastusrada and inserting the sensor data, and analyzing the collected data and making conclusions.
Participant 6, Ospan score 60
The pre-opinion about the road was neutral and stayed the same (after). He/she had no pre-conceptual knowledge about the issue but the level of conceptual knowledge increased by two levels (after). The student evaluated his/her contribution to the group work very highly (5), explaining “We all were working equally ”. He/she was very satisfied with the group members; the group work result; and also with the cooperation in the group, but did not add any specific explanation to the evaluation. This student took upon him/herself one task (in the group work). This student had one difficulty - analyzing the collected data and making conclusions.
Participant 7, Ospan score 64
The pre-opinion about the road construction was neutral but changed into a negative opinion (after). The student had no conceptual knowledge about the issue but the level of conceptual knowledge increased by four levels (after). The student evaluated his/her contribution to the group work as medium (3), explaining “My contribution was enough”. He/she was very satisfied with the group members, explaining “Everyone participated actively”; also with the groupwork result, explaining “In my opinion, everything came out very well and the presentation went smoothly”, and with the cooperation in the group, explaining “Everyone worked equally”. This student took upon him/herself one task (in the group work). This student had four difficulties - understanding the instructions; setting up a hypothesis; navigating between Avastusrada and google documents; analyzing the collected data and making conclusions.
Participant 8, Ospan score 69
The pre-opinion about the road was negative and stayed the same (after). The student had some conceptual knowledge about the issue (level 3) and increased to level 5 (after). The student evaluated his/her contribution to the group work at medium (3), explaining “It was interesting to find out new things”. He/she was very satisfied with the group members, explaining “They managed well”. He/she was very satisfied with the groupwork result, explaining “We made a good presentation”; also with the cooperation in the group, explaining “We managed well”. This student took upon him/herself three tasks (in the group work). This student had two difficulties - setting up robots and sensors, and preparing the presentation.
Participant 9, Ospan score 73
The pre-opinion about the road construction was neutral but changed into a negative opinion (after). The student had some conceptual knowledge about the issue (level 3) and increased to level 5 (after). The student evaluated his/her contribution to the group work rather highly (4), explaining “We worked equally”. He/She was very satisfied with the group-members, explaining “They were good”; also with the group-work result, explaining “The presentation went well”, and with the cooperation in the group, explaining “The cooperation was smooth”. This student took upon him/herself three tasks (in the group work). This student had two difficulties - understanding the instructions, and setting up robots and sensors.
Participant 10, Ospan score 76
The pre-opinion about the road was negative and stayed the same (after). This student had a relatively high conceptual knowledge level (4) before, but it decreased to level 3 (after). The student evaluated his/her contribution to the group work very highly (5), explaining “We all worked equally and a lot”. He/she was very satisfied with the group members, explaining “We had a very good group”; rather satisfied with the group-work result, explaining “It went smoothly but my own idea got stuck”. He/she was very satisfied with the cooperation in the group. This student took upon him/herself four tasks in the group work. This student had six difficulties - understanding the instructions; finding the location points; setting up robots and sensors; following the track in Avastusrada and inserting the sensor data; navigating between Avastusrada and google documents; analyzing the collected data and making conclusions.