Abstract
This study investigates the effects of smart classrooms, comprehensive assessment management information systems, and remediation teaching techniques on teachers ‘and student’s conceptual understanding of physics. The participants included 67 students from Senior One, Senior Two, and Senior Three, purposively sampled from G.S Mushongi in Kirehe district, along with 40 physics teachers selected purposively from the same district. Data was collected using two instruments: the Physics Achievement Test and the Teacher Mixed Multiple-Choice Questionnaire. Both students and teachers underwent remediation and training, respectively. The study focuses on taught specific physics units for each grade level: Current Electricity and Qualitative Analysis of Linear Motion for Senior One; Magnetism and Demagnetization and Reflection of Light in Curved Mirrors for Senior Two; and Heat Transfer and Quantity of Heat, Renewable Energy and Non-Renewable Energy, and Introduction to Electromagnetic Induction for Senior Three. Descriptive and inferential statistics were computed to analyze the data, and the results were presented using tables and figures created using Microsoft Excel and Origin version 2019b software. For Senior-One, Senior-Three students, the post-test scores demonstrated a highly statistically significant improvement (p < .001), suggesting that the remediation positively influenced their performance. However, for Senior Two students, the data analysis did not show any statistically significant improvement in their performance after remediation (p > .05). The results indicated a significant difference after training (p < .001) for the teachers. This research highlights the importance of targeted remediation and smart classrooms in enhancing academic performance and teachers’ perceptions.
Public interest statement
In modern education, classrooms are physical spaces and dynamic environments where knowledge flows between students and teachers. To empower students and tackle misconceptions in complex subjects, we advocate for integrating advanced technological solutions like the Comprehensive Assessment Management Information System (CAMIS) alongside remediation learning techniques. By harnessing these tools, educators can identify struggling students and provide tailored support to bridge learning gaps, ensuring no student is left behind. Equally important is equipping teachers with the necessary technological skills to effectively utilize CAMIS and pinpoint each student’s strengths and weaknesses. This approach enables educators to monitor individual student progress, deliver targeted remediation, and ultimately, drive improved academic outcomes. Through these innovative strategies, we aim to foster a learning environment where every student has the opportunity to thrive, paving the way for a brighter future through quality education.
REVIEWING EDITOR:
Introduction
As we transition from the industrial age to the knowledge age, educators and policymakers emphasize the importance of equipping students with a diverse range of 21st-century skills. These skills include collaboration, problem-solving abilities, and evidence-based reasoning (Tissenbaum et al., 2019). Classrooms are vital for effective skills and knowledge dissemination from instructors to students (Saini & Goel, Citation2019). A study by Wu (Citation2016) demonstrated that technology, such as computer technology, network technology, and multimedia resources, can create adaptable and interactive learning materials, enabling students to engage in self-directed learning with the guidance of teachers. A study by Al-Maroof et al. (Citation2022) revealed that providing resources like audio-visual aids, libraries, and computers improves accessibility for teachers and students, leading to better learning outcomes and overall educational quality. Rubric-based formative assessment can aid in regular assessment and monitoring of students’ performance, facilitating informed decision-making for teachers (Nsabayezu et al., Citation2022). Learner-centered teaching, through remediation and computer-assisted instruction, has been shown to impact students’ performance and enhance their understanding significantly (Nsabayezu et al., Citation2023). However, the effectiveness of this approach is hindered in Rwanda as teachers lack the necessary training to proficiently utilize ICT in their teaching methods, contributing to a shortage of qualified teachers with technology integration skills (Mugiraneza, Citation2021). Thus, contributes to the lack of students’ understanding of physics and poor mathematical skills as significant obstacles contributing to their difficulties in solving physics problems.
The shift toward a competence-oriented curriculum in Rwanda’s education system underscores the need for proficient teachers capable of facilitating active learning in science and various subjects (Dushimimana & Uworwabayeho, Citation2020). Embracing technology and competence-based curriculum can enhance student’s academic achievement and elevate the overall teaching and learning experience with schools. A study by Mushimiyimana and Dushimimana (Citation2021) found that to enhance human capital, teachers should incorporate improvisation, creativity, and innovation into their teaching methods using smart classroom technology. Incorporating technological tools in smart classrooms supports regular assessment and monitoring of student achievement, empowering teachers to make well-informed decisions and efficiently manage the learning process (Uwineza et al., Citation2023). Whereas, a study by Celestin et al. (Citation2021) revealed that the integration of technology in education, especially the use of smart classrooms, has garnered interest due to its potential influence on students’ academic performance. Despite these potential benefits, there is a noticeable research gap in Rwanda regarding the current status of smart classrooms and comprehensive management systems, particularly in physics. This study aims to address this gap by investigating the impact of introducing a comprehensive assessment management information system and remediation learning techniques on the conceptual understanding of physics among teachers and students.
Remedial education, an extracurricular program in educational institutions, aids low-achieving students in meeting academic standards (Melton, 2008). It enhances performance in primary subjects, fosters confidence, and strengthens academic foundations (Ukobizaba et al., Citation2019). By providing a supportive learning environment, remediation accommodates individual differences, assists struggling students, and nurtures interpretation and critical thinking skills (Paulin & Mugiraneza, Citation2023). Targeted interventions, like remedial instruction, aim to improve academic performance by identifying and supporting slow learners (Reylene, Citation2022). Remedial instruction is particularly beneficial for students facing challenges in following typical classroom routines. Luoch (Citation2017) noted that remediation enables governments to harness students’ untapped potential, offering opportunities they might otherwise miss. It bridges knowledge gaps and empowers students to perform optimally, while Asio and Jimenez (Citation2020) observed improved assessment scores and student engagement during remedial sessions.
Rwanda’s government launched the Competence-based curriculum in 2015 and implemented measures such as smart classrooms and one-laptop per child programs to promote the use of ICT and multimedia in education (Iyamuremye & Ndagijimana, Citation2022). The integration of smart classrooms with critical resources such as computers and internet access aims to use ICT to improve the quality of teaching and learning in schools. According to Wallet and Kimenyi (Citation2019) study, a smart classroom is an approach that involves extensive utilization of existing and new ICT infrastructure and devices, creating new digital content aligned with the national curriculum to enhance the quality of teaching and learning. The findings suggest that expanding the availability and effective usage of smart classrooms in Rwandan boarding schools has the potential to increase students’ academic performance (Mushimiyimana & Dushimimana, Citation2021). Smart classrooms create a dynamic learning environment that encourages valuable teacher-student interactions through conversations, improving the overall teaching and learning experience at all stages (Omirzak et al., Citation2022). Smart classrooms provide various benefits to educators and students, including improved learning experiences, targeted cognitive skill development, and support for comprehensive assessment systems with teacher decision-making help (Pellegrino & Quellmalz, Citation2010). Nyirahagenimana et al. (Citation2022) discovered that training core implementers on the use of 5E instructional models resulted in a considerable improvement and fostered more engaging learning experiences for students. The smart classroom setting is intended to encourage cooperative and communal inquiry, providing valuable insights and design principles for study in the learning sciences community.
In the context of our investigation into the impact of remediation on student performance, it is imperative to acknowledge the relevance of Human-Computer Interaction (HCI) (Germanakos, Citation2024; Kumar & Bavankumar, Citation2024) and its paradigms, particularly in the design and evaluation of smarter classrooms. Incorporating concepts from HCI allows for a deeper analysis of the interaction between students, teachers, and educational technology within digitally enhanced learning environments. By considering aspects such as interface design, user experience, and cognitive load, we aim to understand how these factors influence learning outcomes and student engagement. Moreover, the integration of HCI principles enables us to explore the role of physical and virtual cognitive analysis in assessing students’ cognitive processes and behaviors (Kumar & Bavankumar, Citation2024), thus providing valuable insights into the effectiveness of remediation strategies (Paulin & Mugiraneza, Citation2023; Reylene, Citation2022). This holistic approach ensures that our research contributes not only to the field of education but also to the broader discourse within the IT community regarding the design and implementation of technology-enhanced learning environments.
The shift from traditional education to computer-mediated instruction has been embraced by both students and teachers for its flexibility and convenience. Computer technology serves as a catalyst for educational transformation, enhancing teaching and learning methods (Nyirahabimana et al., Citation2023). Educators utilizing computers can expand their knowledge and refine their teaching strategies. Those with high self-efficacy in ICT usage are better equipped to meet the demands of integrating technology into diverse instructional activities (Anderson & Maninger, Citation2007; Teo, Citation2015). As suggested by Tuyizere and Yadav (Citation2023), interactive computer simulations can augment the teaching and learning of physics, warranting educators’ consideration. Modern teaching tools like computers and software offer students instant access to vast information, enhancing subject comprehension and knowledge acquisition (Ndihokubwayo, Citation2024). Nyirahabimana et al. (Citation2023) posit that students are inherently motivated to develop their understanding through computer-mediated instruction, often outperforming those in traditional learning environments. Furthermore, the integration of ICT, as indicated by Kanyesigye et al. (Citation2022), proves beneficial in enhancing students’ conceptual grasp.
The aim of this study is to enhance teachers’ and students’ conceptual understanding in physics by implementing smart classrooms and a comprehensive assessment management information system. Here are the research hypotheses:
Null hypothesis (H01): There is no statistically significant difference in students’ academic performance (senior-one, senior two, and senior-three) in physics before and after receiving remediation learning techniques.
Null hypothesis (Ho2): There is no statistically significant difference in the conceptual understanding of physics teachers after receiving training on smart classrooms and comprehensive assessment management information systems.
Research methods
Research design and research instruments
In quantitative research, measurements and numerical data are fundamental (Bouikidis, 2020). This study employed a quasi-experimental research design with a quantitative approach to investigate the influence of remediation on student performance. The objective was to assess how remediation affects academic outcomes, evaluating the effects of remediation learning techniques, smart classrooms, and comprehensive assessment management information systems using two main research instruments: the Physics Achievement Test (PAT) and the Teachers Mixed Multiple-Choice Questionnaire (TMMCQ).
The PAT comprised questions from seven units, with varying numbers of questions per unit. Senior one covered two units with 20 questions each, senior two covered two units with 15 questions each, and senior three covered three units with 40 questions. The TMMCQ included three parts: Part A related to CAMIS with 13 items, Part B covers smart classroom items with 11 items, and Part C encompasses general ICT items with nine items. Part A and B used a five-point Likert scale, while Part C employed true and false items. Overall, the TMMCQ comprised 33 items. These instruments, along with the chosen research design, facilitated a comprehensive assessment of the impact of remediation on student performance.
Population and sampling techniques
Inappropriate sample size and selection methods can lead to erroneous conclusions in investigations across social science and research (Chu et al., Citation2012). Purposeful sampling offers advantages in tailoring the sample closely to the research purpose, resulting in valid and reliable results, particularly in qualitative research methods in a cultural context (Thomas, Citation2022). This study utilized a purposive sampling technique to meticulously select 67 students (senior one, senior two, senior three) from G.S. Mushongi (Groupe Scholaire Mushongi) out of a total of 80 students (refer to ). Additionally, 40 physics teachers from 20 schools were deliberately chosen. The selection of G.S. Mushongi was influenced by its identification in CAMIS as a school with significantly unsatisfactory performance in certain units taught in lower secondary schools. The teachers were chosen based on their demonstrated strong interest in ICT, and their status as permanent physics teachers, making them eligible candidates for advanced training and enabling them to share their expertise within their respective schools.
Table 1. Participants.
Data collection procedures and intervention
Expert opinions were utilized to establish content validity and reliability (Balta & Eryılmaz, Citation2010). Adeleke and Joshua (Citation2015) proposed that multiple-choice items should undergo psychometric testing to enhance item quality and achieve dependable and valid test outcomes. The data collection procedures and intervention comprised several essential steps to enhance the educational process. Initially, the physics achievement test (PAT) consisted of 75 questions and underwent a rigorous piloting process. It was piloted to 100 senior two students at G.S Mpanga for face validation to ensure the clarity and comprehensibility of the questions. Our finding revealed a significant Pearson product-moment correction coefficient, with an r-value of 0.81, affirming strongly positive results. Additionally, three physics lecturers from the University of Rwanda validated the content of the test to align it accurately with the subject matter. Moreover, the TMMCQ (Teacher Mixed Multiple-Choice Questionnaires) was subjected to validation by two expert ICT lecturers to ensure its effectiveness in assessing ICT-related aspects. The feedback and comments received from the validators were thoughtfully addressed and incorporated into the questionnaires before proceeding to the next phase. Later, we conducted a pilot test of the TMMCQ involving 10 teachers to assess its face validity and reliability. Our finding showed a great Pearson product-moment correlation of r = 0.78 over time, demonstrating favorable results. Following the validation process, the facilitators took the opportunity to explain the research’s purpose and its potential impact to all participants, including students, schools, and the wider community. To effectively track the students’ difficulties in the specific units under investigation, pre-tests were administered to students from senior one to senior three. These pre-tests were conducted on 27th April 2022 and were tailored differently based on the students’ respective grade levels. On that same date, teachers were also provided with pre-tests to gauge their perceptions regarding the implementation and effectiveness of smart classrooms (SC) and CAMIS. This valuable feedback from teachers contributed to the overall evaluation of the educational tools. Subsequently, the remediation phase commenced for senior one, senior two, and senior three students. This remediation was conducted consistently over a two-month period, starting on 1st May 2022 and concluding on 1st July 2022. The remediation sessions were held daily, from 6 am to 7 am, which was equivalent to one period or 60 minutes before the commencement of regular classes. This strategic approach aimed to address the students’ learning difficulties and enhance their understanding of the subject matter. Simultaneously, online training sessions were conducted for teachers, focusing on effectively utilizing smart classrooms SC and CAMIS to enhance their teaching methods and assessment techniques. This training began on 1st May 2022 and concluded on 1st June 2022. Finally, the post-test was conducted on 2nd July 2022 to assess the impact of the remediation and training interventions on both students’ and teachers’ performance. The post-test duration for students was two hours, while teachers underwent a one-hour evaluation.
Comprehensive assessment management information system (CAMIS)
A comprehensive assessment management information system (CAMIS) is an integrated system that plays a crucial role in enhancing the quality of education and supporting data-driven decision-making. Comprehensive Assessment Management Information Systems (CAMIS) are tools or platforms designed to manage and streamline the assessment process within educational institutions (Majernik, Citation2018). CAMIS is a valuable tool for educators and administrators, enabling them to make informed decisions, improve educational outcomes, and ensure that students receive the support they need to succeed academically. The Comprehensive Assessment Management Information System (CAMIS) software application allows teachers to continuously monitor students’ progress and grades (REB, 2020). This system fosters critical thinking and problem-solving skills among students by encouraging their active engagement in the learning process. This system is composed with a bank of standardized questions for each unit. These questions are aligned with the introduced curriculum, known as the Competence-Based Curriculum. In this system, teachers upload marks for each unit depending on the subject, marks for the end of term, and the end of the projects. Uploading the student’s marks thus easily provide a picture of every student in terms of strengths and weaknesses. Additional information about the effectiveness of CAMIS can be assessed using the following link: https://www.globalpartnership.org/blog/lesson-rwanda-comprehensive-assessment-management-software-scores-highly-teachers.
Remediation learning techniques
Using CAMIS, it was observed that lower secondary students faced difficulties in understanding certain units. For senior one, the challenging units were Current Electricity and Qualitative Analysis of Linear Motion. Senior two students found magnetism and demagnetization, as well as reflection of light in curved mirrors, to be problematic. In senior three, Heat Transfer and Quantity of Heat, Renewable Energy and Non-Renewable Energy, and Introduction to Electromagnetic Induction posed challenges. However, Remediation, on the other hand, is an instructional program designed to aid students who struggle to meet the expected competencies outlined in their curriculum (Kalyoma, Citation2016). According to James and Folorunso (Citation2012) found that remediation boasts students’ conceptual understanding, especially students who struggle to achieve expected competence. According to the literature, students facing difficulties in the subjects are helped to excel like others academically through the provision of special care during instruction. As per now, it was noted that remedial instruction proves highly efficacious in assisting exceptionally struggling students, leading to improved academic performance for 70% of them ().
Figure 4. Sample demonstration for renewable and non-renewable sources of energy during remediation instruction (senior three).
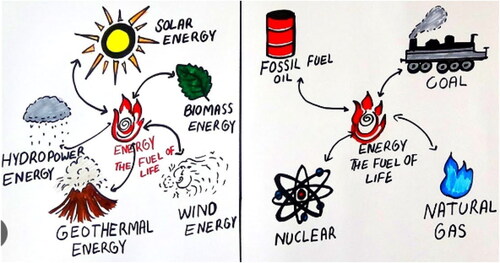
This support aims to enhance performance in core subjects and foster confidence and establish a more robust academic foundation, ultimately benefiting students across all their courses. Additional information related to the remediation learning techniques can be accessed via the following link:https://www.nesa.gov.rw/fileadmin/user_upload/NESA/Ressources/Remedial_and_catch_up_program/GENERAL_GUIDE_TO_REMEDIAL_AND_CATCH_UP_PROGRAMS_FOR_STUDENTS_AT_RISK_OF_REPETITION_AND_DROPPING_OUT_19.11.2020.pdf; https://allafrica.com/stories/202110070044.html
Data analysis techniques
Data analysis was conducted to examine the performance of both students and teachers, involving data entry, filtering, and scoring using Microsoft Excel 2019 and Origin version 2019b. In Microsoft Excel, we generated several figures: Comparative analysis of senior one students in pre-and post-test; Comparative analysis of senior two students in pre-and post-test; Comparative analysis of senior three students in pre-and post-test; Teachers performance in both pre-and post-tests. Subsequently, we turned to Origin 2019b to generate tables and figures for a more comprehensive analysis. To assess the impact of remediation and training, we computed descriptive and inferential statistics to analyze the data. The results were then presented using tables and figures created in Origin version 2019b software. These tables are Descriptive statistics of pre-and post-test-senior one; Inferential statistics-senior one student; Descriptive statistics of pre-and post-test-senior two; Inferential statistics-senior two students; Descriptive statistics of pre-and post-test-senior three; Inferential statistics-senior three students; Descriptive statistics of pre-and post-test-Physics teachers; Inferential Statistics-Physics teachers. Whilst, figures are: Difference in performance of senior one students in pre-and post-test; Difference in performance of senior two students in pre-and post-test; Difference in performance of senior three students in pre-and post-test; Difference in performance of physics teachers in pre-and post-test. To assess the statistical significance between the performance of students and teachers in their pre-and post-tests, we utilized the Wilks’ Lambda nonparametric test. This test allowed us to make meaningful comparisons and draw conclusions regarding the impact of remediation and training on both students and teachers.
Results
Lower secondary students’ performance due to the effectiveness of remediation
presents a comparative analysis of senior students’ performance, with the blue color representing their performance in the pre-test and the orange color representing their performance in the post-test. The assessment covered two senior one units: 'Current Electricity’ and 'Qualitative Analysis of Linear Motion’. Overall, there is an improvement in students’ performance from the pre-test to the post-test. For instance, in the pre-test, student 2 scored 21%, while achieved 77% in the post-test. Similarly, student 16 scored 44% in the pre-test and 67% in the post-test. Although there were some students who scored above 90%, they are not mentioned here as their scores did not change significantly between the pre-test and post-test. However, most students demonstrated improvement, except for student 13, who did not show any progress. Surprisingly, there was a decrease in performance from the pre-test to the post-test for student 6. This scored 39 in the pre-test and 28 in the post-test.
below presents descriptive statistics for the pre-and post-test scores of senior one students in physics. The findings indicate a notable improvement, with the mean score in physics rising by 9.69%, from 53.42% in the pre-test to 63.12% in the post-test.
Table 2. Descriptive statistics of pre-and post-test-senior one students.
In , we employed an inferential analysis using a repeated measure ANOVA through Origin 2016b to determine if there is a statistically significant difference in the impact of remediation provided by teachers to senior one students ().
Table 3. Inferential statistics-senior one students.
presents a comparison of the performance of senior two students. Blue represents their performance in the pre-and post-test, while orange represents their performance in the post-test. The assessment focused on two units: Magnetism and Demagnetization and Reflection of Light in Curved Mirrors’. Overall, there is an improvement in students’ performance from the pre-test to the post-test. For example, student 1 scored 51% in the pre-test, whereas in the post-test scored 70%. Similarly, student 2 scored 42% on the pre-test and 61% on the post-test. Although some students scored above 80%, they are not mentioned because their scores did not change significantly between the two tests. However, most students showed improvement, except for student 13, who did not make any progress. Surprisingly, there was a decline in the performance from the pre-test to the post-test for student 11, who scored 66% in the pre-test and 48% in the post-test.
below shows descriptive statistics for the pre-and post-test scores of senior two students in physics. The results reveal a significant improvement, as the mean score in physics increased by 3.3125%, progressing from 58.37% in the pre-test to 61.68% in the post-test.
Table 4. Descriptive statistics of pre-and post-test-senior two students.
We aimed to determine if there was a statistically significant difference in the impact of teachers’ remediation on senior two students. To achieve this, we utilized parametric analysis, specifically the Repeated Measures ANOVA, using Origin 2019b (see ).
Table 5. Inferential statistics-senior two students.
Before conducting the ANOVA, we thoroughly validated that all necessary assumptions were met, employing Wilks Lambda for this purpose. The findings presented in indicate that there is no statistically significant difference in students’ performance (F = 2.57817, df = 1, p>.05) in favor of the post-test. In other words, the remediation offered by teachers did not result in a statistically significant improvement in students’ performance, according to the data analyzed.
presents a comparison regarding the performance of senior three students. Blue represents their performance in the pre-test, while orange represents their performance in the post-test. The assessment focuses on three units: Heat transfer and quantity of heat, Renewable Energy and non-renewable Energy, Introduction to Electro-magnetism Induction. Before the remediation, the students’ performance was initially low but improved afterward. For instance, in the pre-test, student 5 obtained a score of 31%, whereas in the post-test, the score rose to 85%. Similarly, student 18 scored 51% on the pre-test and 75% on the post-test. Overall, the mean scores of all students show improvement from 49.39% to 68.78%, although two students still require remediation. For example, student 12 does not make any progress even after the remediation. Surprisingly, there is a decline in the performance of student 1, scoring 57% on the pre-test and 45% on the post-test.
illustrates descriptive statistics of three senior students in both pre- and post-tests. The outcomes indicate a remarkable improvement, with the mean score in physics increasing 19.38%, advancing from 49.38% in the pre-test to 68.77% in the post-test ().
Table 6. Descriptive statistics of pre-and post-test-senior three students.
We carried out an inferential statistical analysis using a repeated measure ANOVA through Origin 2019b to determine whether a statistically significant difference exists in the influence of teacher’s remediation on senior three students (refer to ).
Table 7. Influence inferential statistics-senior three students.
Before conducting the ANOVA, we ensured that all necessary assumptions were satisfied by employing Wilks’ Lambda. The results displayed show a remarkably significant disparity in students’ performance (F = 31.27395, df = 1, p<.001) in favor of the post-test. These findings unequivocally demonstrate a substantial improvement in students’ performance attributed to the remediation provided.
displays the performance of physics teachers, and their performance are analyzed using a questionnaire divided into three parts: CAMIS-related items, Smart classroom items, and General items related to ICT. The CAMIS-related items encompass questions from Q1 to Q13, smart classroom items from Q14 to Q24, and ICT-related items from Q25 to Q33. Overall, the average performance of teachers increased from 63.38% in the pre-test to 75.26% in the post-test, indicating an 11.88% improvement after the training. Notably, the average performance of teachers improved for most items in the post-test compared to the pre-test. For example, there is significant progress in the ICT-related items, with Q27 showing a substantial improvement from 41.38% to 66.24%, a difference of 24.93%. However, some items did experience a decrease in performance. For instance, Q13 under CAMIS and Q27 under the smart classroom items declined from 70.49% to 67.00% and 79.51% to 74.75%, respectively. Despite these decreases, the overall trend indicates positive development in teachers’ performance following the training.
presents descriptive statistics illustrating the pre-and post-test scores of teachers. The total number of teachers who sit for pre-and post-tests is 40. Their outcomes indicate a remarkable improvement, with the mean score in physics increasing by 11.88%, advancing from 63.37% in the pre-test to 75.26% in the post-test.
Table 8. Descriptive statistics of pre-and post-test-physics teachers.
We performed an inferential analysis by employing repeated measures ANOVA through Origin 2019b to determine the statistical significance difference of the impact of online training on teachers. The results of the repeated measures ANOVA revealed a highly significant difference in the mean scores between the pre-and post-tests (F = 725.53756, df = 2, p<.001). This finding shows a statistically significance difference in the mean scores after online interventions (refer to ).
Table 9. Inferential statistics-physics teachers.
Figure 9 depicts the contrast in performance between pre-and post-test results of teachers, both before and after participating in the training sessions. The visual representation vividly exhibits a remarkable shift in the scores, with a substantial increase from 63.37% in the pre-test to 75.26% in the post-test, reflecting an 11.88% improvement after receiving the pieces of training. These results provide conclusive evidence of a significant enhancement in teachers’ performance following the training session (refer to )
Discussion of the results
This study delves into the impacts of implementing smart classrooms and a comprehensive assessment management system on the academic performance of senior students in physics. The units covered in the pre-and post-tests differed for each grade: senior one students were examined on ‘current electricity, and qualitative analysis of linear motion’; senior two students were assessed on ‘magnetism and demagnetization and reflection of light in curved mirrors’; and senior three were assessed on ‘heat transfer and quantity of heat, renewable energy and non-renewable energy, and introduction to electromagnetic induction’. Notably, all 67 students received remediation before the post-test. The results of the study revealed varying effects of introducing smart classrooms and the comprehensive assessment management system on the academic performance of students at different grade levels. Specifically, the mean scores for senior one students increased significantly by 9.69%, shifting from 53.42% in the pre-test to 63.12% in the post-test. This improvement was statistically significant. Similarly, senior three students significantly improved their mean scores, progressing from 49.39% in the pre-test to 68.78% in the post-test. The post-test for senior one and three students demonstrated highly statistically significant differences (F = 16.13806, df = 1, p<.001).
Examining individual student performance revealed noteworthy patterns. In senior one, the majority demonstrated score improvements, except for one student who showed no progress. Conversely, in senior three, most students exhibited enhancement, but two still needed remediation, and one student experienced a decline from the pre-test to the post-test. The success observed in senior and senior three students following remediation activities can be attributed to several factors. Firstly, the content covered in the remediation instruction was closely aligned with the specific subject areas covered in their pre- and post-tests. This alignment played a crucial role in reinforcing the students’ understanding of concepts, contributing to significant improvements in academic performance. Additionally, the adapted remediation approach was tailored to address identified learning gaps and difficulties unique to senior one and three students. James and Folorunso (Citation2012) found that remediation boasts students conceptual understanding, especially students who struggle to achieve expected competence. The students who taught through remediation activity demonstrate a significant improvement in their academic scores, strongly suggesting a positive impact of these interventions on their learning outcomes (Kanamugire & Rutakamize, Citation2008). Whereas a finding by James and Folorunso (Citation2012) revealed that incorporating instructional strategies that included feedback and remediation, particularly through the use of formative tests, significantly enhances academic achievements in the subjects. Moreover, a finding by Asio and Jimenez (Citation2020) on remediation activities found a significant difference in students’ performance after intervention. This absence of significant improvement in senior two students might have been attributed to the following factors: misalignment of the teaching methods used in the remediation instruction. The remediation approach might not have been adequately customized to address their unique learning needs, highlighting the importance of tailoring interventions based on the specific difficulties identified in the pre-test. Furthermore, the remediation for senior two may not have incorporated feedback from both students and teachers, missing valuable insights into their specific learning difficulties. A finding by Sonia (2022), found that academic performance is a result of significant correction between teachers’ and student’s relationships. A student’s academic success is influenced by individual traits, learning and teaching methods, and teacher connections (Göktaş & Kaya, Citation2023).
Regarding the teachers, feedback was collected through a questionnaire covering various aspects, including CAMIS-related items, smart classroom items, and general items related to ICT. The results from physics teachers indicated a highly significant difference in the mean scores between the pre-and post-tests (F = 725.53756, df = 2, p<.001). This suggests that the teachers’ performance improved after online training on the significant use of smart classrooms and CAMIS. However, it is worth noting that some items in the questionnaire showed a decrease in performance. For instance, Q13 under CAMIS and Q27 under the smart classroom items experienced a decline in scores from the pre-test to the post-test. This may indicate areas where additional support or training is needed for teachers to optimize the effectiveness of their remediation efforts. The finding by Celestin et al. (Citation2021) on smart classrooms revealed that smart classrooms positively motivate students, leading to a significant difference in performance compared to traditional classrooms. School management and effective use of smart classrooms in the teaching and learning process significantly impact ICT development and economic growth (Mushimiyimana, The finding by Mukagihana et al. (Citation2020) revealed that the resources like audio-visual aids, library facilities, and computers enhance learning outcomes and overall educational quality. The notable improvement in teachers’ abilities require the training on the effective use of ICT (Nyirahagenimana et al., Citation2022). The study by Nsengimana et al. (Citation2021)suggested that successful CBC implementation requires strong and regular training programs that focus on putting new ideas into practice and providing adequate teaching and learning resources for science. According to Mushimiyimana and Dushimimana (Citation2021), it was found that when properly managed, Continuous Professional Development plays a crucial role in improving teachers’ understanding of smart classroom technologies and, thus, ultimately the, academic performances.
Conclusion
This study investigates the effects of introducing comprehensive assessment management information system remediation teaching techniques on teachers’ perceptions and students’ academic performance, respectively. The findings revealed that targeted remediation plays a crucial role in positively influencing student progress, as evidenced by the significant increase in mean scores for Senior One and Senior Three students. Although the study did not show a statistically significant improvement in Senior Two students’ performance after remediation, it highlights the importance of continuous evaluation and support to tailor educational interventions effectively. Moreover, the research emphasizes the benefits of employing modern educational tools such as smart classrooms and assessment management systems, which can significantly impact students’ conceptual understanding. The adoption of these technological advancements also contributes to a positive shift in teachers’ perspectives, as demonstrated by the significant difference in mean scores between the pre- and post-tests for the participating teachers. This study underscores the significance of targeted remediation and advanced technological tools like CAMIS in enhancing academic outcomes.
Recommendations for Rwanda basic education board, schools, and educational stakeholders
Rwanda Basic Education Board should consider integrating smart classrooms in more schools to enhance students’ learning experience. Rwanda Basic Education Board, and other Educational stakeholders should periodically assess the effectiveness of CAMIS, gather feedback from teachers and students, and make necessary adjustments to maximize their impact. Schools should be encouraged to adopt a Comprehensive Assessment Management System to monitor students’ progress, identify areas for improvement, and customize remediation strategies accordingly. Rwanda Basic Education Board and other educational stakeholders are training teachers on utilizing smart classroom technology, incorporating interactive teaching methods, and utilizing assessment management systems to further enhance their pedagogical skills and effectiveness. School administrations and Sector Education Officer should encourage learning platforms that facilitate interaction between students and teachers outside the traditional classroom setting.
Further research
Researchers are encouraged to extend their investigations to other subjects beyond physics to evaluate the effectiveness of SM and CAMIS across various academic disciplines.
Authors’ contributions
This work was carried out in collaboration among all authors. JNT took charge of study design, data collection, analysis, and manuscript drafting. CN made significant contributions by assisting in the literature review and as a supervisor. All authors participated in the review and approved the final manuscript.
Acknowledgments
We express our heartfelt gratitude for the cooperation and active participation of both the students from G.S. Mushongi and the secondary physics teachers, whose invaluable support enabled the successful execution of this study. We express our thanks also to Dr. Ndihokubwayo Kizito for his guidance and support, especially in the area of grammar checking.
Data availability statement
Regarding data availability, interested parties can obtain the dataset used in the study upon request by contacting the corresponding author.
Disclosure statement
No potential conflict of interest was reported by the author(s).
Additional information
Funding
Notes on contributors
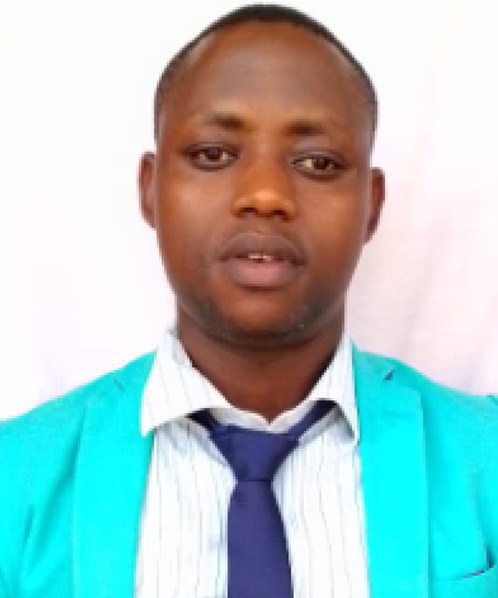
Jean Nepomuscene Twahirwa
Jean Nepomuscene Twahirwa, a master’s graduate in Physics Education from the African Center of Excellence for Innovative Teaching and Learning Mathematics and Science (ACEITLMS) at the University of Rwanda-College of Education, is currently pursuing a master’s in Astrophysics with a focus on Cosmology at Mbarara University of Science and Technology (MUST). With a track record of scholarly publications, his research interests lie at the intersection of physics education and astrophysics. His investigation into the effects of smart classrooms and comprehensive assessment management information systems (CAMIS) on student and teacher performance addresses critical issues in science education. By integrating advanced technological tools and remediation learning techniques, his study seeks to identify and support students struggling to meet academic competencies.
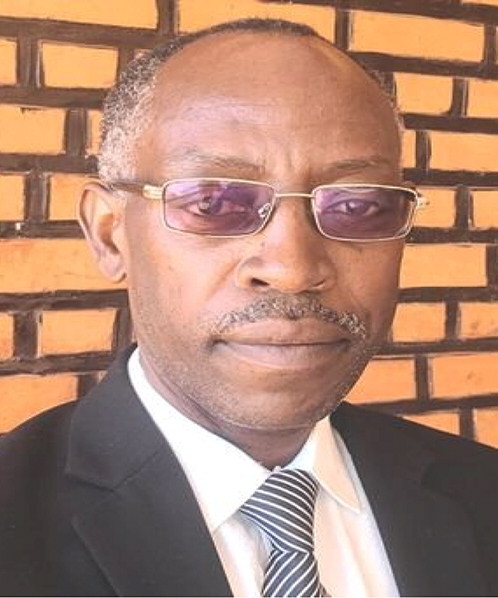
Celestin Ntivuguruzwa
Celestin Ntivuguruzwa, holding a PhD in Physical and Mathematical Sciences from Saint Petersburg State University, Russia, boasts over 23 years of experience in teaching, research, leadership, and community engagement. As an Associate Professor of Physics and Mathematics Education at the University of Rwanda College of Education, he has published numerous papers in physics and mathematics education and serves on various national, regional, and international boards and committees. His leadership in the Mastercard Foundation Scholars Program and involvement in various educational boards underscore a commitment to improving access to quality education and fostering academic excellence. Thus, this research contributes to ongoing efforts to enhance teaching and learning practices, ultimately benefiting students, teachers, and educational institutions.
References
- Adeleke, A. A., & Joshua, E. O. (2015). Development and validation of scientific literacy achievement test to assess senior secondary school students ’ literacy acquisition in physics, 6(7), 28–43.
- Al-Maroof, R. S., Alahbabi, N. M. N., Akour, I., Alhumaid, K., Ayoubi, K., Alnnaimi, M., Thabit, S., Alfaisal, R., Aburayya, A., & Salloum, S. (2022). Students’ perception towards behavioral intention of audio and video teaching styles: An acceptance study. International Journal of Data and Network Science, 6(2), 603–618. https://doi.org/10.5267/j.ijdns.2021.11.004
- Anderson, S. E., & Maninger, R. M. (2007). Preservice teachers’ abilities, beliefs, and intentions regarding technology integration. Journal of Educational Computing Research, 37(2), 151–172. https://doi.org/10.2190/H1M8-562W-18J1-634P
- Asio, J. M. R., & Jimenez, E. C. (2020). Effect of remediation activities on grade 5 pupils. Academic Performance in Technology and Livelihood Education (TLE), 5(4).
- Balta, N., & Eryılmaz, A. (2010). Development of modern physics achievement test : Validity and reliability study. https://doi.org/10.31757/euer.313
- Celestin, N., Pascal, N., Faustin, N., Violette, U., & Jean Baptiste N. (2021). Effect of smart classroom on learners’ performance in organic chemistry: A case study of senior five students in Kicukiro district-Rwanda. GSC Biological and Pharmaceutical Sciences, 16(2), 204–211. https://doi.org/10.30574/gscbps.2021.16.2.0251
- Chu, C., Hsu, A. L., Chou, K. H., Bandettini, P., & Lin, C. P, Alzheimer’s Disease Neuroimaging Initiative. (2012). Does feature selection improve classification accuracy? Impact of sample size and feature selection on classification using anatomical magnetic resonance images. NeuroImage, 60(1), 59–70. https://doi.org/10.1016/j.neuroimage.2011.11.066
- Dushimimana, J. C., & Uworwabayeho, A. (2020). Teacher training college student performance in statistics and probability exams in Rwanda. Rwandan Journal of Education, 5(1), 68–81.
- Germanakos, P. (2024). “It’s time!” Toward a human-AI quantum experience design paradigm: Reinventing the theoretical framework of HCI. In Extended Abstracts of the CHI Conference on Human Factors in Computing Systems (pp. 1–6).
- Göktaş, E., & Kaya, M. (2023). The effects of teacher relationships on student academic achievement: A second order meta-analysis. Participatory Educational Research, 10(1), 275–289. https://doi.org/10.17275/per.23.15.10.1
- Iyamuremye, F., & Ndagijimana, J. B. (2022). Challenges facing the use of multimedia in teaching and learning in secondary schools in Muhanga District, Rwanda. World Journal of Vocational Education and Training, 5(9), 86–109.
- James, A. O., & Folorunso, A. M. (2012). Effect of feedback and remediation on students’ achievement in junior secondary school mathematics. International Education Studies, 5(5), 153–162. https://doi.org/10.5539/ies.v5n5p153
- Kalyoma, M. O. (2016). Effectiveness of remedial teaching as intervention program against low achievement in mathematics among secondary school students in Tanzania.
- Kanamugire, C., & Rutakamize, J. (2008). The remedial programme for out-of-school and drop-out children in Rwanda. PROSPECTS, 38(2), 237–246. https://doi.org/10.1007/s11125-008-9065-y
- Kanyesigye, S. T., Uwamahoro, J., & Kemeza, I. (2022). Difficulties in understanding mechanical waves: Remediated by problem-based instruction. Physical Review Physics Education Research, 18(1). https://journals.aps.org/prper/accepted/99074L91A641480370857b82c918eea0b009ef16e https://doi.org/10.1103/PhysRevPhysEducRes.18.010140
- Kumar, G. S., & Bavankumar, S. (2024). Human computer interaction in daily life using deep. Social Science Journal, 14, 11–18.
- Luoch, T. O. (2017). Readiness: The key factor in remedial teaching and learning. European Journal of Social & Behavioural Sciences, 18(1), 25–41. https://doi.org/10.15405/ejsbs.203
- Majernik, J. (2018). E-assessment management system for comprehensive assessment of medical students knowledge [Paper presentation]. Proceedings of the 2018 Federated Conference on Computer Science and Information Systems, FedCSIS 2018, 15, 795–799. https://doi.org/10.15439/2018F138
- Mugiraneza, J. P. (2021). Digitalization in teaching and education in Rwanda.
- Mukagihana, J., Nsanganwimana, F., & Aurah, C. (2020). Biology instructional resources availability and extent of their utilization in teaching pre-service biology teachers. African Journal of Educational Studies in Mathematics and Sciences, 16(2), 33–50. https://doi.org/10.4314/ajesms.v16i2.3
- Mushimiyimana, J. D. D., & Dushimimana, J. D. D. (2021). The relationship between smart classroom and learners’ academic performance in public boarding schools of Gicumbi District, Rwanda. Inkanyiso, 13(2), 14. https://doi.org/10.4102/ink.v13i2.5
- Ndihokubwayo, K. (2024). Information technologies in African physics classroom : A scientometric study. Educational Journal of Technology and Innovation, 1(1), 1–37. https://doi.org/10.58197/hwx7hx58
- Nsabayezu, E., Iyamuremye, A., Mukiza, J., Mbonyiryivuze, A., Gakuba, E., Niyonzima, F. N., & Nsengimana, T. (2023). Impact of computer-based simulations on students’ learning of organic chemistry in the selected secondary schools of Gicumbi District in Rwanda. Education and Information Technologies, 28(3), 3537–3555. https://doi.org/10.1007/s10639-022-11344-6
- Nsabayezu, E., Mukiza, J., Iyamuremye, A., Mukamanzi, O. U., & Mbonyiryivuze, A. (2022). Rubric-based formative assessment to support students’ learning of organic chemistry in the selected secondary schools in Rwanda: A technology-based learning. Education and Information Technologies, 27(9), 12251–12271. https://doi.org/10.1007/s10639-022-11113-5
- Nsengimana, T., Mugabo, L. R., Ozawa, H., & Nkundabakura, P. (2021). Science competence-based curriculum implementation in Rwanda: A multiple case study of the relationship between a school’s profile of implementation and its capacity to innovate. African Journal of Research in Mathematics, Science and Technology Education, 25(1), 38–51. https://doi.org/10.1080/18117295.2021.1888020
- Nyirahabimana, P., Minani, E., Nduwingoma, M., & Kemeza, I. (2023). Assessing the impact of multimedia application on student conceptual understanding in quantum physics at the Rwanda College of Education. Education and Information Technologies, 29(3), 3423–3444. https://doi.org/10.1007/s10639-023-11970-8
- Nyirahagenimana, J., Uwamahoro, J., & Ndihokubwayo, K. (2022). Assessment of physics lesson planning and teaching based on the 5Es instruction model in Rwanda secondary schools. Contemporary Mathematics and Science Education, 3(1), ep22004. https://doi.org/10.30935/conmaths/11573
- Omirzak, I., Alzhanov, A., Kartashova, O., & Ananishnev, V. (2022). Integrating mobile technologies in a smart classroom to improve the quality of the educational process: Synergy of technological and pedagogical tools. World Journal on Educational Technology: Current Issues, 14(3), 560–578. https://doi.org/10.18844/wjet.v14i3.7194
- Paulin, M., & Mugiraneza, F. (2023). Effects of remedial activities on students’ competences in mathematics subjects in public secondary schools in Rwanda: A case of Ngororero District. Cognizance Journal of Multidisciplinary Studies, 3(12), 71–87. https://doi.org/10.47760/cognizance.2023.v03i12.008
- Pellegrino, J. W., & Quellmalz, E. S. (2010). Perspectives on the integration of technology and assessment. Journal of Research on Technology in Education, 43(2), 119–134. https://doi.org/10.1080/15391523.2010.10782565
- Reylene, O. E. (2022). Worksheet: Remedial materials in teaching mathematics 10. International Journal of Research Publications, 106(1), 363–379. https://doi.org/10.47119/IJRP1001061820223752
- Saini, M. K., & Goel, N. (2019). How smart are smart classrooms? A review of smart classroom technologies. ACM Computing Surveys, 52(6), 1–28. https://doi.org/10.1145/3365757
- Teo, T. (2015). Comparing pre-service and in-service teachers’ acceptance of technology: Assessment of measurement invariance and latent mean differences. Computers & Education, 83, 22–31. https://doi.org/10.1016/j.compedu.2014.11.015
- Thomas, F. B. (2022). The role of purposive sampling technique as a tool for informal choices in a social sciences in research methods. 2(5).
- Tuyizere, G., & Yadav, L. L. (2023). Effect of interactive computer simulations on academic performance and learning motivation of Rwandan students in atomic physics. International Journal of Evaluation and Research in Education (IJERE), 12(1), 252–259. https://doi.org/10.11591/ijere.v12i1.23617
- Ukobizaba, F., Ndihokubwayo, K., Mukuka, A., & Uwamahoro, J. (2019). Insights of teachers and students on mathematics teaching and learning in selected Rwandan secondary schools. African Journal of Educational Studies in Mathematics and Sciences, 15(2), 93–106. https://doi.org/10.4314/ajesms.v15i2.8
- Uwineza, I., Uworwabayeho, A., & Yokoyama, K. (2023). Perceptions of using interactive mathematics software among Rwandan primary school teachers. Cogent Education, 10(1). https://doi.org/10.1080/2331186X.2023.2170113
- Wallet, P., & Kimenyi, E. (2019). Improving quality and relevance of education through mobile learning in Rwanda : A promise to deliver. United Nations Educational, Scientific and Cultural Organization.
- Wu, B. (2016). Analysis on the smart classroom and innovation mode. Physics Teaching Based on MOOC E-Learning Platform, 10(8), 369–380.