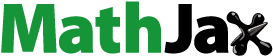
Abstract
In this study the technical efficiency of crop-producing households and the factors that influence it are explored, and comparisons are made using rural–urban migration as a comparative measure, in Gurage Zone, Ethiopia. The data were collected from 384 arbitrary picked rural households and analyzed using a stochastic frontier approach. Non-migrant households, migrant-sending households, and total samples had mean technical efficiency of 45.5%, 72.3%, and 57.4%, respectively, according to the findings. The household head’s age and distance from a neighboring town have a downbeat impact on technical inefficiency, whereas schooling, soil infertility, migratory experience, and distance to a nearby market have an upbeat upshot. With the diversified and specific solutions provided, the study is recommended the government body to focus on making the best use of available agricultural inputs, boosting soil fertility, and regulating the sources of technological inefficiency.
PUBLIC INTEREST STATEMENT
The global population has been steadily increasing over time. As a result, there is a significant gap between global food supply and demand, particularly in Sub-Saharan African countries. Several factors have been identified as contributing to this, the most important of which are the level of crop-producers’ technical efficiency in utilizing limited resources and the type of poverty-relief strategy chosen. Analytical data linking rural–urban migration with farm technical efficiency and the factors that influence it are critical for policymakers developing appropriate agricultural and labor migration policies. Despite the greater implications of these issues, studies are relatively rare in Ethiopia in general, and particularly in the Gurage zone. As a result, this study investigates crop-producing households’ technical efficiency and its determinants, and compares the results using rural–urban migration as a parameter in order to provide specific recommendations based on the analytical data.
1. Introduction
The smallholder farmers make for 96% of the agriculture sector in Ethiopia (Taffesse et al., Citation2012), and it is the most essential economic sector, accounting for 80% of jobs and 41% of GDP, respectively (Eyasu, Citation2018). Ethiopian crop output is among the top, with significant regional and agro-ecological diversity (Eyasu, Citation2018; Taffesse et al., Citation2012). According to the official statistics survey, crop production in the country increased by 8% between 2010 and 2016 (Eyasu, Citation2018). Albeit looking as if to boost crop production over time, market demand for crops is exceptionally strong, and the current food shortage cannot be alleviated. Several socioeconomic factors have been identified as driving factors for crop output scarcity, the most important of which is growers’ ability to use limited resources as efficiently as possible, which includes efficiency level and the factors that influence it (Anbes, Citation2020; Julie et al., Citation2017; Makombe et al., Citation2017; Yimenu, Citation2017). Furthermore, the importance of these factors varies depending on the farmers’ strategic choices; consequently, various researchers have conducted comparative strategy analyses in a variety of ways and provided specific recommendations to policymakers. For example, selecting one crop over another (Binam et al., Citation2005), specialization versus diversification (Julie et al., Citation2017), rain-fed versus irrigation (Gebrehaweria et al., Citation2012), and a comparison of the outcomes of households with and without migration (Adaku, Citation2013; Black, Citation1993).
Migration, particularly from rural to urban areas, is a major issue in the study area. The majority of rural households in the study area prefer rural to urban migration as a means of escaping poverty, and they are eager to send migrants to other cities across the country on a regular basis (Feleke, Pankhurst, Bevan, & Lavers, Citation2006; Worku, Citation1995). As a result, in order to determine whether rural–urban migration is an enabler or a detriment to crop production improvement in the study area, this study compared crop output and technical efficiency levels of rural households with and without migrants.
The empirical research on the relationship between rural–urban migration and crop output has been based on two contradicting hypotheses. The first assumption is that rural–urban migration stimulates economic growth in the departing area through remittances and technological advancements gained by migrants (Adams & Cuecuecha, Citation2013; Black, Citation1993; Faist, Citation2008; Gray, Citation2009; Hull, Citation2007; McCarthy et al., Citation2006; Mendola, Citation2008; Redehegn et al., Citation2019; Simelane, Citation1995) consequently, migrant-sending households will be able to produce more crops. The other opposing hypothesis holds that rural-migration causes labor shortages, fallow farmland, and deterioration of traditional farm technologies in the source (García-Barrio & García-Barrios, Citation1990; Gunjan & Chinnappa, R, Citation2015; Qin, Citation2010; Schmook & Radel, Citation2008), all of which have negative consequences for crop output, productivity, and efficiency. By and large, these studies demonstrated that rural-to-urban labor movement had a complex and variable impact on crop output, efficiency, and homestead technology use.
Several studies conducted in Ethiopia (Anbes, Citation2020; Dessale, Citation2019; Solomon, Citation2014; Tsion et al., Citation2020; Yimenu, Citation2017; Yimer, Citation2017) estimated technical efficiency and identified its determinants on the explicit kind of yields and the complete factor productivity of harvests in general (Fantu, Citation2012; Makombe et al., Citation2017). Despite this, few studies have been conducted to compare the efficacy of one crop or approach to another (Gebrehaweria et al., Citation2012). To the best of the researchers’ knowledge, no comparative study has been undertaken in the study area employing rural–urban migration as a comparison measure for investigating crop-producing households’ technical efficiency and its drivers. This comparative study produced analytical data by answering two major research questions: (1) how well are crop-producing households in the study area utilizing limited resources to maximize output while using rural–urban migration as a livelihood strategy? (2) What are the root causes of crop-producers’ technical inefficiency? As a result, the study is useful for agricultural policymakers in general, and rural households in particular, in selecting appropriate strategies and focusing on maximizing limited resources, because it provides analytical data on whether rural–urban migration is an enabler or an impediment to crop-producing households’ technical efficiency in the study area. Furthermore, the analytical data presented can assist any stakeholders interested in linking rural–urban migration and crop-producers’ technical efficiency, and the study can serve as a springboard for future researches. The introduction, a summary of the examination technique, a discussion of the findings, and a conclusion with recommendations make up the four sections of this article.
2. Methodology
2.1. Description of the study area
The examination was completed to look at the productivity, technical efficiency, reason for technical inefficiency, and the aftereffect of rustic urban migration on the technical efficiency of crop producers in the Gurage zone of the Southern National Regional state in Ethiopia. The geological character of the Gurage zone is exhibited into three classifications. These are the sloping high land (demonstrated by the Gurage mountain succession and isolating the zone east to west by a height of 3600 m), the plateau flat lands and the small broadening region (the western limit of the break valley and the “Wabe-Gibe” gulch enveloping a rise of 1000 meters)
(Wondwossen et al., Citation2018). The zone’s agro-ecology is divided into three categories: “Dega” or highland, “Woina-Dega” or midland, and “Kola” or lowland. The majority of the zone is covered by the mid-highland climate, accounting for 65.3% of the total area, while the highland and lowland agro-ecological zones cover 31.6% and 3.1% of the total area, respectively (Ibid). As reported by CSA (Citation2007), the whole populace of the zone is 1,280,483 with a normal individual of 4.5 per household. From the detailed whole populace, 48.6% and 51.4% are male and female partners correspondingly with a creation of 57.55% and 42.45% provincial and metropolitan occupants, respectively . Agriculture, particularly mixed farming system that incorporates crop production and livestock husbandry, is the principal source of income for rural households in the study area. It employs nearly as many people in diverse regions of the zone as other enterprises combined. Agriculture’s contribution to household income and employment, on the other hand, varies and is declining, especially in the zone’s midlands, which account for 65.3% land area. Accordingly, many rural families are considering different choices for coping with poverty, including migration. The internal labor migration has long been a feature of the area in search of business prospects in various city corridors, and it has made a significant contribution at both the household and community levels (Feleke et al., Citation2006; Worku, Citation1995).
2.2. Sample and sampling technique
To fulfill the stated objectives, multistage sampling and probability proportional to sample size procedures were used to randomly choose 384 rural households. The Cochran (Citation1963) equation was used to determine the sample volume. Let’s say there are several rural households, but we don’t recognize the full extent of those who see rural–urban migration as a way out of poverty.
Therefore, we used a 0.5 proportion value for p and assigned a 95% certainty level, 5% precision, and 1.96 for the Z-value obtained in computable tables. The following sample size is confirmed:
Where n represents sample rural households, Z is the abscissa of the typical curve that overtakes an area α at the appendages (1—equals the 95% certainty level), p is the level of capriciousness in the population, q denotes 1-p, and e represents the ideal degree of exactness.
2.3. Source of data and study variables
This study uses crop output and production input data assembled from ranch households in three agro-ecological zones of Gurage zone, Ethiopia. The necessary information were gathered from 384 haphazardly chose ranch households in the scholastic year 2018/2019. The dependent variable of the research was the output of all crops (Qi) produced in sample households’ farms. To realize consistency in (Bozoglu & Ceyhan, Citation2007; Julie et al., Citation2017; Tsobo et al., Citation2012), the value method was utilized to aggregate the total production into output value by multiplying the gross product by the output price (in Ethiopian Birr value). The gross production was calculated as sales + consumption gift + losses and the yield price information was gotten from review households and it was affirmed by the neighborhood market officials in the examination area. The input variables consist of four categories such as land in acres, labor in working hours, fertilizer in quintal, and chemical (pesticides/ herbicides) used in liters. The input variables were customized as an actual amount form for stochastic outskirts assessment and afterward the technical efficiency and productivity of harvests were dissected from the estimated outskirts.
2.4. Specification of the stochastic frontiers model
In literature frontiers are estimated employing various methods of which stochastic and data envelopment inquiry frontiers are repeatedly used methods which involve parametric and non- parametric (deterministic) approaches respectively (Coelli, Citation1996a; Coelli et al., Citation1998). Stochastic frontier is a typical approach to parametric frontier estimation and this study handled it by following Cobb Douglas production outskirts and assuming half normal allocation. The stochastic outskirts model for cross sectional data encompasses two error terms (and
);
explicates arbitrary effects and
justifies the technical efficiency failure (Charnes et al., Citation1978; Coelli et al., Citation1998; Farrell, Citation1957). The value of
is implicitly two-sided self-regulating (
) and identically dispersed (iid) casual error containing a normal distribution with nil mean and variance (Coelli, Citation1996a). Moreover, on account of several yields, Mastromarco (Citation2008) commend to aggregate the outputs into a solitary output index. Typically, from the predicted stochastic production outskirts the technical efficiency of the ranch units is estimated. Thus, the general version the stochastic production outskirts model is specified as:
(1)
Where is the magnitude of output for the ith household (in the logarithm form);
is a vector of unidentified components to be determined;
is a Kx1 vector of input amounts exploited by the ith rustic household to produce
;
is a casual variable which explains random effect (stochastic error term that include factors apart from household administration) and
is a technical inefficiency effect that causes the farmer to operate below the stochastic frontier (factors that can be controlled by the household).
The technical efficiency of crop-producing households is predicted following Battese (Citation1992) which is a proportion of the actual output to the highest potential output. Hence, the assessment procedure can be formulated as:
. The estimated value normally lies somewhere in the range of 0 and 1
and with
based on Battese and Coelli (Citation1995) computed as;
Where ‘ is the intercept,
is unknown parameters to be estimated,)
is a vector of independent variables that affect the technical efficiency (inefficiency) of crop producing households,
are unnoticeable casual variables supposed to be identically distributed with nil mean and unidentified variance, to such an extent that the value of
is non-negative number (
). Usually, the functional form of
is takes half-normal, truncated normal, exponential, or a gamma distribution. Nevertheless, this survey utilized the truncated typical distribution function as it accounts the share of crop producing households working at full efficiency (Assefa et al., Citation2020; Mkhabela, Citation2005; Ogundari, Citation2013). The stochastic outskirts model of this exploration is empirically specified as:
Where and
are parameters to be predicted,
is the amount of the entire crops produced in each households’ farm and converted into Ethiopian birr value,
= land holding size in acres,
= quantity of labor in working hour,
= quantity of fertilizer use in quintal,
= amount of chemical use (pesticide, herbicides, fungicide) in liter, and
and
accounts for random effects and technical inefficiency, respectively. Alternatively, the empirical model employed to itemize the technical inefficiency’s triggers is alluded to as:
Where,
and
are hidden random variables and z1 is age of the head, z2 is agro-ecological zone, z3is soil infertility problem, z4 is household migration status, z5 is education year for the head of the household, z6 is town proximity in kilometers and z7 is adjacent market place closeness in kilometer.
3. Results and discussion
3.1. Description of respondents according to the variables of the research
shows a comparison of respondents based on aggregate crop output and the number of inputs used in production. The study found that migrant-sending households generated 59.4% less output on average than non-migrant households, while spending just 27.97% less on inputs. Since the average land size for crop production is 1.29 acres, the surveyed households were mainly smallholders. Non-migrant households had an average land size of 1.696 acres, which was slightly larger than migrant-sending households (0.97 acres), suggesting that land availability is a determinant factor in household livelihood strategy selection. Migrant-sending households have lesser land productivity, which is 29 percentage points lower than non-migrant households’ land productivity. The study’s results also revealed that the average amount of labor required for crop production is 831.4 human working hours. The mean labor for migrant-sending and non-migrant households was 855.85 and 800.85 human working hours, respectively, indicating that the amount of labor used for crop production is determined by household member migration. Land planning, planting, weeding, harvesting, and marketing activities all include labor. In addition, the labor productivity of migrant-sending households is 62% lower than that of non-migrant households. It’s possible that this is due to the use of old labor, or that the hired labor isn’t working hard enough. The intermediate inputs such as fertilizer and chemical applied on crop farms are irrespective of the migration status of rural-households. Lastly, the profitability ratio of the sample households was computed by dividing the output price by the total input cost. The study result revealed the profitability ratio of migrant-sending households was 48.73% smaller than non-migrant households. On account of results attained it would be true to conclude that crop production is reliant on the quantity of inputs (land, labor, capital) used.
Table 1. The narrative of respondents as a function of crop output and production inputs
3.2. Stochastic production outskirts assessment
The results linked to the assessment of the stochastic frontier analysis are accessible in . The parameters were predicted using a one-step stochastic approach based on a Cobb Douglas production function with a truncated ordinary distribution for the (in) efficiency term and they are most likely hypothetical forecasts. The sfcross command was employed for regress the log output on the log of the physical quantities inputs (Battese & Coelli, Citation1995; Iheke et al., Citation2013; Julie et al., Citation2017). The level of technical efficiency and the determinants of the inefficiency term were estimated based on the estimated stochastic production frontiers. According to the model output, land and fertilizer are major production frontiers, whereas the significant determinants of crop-producing households’ technical inefficiency are the household head’s age, education status, soil infertility problem, migration participation, distance to the nearby town, and distance to the nearby market. The goodness of the fit of the model was realized as the estimated lambda (λ = 0.479) is different from zero at the 1% significance level. The predicted Sigma_u () value of 0.254 showed that 25.4 % of the entire disparity of crop output is caused by technical inefficiency. The significant variables for the production frontiers and for the technical inefficiency terms were discussed in sections 3.2.1 and 3.2.2 below, respectively.
Table 2. Estimation of the stochastic production frontier and basis of technical inefficiency
3.2.1. The technical efficiency of respondents
Four inputs, including landholding size in hectares, human labor in working hours, fertilizer used in quintals, and chemical used in liters, have been incorporated into the model to find things simpler to predict the coefficients of the production frontiers. All of the inputs have positive coefficients, indicating that they are elastic, according to the model’s prediction. The amount of farmland owned and use of fertilizer are two of the four inputs in the model that have a significant impact on crop-producing farmers’ production frontiers at the 1% significance level. Holding all other variables constant, a 10% increase in land owned size in acres as well as fertilizer used in quintal would increase farmers’ crop output by 5.02 and 0.54 units, respectively, in the study area, according to the frontier model results. This implied that the greater the amount of landholding size and fertilizer utilization, the greater the amount of crop output, and thus the higher the elasticity.
The outcomes of the highest probability assessment reveal that surveyed households in the study area show a substantial amount of technical inefficiency. The modal efficiency score value is found within the range of 0.41–0.50 which accounts for 42.97% of the pooled sample households and followed by 0.71–0.80 which consists of 21.61%. However, only 7.29% of the sampled households have efficiency scores greater than 0.80. The intended technical efficiency of the shared sample was found between 0.295 and 0.894 with a mean of 0.574. The mean predicted TE of 57.4% suggests that there is an opportunity to boost crop yield in the examination area by 42.6% with similar inputs use or there is likelihood to lessen the inputs use by 42.6% to attain the same output. The value can also be interpreted as, on average, 42.6% of the crop yield in the research area is lost because of technical inefficiency. The average households in the joint sample could attain the TE of the most efficient frontier (0.894) either by reducing the cost or increasing the output by 35.79% (1-(0.574/0.894). The predicted TE scores of migrant-sanding households range from 0.295 to 0.728 with a mean of 0.455, whereas for non-migrant households ranged from 0.371 to 0.894 with a mean of 0.723. This suggests that non-migrant households are technically more efficient than migrant-sending households. Generally, the migrant-sending and non-migrant households are supposed to reduce the cost or increase the crop output by 49.1% and 19.13% respectively to realize their most efficient counterparts.
The results of a two-sample independent t-test revealed in also indicated that there is a significant difference in the mean TE of migrant-sending households and households without migrants. The important mean difference in TE score affirmed that TE is connected with household migration status and migration is found to have a household technical efficiency shrinking effect in the research area. This finding verified the results of (Defidelwina et al., Citation2019; Iheke et al., Citation2013). Conversely, the study result is contrasted with the findings of (Liu et al., Citation2019; Mochebelele, Citation2000; Nonthakot & Villano, Citation2008; Yang et al., Citation2016) who reported that migration affects positively the technical efficiency of crop production.
Table 3. Technical efficiency of the respondents from production frontier
Table 4. Two-sample t-test with equal variances on TE
3.2.2. Determinants of the technical inefficiency
So as to make appropriate decisions on the utilization of limited resources scientific recommendations are very essential for policy makers. For this situation, the inefficiency impacts model was utilized to characterize the basis of technical inefficiency following the one-step approach following the one-step approach. Among the 7 explanatory factors fused in the inefficiency effects model (see ) 6 factors were essentially affected the technical efficiency of crop-producing households. The predicted parameters () showed that the household head’s age, education status, soil infertility problem, migration participation, distance to the close town and distance to the close market are the key factors that significantly influence the technical inefficiency of crop producing households in the study area. The age of the household heads and distance to the nearby town are inversely associated with the level of technical inefficiency and thus have a positive contribution on the extent of the technical efficiency of crop producing households in the study area. While, education status, soil infertility problem, migration participation and distance to the nearby market are reduced the level of technical efficiency as they are directly associated with the technical inefficiency. The connotations of the significant factors of farm households’ technical efficiency in the research area were contended beneath.
The age of the household head has been used to substitute experience in this study, and it was found to be related to technical inefficiency in a roundabout way at the 1% level of significance. The inefficiency effects model predicted that one additional year expansion in the age of the household head would diminish the probability of technical inefficiency by 0.59%, holding other things constant. This implies that technical efficiency increases with increased age. This hold ups the idea that farmers with higher age are more probable to build up farming experience which enables them to understand farm challenges and utilizes production inputs in a healthier way. These results are steady with the detections of (Dessale, Citation2019; Getachew & Bamlak, Citation2014; Julie et al., Citation2017; Mkhabela, Citation2005; Ogundari, Citation2013; Tadie et al., Citation2019; Wudineh & Endrias, Citation2015). The findings of (Gul et al., Citation2009; Liu et al., Citation2019; Tolga et al., Citation2009) however, reported the converse.
The coefficients of the education status of the household heads confirmed a positive and significant association with technical inefficiency, which revealed that with increased education rural-households ‘ technical inefficiency level increased. A reasonable justification is that more educated farmers are less probable to engage in agriculture but active in searching non-farm incomes. This result hints that those rural-households who are actively involved in only crop cultivation as permanent workers are more efficient than counterparts, since they dedicated more time to crop cultivation. The study result is in harmony with the findings of Mkhabela (Citation2005). The study results of (Beyan et al., Citation2013; Getachew & Bamlak, Citation2014; Gul et al., Citation2009; Igbekele et al., Citation2006; Tadie et al., Citation2019; Wudineh & Endrias, Citation2015) were reported in the different line.
The migration experience of rustic households showed expected signs and found to have an inefficiency increasing effect at a 1% degree of significance. This suggests that, in the study area migrant-sending crop producing farm households are technically less efficient than households without migrants. A possible justification for this might be because of improper utilization of the available labour and remittance. In the research area, many of the migrant-sending households are remittance dependent and withdraw themselves from monotonous activities related to crop cultivation & wrongly use their recreation time by doing superfluous things like drinking alcohol (Feleke et al., Citation2006; Worku, Citation1995). Thus, wrong utilization leisure time, an acute remittance dependency and sluggishness are more likely to increase farm technical inefficiency. Similar results were met by (Liu et al., Citation2019; Sauer et al., Citation2015) who reported a positive significant effect of migration on technical inefficiency. During the FGD session in the study area, analogous connotations were obtained and stated as follows: “Every family in our hamlet wishes to send their children to town. As a result, it’s difficult to find labor for crop cultivation, and we’re forced to pay more for hired labor, no matter how bad it is. Finally, we are forced to harvest low.” said a female FGD participant in Ezha Woreda’s Shebraden Kebele.
Results of this study also exposed a positive connection between soil infertility and technical inefficiency, at a 5% degree of significance. This positive association implies that as the soil infertility problem enhances the technical efficiency of crop producing households would be declined because of the increased inefficiency. An allusion to this is that households with soil infertility problems are technically more inefficient than their partners who haven’t faced soil infertility problems. This can be accredited to the way that the technical inefficiency of crop producing households in the investigation region is probably going to expand, as the soil infertility problem enhances. This finding verified the outcome of (Al-Amin et al., Citation2016; Assefa et al., Citation2020; Raimi et al., Citation2017). Related results were also confirmed by (Rapsomanikis, Citation2015) who reported a direct significant effect of soil quality on productivity across nine countries.
The coefficient of distance to the nearby town is negatively interconnected with farm technical inefficiency at a 5% level of significance. The investigation result affirmed that an expansion somewhere far off to the close by town by one km leads to decline the technical inefficiency of crop producing households by 1.98%, holding other factors steady. This hints that crop producing farm households who are located far-away from the close towns are more technically efficient and optimize their farm activity more than their partners who are situated in nearby towns. A potential justification this may be the way that the far distance between the households’ home and the town would increase transport and opportunity costs. This is an opportunity for successful allocation of working population; reduce unnecessary town vacation and minimization of occasional costs which are the basis for attaining superior technical efficiency. This investigation is similar with the study results of (Wudineh & Endrias, Citation2015) who stated that wheat producing farmers who are living in the nearby town and engaged in off-farm & non-farm works have realized a reduced amount of technical efficiency in Ethiopia. However, (Bhatt & Bhat, Citation2014) in Bangladesh reported contrary results to this study.
This study also revealed that distance to the nearby market had an indirect substantial relationship with technical efficiency. The inefficiency impact model predicted that one kilometer increase in market distance decreases the technical efficiency of crop-producing households by 1.9%, custody other variables constant. A possible explanation for this could be the availability of non-farm and off-farm jobs in the nearby market, causing rural households to spend less time on their farm and thus harvest with lower technical efficiency. Another possible clarification for this might be linked with higher costs as the occasion costs of production and marketing are increased with the increased market distance then the technical efficiency of crop-producing households is probably less. Moreover, the accessibility and choice of production inputs are determined by market access which reduces farmers’ susceptibility to shocks and widens economic opportunities. Subsequently, households are bound to be adversely affected by the expanded market distance. A comparable finding was reported by (Agerie et al., Citation2019) investigating the effect of market distance on the technical efficiencies’ of potato producing farmers in Ethiopia. The findings of (Getachew & Bamlak, Citation2014; Hagos, Citation2014; Rapsomanikis, Citation2015; Tamirat et al., Citation2020) were also reported in the same line as the finding of the study.
4. Conclusions and the way forwards
This study looks at crop-producing households’ technical efficiency and its determinants, and the results are compared based on the migration status of rural households. According to this analysis, the technical efficiency of crop-producing households varies greatly depending on the household’s migration status, with migrant-sending households having a lower value. Crop-producing migrant-sending households, non-migrant households, and shared samples had 45.5%, 72.3%, and 57.4% technical efficiency, respectively. So, the consequence of rural–urban migration on technical efficiency is dismal, as migrant-sending households are less efficient in maximizing the limited resources available to produce the best output. The study also found that the age of the household head and the distance to the nearest town have an adverse influence on the technical inefficiency of crop-producing households in the study area. While the technical inefficiency of crop-producing households is being exacerbated by the household head’s education, soil infertility problems, household migration status, and distance to the nearest market place. Therefore, the study recommended the government bodies to develop appropriate farm plans based on the level of technical efficiency, work on the efficient use of limited resources, and regulate the drivers of technical inefficiency. This necessitates policies that address how to compensate for labor losses due to migration, and how to best use the scarce and idle resources to increase crop yield in the study area. To that end, the relevant body must encourage greater use of agro-chemicals, improved seeds, and automation technologies, particularly in cropland of migrant-sending households, in order to ensure short- and long-term crop production efficiency. The actual action of the appropriate body is also relevant in the areas of land resource management and agricultural extension service provision. This could include promoting integrated soil fertility management practices to improve soil quality, launching cluster or contract farming to fill vacant cropland, strengthening monitoring and evaluation systems to persuade farmers to use full extension services, and working to improve major commodity input supply and distribution networks by addressing infrastructure issues.
Disclosure statement
No potential conflict of interest was reported by the author(s).
Additional information
Funding
Notes on contributors
Mesfin Agza
Mesfin Agza is a PhD student in the Center for Rural Development at Addis Ababa University, Addis Ababa, Ethiopia. He is also a lecturer in the department of Agricultural Economics at Wolkite University and a corresponding author of this study. ORCID ID: https://orcid.org/0000-0002-9681-8717; email address: [email protected]
Bamlaku Alamirew
Bamlaku Alamirew is an Associate Professor at Yom postgraduate college and a Certified International Project Manager (CIPM) in Ethiopia. Email address: [email protected]
Admasu Shibru
Admasu Shibru is an Associate Professor at the Center for Food Security Studies, Addis Ababa University, Ethiopia. Email address: [email protected]
References
- Adaku, A. A. (2013). The effect of rural-urban migration on agricultural production in the northern region of Ghana. Journal of Agricultural Science and Applications, 2(4), 193–14. https://doi.org/10.14511/jasa.2013.020402
- Adams, R. H., & Cuecuecha, A. (2013). The impact of remittances on investment and poverty in Ghana:. World Development, Elsevier, 50(C), 24–40. https://doi.org/10.1016/j.worlddev.2013.04.009
- Agerie, N. W., Tigabu, D. K., & Abebe, D. K. (2019). Analysis of technical efciency of potato (Solanum tuberosum L.) production in Chilga District, Amhara National Regional State, Ethiopia. Journal of Economic Structures, 8(34), 1–18. https://doi.org/10.1186/s40008-019-0166-y
- Al-Amin, A. K., Rahman, M. S., Hossain, M. I., & Sayem, S. M. (2016). Impact of land fragmentation and soil fertility on rice producers’ technical efficiency: An empirical study in Bangladesh. Asia-Paciöc Journal of Rural Development, XXVI(2), 1–20.
- Anbes, T. (2020). Technical Efficiency of Smallholder Agriculture in Developing Countries: The Case of Ethiopia. Economies, 8(34), 1–27. https://doi.org/10.3390/economies8020034
- Assefa, A., Meded, A., Alemayehu, O., Mulubrhan, B., Ibrahim, A., & Abduselam, F. (2020). Determinants of productivity and efficiency of teff production in southwest Ethiopia. Research Square, 1–26.
- Battese, G. E. (1992). Frontier production functions and technical efficiency: A survey of empirical applications in agricultural economics. Agricultural Economics, 7(3–4), 185–208. https://doi.org/10.1016/0169-5150(92)90049-5
- Battese, G. E., & Coelli, T. (1995). A model of technical inefficiency effects on stochastic frontier production for panel data. Empirical Economics, 20(2), 325–345. https://doi.org/10.1007/BF01205442
- Beyan, A., Jema, H., & Endrias, G. (2013). Analysis of farm households’ technical efficiency in productionof smallholder farmers: The case of Girawa District, Ethiopia. American-Eurasian Journal of Agriculture & Environ. Science, 13(12), 1615–1621.
- Bhatt, M. S., & Bhat, S. A. (2014). Technical efficiency and farm size productivity- micro level evidence from Jammu & Kashmir. International Journal of Food and Agricultural Economics, 2(4), 27–49. https://doi.org/10.22004/AG.ECON.190809
- Binam, J. N., Tonye, J., & Wandji, N. (2005). Sources of technical efficiency among smallholder maize and peanut farmers in the slash and burn agriculture zone in Cameroon. Journal of Economic Cooperation, 26(1), 193–210.
- Black, R. (1993). Migration, return, and agricultural development in the Serra Do Alvao, Northern Portugal. Economic Development and Cultural Change, 41(3), 563–585. https://doi.org/10.1086/452032
- Bozoglu, M., & Ceyhan, V. (2007). Technical Efficiency in a Vegetable (2007). Measuring the Technical Efficiency and Exploring the Inefficiency Determinants ofVegetable Farms in Samsun Province, Turkey. Agricultural Systems; No, 94(3), 649–659. https://doi.org/10.1016/j.agsy.2007.01.007
- Charnes, A., William., W., . C., & El, R. (1978). Measuring the efficiency of decision making units. European Journal of Operation Research, 2(6), 429–444. https://doi.org/10.1016/0377-2217(78)90138-8
- Cochran, W. G. (1963). Sampling Techniques (2nd Ed. ed.). John Wiley and Sons, Inc.
- Coelli, T. (1996a). A guide to data envelopment analysis (Computer Program) version 2.1. CEPA working papers No. 8/96. Armidale NSW 2351, Department of Econometrics. University of New England.
- Coelli, T., Rao, D. S., & Battese, G. E. (1998). An introduction to efficiency and productivity analysis. Kluwer Academic Publishers.
- CSA. (2007). Summary and statictical report of population and Housing Census. United Nations Population Fund (UNFPA).
- Defidelwina, J., Waluyati, L. R., & Widodo, S. (2019). The impact of migration on rice farming technical efficiency in Indonesia. International Journal of Mechanical Engineering and Technology, 10(3), 1703–1712. http://www.iaeme.com/ijmet/issues.asp?JType=IJMET&VType=10&IType=3
- Dessale, M. (2019). Analysis of technical efficiency of small holder wheat-growing farmers of Jamma district, Ethiopia. Agriculture & Food Security, 8(1), 1–8. https://doi.org/10.1186/s40066-018-0250-9
- Eyasu, A. (2018). The crop sector in Ethiopia: Current and future prospects. In 2nd International Agro- Industry Investment Forum (pp. 1–31). MoANR.
- Faist, T. (2008). Migrants as transnational development agents: An inquiry into the newest round of the migration–development Nexus. Population, Space and Place, 14(1), 21–42. https://doi.org/10.1002/psp.471
- Fantu, N. B. (2012).Growth in Total Factor Productivity in the Ethiopian Agriculture Sector: Growth Accounting and Econometric Assessments of Sources of Growth: Ethiopia Strategy Support Program II (ESSP II) ESSP II Working Paper 37. Addis Ababa: International Food Policy Research Institute and Ethiopian Development Research Institute.
- Farrell, M. The Measurement of Productive Efficiency. (1957). Journal of the Royal Statistical Society. Series A (General), 120(3), 253–281. Part 3. https://doi.org/10.2307/2343100
- Feleke, T., Pankhurst, A., Bevan, P., & Lavers, T. (2006). Migration and rural-urban linkages in Ethiopia and implications for policy and development (pp. 1–97). University of Bath: Research Group on Wellbeing in Developing Countries, Ethiopia Programme.
- García-Barrio, R., & García-Barrios, L. (1990). Environmental and technological degradation in peasant agriculture: A consequence of development in Mexico. World Development, 18(11), 1569–1585. https://doi.org/10.1016/0305-750X(90)90044-X
- Gebrehaweria, G., Namara, R. E., & Holden, S. (2012). Technical efficiency of irrigated and rain-fed smallholder agriculture in Tigray, Ethiopia: Acomparative stochastic frontier production function analysis. Quarterly Journal of International Agriculture, 51(3), 203–226.
- Getachew, M. K., & Bamlak, A. A. (2014). Technical efficiency of farming systems across agro-ecological zones in Ethiopia: An application of stochastic frontier analysis. Science, Technology and Arts Research Journal , 3(3), 204–2012.
- Gray, C. L. (2009). Rural out-migration and smallholder agriculture in the Southern Ecuadorian Andes. Population and Environment, 30(4–5), 193–217. https://doi.org/10.1007/s11111-009-0081-5
- Gul, M., Koc, B., Dagistan, E., Akpinar, M. G., & Parlakay, O. (2009). Determination of technical efficiency in cotton growing farms in Turkey: A case study of Cukurova region. African Journal of Agricultural Research, 4(10), 944–949. http://www.academicjournals.org/AJAR
- Gunjan, B., & Chinnappa, R, B. V. (2015). Impact of Out-Migration on Agriculture and Women Work Load: An Economic Analysis of Hilly Regions of Uttarakhand, India. Indian Journal of Agricultural Economics, 70(3), 396–404. https://doi.org/10.22004/ag.econ.230218
- Hagos, W. (2014). The determinants of technical efficiency of farmers in Teff, Maize and Sorghum production: Empirical evidence from central zone of Tigray region. Ethiopian Journal of Economics, XXIII(2), 1–36.
- Hull, J. (2007). Migration, remittances, and monetization of farm labour in subsistence sending areas. Asian & Pacific Migration Journal, 16(4), 451–484. https://doi.org/10.1177/011719680701600402
- Igbekele, A. A., Adebiyi, G. D., & Abiodun, O. F. (2006). Technical efficiency of small scale farmers: An application of the stochastic frontier production function to rural and urban farmers in Ondo State, Nigeria. International Economic Journal, 20(1), 87–107. https://doi.org/10.1080/10168730500515498
- Iheke, O., Nwaru, J., & Onyenweaku, C. E. (2013). The impact of migrant remittances on the technical efficiency of arable crop farm households in South Eastern Nigeria. In 4th international conference of the African Association of Agricultural Economics (pp. 1–15). Hammamet, Tunisia: ICAAAE-africa.org.
- Julie, N. T., Engwali, F. D., & Claude, B. J. (2017). Technical Efficiency of Diversification Versus Specialization of Vegetable-Based Farms in the WestRegion of Cameroon. American Journal of Agriculture and Forestry, 5(4), 112–120. https://doi.org/10.11648/j.ajaf.20170504.15
- Liu, J., Zhang, C., Hu, R., Zhu, X., & Cai, J. (2019). Aging of Agricultural Labor Force and Technical Efficiency in Tea Production: Evidence from Meitan County ( 1–16). Sustainability.
- Makombe, G., Namara, R. E., Awulachew, S. B., Hagos, F., Ayana, M., & Kanjere, M. (2017). An analysis of the productivity and technical efficiency of smallholder irrigation in Ethiopia. Water SA, 43(1), 48–57. https://doi.org/10.4314/wsa.v43i1.08
- Mastromarco, C. (2008). Stochastic Frontier Models. Cambridge University Press.
- McCarthy, N., Carletto, G., Davis, B., & Maltsoglou, I. (2006). Assessing the impact of massive outmigration on agriculture: Agricultural and development economics division, ESA Working Paper No. 06–14. Washington, DC: The Food and Agriculture Organization of the United Nations.
- Mendola, M. (2008). Migration and Technological Change in Rural Households: Complements or substitutes? Journal of Development Economics, 85(1–2), 150–175. https://doi.org/10.1016/j.jdeveco.2006.07.003
- Mkhabela, T. (2005). Technical Efficiency in a Vegetable Based Mixed-Cropping Sectorin Tugela Ferry, Msinga District, Kwazulu-Natal. Agrekon, 44(2), 187–204. https://doi.org/10.1080/03031853.2005.9523709
- Mochebelele, M. W.-N. (2000). Migrant labour and farm technical efficiencyin Lesotho. World Development, 28(1), 143–153. https://doi.org/10.1016/S0305-750X(99)00116-3
- Nonthakot, P., & Villano, R. (2008). Migration and farm efficiency: Evidence from Northern Thailand. In AARES 52nd annual conference (pp. 1–18). Armidale, NSW, 2351: University of New England, School of Business, Economics and Public Policy.
- Ogundari, K. (2013). Crop Diversification and Technical Efficiency in Food Crop Production: A Study of Peasant Farmers in Nigeria. International Journal of Social Economics, 40(3), 84–91. https://doi.org/10.1108/03068291311291536
- Qin, H. (2010). Rural-to-Urban Labor Migration, Household Livelihoods, and the Rural Environment in Chongqing Municipality, Southwest China. Human Ecology, 38(5), 675–690. https://doi.org/10.1007/s10745-010-9353-z
- Raimi, A., Adeleke, R., & Roopnarai, A. (2017). Soil fertility challenges and Biofertiliser as a viable alternative for increasing smallholder farmer crop productivity in sub-Saharan Africa. Cogent Food & Agriculture, 3(1), 1–26. https://doi.org/10.1080/23311932.2017.1400933
- Rapsomanikis, G. (2015). The economic lives of smallholder farmers: An analysis based on household data from nine countries. Food and Agriculture Organization of the United Nations.
- Redehegn, M. A., Sun, D., Eshete, A. M., & Gichuk, C. N. (2019). Development impacts of migration and remittances on migrant-sending communities: Evidence from Ethiopia. PLOS ONE, 14(2), 1–20. https://doi.org/10.1371/journal.pone.0210034
- Sauer, J., Gorton, M., & Davidova, S. (2015). Migration and farm technical efficiency: Evidence from Kosovo. The Journal of the International Association of Agricultural Economics, 46(5), 629–641. https://doi.org/10.1111/agec.12159
- Schmook, B., & Radel, C. (2008). International Labor Migration from a Tropical Development Frontier: Globalizing Households and an Incipient Forest Transition. Human Ecology, 36(6), 891–908. https://doi.org/10.1007/s10745-008-9207-0
- Simelane, H. S. (1995). Labour migration and rural transformation in post-colonial Swaziland. Journal of Contemporary African Studies, 13(2), 207–226. https://doi.org/10.1080/02589009508729573
- Solomon, B. W. (2014, January). Efficiency of agriculturalproduction: Technicalefficiency ofmajor cropsin Ethiopia, stochasticfrontiermodel [Unpublished Master thesis for the Master of Philosophy in Environmental and Development Economics]. Department of Economics, Universitetet I Oslo.
- Tadie, M. A., Abebe, B. D., & Taye, M. M. (2019). Technical efficiency of smallholder farmers in red pepper production in North Gondar zone Amhara regional state, Ethiopia. Journal of Economic Structures, 8(18), 1–18. https://dx.doi.org/10.1186/s40008-019-0150-6
- Taffesse, A. S., Dorosh, P., & Asrat, S. (2012).Crop production in Ethiopia: Regional patterns and trends. Ethiopia Strategy Support Program II ESSP II Working Paper No. 0016. Addis Ababa, Ethiopia: International Food Policy Research Institute and Ethiopian Development Research Institute. http://ebrary.ifpri.org/cdm/ref/collection/p15738coll2/id/127350
- Tamirat, B., Wondaferahu, M., & Tesfaye, M. (2020). Technical efficiency and impact of improved farm inputs adoption on the yield of haricot bean producer in Hadiya zone, SNNP region, Ethiopia. Cogent Economics & Finance, 8(1), 1–21. https://doi.org/10.1080/23322039.2020.1833503
- Tolga, T., Nural, Y., Mehmet, N., & Bahattin, Ç. (2009). Measuring the technical efficiency and determinants of efficiency of rice (Oryza sativa) farms in Marmara region, Turkey. New Zealand Journal of Crop and Horticultural Science, 37(2), 121–129. https://doi.org/10.1080/01140670909510257
- Tsion, Y., Anbes, T., & Zerhun, G. (2020). Technical efficiency of agricultural production in Ethiopia. Journal of Natural Sciences Research, 10(5), 46–54. http://dx.doi.org/10.7176/JNSR/10-5-05
- Tsobo, B. A., Omotesho, O. A., Salau, S. A., & Adewumi, M. (2012). Determinants of technical, allocative and economic efficiency among dry season vegetable farmers inSokoto state, Nigeria. Journal of Agricultural Science, 3(2), 113–119. https://doi.org/10.1080/09766898.2012.11884692
- Wondwossen, G., Zewde, W., Tesfaye, H., & Habtamnesh, A. (2018). Characterization of agro forestry practices and their socioeconomic role in selected districts of Gurage ZoneEthiopia. International Journal of Research in Agriculture and Forestry, 5(11), 30–40. SRYAHWA.
- Worku, N. (1995). The Impact of Urban Migration in Village Life: The Gurage case. MA thesis in Social Anthropology. Addis Ababa University, Ethiopia: CODESTRIA- LIBRARY.
- Wudineh, G. T., & Endrias, G. (2015). Technical efficiency of smallholder wheat farmers: The case of Welmera district, Central Oromia, Ethiopia. Journal of Development and Agricultural Economics, 8(2), 39–51. https://doi.org/10.5897/JDAE2015.0660
- Yang, J., Wang, H., Jin, S., Chen, K., Riedinger, J., & Peng, C. (2016). Migration, local off-farm employment, and agricultural production efficiency: Evidence from China. Journal of Productivity Analysis, 45(3), 247–259. https://doi.org/10.1007/s11123-015-0464-9
- Yimenu, K. (2017, June). Determinants of Technical Efficiency of Maize Production of Smallholder Farmers; the Case of Fogera District, South Gondar Zone. Unpublished Master Thesis Submitted to the Department of Agricultural Economics. University of Gonder.
- Yimer, A. (2017, June). Analysis of technical efficiency of smallholder Teff production: The case of Legehida Woreda, South Wollo [Unpublished master thesis submited to the Department of Agricultural Economics]. University of Gonder.