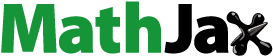
Abstract
A dynamic system consisting of donations and various sports’ success is estimated to examine relationships among winning and donations. Differences between Power 5, Non-power 5, and Football Championship Series alignments exist. Evidence that successful athletic programs have a positive impact on donations is found regardless of the conference alignment, but the impact varies by sport and alignment. The effects, however, are short lived. Donations, however, appear to have little impact on winning. There is some degree of interaction among winning in different sports.
Public Interest Statement
Collegiate athletic departments are reliant on donations to operate. Understanding how winning influences donations and how donations influence winning is critical to fundraisers in their quest to maintain and increase donations. A dynamic system of equations consisting of donations to U.S. universities athletic programs and various sports’ success is estimated to provide information on (1) the relationships among winning and donations and (2) interactions among winning between sports programs within a university. Evidence that successful athletic programs have a positive impact on donations is found, but the impact varies by sport and conference alignment. The effects, however, are short lived; suggesting fundraisers must continually wine and dine donors. Donations, however, appear to have little direct impact on winning. There is some degree of synergism among winning in different sports; winning in one sport’s program can lead to winning in other sports’ programs. This synergism, however, is also short lived leading to only small increases in winning.
1. Introduction
Organized U.S. intercollegiate sporting events began in 1852 when the rowing teams of Yale and Harvard competed at Lake Winnipesaukee, New Hampshire (Lewis, Citation1970). In 1869, the Scarlet Knights of Rutgers met the Princeton Tigers on the football field, marking the first collegian football game (Ours, Citation1999). From these meager beginnings, intercollegiate athletics has been transformed into a vital role for universities. One may view universities’ roles as having changed from the three traditional roles of research, teaching, and outreach/extension to include a fourth vital role, entertainment. As an example of these changes, consider donations to athletic departments. Average donations per athletic department in nominal dollars increased from $116,000 in 1968–1969 to $437,000 in 1981–1982 (Coughlin & Erekson, Citation1984) to $5,075,720 in 2004 to 2011 (Berkowitz, Schnaars, & Upton, Citation2012).
University athletic programs use revenue-generating sports such as football and men’s basketball to provide funding for sports that do not generate profits (Burk & Plumly, Citation2003). Gate revenues from these sports, however, may be insufficient to support athletic programs (Coughlin & Erekson, Citation1984). As such, athletic departments rely on other revenue categories including donations to keep programs operating (Grant, Leadley, & Zygmont, Citation2008). Donations to athletic departments are vital in today’s athletic departments being used to maintain and improve facilities and programs (Grant et al., Citation2008). Even including donations and a broad definition of financially self-sufficient, only 53 athletic programs at public universities are self-sufficient (Brady, Berkowitz, & Schnaars, Citation2015). Of these 53 programs, 50 are in the Power 5 conferences (Brady et al., Citation2015).
Given the importance of donations to athletic programs, it is not surprising the number of studies addressing donations to such programs. This literature tends to follow two threads, analyzing reason why individuals contribute and examining total contributions to the program (see the literature review). Regardless of the thread, interrelations and dynamics are for the most part ignored. To address this deficiency, this study develops a dynamic system of donations to public universities’ athletic departments and athletic success to address the following questions: (1) What are the relationships between athletic success and exogenous factors?; (2) What are the dynamics between success in one sports program and success in other sports?; and (3) What are the dynamic relationships, if any, between donations and athletic success? The first question has been addressed in the literature exclusively (to the authors’ knowledge) in single-equation, non-dynamic settings. Exploring links between athletic programs asks questions such as does winning in football translate into basketball success and vice versa. Finally, the last question seeks to explore year-to-year relationships not only among successes in different sports, but also among athletic successes and donations. If athletic successes translate into increased donations as many studies conclude, is this increase short or long lived?
Specifically, the objective of this study is to analyze the dynamic relationships, if any, among donations to public universities’ athletic departments and athletic winning percentages. A dynamic model which includes donations to the athletic department, winning percentages for football, men’s’ basketball (henceforth, basketball), and a combined other sports’ winning percentage is developed. Because of potential alignment differences (Brady et al., Citation2015), the schools are arranged by the three conference groupings: Power 5, Non-power 5, and Football Championship Series. First, potential influences of covariates are removed using panel regression. Then using panel vector autoregression (PVAR), dynamic relationships between the variables are estimated. Dynamics relationships are examined using impulse responses and forecast error variance decompositions which is common in the times series literature.
Observational data on winning percentages in football, basketball, other sports and the corresponding athletic department donations from U.S. universities over the recent past are used. Using observational data creates limited possibilities to construct formal hypothesis testing as laid out in Haavelmo (Citation1944). That is, the approach is using what Haavelmo (Citation1944) labeled as passive observations as opposed to experimental data. This study, therefore, is empiricist along the lines suggested by Haavelmo (Citation1944, p. 14) “… we try to adjust our theories to reality as it appears before us.” Sims (Citation1980) formalized this notion for vector autoregression models. In the vector autoregression approach, every variable studied is permitted to affect every other variable studied with time lags, making for a system of endogenous variables. Using this approach, this study develops a dynamic system containing four endogenous variables (winning percentages in football, basketball and other sports and donations to athletic departments) giving rise to four equations each dependent on lagged (past) values of itself and the other three series.
As noted, two aspects of using this approach to model donations and winning are not in the literature. First, by allowing each variable to affect all other variables, the model can address question two by examining if winning in one sport is associated with winning in other sports and vice versa. Does a culture of winning occur or are the sports independent? Further by modeling as a system, the model allows not only winning to influence donations but also donations to influence winning. Second, dynamics allow the model to examine question three by looking at if the above effects exist, how long do the effects last? If a football winning season increases donations, for example, does this increase in donations last for one year or longer. Similar dynamic effects are obtained for all endogenous variables interacting with all other variables. This approach allows for a more complete modeling of athletic departments than the single equation approach of previous studies. These two additions to the literature, interactions and dynamics, are the contribution of this study.
2. Literature review
With the increasing dependence on donations in the 1970’s (Coughlin & Erekson, Citation1984), studies began looking into the effects of college athletics and their impact on universities’ donations (Reynolds, Citation2015). As previously noted, this literature tends to follow two threads. The first thread attempts to understand the motivations and behaviors of individual donors (Gladden, Mahony, & Apostolopoulou, Citation2005; Ko, Rhee, Walker, & Lee, Citation2014; Mahony, Gladden, & Funk, Citation2003; Staurowsky, Parkhouse, & Sachs, Citation1996; Verner, Hecht, & Fansler, Citation1998). These studies’ theoretical models are similar to the more general charitable giving models of services-philanthropic giving model (Brady, Noble, Utter, & Smith, Citation2002), the identity-salience model (Arnett, German, & Hunt, Citation2003), and the utility-maximizing model (Coughlin & Erekson, Citation1984). Inferences from this thread suggest that athletic programs’ successes positively influence donations.
The second thread attempts to explain total donations to universities or athletic departments. Although related to the first thread, there are obvious differences that translate into different variables used in explaining donations. This second thread is of main interest here. Sigelman and Carter’s (Citation1979, p. 287) review of early studies finds little evidence linking on field performance to alumni donations, “What we have is a wealth of speculation and a lack of conclusive evidence concerning the impact of athletic success on alumni giving.” Thirty years later, Martinez, Stinson, Kang, and Jubenville’s (Citation2010, p. 36) review opens with a similar statement studies “… have failed to generate generalizable knowledge.” Martinez et al. (Citation2010) conduct a meta-analysis of 14 studies between 1976 and 2008. Their findings indicate that intercollegiate athletics have a statistically significant but small influence on donations to universities.
Independent variables, study years, universities, and conferences included vary greatly between studies (Reynolds, Citation2015). Reynolds (Citation2015) summarizes previous studies by listing 139 variables that were included in 28 studies conducted between 1971 and 2014. Such diversity may help explain why mixed results are obtained. Some studies examine the effects of alumni donations attributed to athletic success (Baade & Sundberg, Citation1996; Grimes & Chressanthis, Citation1994); whereas, other studies analyze the effects athletics have on both alumni and non-alumni donations (Humphreys & Mondello, Citation2007; Stinson & Howard, Citation2004). Grimes and Chressanthis (Citation1994) examine only public schools, while Rhoads and Gerking (Citation2000) and Humphreys and Mondello (Citation2007) include both public and private schools. Still other studies examine the effects at a single university (Stinson & Howard, Citation2004), while most studies include multiple universities (Baade & Sundberg, Citation1996; Humphreys & Mondello, Citation2007; Stinson & Howard, Citation2008; Tucker, Citation2004).
Football success appears to display a stronger influence on donations than basketball or other sports (Baade & Sundberg, Citation1996; Goff, Citation2000; Humphreys & Mondello, Citation2007; McCormick & Tinsley, Citation1990; Rhoads & Gerking, Citation2000; Stinson & Howard, Citation2008). Mixed results for basketball are found. Of the many studies including basketball (Baade & Sundberg, Citation1996; Brooker & Klastorin, Citation1981; Coughlin & Erekson, Citation1984; Goff, Citation2000; Grimes & Chressanthis, Citation1994; Koo & Dittmore, Citation2014; Rhoads & Gerking, Citation2000; Sigelman & Bookheimer, Citation1983; Sigelman & Carter, Citation1979; Stinson & Howard, Citation2008; Tucker, Citation2004), only several find basketball success to have a statistically significant positive effect on donations (Brooker & Klastorin, Citation1981; Grimes & Chressanthis, Citation1994; Sigelman & Bookheimer, Citation1983; Stinson & Howard, Citation2008; Walker, Citation2015).
Post-season play has also been examined. Sigelman and Carter (Citation1979) and Goff (Citation2000) look specifically at football post season play, while Coughlin and Erekson (Citation1984), Grimes and Chressanthis (Citation1994), Baade and Sundberg (Citation1996), Rhoads and Gerking (Citation2000), and Tucker (Citation2004) analyze the effects of both appearances in bowl games and NCAA basketball tournament bids. Results suggest there is more of an effect on donations for attending a bowl game, than there is for accepting a bid to the NCAA basketball tournament.
Studies such as Grimes and Chressanthis (Citation1994) and Rhoads and Gerking (Citation2000) added baseball wins and losses, along with post-season play for football, basketball, and baseball, and athletic sanctions. Results suggest baseball success has no significant impact on donations. Grimes and Chressanthis (Citation1994), Rhoads and Gerking (Citation2000) and Hughes and Shank (Citation2008) find sanctions to decrease donations received by universities.
Sigelman and Bookheimer (Citation1983) and McCormick and Tinsley (Citation1990) suggest there is competition between college donations and attending professional sporting events. Distance from major cities is used as a proxy for competition to attend other venues and not contribute to universities. Other studies have shown positive correlations between the overall improvements in economic conditions and donations (Brooker & Klastorin, Citation1981; Coughlin & Erekson, Citation1984; Grimes & Chressanthis, Citation1994; Humphreys & Mondello, Citation2007; Koo & Dittmore, Citation2014; Murphy & Trandel, Citation1994; Stinson & Howard, Citation2007). See Sigelman and Carter (Citation1979), Martinez et al. (Citation2010), and Reynolds (Citation2015) for reviews of this literature. Besides the two threads discussed here a multitude of other issues (for example, see Anderson, Citation2017) associated with donations, athletics, and success are found in the literature, which is too large and diverse to discuss here.
3. Methodology
As previously discussed, a four equation dynamic system is estimated to address the questions of relationships among donations and athletic success and the dynamics of the system. Dependent (endogenous) variables are donations in millions and winning ratios (in decimals) for men’s football, men’s basketball, and an index of combined winning percentage for other sports in which year-end winning percentages are generated. Data (discussed later) are comprised of 160 universities over eight years, making the model a time series panel model. A two-step procedure is used to estimate the model to capture the effects of exogenous variables and dynamics.Footnote1
3.1. Step 1—Panel equation estimation
The first step is to remove the influence of the exogenous variables by estimating individual equations for the four dependent variables. In estimating the four equations, each equation contains the same variables; therefore, there is no efficiency gain using a systems estimator. The panel regression model (Greene, Citation2002) is(1)
(1)
where j represents the four equations for the endogenous variables (donations and the three spots winning percentages), i represents university, N varies by conference grouping, t the year, βj is the intercept, ϑitj is the dependent variable, ρijZitj is a K-dimensional row vector of exogenous variables, uij is an individual specific effect, and is an idiosyncratic error term. Xtreg panel estimation procedure which uses generalized least squares (assuming random effects) is used to estimate the equations (StataCorp, Citation2011).Footnote2
Based on data availability and previous studies, exogenous variables are:
FBS Bowl—football bowl game appearance lagged one year;
FBS Bowl Win—one if won bowl game lagged one year;
NCAA BB—NCAA basketball tournament appearance lagged one year;
Student—student enrollment in thousands;
Right licensing—the total amount of money the university receives for media rights in millions lagged one year;
Salary—total coaches’ salaries in millions;
GDPdiff—first differences in US gross domestic product in trillions of nominal dollar;
Distance—hundreds of miles from campus to the nearest city where the population center that exceeds 200,000;
Football conference alignment; and
Ticket donation tiers (No donation to $500—Tier 1 used as the base; $500 to $2,000—Tier 2; and $2,000 and above—Tier 3).Footnote3
All variables are reported on a calendar year basis. Overall, the variables are predetermined before the year reported for winning percentage or donations, minimizing potential endogeneity issues.
3.2. Step 2–Panel VAR estimation
PVAR methodology is particularly suited for this study because PVARs capture dynamic interdependencies while treating the links across units in a semi-unrestricted fashion (Canova & Cicarelli, Citation2013). PVARs are based on vector autoregressive models, but add the cross-sectional dimension (Canova & Cicarelli, Citation2013). Redefined (in terms of the exogenous effects being removed) endogenous variable are obtained for each observation by combining the individual specific effects, uij, and the idiosyncratic terms, . The redefined variables are then used to estimate the PVAR model
(2)
(2)
where αit is a 1 × 4 vector containing the redefined variables from the four panel estimations for university i, in year t, Γ1, are a matrix of coefficients to be estimated, and, is the vector of second stage error terms. The PVAR is estimated through generalized methods of moments, a heteroskedastic autoregressive consistent estimator of unknown parameters. The PVAR procedure was developed by Love and Zicchino (Citation2006) and implemented within StataCorp (Citation2011). With only eight years of data, the number of lags is assumed to be one in the PVAR. The PVAR form applied here does not allow exogenous variables; thus, the two step procedure is used. A Helmert transformation is used to remove fixed effects, following Love and Zicchino (Citation2006).
3.3. Post-estimation–Dynamic analysis
Because estimated coefficients from PVAR’s are difficult to interpret, dynamics among the four endogenous variables are examined through impulse response functions and forecast error variance decompositions (as suggested by Sims, Citation1980). To perform innovation accounting analysis, contemporaneous correlations must be removed. A Cholesky decomposition, which requires an ordering for the variables, is used to remove these correlations. The calendar timing of the variables is: donations and other sports span the entire year; basketball is at the beginning of the year; and football is at the end of the year. Given this timing, the assumed order is: basketball, football, and other sports winning percentages, and donations.
Impulse response functions are measures of the time profile of the effect of a shock on the behavior of each series (Koop, Pesaran, & Potter, Citation1996). Impulse response functions provide a visual measure of size and direction of responses. Forecast error variance decompositions are used to determine where information arises over time in the system. Endogenous variables receive most of their information from other variables series within the system (Olsen, Mjelde, & Bessler, Citation2014). A series whose information is dominated by itself are considered exogenous. “Perfectly endogenous” series is one in which all of its forecast error variance is explained by information arising from other series. If four variables, for example, are included in the system, the decompositions for a “perfectly endogenous” variable would be 0% for the perfectly endogenous variable and 100% (combined) for the other three variables. On the other extreme, if one of the four variables is “perfectly exogenous” its own decomposition would be 100%, whereas, decompositions attributed to the remaining variables would be 0%.
4. Data
Data from the years 2004 to 2011 for 160 public universities’ athletic departments in the United States are used (see Appendices A and B). Donations, coaches’ salaries, and right licensing are from Berkowitz et al. (Citation2012). The Voluntary Support of Education (VSE) database is used to obtain school student enrollments and to validate the donation information (Council for Aid to Education, Citation2014). On field performance measures, wins and losses come from the NCAA Archives (Citation2014).
Post-season play may help in recruiting athletes and gaining donations. Three measures of post-season play included are lagged values of the football program reaching a bowl game, winning the bowl game, and NCAA basketball tournament appearance. By including both participation and winning, one is able to determine if winning a bowl game is more important than just being invited to the bowl. Lagged NCAA basketball tournament appearance, similar to football bowl games, may be important in determining the success of the basketball program. Because the NCAA basketball tournament is a playoff system with a potential for several games, only appearance is considered. All three variables enter as 0–1 dichotomous variables. One difference in exogenous variables between the three models is that FCS teams participate in a football playoff series instead of bowl games. Participation in and winning a bowl game are replaced by a 0–1 variable representing if the team made the playoffs. Bowl wins are not included in the FCS model given the multigame nature of the playoff system.
Enrollment serves as a proxy for the size of the university, therefore, its alumni base. Media right licensing is included to help understand the effect of such payments to the university for televising their games. Adding this variable is a proxy for all games played on TV, and the potential benefit to donations and helping recruit to athletic programs because of national exposure. Coaches’ salaries may be important in determining teams’ success. Universities deliver large salaries to coaches who are proven winners or who have turned a program around and gained athletic success. One expects the higher the total salary for all coaches employed the more successful the athletic program.
The influence the economy has on donations is examined by including U.S. gross domestic product (GDP). Nominal GDP is from the U.S. Bureau of Economic Analysis (Citation2014). Distance to the nearest city with a population over 200,000 is used as a proxy for understanding if there is potential competition for donors’ funds between available sporting events. Distance is collected from Google maps (Google Maps, Citation2014). Conference alignment for football (NCAA Archives, Citation2014) is included as a 0–1 dichotomous variable based on the conference the football team participates. For some schools, conference alignment varies by year.
Many athletic departments require donations to be able to purchase football seats. Ticket donations are been broken into a three tiered system: no donation to $500, $500 to $2,000, and over $2,000 per seat donation. Tier levels are based on distribution of the tiers giving approximately one-third of the observations in each tier. The first tier is used as the base. Variables are assigned a 0 or 1 depending on which tier the university used for the 2014 season. Data were obtained through telephone calls to each university’s athletic department ticket office or the universities’ website. Other studies have looked at NCAA sanctions; however, only 8 of the 1,280 observations (school and year) encompass sanctions (NCAA Archives, Citation2014); therefore, sanctions are not included in the model.
5. Results and discussion
Three models are estimated based on the football conference alignments: Power 5 conferences; Non-power 5 conferences but teams are aligned with the Football Bowl Subdivision (FBS); and conferences aligned with Football Championship Subdivision (FCS). Within the FCS model, several conferences are combined based on similarities because data is available for only a limited number of schools. The reason for estimating three models is because of potential differences in athletic programs and schools that may not be captured by the exogenous variables among the three different alignments. A significance level of five percent is assumed in the discussion; however, exact p-values are given in the tables.
5.1. Panel estimation
5.1.1. Power 5 conferences
Coaches’ salaries is the only variable statistically significant in the donation equation; salaries are positively related to donations (Table ). Every million dollar increase in total coaching staff salaries is associated with a $0.535 million increase in donations. None of the conferences are significantly different from the other conferences (Tables and ). For football winning percentage, FBS bowl, coaches’ salary, and SEC are positive and significant. Right licensing is negative and significant. Only participating in the NCAA basketball tournament last year is significant in the basketball winning percentage model; the coefficient is positive. Distance from a major city is associated with a decrease in other sports winning percentages; whereas, Tier 3 is associated with an increase in winning percentage.
Table 1. Panel regressions – Power 5 Conferences, Pacific-12 Conference is the base conferenceTable Footnote1
Table 2. p-values of differences in contributions between Power 5 Conferences using Pairwise F-tests, Pacific-12 Conference is the base conference
5.1.2. Non-power 5 conferences
Similar to the Power 5 conferences, coaches’ salaries are positively related to donations (Table ); each $1 million in coaches’ salaries increases donations by $0.472 dollars. Larger student bodies and increases in right licensing are associated with smaller donations. Conference differences are found for donations (Tables and ). Schools in the Big East Conference tend to have larger donations than that of schools in the other conferences. MAC schools tend to have smaller donations than schools in C-USA and MWC. Participation in the post season is positively associated with football and basketball winning percentages. Distance and Tier 3 variables are negative and significant in the basketball winning percentage equation; whereas, right licensing is positive and significant. Other than the constant, no variable is significant in the other sports equation.
Table 3. Panel regressions – Non-Power 5 Conferences, Western Athletic Conference is the base conferenceTable Footnote1
Table 4. p-values of differences in contributions between Non-power 5 Conferences using Pairwise F-tests, Western Athletic Conference is the base conference
5.1.3. FCS conferences
As with the Non-power 5 model, some differences in conferences in donations are seen (Tables and ). Schools in the MEAC/SWAC and OVC/PFL conferences generally have smaller donation levels than schools in the other conferences. Increases in coaches’ salaries increases donations, Participating in the FCS playoffs and distance are positively related to football winning percent. Other than the constant no variable is significant in the basketball winning percentage equation. Participation in the NCAA basketball tournament is associated with an increase in other sports winning percentages. Some conference differences are seen in football and other sports winning percentages.
Table 5. Panel regressions – FCS Conferences, Southland Conference is the base conferenceTable Footnote1
Table 6. p-values of differences in contributions between FCS Conferences using Pairwise F-tests, Southland Conference is the base conference
5.1.4. Panel models discussion
Several similarities and differences are seen in the above three models. In all models, it appears hiring high profile coaches is associated with increases in donations to the athletic program. No statistically significant differences in conference alignment are found in donations for the Power 5 conferences. Donations amounts cannot explain schools switching conferences for the Power 5 conferences, some other factor(s) must be at play. (It should be noted, studies such as Pincin and Hoffer (Citation2013) find conference changes can influence revenues). In the Non-power 5 model, schools in the Big East Conference tend to have larger donations amounts than schools in the other conferences. One potential reason for the differences in the Power 5 and Non-power 5 conferences model is the inclusion of the Big East in the Non-power 5 model. The Big East is a powerhouse conference in basketball; differences between this conference and the other Non-power 5 conferences are seen. The conference inclusion, however, is based on football alignment.
The influence of coaches’ salaries on football winning percentage varies by model. Recall, salary is the total of all coaches’ salaries; however, given the number of football coaches, salary will reflect football salaries. Increased salaries are associated with increases in football winning percentages in the Power 5 conferences, but are not significant in the Non-power 5 and FCS conferences. Additional studies are necessary to determine why this is the case, including breaking down salaries by sports. This finding is partially in-line with studies finding increased expenditures lead to on-field success (Jones, Citation2013).
Participation in post season play increases winning percentages. Postulated reasons include: (1) post season play is an indicator of a winning program; and (2) the publicity generated helps in recruiting. Distance from a large city appears not to be a disadvantage when it comes to recruiting in FCS conferences, but may be a disadvantage in the Power 5 and Non-power 5 conferences. Distance is significant and negative for basketball in the Non-power 5 alignment and other sports for the Power 5 alignment, but positive for football in FBS alignment. Right licensing is more important in explaining donations and winning percentages in the Power 5 and Non-power 5 alignments than in the FCS schools.
5.2. PVAR
5.2.1. Impulse response functions
Impulse response functions depicting how donations respond to shocks in the endogenous variables are presented in Figure . The most prominent feature in the graphs is that any shock to an endogenous variable has a short-lived effect lasting only for the initial period. A second feature is the large confidence intervals, especially for the Non-power 5 alignment. The large intervals are most likely because of the heterogeneity of the schools. For the Power 5 alignment, a shock to any of the variables increases donations for the initial period. In the Non-power 5 alignment, a shock to any variable except football increases donations for the initial period. A shock to football decreases donations for one period. For FCS schools, a shock to basketball and donations increases donations; whereas, a shock to football and other sports decreases donations. After the initial period, no shocks have effects in which zero is outside the 95% confidence interval for any of the models.
Figure 1. Responses of donations to a one time shock in the endogenous variables (listed beneath the graphs) along with 95% confidence interval.
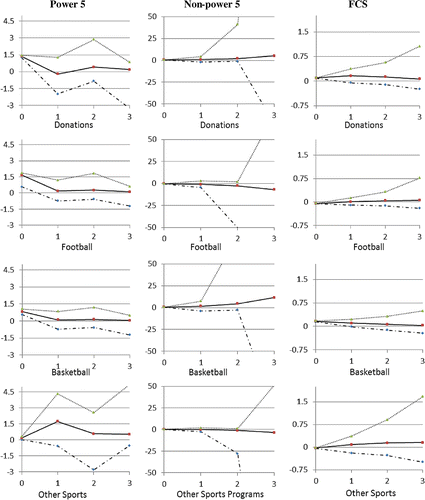
Winning percentages responds to shocks in the endogenous variables are presented in Figures . Regardless of the alignment, winning percentages do not respond to shocks in donations (zero lies within the 95% confidence interval). For all alignments, each sport’s responses to a shock in itself are positive lasting for one period. Basketball does not respond to shocks in football or other sports. In the Power 5 alignment, other sports responses to a shock in football are significant and positive lasting for the initial period, but negative for a basketball response. Other sports responses to a shock in football are positive for Non-power 5 alignment. For the FCS alignment, both football and other sports respond statistically significant to a shock in basketball lasting for one period.
Figure 2. Responses of winning percentages (listing in each column row) to a one time shock in the endogenous variables (listed beneath the graphs) for Power 5 Conferences along with 95% confidence interval.
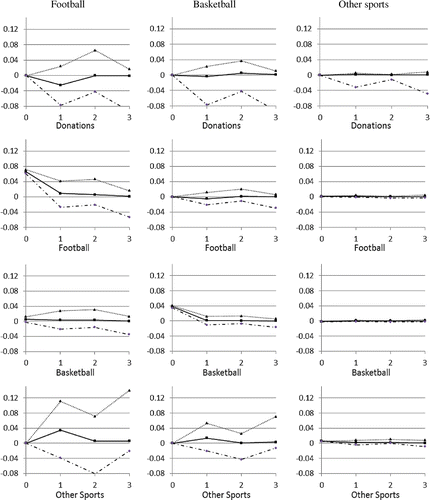
5.2.2. Forecast error variance decompositions
Forecast error variance decompositions (FEVD) for the two conference alignments show similarities and differences (Tables ). Donations are primarily explained by donations itself, and football and winning percentage for the Power 5 alignment (Table ). Dependent on the period, the percentage of donations explained by itself is between 22–34%. The percentage explained by football winning percentage starts at 52% and decreases to 31%. Other sports programs winning percentage explains 0% of donations initially but increases to 39%. Basketball explains between 7 and 13% of donations. Regardless of the sport, over 69% is explained by itself. The percentage of football winning percentage in Power 5 conferences, for example, explained by football (Table ) decreases from 100% in the initial period to 70% by the third period. Basketball and other sports are more exogenous than football with over 80% of the variation explained by itself. Other sports explain 12% or more for the three winning percentages variables.
Table 7. Forecasts error variance decompositions for the Power 5 Conferences from the PVAR assuming a Cholesky decomposition order of basketball, football, other sports programs, and donations
Table 8. Forecasts error variance decompositions for the Non-power 5 Conferences from the PVAR assuming a Cholesky decomposition order of basketball, football, other sports programs, and donations
Table 9. Forecasts error variance decompositions for the FCS Conferences from the PVAR assuming a Cholesky decomposition order of basketball, football, other sports programs, and donations
Although there are differences initially, the FEVD are similar by the third period for all four endogenous variables (Table ) for the Non-power 5 conferences. Donations are explained by about 12% by itself with football explaining approximately 22% and basketball approximately 60%. Other sports explain approximately 5% of donations. Basketball is more exogenous than football or other sports. For the FCS conferences, donations FEVD are similar to the Power 5 conferences with basketball replacing football in importance (Table ). The percentage of donations explained by itself starts at 24% and increases to 36%. Other sports winning percentage starts at two percent but increases 31%. The percentage of donations explained by basketball winning starts at 67% and decreases to 28%. Football winning percentage remains around four percent throughout the periods. Basketball and other sports winning percentages are more exogenous than football winning percentage, with each explaining over 53% themselves in each winning percentage models. Similar to the Power 5 alignment, other sports explain over 14% regardless of variable or period after the initial period.
6. Conclusions
This study posed three questions to be addressed: relationships between athletic success and exogenous factors; the dynamics among success in different sports; and the dynamic relationships between donations to public universities’ athletic departments and athletic success. To address these issues first panel models are estimated to remove the impact of exogenous covariates. Endogenous variables with the exogenous effects removed are used in estimating dynamic systems for three conference alignments to address the dynamic questions.
6.1. Exogenous variables
While similarities are found, differences between the classifications of the conferences are found in terms of significance of exogenous variables. Conference differences in donations are not found between the Power 5 Conferences, but conference differences are found in the Non-power 5 and FCS conferences. Which conferences and schools included in a study may lead to different inferences. Schools in the Non-power 5 conferences are more heterogeneous than in the other classifications. Although further research on this issue is necessary, results suggest studies need to carefully consider how to group conferences and schools. Further, the p-values for several of the variables indicate significance levels at or below 15%. In other samples these variables may be considered significant which may help explain why previous results vary by study. Level of significance considered and sample has some influence on inferences.
Hiring high profile coaches (increase in salary) tends to increase donations to the athletic departments. Increase in salaries, however, may not pay for themselves in terms of donations; a dollar increase in salaries brings about a direct increase in donations of $0.08 to $0.54 in contemporaneous time. The Power 5 alignment has the highest return, which is most likely related to football programs and all the media attention given to new coach hires and the media savvy of high profile coaches. With regard to winning percentages, obtaining post season play is important in increasing next seasons’ winning percentage. The most likely reason is the additional exposure which helps recruiting.
6.2. Dynamic interrelationships
Any dynamic effects on donations and winning are generally short-lived. Results cohere with a university sports climate consistent with a “What have you done for me lately?” environment. (They may well be consistent with other motivational structures as well.) Short-lived effects indicate for schools to maintain and increase donations they need to continuous expend effort on fund raising. A similar need is necessary to maintain winning within each sport.
The Power 5 and FCS systems are similar except for the importance of football and basketball winning percentages on donations. In the Power 5 conferences, football explains over 31% of the variance in donations with basketball winning percentages explaining a minor portion; whereas, in the FCS conferences basketball explains about 28% with football helping to explain a modest proportions of variance in donations. One likely reason for this is the difference in the level of division played for football and basketball. Power 5 conferences play at the highest level of both football and basketball, whereas, FCS play at a lower level in football, but most play Division I in basketball. Generally, the higher the level played the more media exposure. In both the Power 5 and FCS conferences other sports’ winning percentages play a role in explaining donations forecast error variances. Most likely it is not the other sports winning that directly increase donations, but the variable is a proxy for overall commitment of the athletic department. Overall commitment to entire athletic program and not just to a single sport increases donations to the athletic department. Other sports, however, do not play as large of a role in Non-power 5 conferences. It is not clear why this is the case.
Donations, however, do not necessarily translate into increased winning. In the impulse response functions, winning percentage responses to a shock in donations show no significant response. Further, forecast error variance decompositions show donations explain less than 12% of the variance in winning percentage for any sport in the Power 5 and Non-power 5 conferences. For the FCS, decompositions show donations explain 20% of the variance in football and other sports by period three but less than 5% for basketball. This difference in the importance of donations is most likely related to the size of the sports program and revenue from attendance. It generally appears to be a one-way street, winning helps increase donations, but donations do not necessarily increase winning.
Although there is some degree of information exchanged among the different sports programs, there still is a high level of exogeneity between the sports. For the Power 5 and FCS alignments, information on winning percentage arises in both football and other sports. For the Non-power 5 conferences, more information arises in football and basketball than arises from other sports. If there is a culture of winning it is weak.
The dynamic nature of donations giving needs to be further explored. It appears donations are short-lived. Data limitations do not allow for an answer only speculation as to why. Is it because large one-time donations are driving the amounts or the finicky nature of donors giving not only to universities, but giving in general? Donations to the athletic department and universities, in general, are, and will continue to play, a major component of universities’ budgets; this role provides a fruitful area of research to help understand the broader issue of nonprofit giving. The relationship between overall commitment to sports programs and donations may provide a fruitful avenue for further research. Is there a cultural of winning? If so, does the lack of a clear link from donations to subsequent winning suggest a weakening of this culture? Or are donations better understood as support of the athletic program, regardless whether performance materializes? Knowing one is playing a role in continuing the legacy of the programs of our universities may be incentive enough.
Available data was used at the time of this study. Because the general conferences and NCAA structural alignment has not changed, it is expected the results will generally transfer to current time; however, using data from additional years as they become available, it would be interesting to examine if the generating structure in the model shows evidence of changing. Finally, the results are for U.S. universities. Cugnon (Citation2015) notes the prevalence of sports is a major difference between U.S. and European universities. Because the importance of collegiate athletics in European is small but increasing and donations are in the conversations (European University Sports Association, Citation2017), European universities may learn from U.S. experiences; universities’ roles may be changing to include sports’ entertainment.
Funding
The authors received no direct funding for this research.
Acknowledgements
The authors thank the various athletic departments that provided information. Further, we thank the Department of Agricultural Economics Texas A&M University seminar attendees for helpful comments. Comments by Dr James Flagg throughout this study are greatly appreciated.
Additional information
Notes on contributors
Ryan Reynolds
Ryan Reynolds holds a master’s degree from Texas A&M University. The basis for this paper is his master’s thesis. Currently, Ryan is a Trader at Pedestal Commodity Group, Hartley, TX.
James W. Mjelde
James W. Mjelde and David A. Bessler are professors in the Department of Agricultural Economics at Texas A&M University. Dr Mjelde is a natural resource economist with degrees from Montana State University and the University of Illinois. Dr Mjelde’s research currently involves issues influencing universities.
David A. Bessler
David A. Bessler is a time series econometrician, trained at the University of Arizona and University of California, Davis, specializing in uncovering the dynamics of economic systems.
Notes
1. The procedure relies on as a first approximation the Frisch-Waugh and Orthogonal Partitioned Regression theorems (Baltagi, Citation2002; Greene, Citation2002) under conditions of orthogonal variables allow one to use only the residuals from estimated equations in further analysis. Although the Frisch-Waugh theorem has only been proven for ordinary least squares, this study assumes it holds for generalized least squares using panel data.
2. Using random effects allows for conference dichotomous variables to be included in the model to test for conference differences. Inferences between fixed and random effects models estimated using different estimators are similar. Using robust errors does not allow for using of Hausmam test for model specification (StataCorp, Citation2011).
3. In addition to these variables, an attempt was made to consider school rankings, however, no ranking including all schools was found. Complete ranking for the Power 5 conferences was considered, but these rankings had no significant effect on donations. As such, rankings are omitted from further consideration.
References
- Anderson, M. L. (2017). The benefits of college athletic success: An application of the propensity score design. Review of Economics and Statistics, 99, 119–134.10.1162/REST_a_00589
- Arnett, D. B., German, S. D., & Hunt, S. D. (2003). The identity salience model of relationship marketing success: The case of nonprofit marketing. Journal of Marketing, 67, 89–105.10.1509/jmkg.67.2.89.18614
- Baade, R., & Sundberg, J. (1996). Fourth down and goal to go? Assessing the link between athletics and alumni giving. Social Science Quarterly, 77, 789–803.
- Baltagi, B. H. (2002). Econometrics (3rd ed.). New York, NY: Springer.10.1007/978-3-662-04693-7
- Berkowitz, S., Schnaars, C., & Upton, J. (2012). Retrieved 2013, May 8 from http://www.usatoday.com/sports/college/schools/finances/ and http://usatoday30.usatoday.com/sports/graphics/datatables/2012/0515-NCAAFinance/NCAAFinance.htm
- Brady, E., Berkowitz, S., & Schnaars, C. (2015, May 26). College athletics finance report: Non-power 5 schools face huge money pressure. USA Today. Retrieved 2016, May 20 from http://www.usatoday.com/story/sports/college/2015/05/26/ncaa-athletic-finances-revenue-expense-division-i/27971457/
- Brady, M. K., Noble, C. H., Utter, D. J., & Smith, G. E. (2002). How to give and receive: An exploratory study of charitable hybrids. Psychology and Marketing, 19, 919–944.10.1002/(ISSN)1520-6793
- Brooker, G., & Klastorin, T. (1981). To the victors belong the spoils? College athletics and alumni giving. Social Science Quarterly, 62, 744–750.
- Burk, M., & Plumly, N. (2003). Who owns sports – The politics of Title IX. Marquette Sports Law Review, 14, 49–55.
- Canova, F., & Cicarelli, M. (2013). Panel vector autoregressive models a survey. VAR models in macroeconomics–New developments and applications: Essays in Honor of Christopher A. Sims. Advances in Econometrics, 32, 205–246. Firenze: Emerald Group Publishing Limited.
- Coughlin, C., & Erekson, O. H. (1984). An examination of contributions to support intercollegiate athletics. Southern Economic Journal, 51, 180–195.10.2307/1058331
- Council for Aid to Education. (2014). VSE data miner system. 2004–11 Fiscal Year. Retrieved 2014, February 3 from www.cae.org
- Cugnon, M. (2015). A hop across the pond: Comparing European vs. American college experiences. USA Today College. Retrieved 2017, April 29 from http://college.usatoday.com/2015/08/27/europe-vs-united-states-college-experience/
- Doan, T. (2000). RATS: User’s guide version 5. Evanston, IL: Estima.
- European University Sports Association. (2017). About EUSA. Retrieved 2017, April 29 from https://www.eusa.eu/eusa/about-eusa
- Gladden, J. M., Mahony, D. F., & Apostolopoulou, A. (2005). Toward a better understanding of college athletic donors: What are the primary motives? Sport Marketing Quarterly, 14, 18–30.
- Goff, B. (2000). Effect of university athletics on the university: A review and extension of empirical assessment. Journal of Sports Management, 14, 85–104.10.1123/jsm.14.2.85
- Google Maps. (2014). Retrieved 2014, March 15 from https://www.google.com/maps/
- Grant, R., Leadley, J., & Zygmont, Z. X. (2008). The economics of intercollegiate sports. Hackensack, NJ: World Scientific.10.1142/6172
- Greene, W. H. (2002). Econometric analysis (5th ed.). Englewood Cliffs, NJ: Prentice Hall.
- Grimes, P., & Chressanthis, G. (1994). Alumni contributions to academics: The roll of intercollegiate sports and NCAA sanctions. American Journal of Economics and Sociology, 53, 27–40.10.1111/ajes.1994.53.issue-1
- Haavelmo, T. (1994). Probability approach in econometrics. Econometrica, Journal of the Econometric Society, 12, 1–115.
- Hughes, S. F., & Shank, M. D. (2008). Assessing the impact of NCAA scandals: an exploratory analysis. International Journal of Sports Management and Marketing, 3, 78–99.10.1504/IJSMM.2008.015962
- Humphreys, B., & Mondello, M. (2007). Intercollegiate athletic success and donations at NCAA division one institutions. Journal of Sport Management, 21, 265–280.10.1123/jsm.21.2.265
- Jones, W. A. (2013). Exploring the relationship between intercollegiate athletic expenditures and team on-field success among NCAA division I institutions. Journal of Sports Economics, 14, 584–605.10.1177/1527002511433469
- Ko, Y. J., Rhee, Y. C., Walker, M., & Lee, J. H. (2014). What motivates donors to athletic programs a new model of donor behavior. Nonprofit and Voluntary Sector Quarterly, 43, 523–546.10.1177/0899764012472065
- Koo, G., & Dittmore, S. (2014). Effects of intercollegiate athletics on private giving in higher education. Journal of Issues in Intercollegiate Athletics, 7, 1–16.
- Koop, G., Pesaran, M. H., & Potter, S. M. (1996). Impulse response analysis in nonlinear multivariate models. Journal of Econometrics, 74, 119–147.10.1016/0304-4076(95)01753-4
- Lewis, G. (1970). The beginning of organized collegiate sport. American Quarterly, 22, 222–229.10.2307/2711645
- Love, I., & Zicchino, L. (2006). Financial development and dynamic investment behavior: Evidence from panel VAR. The Quarterly Review of Economics and Finance, 46, 190–210.10.1016/j.qref.2005.11.007
- Mahony, D. F., Gladden, J. M., & Funk, D. C. (2003). Examining athletic donosrs at NCAA division I institutions. International Sports Journal, 7, 9–27.
- Martinez, J. M., Stinson, J. L., Kang, M., & Jubenville, C. B. (2010). Intercollegiate athletics and institutional fundraising: A meta-analysis. Sports Marketing Quarterly, 19, 36–47.
- McCormick, R. E., & Tinsley, M. (1990). Athletics and academics: A model of university contributions. In B. L. Goff, & R. D. Tollison (Eds), Sportometrics (pp. 193–204). College Station, TX: Texas A&M University Press.
- Murphy, R. G., & Trandel, G. A. (1994). The relation between a university’s football record and the size of its applicant pool. Economics of Education Review, 13, 265–270.10.1016/0272-7757(94)90014-0
- NCAA Archives. (2014). NCAA archived win loss records. Retrieved 2013, July 9 from http://web1.ncaa.org/stats/StatsSrv/careersearch
- Olsen, K., Mjelde, J. W., & Bessler, D. A. (2014). Price formulation and the law of one price in internationally linked markets: An examination of the natural gas markets in the USA and Canada. The Annals of Regional Science, 54, 117–142.
- Ours, R. (1999). Introduction: A brief history of college football. Retrieved 2014, December 8 from http://www.collegefootballpoll.com/history_of_college_football.html
- Reynolds, R. (2015). Athletic success and contributions to universities’ athletic departments (M.S. Thesis). Texas A&M University, College Station, TX.
- Pincin, J., & Hoffer, A. (2013). NCAA athletic departments: An empirical investigation of the effects of revenue and conference changes.
- Rhoads, T. A., & Gerking, S. (2000). Educational contributions, academic quality and athletic success. Contemporary Economic Policy, 18, 248–258.10.1111/coep.2000.18.issue-2
- Sigelman, L., & Bookheimer, S. (1983). Is it whether you win or lose? Monetary contributions to big-time college athletic programs. Social Science Quarterly, 64, 347–359.
- Sigelman, L., & Carter, R. (1979). Win one for the giver? Alumni giving and big-time college sports. Social Science Quarterly, 60, 284–294.
- Sims, C. (1980). Macroeconomics and reality. Econometrica, 48, 1–48.10.2307/1912017
- StataCorp. (2011). Stata statistical software: Release 12. College Station, TX: StataCorp LP.
- Staurowsky, E. J., Parkhouse, B., & Sachs, M. (1996). Developing an instrument to measure athletic donor behavior and motivation. Journal of Sport Management, 10, 262–277.10.1123/jsm.10.3.262
- Stinson, J. L., & Howard, D. R. (2004). Scoreboards vs. mortarboards: major donor behavior and intercollegiate athletics. Sport Marketing Quarterly, 13, 129–140.
- Stinson, J. L., & Howard, D. R. (2007). Athletic success and private giving to athletic and academic programs at NCAA institutions. Journal of Sports Management, 21, 235–264.10.1123/jsm.21.2.235
- Stinson, J. L., & Howard, D. R. (2008). Winning does matter: Patterns in private giving to athletic and academic programs at NCAA division I-AA and I-AAA institutions. Sports Management Review, 11(1), 1–20.10.1016/S1441-3523(08)70101-3
- Tucker, I. B. (2004). A reexamination of the effect of big-time football and basketball success on graduation rates and alumni giving rates. Economics of Education Review, 23, 655–661.10.1016/j.econedurev.2004.03.001
- U.S. Bureau of Economic Analysis. (2014). Current-dollar and real GDP. Retrieved 2014, July 9 from http://www.bea.gov/national/index.htm#gdp
- Verner, M. E., Hecht, J. B., & Fansler, A. G. (1998). Validating an instrument to assess the motivation of athletic donors. Journal of Sport Management, 12, 123–137.10.1123/jsm.12.2.123
- Walker, A. G. (2015). Division I intercollegiate athletics success and the financial impact on universities. SAGE Open, 5, 2158244015611186.