Abstract
Social media networks are expanding rapidly, increasing the spread and scale of information diffusion. Researchers have highlighted distinguishing features of social media network platforms: network structure transparency, public self-monitored digital profiles, homogenized network connections, and node-created digital content. These features, while adding utility to social media platform providers and users, can also be exploited to manipulate users’ behavior and overall network outcomes. This paper posits the importance of network theory as critical foundational “laws” upon which the responsible innovation of social media can be built to minimize such manipulation, how such theory can be used to predict the potential impacts of new network innovations, and the resulting difficulty this framing suggests for self-governance on the part of platform providers. Through a case study analysis of Russian social media interference in the 2016 U.S. presidential election, the value of a network theoretic lens is highlighted. The concept of “supranodes”, social media nodes empowered via theoretical knowledge and network awareness to socially engineer network structures and outcomes, is developed and the network theoretic features they exploit discussed.
1. Introduction
Social media platforms leverage functionality enabled by Web 2.0; examples of such platforms include Facebook, Instagram, and Twitter (De Bakker & Hellsten, Citation2013; Kahn & Kellner, Citation2004). Market data notes the large number of monthly active users (MAUs) on such platforms: Facebook at 2.23 billion MAUs, Instagram at 1 billion MAUs and Twitter at 321 million MAUs (Lua, Citation2019; Noyes Citation2019). This pervasive adoption is facilitated by the ease of internet access and the free use of these platforms, which are frequently supported by advertising revenue business models, allowing the platforms to leverage their user networks for economic benefit.
In the parlance of network theory, social media users are viewed as nodes connected by ties that represent relationships or interactions. As do non-virtual relationships and interactions, social media ties vary in form. They may be cognitive (friend or colleague), affective (like or dislike) or event driven (sending or receiving communications; Borgatti & Halgin, Citation2011). These ties create paths that connect nodes in a network and form the overall network structure within which the various nodes have unique positions. A node’s position is what determines its access to information and resources, its social capital, and its ability to influence or its propensity to be influenced by others (Butler, Citation2001). Researchers have related these varying network structures and node positions to both network and individual outcomes (Borgatti & Halgin, Citation2011).
Social media networks have unique characteristics relative to their non-social media counterparts (Kane et al., Citation2014). Building on foundational research (Barton, Citation2009; Besiou et al., Citation2013; boyd & Ellison, Citation2007; Donath & Boyd, Citation2004; Gilbert & Karahalios, Citation2009), Kane et al. (Citation2014) propose four unique features. First, users’ digital profiles are publicly displayed and represent an integration of information input by the user, by members of the network and by the social media platform. Second, as users build their networks, they form ties which are visible (both for free and, on an expanded basis, for fee). As a result of this, the network’s structure is transparent, including the path and scale of information flows. Third, social media platforms limit users’ ability to nuance their relationship with others, who often get lumped in as “friends”, “family”, or “coworkers”, as if all were equal within categories homogenizing relational connections (Gilbert & Karahalios, Citation2009). Finally, social media networks allow users to create, access, and manipulate digital content, for the most part free of charge.
Resulting social media interactions operate under limited formal management. For instance, viral messaging across users can enable the rapid organization of groups without the need or time for managerial control, enabling large loosely connected groups to take credible, coordinated action of the sort that was typically the purview of formal organizations (Ansari & Phillips, Citation2011; Shirky, Citation2011). As nodes join a conversation, they can form and expand a specific issue network very rapidly by tapping into other networks through bridges between disparate groups of platform users. Because social media networks distribute digital content, nodes benefit from greater influence on the framing and agenda-setting of issues through the production of their own information about an issue—even if untrue (Besiou et al., Citation2013; Fieseler & Fleck, Citation2013). Specifically, social media networks empower nodes to influence perceptions of their issue’s importance by assigning it values and attributes with very few (if any) gatekeepers to edit or verify accuracy (Besiou et al., Citation2013; Carroll & McCombs, Citation2003; McCombs, Citation2004) allowing for the self-interested leverage of a network’s properties to manipulate the beliefs and behaviors of its users. Indeed, social media networks allow some nodes to design and control the nature of the network’s ties (Kane et al., Citation2014), enabling an unprecedented ability to disseminate content and influence outcomes (Besiou et al., Citation2013).
In this paper, we utilize a single case study to demonstrate self-interested exploitation of social media platform network features, as documented in the findings of the Mueller report investigating Russian social media interference, by their Internet Research Agency (IRA), in the 2016 U.S. presidential election (Mueller, Citation2019).
1.1. Supranodes
This analysis informs the study of power in networks by identifying unique social media network enabled nodes, such as the IRA and social media platforms, which we term more broadly as supranodes. These nodes are empowered via social media network awareness to socially engineer network structures to drive massive diffusion of information across networks and to influence outcomes. The social media scale and reach of the IRA activities analyzed in this case is visualized in Figure .
Figure 1. IRA supranode presence on Twitter, Facebook and Instagram during the U.S. 2016 presidential elections.
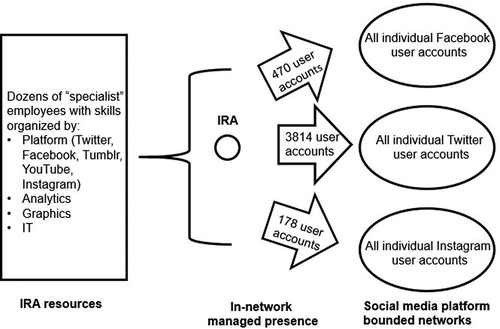
Supranodes are in many cases extra-network nodes, not visible within the network as explicit nodes within the boundaries chosen for analysis. However, supranodes create and manipulate within boundary network structures to achieve their desired outcomes as demonstrated with this analysis of IRA activities. Facebook’s creation of enabling features and muted response to Russian interference supports the classification of social media platforms as another alternative form of supranode that will be considered, highlighting the difficulty of social media platform self governance.
This paper is organized as follows: We begin with a selective literature review of network theory and social media network features that supranodes can exploit. Next, we discuss our case and methods and identify IRA actions demonstrating their exploitation of network features. The paper concludes with a broader consideration of supranode archetypes and the implications of these findings to the structure and governance of responsible innovation in a social media context.
2. Literature review
Borgatti and Halgin (Citation2011) note that network theories are framed in three different ways: network theory, the theory of networks and the network theory of networks. Network theory focuses on the structural impact of networks on non-network outcomes (i.e. election outcomes), independent of the consideration of individual node attributes. The theory of networks focuses on non-network independent variables (IV) and network dependent variables (DV). The network theory of networks considers situations studying network independent and dependent variables. To varying degrees supranodes are critical, active structural elements requiring consideration in all three network theoretic areas as suggested in Figure .
2.1. Social media and network theory
As noted by Wasserman and Faust (Citation1994) social network theory helps conceptualize network features in a social media context. In this section, we consider the social network theory underlying such features.
Node agency-driven behaviors, that is, manipulation of other users’ behavior and network outcomes to achieve desired results, become exacerbated in social media networks given the opportunity for variations in nodes’ network theoretic and social media platform knowledge (Devaraj & Kohli, Citation2003). On social media platforms, network structures and information flows can be manipulated by knowledgeable users.
Nodes can also use social media platform features in unintended ways (Boudreau & Robey, Citation2005). As an example, system features such as providing visibility to a node’s eigenvector centrality measurement, where greater centrality suggests a node has higher levels of connectivity to other highly influential nodes in the network, helps supranodes target such nodes for connectivity and content. Such network feature transparency facilitates the ability to manipulate networks even if this was not the driver for this transparency (Ren et al., Citation2007). In addition, despite the network data provided by the system, it is likely that many network nodes remain unable or indifferent to leveraging this information, expanding the knowledge gap between the network-aware nodes and others. The reality is that nodes in general vary considerably in their ability (and desire) to discern group network relations (Krackhardt, Citation1990; Krackhardt & Kilduff, Citation1999) or even to visualize their own networks (Marineau et al., Citation2018).
2.2. Network structure transparency
Network researchers provide guidance to knowledge-seeking nodes on how to leverage the information available about a social media network. For example, Maiz et al. (Citation2016) suggest methods to optimize social interactions by targeting content dissemination among Facebook followers through consideration of density and clustering data available on the platform. Density and clustering coefficient measures provide information on the level of connectedness of nodes in a network. Density measures the percent of potential ties in a network that are actually present and the cluster coefficient is a ratio of actual triangular node connections to the total number of possible triangular node connections within a network cluster. Knowing such information allows supranodes to target node and group (cluster) connections and content dissemination to maximize the spread of information they promote. Social media platforms provide access to numerous other measures representing the level of connectivity of individual nodes and assist in identifying clusters of connectivity within larger networks. Table provides a more complete list of such network theoretic measures. Providing access to such measures increases the efficiency of information dissemination for legitimate users, such as marketers, while at the same time arming supranodes to exploit this transparency.
Table 1. Network structural measures and IRA supranode impacts
2.3. Digital profiles and homogenized relational connections
Social media networks are larger than non-social media networks. The average number of Facebook user friends is 338 with a mean of 200 (Smith, Citation2019). Platforms allow extremely large numbers of connections, for example, 5000 friends on Facebook (Facebook, Citation2019). By contrast, in non-social media settings nodes typically maintain relationships with up to 150 individuals, including about 15 close friends (Dunbar & Hill, Citation2002). Indeed, there are limits to the number of ties that a node can effectively maintain (Pollet et al., Citation2011).
A distinguishing feature of social media networks is the prevalence of clusters of nodes whose only commonality is a specific topic of interest. That is, clusters form among nodes who share no interpersonal relationship but commonly care about a given topic or the viewpoint represented by the cluster, with members having strong issue identity. As such, these ties serve the purpose of asserting one’s position regarding an issue while remaining disconnected in every other way to other nodes doing the same. A node’s participation in a cluster may enable information flow either explicitly, via retweets, or implicitly, via posts appearing on one’s Facebook timeline, as examples. In clusters, nodes have predominantly homogeneous views on the narrow issue they endorse and a low threshold related to the dissemination of related information both within and beyond the cluster. The ease with which these clusters form through social media dramatically increases a node’s bridging power. These clusters of ties enable greater homogeneity, which happens through two related mechanisms: the ease of access to issue networks enables nodes to learn about the values and activities of a group prior to joining, and a node’s ability to start their own group based on their particular views or to be ostracized from a group by members attempting to protect the group’s identity (Sunstein, Citation2001). These resulting ties impact network structures and behavior by establishing clusters of nodes who share common characteristics at deep levels (emotionally and psychologically) as opposed to the homogeneity of non-social media clusters that are largely rooted in characteristics such as geographic location, demographics, or lifestyle (Rogers, Citation2003).
The relative anonymity of online group members intensifies the need for nodes to adopt group identities because they rely primarily on limited cues when conforming to group norms (Cha et al., Citation2010) where the main cue is issue endorsement (Donath & Boyd, Citation2004). In more homogeneous networks, institutional norms and values diffuse rapidly, resulting in mimetic behaviors and shared behavioral expectations across the network (Oliver, Citation1991; Rowley, Citation1997). Indeed, studies show that cybernorms tend to diffuse more quickly than traditional social norms (Major, Citation2000). Taken together, these characteristics of social media networks facilitate the prevalence of clusters among which network participants tend to present more convergent viewpoints (Balzarova & Castka, Citation2012).
Through curated and limited profiles nodes become homogenized and fail to reflect the diversity they represent. The constrained node profile data also encourages pseudonymity, identities consistent with the network context but unrelated to a node’s offline identity (Kane et al., Citation2014). Aliases can also be created to conceal and expand overall node presence in a network and have been observed on social media networks related to protests, dating, and election campaigns (Salge & Karahanna, Citation2018).
In social media networks, many ties and clusters are no longer formed based on complex personal interactions. Thus, connections can also be established by virtual, computer-generated individuals or groups. These bot tie connections are typically created to artificially indicate support for an issue by participating in clusters or serving as bridges for information to diffuse across clusters. Studies in the field of human-computer interaction indicate that people treat bots similarly to real people (Nass & Moon, Citation2000; Nass et al., Citation1995) and respond to computer personalities in the same way they do to humans (Nass et al., Citation1999; Reeves & Nass, Citation1996). Thus, although bot ties are non-human nodes, they function as perceived human connections in the attenuated contact environment of a social media network and have real effects on the diffusion of issues and network structure.
2.4. Node created digital content
Digital profiles and trace provide additional details on nodes through pre-defined, albeit limited, content that communicates node features and activity (i.e. comments, status updates, views, likes) The fact that digital profiles and trace are visible to nodes on the network impacts network behavior, facilitating herd behaviors (Oh & Jeon, Citation2007) and information cascades (Aral & Walker, Citation2011; Bampo et al., Citation2008; Hinz et al., Citation2011). Such data also inform supranodes allowing them to create and target content and connections to easily influenced nodes.
In addition, through information streams (i.e. Facebook NewsFeed) and algorithmic search capabilities enabled by digital profiles and network transparency (Ellison & boyd, Citation2013) social media networks also enable information flows among non-connected nodes through various content access mechanisms. These features amplify the presence of trending data streams, whether true or fabricated, and allows access to other nodes’ information without even establishing relational connections with the source node (Kane et al., Citation2014).
2.5. Impacting network outcomes: the example of information cascades
Even where a connection is established with another tie, the strength of ties, a common measure used in analyses of non-social media networks, is typically missing from social media network informatics. Network theorizing in this area builds off Granovetter’s (Citation1973) strength of weak ties theory and its strong versus weak tie dichotomy and transitivity considerations (Freeman, Citation1979). Both Granovetter’s (Citation1973) tie strength and Burt’s (Citation1992) related work on structural holes focus on similar consequences of varying tie typologies and novel information access (Borgatti & Halgin, Citation2011). More recently, tie typologies have been expanded by Borgatti et al. (Citation2009) into similarities (proximates), social relations, interactions, and flows to better account for the context of ties in social media networks.
Traditional measures of tie strength include temporal duration, emotional intensity, intimacy, and reciprocal services (Burt, Citation1992; Granovetter, Citation1973). Various measures of frequency and closeness have been applied to measure tie strength in previous research studies and in practice (c.f. Hansen, Citation1999). Nodes are expected to have fewer strong ties and many weak ties, and are posited to focus resources on strong tie maintenance (Burt, Citation1992), thus creating a practical limit on the ability to make and maintain these stronger ties (Mayhew & Levinger, Citation1976). Connections to bots and many clusters are made via weak ties; however, the scale and collective action characteristics of these ties allow them to have a distinctive and oversized role in network behaviors and outcomes. Given the above, the dramatic growth in a node’s ties in social media contexts is primarily driven by an expansion of weak ties.
A widely accepted model for understanding how information and behavior diffuse in traditional social networks is the thresholds model (Granovetter, Citation1978). Thresholds represent “the number or proportion of others who must make one decision before a given actor does so” (Granovetter, Citation1978, p. 1420) and enable modeling how individual preferences for engaging in a behavior or spreading information interact and aggregate. The threshold model helps explain network cascades, where individuals follow others’ behavior (Anderson & Holt, Citation1997; Barton, Citation2009; Welch, Citation1992). Cascades are explained by the theory of observational learning (Bandura, Citation1977; Bastos & Farkas) occurring mainly due to individuals’ inherent desire to conform with social norms and to be accepted in social networks, leading them to follow the behavior of others (Bikhchandani et al., Citation1992; Smith & Sorensen, Citation2000; D. Watts, Citation2002). Celen and Kariv (Citation2004) observe that information cascades often arise (35% of the time in their research) due to Bayesian updating, using data to continuously update the probability of action based upon the observation of others. Observational learning theory and information cascade formation require a discrete choice: a node does or does not like a cause on Facebook as an example, a common situation in social media contexts (Bikhchandani et al., Citation1998). In a related manner, social contagion and influence can also trigger cascades.
A number of models help in understanding information cascades. In studies of binary choice models, the likelihood of a cascade occurring increases with the number of nodes involved and as the nodes’ probability for responding to a cascade topic becomes greater than 50% (Bikhchandani et al., Citation1992; D. Watts, Citation2002). The larger volumes of ties in social media networks, the ease of visibility and action, conformity pressures (Bikhchandani et al., Citation1998), dee-seated commonalities around issues (Chesney, Citation2016), and the binary nature of many platform choices lead to accelerated and larger information cascades in social media contexts.
Bot ties further add to these phenomena by magnifying diffusion in networks, at times even artificially crafting the appearance of a cascade. Altogether, the structural elements of social media favor the occurrence of cascades by making it easier for each node to diffuse issues (Besiou et al., Citation2013). Bots and clusters result in more nodes, more connections, and more nodes (% and actual) supporting potential cascade issues, thus positively increasing variables that result in greater cascade formation (Bikhchandani et al., Citation1992; D. Watts, Citation2002). While a node’s influence is D. J. Watts (Citation2004), (Citation2007)) indicate that a critical mass of easily influenced nodes may be far more significant to diffusion. In social media, bots and clustering contribute to the perception of a critical mass and raise awareness, feeding information cascades by increasing the likelihood of exceeding node thresholds.
Bot ties, at an interpretive and impact level, act as “real” ties in impacting the flow of information in social media networks. In Table bots are positioned within the existing tie typology of Borgatti et al. (Citation2009) with their distinctive role in cascade generation.
Table 2. Type of network ties and role in generating cascades
3. Research method
This analysis explores how supranodes exploit the unique characteristics of social media networks as outlined by Kane et al. (Citation2014): network structure transparency, digital profiles, homogenized network connections and node created digital content. The case method used in this analysis is uniquely positioned to respond to such “how” questions (Yin Citation2009) and is particularly powerful in studying causal mechanisms or processes (George & Bennett, Citation2005), such as how a supranode’s network manipulation impacts outcomes.
The Mueller Report provides a unique, extreme and revelatory single holistic case for supranode theoretical development. Single case analyses are a common research design in such case contexts. (Yin Citation2009). The unit of analysis for this case is the time-bound behavior of the IRA during the 2016 U.S. Presidential election period. As noted in the report “The IRA … used social media accounts and interest groups to sow discord in the U.S. political system through what it termed ‘information warfare.’ The campaign evolved … to a targeted operation that by early 2016 favored candidate Trump and disparaged candidate Clinton.”
3.1. Case data, indexing, and analysis
The scale and rigor of the Mueller investigation meet the three critical design principles of case data collection: multiple sources of evidence, a documented dataset of findings, and a chain of evidence, typical in such forensic investigations (Yin Citation2009). The investigation into Russian interference took place between May 2017 and March 2019 at a cost of nearly $32 million (Breuninger, Citation2019). Special Counsel Mueller had access to both classified and unclassified information available from the FBI that had been investigating Russian interference for ten months prior to the Special Counsel’s appointment. The Special Counsel’s team employed 19 attorneys, 3 paralegals, 9 administrative staff and was assisted by 40 FBI support team members. In the course of the investigation approximately 500 witnesses were interviewed and 2,800 subpoenas, 500 search and seizure warrants, and 230 orders for communication records were issued (Mueller, Citation2019).
NVivo qualitative data analysis software was used to facilitate the text analysis of the 448-page Mueller report, a tool commonly used in case research (Jackson & Bazeley, Citation2019). The text was initially parsed using keywords proposed by the authors, which included: bot, troll, social media, Instagram, Twitter, Facebook, personas, online, media, advertisement, target, search, digital, display, IRA, internet research agency, posts, likes, tweets, and internet. NVivo’s auto code feature was next utilized to suggest additional keywords for tagging resulting in the addition of the following tags to the search based on the author’s determination of potential relevance to social media manipulation: fictional, information warfare, falsified, disinformation, suppression, foreign influence, asymmetric, authentic, automated, propaganda, leaked, gru, dcleaks, computers, cyber, and fake.
Section headings also helped guide the text analysis. Sections of the report highly relevant to this analysis included: Russian “active measures” social media campaign; Structure of the Internet Research Agency (IRA); IRA targets U.S. elections; IRA ramps up U.S. operations as early as 2014; U.S. operations through IRA-controlled social media accounts; U.S. operations through Facebook; U.S. operations through Twitter (individualized accounts; IRA botnet activities); and Targeting and recruitment of U.S. persons (Mueller, Citation2019).
The resulting parsed text was reviewed by the authors and indexed to reflect relevance to Kane et al. (Citation2014) four posited distinct social media network features. This method of indexing case study data via propositions (in this case the distinct network features enabling potential media exploitation) is the preferred analytical approach to assist in sorting through large volumes of text (Yin Citation2009). The parsed text, as quotes or in summary form, is reported in the following section.
4. Findings and discussion
In this section, we consider how the IRA supranode exploited the unique features of social media networks, as outlined in Kane et al. (Citation2014) to influence the 2016 U.S. Presidential election. All quotes in this section come from the Mueller report (Mueller, Citation2019).
4.1. Digital profiles and homogenized relational connections
IRA employees leveraged the ability to create non-verified, attenuated social media profiles to create fictitious U.S. individual personas and organizations. These fictitious entities attracted U.S. audiences with fabricated messages directly targeted to real social media users that the platform data suggested would be interested in the divisive U.S. political and social issues that the IRA desired to amplify.
“ … IRA employees posed as U.S. grassroots entities and persons and made contact with Trump supporters and Trump Campaign officials … Using fictitious U.S. personas, IRA employees operated social media accounts … designed to attract U.S. audiences [and] … claimed to be controlled by U.S. activists.”
The IRA also utilized impersonation while operating on these networks to hide their identity at the platform level: “ … buying political advertisements on social media in the names of U.S. persons and entities.”
The IRA created fake organizations to attract nodes to join their groups to increase clustering and reach. “ … The IRA began to create larger social media groups … that claimed (falsely) to be affiliated with U.S. political and grassroots organizations.”
The report further notes: “ … the IRA created accounts that mimicked real U.S. organizations. For example, one IRA-controlled Twitter account, @TEN_GOP, purported to be connected to the Tennessee Republican Party. More commonly, the IRA created accounts in the names of fictitious U.S. organizations and grassroots groups, using these accounts to pose as anti-immigration groups, Tea Party activists, Black Lives Matter protestors, and other U.S. social and political activists.”
The attenuated/unverifiable nature of social media digital profiles made the transmission of false content effective, bridging in many cases beyond the social media platforms and being effectively promoted by high profile targeted individuals. “ … U.S. media outlets also quoted tweets from IRA-controlled accounts and attributed them to the reactions of real U.S. persons. Similarly, numerous high-profile U.S. persons … responded to tweets posted to these IRA controlled accounts. Multiple individuals affiliated with the Trump Campaign also promoted IRA tweets.”
The limitations of digital profiles and the homogenization of relational connections makes it easy for supranodes to create bot accounts to amplify diffusion: “ … the IRA operated a network of automated Twitter accounts (commonly referred to as a bot network) that enabled the IRA to amplify existing content on Twitter.” In doing this the IRA leveraged network features to engage the logic of numbers (Diani, Citation2000), triggering thresholds to create information cascades. The IRA-established groups and formed clusters that facilitated information flows through low group diffusion thresholds.
4.2. Network structure transparency
The IRA used profile/trace capabilities to target nodes on the networks. Network clusters were targeted based on their likelihood to effectively disseminate IRA content. Due to the targeted nature of the outreach and messaging IRA bot content was blindly liked, forwarded and followed by target nodes.
Target nodes were also engaged to create content and conduct tasks: “ … the IRA instructed its employees to target U.S. persons who could be used to advance its operational goals … frequently used Twitter, Facebook, and Instagram to contact and recruit U.S. persons … . the IRA tracked U.S. persons with whom they communicated and had successfully tasked (with tasks ranging from organizing rallies to taking pictures with certain political messages).”
4.3. Node created digital content
A key strategy of the IRA supranode was to create and disseminate divisive digital content “ … by continuously posting original content to the accounts while also communicating with U.S. Twitter users directly (through public tweeting or Twitter’s private messaging) … .” Posts were socially engineered to affirm the beliefs of targeted nodes and to encourage diffusion. IRA Facebook groups addressed a range of political issues and included purported conservative groups (with names such as “Being Patriotic,” “Stop All Immigrants,” “Secured Borders,” and “Tea Party News”).
The IRA also purchased 3,500 advertisements to promote its’ groups on Facebook’s News Feed. A specific example of these actions includes: “ … on 6 April 2016, the IRA purchased advertisements for its account ‘Black Matters’ calling for a ‘flashmob’ of U.S. persons to ‘take a photo with #HillaryClintonForPrison2016 or #nohillary2016.”
IRA Facebook, Twitter and Instagram accounts attracted hundreds of thousands of real users. “ … Multiple IRA-controlled Facebook groups and Instagram accounts had hundreds of thousands of U.S. participants. IRA-controlled Twitter accounts separately had tens of thousands of followers … .” As explicit examples “ … the IRA’s ‘United Muslims of America’ Facebook group had over 300,000 followers, the ‘Don’t Shoot Us’ Facebook group had over 250,000 followers, the ‘Being Patriotic’ Facebook group had over 200,000 followers, and the ‘Secured Borders’ Facebook group had over 130,000 followers.” Colin Stretch, General Counsel of Facebook “ … testified that Facebook had identified 170 IRA created Instagram accounts that posted approximately 120,000 pieces of content … .”
The IRA attempted to incite groups through suggestive titles and initial posts. However, it was actual users’ posts, discussions, shares, likes, actions, and behaviors that shaped the group’s values, identity and expansive content dissemination.
4.4. Network structural impacts of IRA actions
IRA activities intensified information flows via these IRA-established nodes and groups.
“In January 2018, Twitter publicly identified 3,814 Twitter accounts associated with the IRA. … in the ten weeks before the 2016 U.S. presidential election, these accounts posted approximately 175,993 tweets … [and] Twitter … notified approximately 1.4 million people who Twitter believed may have been in contact with an IRA-controlled account. Facebook testified … that roughly 29 million people were served content in their News Feeds directly from the IRA’s 80,000 posts over the two years. Posts from these pages were also shared, liked, and followed by people on Facebook and, as a result, three times more people may have been exposed to a story that originated from the Russian operation. [The] … best estimate is that approximately 126 million people may have been served content from a page associated with the IRA at some point during the two-year period.”
4.5. Operational scale
Despite disguising its operations as disconnected grassroots efforts, the IRA in reality employed a large staff tasked with managing their social media efforts and with hiding their identity. As noted: “[the] IRA subdivided the Translator Department into different responsibilities, ranging from operations on different social media platforms to analytics to graphics and IT. … Dozens of IRA employees were responsible for operating accounts and personas on different U.S. social media platforms. The IRA referred to employees assigned to operate the social media accounts as ‘specialists.’ … [and] the IRA closely monitored the activity of its social media accounts.”
The IRA supranode, which is an extra-network structural feature, leverages intra-network nodes that it creates (bots) or effectively controls (proxies, aliases) or manipulates (via ties to targeted nodes or policies established) to achieve its objectives. The supranode leverages the bond model of network research (Borgatti & Halgin, Citation2011), establishing relative power advantages over other nodes in the network. The unique features of social media networks empower supranodes, impacting network structures and outcomes as outlined earlier in Table and in Table below.
Table 3. Supranode sample actions and impacts on network structure and outcomes
5. Conclusion
Facebook, as a leading social media platform, was aggressively leveraged by the IRA as highlighted by the 309 references to the platform in the Mueller report (Mueller, Citation2019). As noted in The New York Times in a 14 November 2018 article, Facebook engineers and senior management were aware of the IRA campaign to disrupt the 2016 Presidential election well before the election. Warning signs were ignored and an effort was made to conceal this knowledge from the public. Facebook was aware of probes by Russian hackers of user accounts and their messaging of journalists on the platform to share information from the Democratic campaign’s stolen emails. These activities were investigated by Facebook’s chief security officer raising the ire of Facebook’s senior leadership for making the company “exposed legally.”
A project to study false news on the site was officially launched concluding that the scale of the Russian interference was much larger than initially noted. To avoid a potential political backlash and user anger, Facebook’s senior management decided not to visibly act upon their findings and proceeded to make public claims that no significant Russian interference took place. However, internal audits and external queries from reporters on specific accounts and posts suggested obfuscation was not a viable strategy and Facebook made a public blog post stating that Russian agents had spent a modest $100,000 on approximately 3,000 platform ads. On 7 September 2017, The New York Times published another article on “The Fake Americans Russia Created to Influence the Election” followed by an October 2017 Facebook acknowledgement that Russian posts had been presented to 126 million Facebook users. Facebook simultaneously launched aggressive lobbying and deflection campaigns to redirect political attention to other issues and firms.
Post the 2016 election exposés Facebook executed several platform modifications and undertook internal research on the impact of the platform on users; however, learnings from the IRA interference campaign and other internal Facebook platform research frequently did not translate into action. During the week of September 13 2021 The Wall Street Journal posted a series of articles titled “The Facebook Files.” These articles highlighted Facebook feature modifications, policies and continued obfuscation of research findings that benefited the platform but that similarly enabled rogue nodes and negatively impacted user outcomes. As examples, the articles exposed a Facebook program called “Xcheck” where the platform exempts millions of high-profile account holders from the platform’s appropriate use policies. Facebook also modified its News Feed algorithm to purportedly boost “meaningful social interactions” (MSI) between friends and families with Facebook’s CEO stating that the algorithm change was designed to strengthen bonds between users and to improve their well-being. Internal research at Facebook and external feedback suggested that this change was having the opposite effect, encouraging outrage and sensationalism with “misinformation, toxicity, and violent content … inordinately prevalent among shares.” As noted by internal researchers, the algorithm did increase comments and reactions, driving Facebook views and traffic to be monetized.
5.1. Socialwashing by Facebook?
In the environmental/climate arena the term greenwashing was coined to label a corporation’s emphasis on observable aspects of environmentally responsible activities while neglecting less visible irresponsible behaviors (Wu et al., Citation2020). The term also describes firm efforts at a product level to mislead consumers about the environmental friendliness of their offerings (Delmas & Burbano, Citation2011). Greenwashing activities in general are the misleading of stakeholders about environmental concerns with a company’s products or activities (Netto et al., Citation2020).
So how do we classify Facebooks failure to be forthcoming about IRA activities or to take corrective action in a timely manner? How is the Xcheck policy that exempts influential nodes from platform rules of behavior equitable or appropriate? Why did Facebook proceed with the new NewsFeed algorithm, promoting its benefits to users while knowingly concealing the negative impact of expanded misinformation dissemination to name just one of the issues highlighted? These behaviors are similar to greenwashing in terms of approach and intent however the context and nuances are distinct given the social media context, warranting a distinctive classification which we term socialwashing.
There are numerous examples of socialwashing by Facebook, beyond those already identified. In a classic case of socialwashing Facebook in 2018 created a high-profile oversight board to review decisions on how it handles specific user posts as highlighted on the Facebook Oversight Board website. Once fully constituted the Board will be made up of 40 outside experts and civic leaders chartered to “ … answer some of the most difficult questions around freedom of expression online: what to take down, what to leave up, and why.” As the Board website notes the “board only selects a small number of appeals to review.”
The oversight board has a limited charter to address the concerns of more than 2.2 billion active users. As noted in an 13 April 2021 story by Reuters the initial Board charter was to review content that had been removed from the platform at Facebook’s request; this was adjusted in 2021 to allow review of content still posted on the platform. While the board can also recommend policy changes these recommendations are not binding on the firm. At the time of Reuter’s publication, the board had received 300,000 appeal requests. The board size and constrained charter, given the scale and impact potential of the issues involved, suggests a failure of reasonableness to this approach.
The New York Times in an 20 August 2021 article (updated 4 October 2021) referenced additional Facebook socialwashing. In this instance, the firm prevented the public release of a report on its most viewed posts in the U.S. for the first quarter of 2021 given the negative optics of the findings, with the most-viewed post faulting the coronavirus vaccine for the death of a doctor. The report was released post the publication of The New York Times article. A report for the second quarter of 2021 on popular posts with innocuous findings was released without such outside pressure.
5.2. Supranode archetypes
Network theory acknowledges the importance of node attributes to outcomes, showing how homophily tends to lead to stronger ties (McPherson et al., Citation2001) as an example. Individual node agency, when used to shape networks, is acknowledged as critical; yet the network outcome of the shaping is typically considered separately in network analyses (Borgatti & Halgin, Citation2011). Supranodes, however, represent a form of collective agency at a network level. Nodes under a supranode’s control will all actively behave with a consistency that impacts network structures as well as outcomes. Supranodes may also externally dictate node participation and behavior in an active manner (if you act in a certain way you are removed from the network/group or with Facebook’s Xcheck not removed if you are an influential individual, as examples) and define the rules of network engagement (visibility or not to node characteristics as examples).
The IRA presence was via the fake groups and nodes it established; however, the IRA was not explicitly present in these networks but rather represented by the network elements under its control. To describe the IRA, we utilized the term supranode, leveraging supra’s definition to indicate the distinctiveness of this feature: above, over, beyond the limits of (Dictionary.com, Citation2019).
Power advantages accrue to supranodes, and the cost of supranode actions is moderated by platform capabilities. Self-interested behavior (to the extent that is the supranode intent) is common online as shown in a study of crowdsourcing competitions suggesting that malicious behavior is a norm, not an anomaly, in that online context (Naroditskiy et al., Citation2014).
In Table we suggest a range of supranode archetypes with exemplars and a categorization of the supranode drivers utilizing the collective intelligence genome constructs from Malone et al. (Citation2010) and the pressures they exert on existing network nodes from Suchman (Citation1995). Supranode activities impact various network structures as noted.
Table 4. Supranode archetypes, exemplars, drivers, and pressures
This paper frames an argument for the importance of network theory to social media responsible innovation governance; however, other theoretical frameworks are also highly relevant to understanding social network features and consequences. Other research studies have applied propaganda theory to the analysis of the IRA (CitationBastos & Farkas,) and agenda setting theory to the analysis of fake news (Guo & Vargo, Citation2018). Future research may suggest the extension of these and other communication theories, such as social cognitive theory and excitation transfer theory as examples, to the bedrock upon which social media responsible innovation is conducted.
5.3. Governance of social media responsible innovation
Socialwashing on the part of Facebook and other social media platforms is not surprising given their network position of power as a supranode and the conflicted revenue versus pro-social behavioral tradeoffs they face in making responsible innovation decisions. The network theoretic features that can result in negative societal consequences are often times the same features that drive critical revenue streams. Self-governance is extremely difficult given these challenges and the potential relative negative impact on platform providers that take a pro-social stance that is not enforced industry wide. These challenges are not unique to Facebook in the social media arena and their predictable responses no different than the greenwashing by big oil and gas relative to climate change or big tobacco’s obfuscation of health risks from smoking.
Trust issues can also arise regarding functionality that is typically viewed as key value added from social media platforms, such as product reviews, which may be generated by bots but nevertheless be relied upon by consumers. This has important implications for new and existing social media empowered services. In addition, the reduced expense of manipulative campaigns to achieve social media empowered objectives has implications for the advertising business models employed by many of the most successful global social media companies. The need for independent regulation and oversight of social media responsible innovation, informed by a network theoretic lens, for the benefit of both industry and society is thus suggested.
To effectively function, social media governance must be grounded in theory. Board members and regulatory staff responsible for such governance should be trained in network science similar to the financial expertise required for fiduciary board governance or environmental science competence for Environmental Protection Agency staff. The importance of the independence of governance from platform operations is also clear given the conflicted nature of the tradeoffs required. Given the competitive impact of such decisions, industry-wide versus targeted actions or self-governance seems warranted since all social media platform providers face the same issues/tradeoffs.
5.4. Outcomes
The Mueller report (Mueller, Citation2019) acknowledged Russian interference in the 2016 U.S. election; however, given the legal lens applied, it did not state that the election outcome was changed by these efforts, although Donald Trump, the benefitting candidate, was elected President. The continued engagement by Russia in election interference in Ukraine post their U.S. efforts suggests the initiative outcomes to have been viewed favorably by its orchestrators (Polyakova, Citation2019).
A network theoretic lens suggests that interference which meaningfully impacts network independent variables (numbers of nodes, ties, groups) should influence outcomes (information flows, election results). In the 2016 U.S. Presidential election Hillary Clinton won the popular vote by 2,868,186 votes despite the IRA supranode’s campaign favoring Donald Trump. However, the outcome in a U.S. Presidential election is a more nuanced matter than popular vote counts reflect given the use of state-level electors in determining the prevailing candidate. In the majority of states, electors are assigned in a winner-take-all manner (National Archives, Citation2022). This mechanism enables a small number of swing states, those with closely split electoral support, to play a critical role in determining the outcome of such federal elections. An analysis of IRA supranode activities suggest that these states were explicitly targeted by these interference campaigns (McCombie et al., Citation2020).
What type of impact would the supranode’s interference have needed to trigger a change in election outcomes? In the swing states of Pennsylvania, Wisconsin and Michigan Trump’s popular vote margin was 44,292, 22,748 and 10,704 respectively (Federal Election Commission, Citation2016). If just over half these votes had gone for Clinton instead of Trump (38,875 total votes or 0.3% of the overall votes in these three states) the overall election outcome would have been different.
Beyond the Mueller report additional research studies considered the scale of 2016 election interference. Grinberg et al. (Citation2019) studied registered voter’s exposures to fake news in the final months of the election noting 1.18% of political news exposures being “fake” during this period. Guo and Vargo (Citation2018) noted that fake news online added noise to an already sensationalized media environment. In a larger analysis of 171 million tweets in the five months preceding the election Bovet and Makse (Citation2019) suggest that 25% of tweets spread either fake or extremely biased news.
At a minimum, a network theoretic framing would change the form of discourse on this topic. A common statement on IRA interference notes that there is no proof that IRA interference changed the outcome of the 2016 election (Mueller, Citation2019); however, this statement begs the question as to what exactly would constitute proof in a social media interference campaign context? As suggested by this paper, a more accurate response would be “utilizing a network theoretic lens and given the scale of interference it is likely that the IRA social media campaign impacted the 2016 election outcomes.” This impact on discourse is in and of itself a valuable benefit to applying a network theoretic lens to such considerations.
A network theoretic lens also suggests that platform features that unlevel the playing field for users should be scrutinized carefully, with Facebook’s Xcheck program an obvious candidate for such review. Features such as MSI that knowledgeable platform providers will exploit through sensationalized postings to increase views for their own and the platform’s benefit similarly fits within the suspect category against a network theoretic lens. Change is clearly needed in the social media responsible innovation space with the modest proposals from this paper a necessary but insufficient step. In addition, platform providers have unique access to user data that can inform research and assist in evaluating current and future innovations. Providing such data for academic use would be a valuable step toward transparency and non-bias for reported outcomes.
Disclosure statement
No potential conflict of interest was reported by the author(s).
Additional information
Funding
Notes on contributors
Patrick McHugh
Dr. Patrick McHugh is Director of and Professor of the Practice in the School of Engineering Innovation Management & Design Engineering Group at Brown University where he teaches courses in finance and strategy. His research interests focus on innovation, legitimacy and network theory in social media and decision-making contexts. His work has been published in the Journal of General Management, Journal of Management and Organizations, Journal of Prediction Markets, and MIS Quarterly Executive. He has over 25 years of industry experience, including executive roles at three venture-backed data security firms. He is the recipient of two patents in the area of call center automation and holds B.S. and M.S. degrees in engineering from Columbia University, an M.B.A. from Harvard Business School, and a Ph.D. in management from Bentley University.
Elise Perrault
Dr. Elise Perrault is an Associate Professor of Management in the Department of Management & Marketing at the College of Charleston’s School of Business. Her research interests center on stakeholder management, shareholder activism, and corporate governance. She has published in the Journal of Business Ethics, Business & Society and the Journal of Management & Organization, among others. Dr. Perrault obtained her Ph.D. in Business Strategy from Bentley University in 2012 and holds an MBA from McGill University. She is fluent in both French and Spanish. Currently she teaches the Business degree’s capstone course, ”Business Policy” and the course in “Leadership & Social Responsibility”. Elise is the Director for the School of Business’ ”Think Differently Forum” which is comprised of a term of students and held each semester. Prior to joining academia, Elise was a successful entrepreneur and consultant, specializing in business transition, planning, and strategy.
References
- Anderson, L. R., & Holt, C. A. (1997). The American Economic Review, 87(5), 847–18.
- Ansari, S., & Phillips, N. (2011). Text me! New consumer practices and change in organizational fields. Organization Science, 22(6), 1579–1599. https://doi.org/10.1287/orsc.1100.0595
- Aral, S., & Walker, D. (2011). Creating social contagion through viral product design: A randomized trial of peer influence in networks. Management Science, 57(9), 1623–1639. https://doi.org/10.1287/mnsc.1110.1421
- Balzarova, M. A., & Castka, P. (2012). Stakeholders’ influence and contribution to social standards development: The case of multiple stakeholder approach to ISO 26000 development. Journal of Business Ethics, 111(2), 265–279. https://doi.org/10.1007/s10551-012-1206-9
- Bampo, M., Ewing, M. T., Mather, D. R., Stewart, D., & Wallace, M. (2008). The effects of the social structure of digital networks on viral marketing performance. Information Systems Research, 19(3), 273–290. https://doi.org/10.1287/isre.1070.0152
- Bandura, A. (1977). Social learning theory. Prentice Hall.
- Barton, A. M. (2009). Application of cascade theory to online systems: A study of email and Google cascades. Minnesota Journal of Law, Science & Technology, 10(2), 473–502. scholarship.law.umn.edu/cgi/viewcontent.cgi?article=1199&context=mjlst
- Bastos, M., & Farkas, J. Donald Trump is my President!: The internet research agency propaganda machine. Social Media + Society: 1–13 https://doi.org/10.1177/2056305119865466
- Besiou, M., Hunter, M. L., & Van Wassenhove, L. N. (2013). A web of watchdogs: Stakeholder media networks and agenda-setting in response to corporate initiatives. Journal of Business Ethics, 118(4), 709–729. https://doi.org/10.1007/s10551-013-1956-z
- Bikhchandani, S., Hirshleifer, D., & Welch, I. (1992). A theory of fads, fashion, custom, and cultural change as informational cascades. Journal of Political Economy, 100(5), 992–1026. https://doi.org/10.1086/261849
- Bikhchandani, S., Hirshleifer, D., & Welch, I. (1998). Learning from the behavior of others: Conformity, fads and informational cascades. Journal of Economic Perspectives, 12(3), 151–170. https://doi.org/10.1257/jep.12.3.151
- Borgatti, S. P., & Halgin, D. S. (2011). On network theory. Organization Science, 22(5), 1168–1181. https://doi.org/10.1287/orsc.1100.0641
- Borgatti, S. P., Mehra, A., Brass, D. J., & Labianca, G. (2009). Network Analysis in the Social Sciences. Science, 323(5916), 892–895. https://doi.org/10.1126/science.1165821
- Boudreau, M. C., & Robey, D. (2005). Organization Science, 16(1), 3–18.
- Bovet, A., & Makse, H. (2019). Influence of fake news in Twitter during the 2016 US presidential election. Nature Communications, 10(1), 7. https://doi.org/10.1038/s41467-018-07761-2
- boyd, D. M., & Ellison, N. B. (2007). Social network sites: Definition, history, and scholarship. Journal of Computer-Mediated Communication, 13(1), 210–230. https://doi.org/10.1111/j.1083-6101.2007.00393.x
- Breuninger, K. 2019. Robert Mueller’s Russia probe cost nearly $32 million in total, Justice Department says. CNBC, https://www.cnbc.com/2019/08/02/robert-muellers-russia-probe-cost-nearly-32-million-in-total-doj.html
- Burt, R. (1992). Structural holes: The social structure of competition. Harvard University Press.
- Butler, B. (2001). Membership size, communication activity, and sustainability: A resource-based model of online social structures. Information Systems Research, 12(4), 346–362. https://doi.org/10.1287/isre.12.4.346.9703
- Carroll, C. E., & McCombs, M. E. (2003). Agenda-setting effects of business news on the public’s images and opinions about major corporations. Corporate Reputation Review, 6(1), 36–46. https://doi.org/10.1057/palgrave.crr.1540188
- Celen, B., & Kariv, S. (2004). Distinguishing informational cascades from herd behavior in the laboratory. American Economic Review, 94(3), 484–498. https://doi.org/10.1257/0002828041464461
- Cha, M., Haddadi, H., Benevenuto, F., & Gummad, K. P. 2010. “Measuring user influence on Twitter: The million-follower fallacy.” AAAI Conference on Weblogs And Social Media, May 16 https://www.aaai.org/ocs/index.php/ICWSM/ICWSM10/paper/view/1538/1826
- Chesney, T. (2016). The cascade capacity predicts individuals to seed for diffusion through social networks. Systems Research and Behavioral Science, 34(1), 51–61. https://doi.org/10.1002/sres.2398
- de Bakker, F. G. A., & Hellsten, I. (2013). Capturing online presence: Hyperlinks and semantic networks in activist group websites on corporate social responsibility. Journal of Business Ethics, 118(4), 807–823. https://doi.org/10.1007/s10551-013-1962-1
- Delmas, M., & Burbano, V. (2011). The drivers of greenwashing. California Management Review, 54(1), 64. https://doi.org/10.1525/cmr.2011.54.1.64
- Devaraj, S., & Kohli, R. (2003). Performance impacts of information technology: Is actual usage the missing link? Management Science, 49(3), 273–289. https://doi.org/10.1287/mnsc.49.3.273.12736
- Diani, M. (2000). Social movement networks virtual and real. Information, Communication & Society, 93(3), 386–401. https://doi.org/10.1080/13691180051033333
- Dictionary.com. 2019. https://www.dictionary.com/browse/supra-
- Donath, J., & Boyd, D. (2004). Public displays of connection. BT Technology Journal, 22(4), 71–82. https://doi.org/10.1023/B:BTTJ.0000047585.06264.cc
- Dunbar, R., & Hill, R. (2002). Social network size in humans. Human Nature, 14(1), 53–72. researchgate.net/publication/281203308_Social_Network_Size_in_Humans
- Ellison, N. B., & boyd, D. M. (2013). Sociality through Social Network Sites. In W. H. Dutton (Ed.), The Oxford Handbook of Internet Studies (pp. 151–172). Oxford University Press.
- Facebook. 2019. https://www.facebook.com/help/community/question/?id=765037383609149
- Federal Election Commission. 2016. Federal elections 2016 election results for the U.S. President, the U.S. Senate and the U.S. house of representatives. https://www.fec.gov/resources/cms-content/documents/federalelections2016.pdf
- Fieseler, C., & Fleck, M. (2013). The pursuit of empowerment through social media: Structural social capital dynamics in CSR-blogging. Journal of Business Ethics, 118(4), 759–775. https://doi.org/10.1007/s10551-013-1959-9
- Freeman, L. (1979). Centrality in social networks: Conceptual clarification. Social Networks, 1(3), 215–239. https://doi.org/10.1016/0378-8733(78)90021-7
- George, A., & Bennett, A. (2005). Case studies and theory development in the social sciences. MIT Press.
- Gilbert, E., & Karahalios, K. 2009. Predicting tie strength with social media. Proceedings of the 27th International Conference on Human Factors in Computing Systems 211–220.
- Granovetter, M. (1973). The strength of weak ties. American Journal of Sociology, 78(6), 1360–1380. https://doi.org/10.1086/225469
- Granovetter, M. (1978). Threshold models of collective behavior. The American Journal of Sociology, 83(6), 1420–1443. https://doi.org/10.1086/226707
- Grinberg, N., Joseph, K., Friedland, L., Swire-Thompson, B., & Lazer, D. (2019). Fake news on Twitter during the 2016 U.S. presidential election. Science, 363(6425), 374–378. https://doi.org/10.1126/science.aau2706
- Guo, L., & Vargo, C. (2018). Fake news and emerging online media ecosystem: An integrated intermedia agenda-setting analysis of the 2016 U.S. Presidential election. Communications Research, 47(2), 178–200. https://doi.org/10.1177/0093650218777177
- Hansen, M. (1999). The search-transfer problem: The role of weak ties in sharing knowledge across organization subunits. Administrative Science Quarterly, 44(1), 82–111. https://doi.org/10.2307/2667032
- Hinz, O., Skiera, B., Barrot, C., & Becker, J. (2011). Seeding strategies for viral marketing: An empirical comparison. Journal of Marketing, 75(6), 55–71. https://doi.org/10.1509/jm.10.0088
- Jackson, K., & Bazeley, P. (2019). Qualitative data analysis with NVivo. SAGE Publications.
- Kahn, R., & Kellner, D. (2004). New media and internet activism: From the ‘Battle of Seattle’ to blogging. New Media & Society, 6(1), 87–95. https://doi.org/10.1177/1461444804039908
- Kane, G. C., Alavi, M., LaBlanca, G., & Borgatti, S. P. (2014). What’s different about social media networks? A framework and research agenda. MIS Quarterly, 38(1), 275–304. https://doi.org/10.25300/MISQ/2014/38.1.13
- Krackhardt, D. (1990). Assessing the political landscape: Structure, cognition, and power in organizations. Administrative Science Quarterly, 35(2), 342–369. https://doi.org/10.2307/2393394
- Krackhardt, D., & Kilduff, M. (1999). Whether close or far: social distance effects on perceived balance in friendship networks. Journal of Personality & Social Psychology, 76(5), 770–782. https://doi.org/10.1037/0022-3514.76.5.770
- Lua, A. 2019. 21 Top social media sites to consider for your brand. Buffer Marketing Library. https://buffer.com/library/social-media-sites
- Maiz, A., Arranz, N., & de Arroyabe, J. (2016). Factors affecting social interaction on social network sites: The Facebook case. Journal of Enterprise Information Systems, 29(5), 630–649. researchgate.net/publication/307981423_Factors_affecting_social_interaction_on_social_network_sites_the_Facebook_case
- Major, A. M. (2000). Norm origin and development in cyberspace: Models of cybernorm evolution. Washington University Law Quarterly, 78(1), 59–111. https://openscholarship.wustl.edu/law_lawreview/vol78/iss1/2/
- Malone, T., Laubacher, R., & Dellarocas, C. (2010). The collective intelligence genome. MIT Sloan Management Review, 51(3), 21–31. sloanreview.mit.edu/article/the-collective-intelligence-genome/
- Marineau, J. E., Labianca, G., Borgatti, S. P., & Brass, D. J. (2018). Individuals’ formal power and their social network accuracy. Social Networks, 54, 145–161. https://doi.org/10.1016/j.socnet.2018.01.006
- Mayhew, B., & Levinger, R. (1976). Size and the Density of Interaction in Human Aggregates. American Journal of Sociology, 82(1), 86–110. https://doi.org/10.1086/226271
- McCombie, S., Uhlmann, A., & Morrison, S. (2020). The US 2016 presidential election and Russia’s troll farms. Intelligence and National Security, 35(1), 95–114. https://doi.org/10.1080/02684527.2019.1673940
- McCombs, M. E. (2004). Setting the agenda: The mass media and public opinion. Polity Press.
- McPherson, J. M., Smith-Lovin, L., & Cook, J. M. (2001). Birds of a feather: Homophily in social networks. Annual Review of Sociology, 27(1), 415–444. https://doi.org/10.1146/annurev.soc.27.1.415
- Mueller, R. 2019. Report on The Investigation into Russian Interference in the 2016 Presidential Election. U.S. Department of Justice. https://www.justice.gov/storage/report.pdf
- Naroditskiy, V., Jennings, N., Van Hentenryck, P., & Cebrian, M. (2014). Crowdsourcing contest dilemma. Journal of the Royal Society Interface, 11. http://dx.doi.org/10.1098/rsif.2014.0532
- Nass, C., & Moon, Y. (2000). Machines and mindlessness: Social responses to computers. Journal of Social Issues, 56(1), 81–103. https://doi.org/10.1111/0022-4537.00153
- Nass, C., Moon, Y., & Carney, P. (1999). Are people polite to computers? Responses to computer-based interviewing systems. Journal of Applied Social Psychology, 29(5), 1093–1110. https://doi.org/10.1111/j.1559-1816.1999.tb00142.x
- Nass, C., Moon, Y., & Fogg, B. (1995). Can computer personalities be human personalities? International Journal of Human-Computer Studies, 43(2), 223–239. https://doi.org/10.1006/ijhc.1995.1042
- National Archives. 2022. Electoral College. https://www.archives.gov/electoral-college/faq
- Netto, S., Sobral, M., & Soares, G. (2020). Concepts and forms of greenwashing: A systemic review. Environmental Science Europe, 32, 1. enveurope.springeropen.com/articles/10.1186/s12302-020-0300-3
- Noyes, D. 2019. Top 10 Twitter Statistics. Zephoria Digital Marketing. https://zephoria.com/twitter-statistics-top-ten/
- Oh, W., & Jeon, S. (2007). Membership herding and network stability in the open source community: The ising perspective. Management Science, 53(7), 1086–1101. https://doi.org/10.1287/mnsc.1060.0623
- Oliver, C. (1991). Strategic responses to institutional processes. Academy of Management Review, 16(1), 145–179. https://doi.org/10.2307/258610
- Pollet, T. V., Robert, S. G., & Dunbar, R. I. (2011). Use of social network sites and instant messaging does not lead to increased offline social network size, or to emotionally closer relationships with offline network members. Cyberpsychology, Behavior, and Social Networking, 14(4), 253–258. https://doi.org/10.1089/cyber.2010.0161
- Polyakova, A. 2019. Want to know what’s next in Russian election interference? Pay attention to Ukraine’s elections. BROOKINGS. https://www.brookings.edu/blog/order-from-chaos/2019/03/28/want-to-know-whats-next-in-russian-election-interference-pay-attention-to-ukraines-elections/
- Reeves, B., & Nass, C. (1996). The media equation: How people treat computers, television, and new media like real people and places. Cambridge University Press.
- Ren, Y., Kraut, R., & Kiseler, S. (2007). Applying common identity and bond theory to design of online communities. Organization Studies, 28(3), 377–408. https://doi.org/10.1177/0170840607076007
- Rogers, E. (2003). Diffusion of innovations. Free Press.
- Rowley, T. J. (1997). Moving beyond dyadic ties: A network theory of stakeholder influences. Academy of Management Review, 22(4), 887–910. https://doi.org/10.5465/amr.1997.9711022107
- Salge, C., & Karahanna, E. (2018). Protesting corruption on Twitter: Is it a bot or is it a person. Academy of Management Discoveries, 4(1), 32–49. https://doi.org/10.5465/amd.2015.0121
- Shirky, C. (2011). The political power of social media. Foreign Affairs, 90(1), 28. https://www.jstor.org/stable/25800379
- Smith, K. 2019. 53 Incredible Facebook statistics and facts. Brandwatch https://www.brandwatch.com/blog/facebook-statistics/
- Smith, L., & Sorensen, P. (2000). Pathological outcomes of observational learning. Econometrica, 68(2), 371–398. https://doi.org/10.1111/1468-0262.00113
- Suchman, M. (1995). Managing legitimacy: Strategic and institutional approaches. Academy of Management Review, 20(3), 571–610. https://doi.org/10.2307/258788
- Sunstein, C. (2001). Republic.com. Princeton University Press.
- Wasserman, S., & Faust, K. (1994). Social network analysis: Methods & application. Cambridge University Press.
- Watts, D. (2002). A simple model of global cascades on random networks. Proceedings of the National Academy of Sciences of the United States of America, 99(9), 5766–5771. https://doi.org/10.1073/pnas.082090499
- Watts, D. J. (2004). Six degrees: The science of a connected age. WW Norton & Company.
- Watts, D., Duncan, J., & Dodds, P. (2007). Influentials, networks, and public opinion information. Journal of Consumer Research, 34(4), 441–458. https://doi.org/10.1086/518527
- Welch, I. (1992). Sequential sales, learning and cascades. Journal of Finance, 47(3), 695–732. https://doi.org/10.1111/j.1540-6261.1992.tb04406.x
- Wu, Y., Zhang, K., & Xie, J. (2020). Bad greenwashing, good greenwashing: Corporate social responsibility and information transparency. Management Science, 66(7), 3095–3112. https://doi.org/10.1287/mnsc.2019.3340
- Yin, R.K. (2009). Case Study Research Design and Methods. Sage.