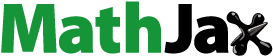
Abstract
Cameroon’s semi-arid zone (northern and far northern regions) is an important part of the local ecosystem that is vulnerable to climate change. This vulnerability raises concerns about rural households’ well-being. Adapting to this vulnerability could mitigate some of the negative effects, agricultural shocks, and catastrophic risks associated with agricultural ecosystem destruction. Thus, the goal of this paper is to assess the impact of climate change adaptation strategies on rural households’ sorghum productivity in Cameroon’s semi-arid zone. On 721 households’ secondary data, we used the endogenous switching regression model and the matching method. The results show that adopting adaptation strategies increases sorghum productivity in adapted households by approximately 21,99% more than in non-adapted households. In the absence of coping strategies, these households will lose 11,22% of their sorghum productivity. The importance of these findings adds to the debate about the need to implement climate change adaptation programmes and policies that address the concerns of all households.
1. Introduction
Several studies have identified agriculture as the sector most vulnerable to climate change (Faostat,). The Intergovernmental Panel on Climate Change (IPCC) states in its Sixth Assessment Report that climate trends have had a negative impact on agricultural production in several regions around the world (IPCC, Citation2001). Furthermore, even minor temperature increases have a negative impact on agricultural production (Bandara & Cai, Citation2014; Challinor et al., Citation2014a; Morton, Citation2007; Sarker et al.,). Although some authors (Di Falco et al., Citation2011; Reid et al., Citation2007; Tingem et al.,) highlight appropriate adaptation strategies to maximise future benefits from climate change, it is critical to evaluate the impact of adaptation strategies on grain productivity.
The agricultural sector in Sahelian countries is highly vulnerable to the effects of climate change due to its exposure to extreme weather events (drought and floods), poor production environment (rudimentary tools, small areas under cultivation, lack of agricultural inputs), low adaptive capacity, high social vulnerability of communities, lack of knowledge and resources (lack of training and financial means), and thus preventing rural households from adopting adaptation measures (Adger et al., Citation2003; Aryal et al., Citation2014, Citation2016; Fatemi et al., Citation2017; Huq et al., Citation2004; Islam et al., Citation2016; Morton, Citation2007; Nelson et al., Citation2010). Because agriculture is the primary source of livelihood, adaptation to climate hazards is critical to ensuring food security (Bryan et al., Citation2009; IPCC & Pachauri, Citation2014). Thus, adaptation to climate change refers to the modification of natural or human systems in response to actual or anticipated climatic stimuli or their effects in order to mitigate harm or capitalise on advantageous opportunities (IPCC, Citation2001).
Adaptation is also defined as the modification of agronomic practises, farming processes, and capital investments in response to observed or predicted climate threats (Easterling et al., Citation1994). Households adapt to these climatic hazards by changing the agricultural calendar, growing different crop species and varieties, implementing soil and water conservation practises, diversifying activities, and installing an irrigation system (Asseng & Pannell, Citation2013; Devkota et al., Citation2017; Niles et al., Citation2016; Zhang et al., Citation2015). To deal with so-called climate hazards, some people rely on local knowledge or local people’s knowledge based on observations and interpretation of natural phenomena (the height of an ant nest at the foot of a tree and the colour of frogs; Molua, Citation2006).
Agriculture generates an annual average of 22% of Cameroon’s GDP, accounts for more than 55% of trade, provides more than 50% of raw materials for industries, and is the primary source of income and employment for the active population (Faostat,). According to the National Institute of Statistics (Citation2015), approximately 90% of household land in Cameroon is less than 2 hectares. Despite the agricultural sector’s social importance, sorghum productivity in the semi-arid zone remains low (1006.9 kg/ha), compared to the national average of 1269.5 kg/ha (INS, 2015). Furthermore, the National Observatory on Climate Change (ONACC) report (Citation2019) identifies constraints to the development of this sector, such as rising temperatures, a decrease in the number of rainy days, poor spatial distribution of rainfall, and an increase in extreme weather events (high winds, sandstorms, tornadoes, etc.). Furthermore, research conducted in 2015 as part of the national adaptation to climate change (Minepded, Citation2015) shows that average rainfall from 1981 to 2000 was 20 to 40% lower than from 1961 to 1980. Since 1960, there has been a − 2.2% per decade decrease in rainfall (i.e., about 3 mm less per month) and a shortening of the rainy season in the country. In recent years, there has been an overall rise in temperature. Rapid rates of increase of 0.19° per decade are recorded in March, April, and May.
The situation in the semi-arid zone (900 mm of rainfall per year) is more concerning (decrease in rainfall) than in other parts of the country (1500 to 2000 mm per year). In this zone, temperatures are also rising (between 0.3 and 0.5°C per year) compared to other parts of the country (0.024°C per year; Koguia et al., Citation2021; UNDP, Citation2008). Unsurprisingly, projections show that future climatic conditions in Cameroon’s semi-arid zone will be even more devastating, with even greater effects on agricultural production (ONACC, 2019). Future climatic conditions in Cameroon’s semi-arid zone are expected to be even more devastating, with even greater impacts on agricultural production (ONACC, 2019). Furthermore, forecasts indicate an increase in temperature of +0.7° C by 2025; +1.2° C in 2035; +2.5° C in 2055; +3.6° C in 2075 and +4.8° C in 2100 (Csc, Citation2013; CSC AND Wur, Citation2013; UNDP, Citation2008). Similarly, the Onacc (National Observatory on Climate Change), Citation2019a; Onacc (National Observatory on Climate Change), Citation2019b) indicates an annual decline in rainfall of 8 mm by 2030, 20 mm by 2060 and 25 mm by 2090. Already, these constraints amplify annual cereal productivity losses of around 0.27 t/ha for maize, 0.03 t/ha for millet and sorghum, 0.07 t/ha for groundnuts, and 0.05 t/ha for rice (ONACC, Citation2019a). They also increase food insecurity, which is 22% in the semi-arid zone versus 10.5% in the rest of the country, with a prevalence rate of 33% in the zone (Faostat,). In other words, the average annual costs of the zone’s flagship crops are estimated to be 1,332,600,440 FCFA for groundnuts, 2,672,164,142 FCFA for maize, 265,762,840 FCFA for onions, 256,183,704 FCFA for rice, and 2,694,465,560 FCFA for millet/sorghum, as shown in the various figures below (Figures ).
Although theoretical and empirical studies on the relationship between climate change adaptation strategies and agricultural yields in vulnerable areas are increasing, they are mostly limited to developing countries on the American and Asian continents (Khanal et al., Citation2018a; Khanal et al., Citation2018b; Finger et al., Citation2011; Quan et al., Citation2020b; Rosenzweig & Parry, Citation1994). Others have looked into the cases of some African countries, but they have only looked into Ethiopia, Ghana, Nigeria, and Mali (Diallo et al., Citation2020b; Di Falco et al., Citation2011; Onyeneke, Citation2019). Cameroon studies are almost non-existent (Tingem et al.,). Most studies in Cameroon examine the impact of climate change (Molua, Citation2006, p. Citation2007) or people’s perceptions of climate change (Koguia et al., Citation2021). Work on assessing the impact of adaptation strategies on productivity has only focused on a holistic analysis of adaptation. They also did not combine methods (Endogeneous Switching Regression Model and Propensity Score Matching) to achieve robust results. Khanal et al. (Citation2018a) and Diallo et al. (Citation2020b), on the other hand, consider strategy types in their analyses.
As a result, the goal is to assess the impact of climate change adaptation strategies on sorghum productivity of rural households in Cameroon’s semi-arid zone. Furthermore, the study intends to investigate the effect of adaptation on sorghum productivity. The study intends to contribute to the climate literature by increasing empirical evidence on farm households’ knowledge of climate change, as well as the impact of adopted strategies on sorghum productivity. Its findings complement those of previous studies conducted in the African context to examine the impact of climate change adaptation on agricultural productivity and food security (Dessalegn et al., Citation2022; Diallo et al., Citation2020b; Di Falco et al., Citation2011; Onyeneke, Citation2019). The study’s main findings show that adaptation increases sorghum productivity in adapted households by about 21.99% more than in non-adapted households. The importance of these findings adds to the debate about the need to implement climate change adaptation programmes and policies that address the concerns of all households. Following the introduction, the second section presents the methodological analysis, the third section presents the results and discussions, and the final section concludes.
2. Methodology analysis
To achieve the aforementioned goal, the methodology presented in this work will revolve around the data source, variable measurement, and model specification.
2.1. Study area
Cameroon is affectionately known as Africa in miniature because its agro-ecological diversity reflects Sub-Saharan (African) reality. Its poverty rate of 54% is 24 percentage points higher than the average for Sub-Saharan Africa (Ins, Citation2017). The country is divided into four broad agro-ecological zones: the southern rainforests (East, Centre, Littoral, and South-West regions), the western highlands, the central savannah (southern part of the North region and Adamawa), and the semi-arid zone (Far North and North region) (onacc, Citation2019a). Agriculture is based on peasants, particularly cereal cultivation (onacc, Citation2019b).
The North and Far North regions of the semi-arid zone were chosen because of their history and experience in cereal cultivation (sorghum, maize, millet, rice, etc.). This zone is located in northern Cameroon. It is bounded to the north by Chad, to the west by Nigeria, and to the east by the Central African Republic (CAR). This area, subdivided into ten departments, covers 100,350 km2 or 21% of the country’s surface area. Its population is estimated to be around 5,530,643 people, accounting for 29% of the total population of the country (BUCREP, Citation2010). The area has two distinct seasons: the rainy season, which lasts from June to August, and the dry season, which lasts from September to March as shown in on Agro-ecological Zoning of the Northern and Far North Regions in Cameroon.
2.2. Data sources
Data from a cross-sectional survey of 721 farmers in Cameroon’s North and Far North regions (semi-arid zone) were used in this study. These data were collected as part of the Institute of Agricultural Research for Development’s (IRAD- C2D) agrosystems project in the North and Far North, which was piloted in 2016. This open-ended questionnaire survey asks about adaptation strategies, socioeconomic and demographic characteristics of households, agricultural production structure, socio-institutional environment, and farmers’ perceptions of climate change. The goal of this survey is to design, evaluate, and disseminate efficient and sustainable agrosystems in rural areas of northern and far northern Cameroon. This goal allows us to contribute to the long-term improvement of polyculture agricultural productivity by proposing adapted and efficient techniques to agricultural development structures. Based on the agro-ecological zoning of Dugué et al, (Dugué & Harmand, Citation1994), the survey considered physical criteria (climate and soils), demographic criteria (population density and movement), potentials and constraints, agricultural conditions, crops in the area, and economic criteria (market presence and isolation). Despite the fact that this technique identified seven villages, the survey only included four due to the security situation surrounding the “Boko Haram” problem. In each of the four reference locations, 800 farmers were sampled (Mowo, Siriawe, Zera, and Sanguere). At the end of the survey, the questionnaire rejection rate (incorrectly filled in, doubtful responses, non-farming households) was 7.5%, 10.5%, 17%, and 4.5% for Mowo, Siriawe, Zera, and Sanguere, respectively. This rejection represented a negligible proportion of the sample of 79 farmers (or 9.87%). Thus, the data comes from all four zones, with 25.66% for Mowo, 24.83% for Siriawe, 24% for Zera, and 25.5% for Sanguere.
2.3. Measurement of climate change adaptation variables and productivity
Economists are increasingly using the concept of adaptation to climate change to study the reactions of economic agents to these changes. According to the IPCC (Citation2001), adaptation is the modification of natural or human systems in response to current or anticipated changes in weather (or their effects), in order to mitigate negative consequences and capitalise on opportunities. Finnigan (Citation2009) contends that community adaptation to climate change necessitates what he refers to as a “culture of change”, i.e., a new approach based on environmental considerations in any manufacturing process. This modification is regarded as a social innovation (Bergmann et al., Citation2011) or an alternative method of dealing with the negative effects of climate change on agriculture (Mendelsohn et al., ; Onoja et al., Onoja, Citation2019).
Agricultural households, societies, and economies have adapted and continue to adapt, in various ways and with varying degrees of success, to changes in their environment and climate variability. Regardless of the increased intensity and/or the emergence of new climatic constraints, households are accustomed to dealing with a certain level of climate risk, for which they employ the available means of adjustment. Indeed, 89% of households in the area have adopted at least one strategy to deal with climate change. Diversification of activities (76.97%), early sowing (58.39%), intensification of plant protection (58.66%), crop association (57.69%), and field irrigation (56.31%) are the most widely used strategies in the zone. As a result, the variable adaptation to climate change is binary, worth one if the household has adopted an adaptation strategy and zero otherwise.
Ellis (Citation2000) defines activity diversification as the process by which rural households build an ever-expanding portfolio of diverse activities and assets in order to survive and improve their standard of living. According to Niehof (Citation2004), diversification is an important strategy for reducing survival vulnerability. As a result, extensive livestock rearing is used as an intra-household diversifier. At this scale, livestock farming allows for the use of uncultivated land, the utilisation of crop byproducts, and income diversification. Many farms’ survival cannot be explained on this basis alone. The operation of a rural society necessitates the performance of various artisanal, forestry, commercial, or other activities. Furthermore, diversification through the valorisation of animal waste leads to soil fertilisation for increased productivity.
In response to declining sorghum yields and new climatic conditions, households are incorporating new sorghum varieties into their cropping systems. The Institute of Agricultural Research for Development (IRAD) and non-governmental agricultural organisations are introducing these new varieties to farmers. This variety makes households less vulnerable to pockets of drought and shorter rainy seasons. This method involves planting at the beginning of the rainy season to promote faster germination and increased productivity.
Furthermore, in order to limit the impact of weeds, with which they are increasingly confronted, households have increased their use of plant protection products. Climate change, according to Kate et al. (Citation2014), causes changes in soil characteristics such as organic reserve, nutrients and acidity, redox conditions, and water and physical characteristics. Thus, the use of phytosanitary products improves soil fertility, resulting in long-term productivity.
Households rely on rainwater from catchment areas to irrigate their crops. Climate change, on the other hand, is affecting the hydrological regime, making the land drier and stressing many crops. As a result, some households are opting for irrigation to increase water availability on cultivation plots. Furthermore, the majority of households report using this method for off-season sorghum and vegetable production. All of this implies that there will be plenty of water available not only for plant growth but also for increased production.
Crop association is the practise of combining varieties grown in the same area. The varieties must coexist for a significant period of their growth, even if they are not necessarily sown and harvested at the same time. This technique reduces soil depletion, strengthens plant natural defences, and conserves water.
In terms of productivity, the questionnaire on the structure of production collects data on the quantities produced as well as the location of agricultural households. This productivity is the ratio of each household’s quantity produced in kilogrammes (kg) to its area in hectares (ha). This corresponds to the theoretical view that land productivity is defined as the ratio of annual production quantity to total area of the production unit. The latter differs from both labour productivity, which measures the efficiency of labour used in the production process, and capital productivity.
Several crops are also grown in the semi-arid zone. Sorghum, maize, groundnuts, rice, onions, sesame, soya, fonio, potatoes, and other grains are examples. Sorghum is one of the most widely grown crops in the region (69.1%). It is used in the production of the local beer (Bili Bili). Brewing companies value it as well.
2.4. Specification of the econometric model related to the adoption of climate change adaptation strategies
A variety of impact assessment methods are available in the literature:
The random assignment method is frequently used in impact evaluation studies on both large and small scales. The disadvantage of this method is that it is primarily based on a random draw.
The discontinuous regression method begins by defining an index to determine which individuals or households are or are not eligible for treatment. The limitation of this method is that the average local impact is estimated only around the eligibility threshold, i.e., at the point where the treatment group and the comparison group are most similar. Another limitation is that this method does not allow for the calculation of the average effect, which may be advantageous or disadvantageous depending on the circumstances.
The double-difference method (Yorobe et al., Citation2011) compares differences in outcomes over time between populations that participate in a programme (treatment group) and those that do not (comparison or control group). It is necessary for the treatment and control groups to have the same assessment of the trend in outcomes. It has the advantage of being simple and easy to use, and it addresses non-random observations. The limitation of this method is that, even if the trends between groups are equivalent, it may be biassed, and participants must be pre-identified in the baseline data.
The Propensity Score Matching method assumes that the only differences between the treated and untreated populations are the individuals’ individual characteristics and the treatment. It is not necessary for any treatment or control group to have unobservable characteristics (Mendola, Citation2007). Its disadvantage is that if there are unobservable characteristics, it may be biassed.
As a result, the Endogeneous Switching Regression Model, which considers both observed and unobserved factors when estimating the impact of adaptation on productivity, is a more appropriate method (Di Falco et al., Citation2011; Khanal et al., Citation2018a; Khanal et al., Citation2018b). The regime-switching model can be used to address issues of selection bias. This model is widely used to assess the impact of adaptive practises on crop production across multiple agro-ecological zones (Lokshin & Sajaia, Citation2004; Di Falco et al., Citation2011; khanal et al., Citation2018; Onyeneke, Citation2019; Diallo et al., Citation2020b).
The advantage of this method over the other four is that it can reduce the problem of bias introduced by other methods, despite their effectiveness in terms of statistical analysis robustness. It does not necessitate the expenditure of baseline data or randomization. Given this characteristic difference, and drawing on recent work (Coromaldi et al., Citation2015; Di Falco & Veronesi, Citation2020b; Di Falco et al., Citation2011; Kassie et al., Citation2011; Abdulai & Huffman, Citation2014, Khanal et al., Citation2018a; Citation2018b), this work assesses the robustness of results using the two-stage endogenous switching regression model and propensity score matching.
First, because Cameroon’s semi-arid zone is extremely vulnerable to climatic shocks, a farm household makes a “dichotomous” (yes or no) decision to implement an adaptation strategy if it believes it will allow it to maximise its future agricultural yield. This dichotomous choice (yes and no) corresponds to a yield function (Y) that can be written as:
As this adoption of coping strategies (Ai) is a visible manifestation of the unobservable latent variable (Ai) of the household, the problem is modelled in terms of a function of return (Y) of the farm household. For a farm household i with characteristic Zi, the adoption of coping strategies is conditional on a certain expected return Y (1, Zi), while the refusal to adopt coping strategies is conditional on an expected return Y (0, Zi). We have:
A= 1 if Y (1, Zi)> Y (0, Zi) Or A = 0 if Y (1, Z)< Y (0, Z).
The farm household selects the situation that allows it to maximise its future returns based on the theory of profit (production). This brings us back to the latent variable, which captures the benefits of choosing whether or not to implement adaptation strategies. Thus, we have:
Where Zi = (1, zi1, zi2, zi3, …,zik) is the vector of explanatory variables,α the vector of parameters to be estimated,ε i the realization of random events distributed according to a normal distribution. The adoption of adaptation strategies (A =1 or A*> 0) can be influenced by current climate factors, perception of climate change, access to finance, access to information on climate change, access to agricultural extension, household and farm characteristics.
3. Endogeneous switching regression model (ESR)
The impact of adaptation strategies on agricultural yield is then mobilised in the second step. The simplest way to examine the impact of coping strategies is to include a dummy variable (A) equal to 1 if the household used these strategies in the yield equation. Then use Ordinary Least Squares to solve the problem (OLS). However, because it assumes that this adaptation is exogenously determined when it is potentially endogenous, this method may produce biassed estimates. Individual household self-selection may influence whether or not a strategy is implemented. Households that use them may exhibit systematically different characteristics than those that do not. Furthermore, farm households may have chosen the strategies based on expected returns. Unobservable characteristics of the household head and the household may influence both the adoption decision and farm yield, resulting in inconsistent estimates of the effect of adaptation on productivity. Thus, we estimate a simultaneous equation model of coping strategy adoption and farm yields with endogenous maximum likelihood switching to full information, taking into account the endogeneity of the decision to adopt coping strategies. In contrast to Quan et al. (Citation2020b), who use fitted values generated automatically by the selection model’s non-linearity to control for endogeneity, we use an exclusion restriction to identify this model (Lokshin & Sajaia, Citation2004; Abdulai & Huffman, Citation2014; Khanal et al., Citation2018a). This constraint is required when some variables directly affect the selection variable (adoption of coping strategies), but not the outcome variable approximated by productivity (Coromaldi et al., Citation2015).
As a result, we use variables related to climate change information (from radio, extension agents, and producer organisations) and perception of such changes as selection instruments for the agricultural yield function. After a sample homogeneity test and a falsification test, the admissibility of this instrument (perception of climate change) is effective (Di Falco et al., Citation2011). As a result, if the selection instrument is valid, it will influence the decision to implement adaptation strategies but not the amount of production (output) of households that have not implemented adaptation strategies. Furthermore, to account for selection bias, we use an endogenous switching regression model of agricultural yield in which households are given two options: (1) adopt coping strategies or (2) do not adopt them. Di Falco et al. (Citation2011); Abdulai and Huffman (Citation2014); and Khanal et al, (2015) defined the regression model (Citation2018a).
The regression model is defined as in Di Falco et al.
Where represents the quantity produced per hectare in Equationequations (2a)
(2a)
(2a) and (Equation2b
(2b)
(2b) ),
represents the vector of inputs and the characteristics of the head of household, those of the farm household and climatic factors.
and
are the vectors of the parameters to be estimated, the terms
and
of error in the selection and outcome equation are assumed to have a trivariate normal distribution, with zero mean and the covariance matrix
i.e.
(Di Falco et al., Citation2011; Khonje et al., Citation2015; Khanal et al., Citation2018a)
With
Following Di Falco et al. (Citation2011) and Khanal et al, (Citation2018a), the expected values of are non-zero, and given as:
According to Di Falco et al. (Citation2011), the endogenous switching regression model can be used to study four conditional expectations of productivity.
The expected sorghum productivity of adapting farm households versus non-adapting farm households is represented by ; 4b). EquationEquation (4c)(4c)
(4c) represents the expected sorghum productivity of adopting farm households if they had not adapted, whereas Equationequation (4d)
(4d)
(4d) represents non-adopting farm households if they had adapted. Furthermore, in accordance with Heckman and Vytlacil (), we calculate the effect of the “adapted” treatment on the (TT) treatment as the difference between Equationequations (4a)
(4a)
(4a) and (Equation4c
(4c)
(4c) ), which represents the effect of climate change adaptation on sorghum productivity of farm households that actually adapted to the changes.
Similarly, we calculate the effect of the treatment on untreated farm households (TUs) that did not actually adapt to climate change as the difference between (4d) and (4b).
More details on the endogenous switching regression model can be found in Lokshin and Sajaia (Citation2004); Di Falco et al.
4. Propensity score matching (PSM)
Propensity score matching (PSM) is used to assess the robustness of treatment effects because the results of endogenous switching regression (ESR) can be sensitive to the choice of instrumental variable assumptions. Where Y1 represents the return to households that have adopted coping strategies (A = 1) and Y2 represents the return to households that have not (A = 0). The outcome variable of households that adopted the coping strategies (E (Y| A = 1)) can be observed, but not the outcome variable of non-adopters if they had adopted them (Y2 | A = 1). As a result, the estimation of ATT using equation (7) may be biassed. SHP estimation linked to three assumptions (conditional independence, the presence of a common support, and the treatment unit value) allows for clearer treatment effects (Rosenbaum & Rubin, Citation1983; Fourgère, Fougère, Citation2010; Khandker et al., Citation2018b). The conditional independence assumption assumes that the conditional probability of the outcome variable in the absence of coping strategy adoption (Y2) and the status of their adoption (A) are statistically independent, and it defines the propensity score for coping strategy adoption as follows: A(X) = Pr(A = 1)|X.
Furthermore, if there are non-adopting households with characteristics similar to those of the adopters, this matching method can be used. Thus, the households that are compared have the same probability of adopting or not adopting coping strategies such that 0 < A(X)<1. The respect of these hypotheses leads to the specification of the estimator of the ATT by the SHP as follows:
ATT = E {Y|A = 1, A(X)}- E {Y|A = 0, A(X) (7)
The estimation of this equation is done in several steps: first, the probability of adopting coping strategies is estimated by a probit model that estimates the propensity scores for each household. Subsequently, each household that has adopted coping strategies is matched with a non-adopting household with a similar propensity score in order to estimate the ATT. Through this technique, SHP compares the difference between the outcome variables of adopting and non-adopting households with similar characteristics (Ngoucheme, Citation2018) and (Takam-Fongang et al., Citation2019).
4.1. Descriptive statistics
Table presents descriptive statistics on the socioeconomic and demographic characteristics of the households, some of which show a significant difference in adaptation to climate change. According to the data in this table, approximately 88.9% of the 721 farm households have implemented at least one adaptation strategy, while 11.1% have not. There are several types of adaptation constraints. For example, 13.86% of adapted households have access to finance, whereas 14.19% of non-adapted households do not. Furthermore, 21.49%, 28.57%, and 23.43% of adapted households have access to climate information via radio, extension agents, and producer organisations, respectively, compared to 22.3%, 31.04%, and 22.15% of non-adapted households. Furthermore, climate change is perceived by 99.21% of the adapted compared to 93.75% of the non-adapted.
Table 1. Definition and descriptive statistics of variables
In terms of climate variability, 53% of households reported an increase in temperature, including 49% of adapted households and 90% of non-adapted households. Flooding was also mentioned by 20% of them, including 19% of the adapted and 27% of the non-adapted. In addition, 86% reported a significant decrease in the number of rainy days (92% of adapted and 38% of non-adapted). Despite this widespread belief among the unadapted, access to finance and ancestral beliefs (culture) continue to be major barriers to adaptation.
The average sorghum productivity in farming households in the semi-arid zone is 1107.9 kg/ha. Thus, adapted households have a productivity (sorghum) of 1058.73 kg/ha, compared to non-adapted households’ productivity of 803.85 kg/ha. This implies that the strategies will have an immediate positive impact on sorghum productivity. Furthermore, households have an average of 2.62 hectares of land to cultivate. Furthermore, men dominated the surveyed households (89%), with 91.73% adapted and 75% non-adapted, compared to 11% women (8.27% adapted and 25% non-adapted). This depiction of men in the agricultural sphere in Cameroon’s semi-arid zone corresponds to the legal (cultural) view that only male household heads have the right to own land. Furthermore, more than half (62%) are educated, with the majority (33%) having a primary level, of which 32.29% are adapted and 40% are not, and a minority (16%) having a secondary level, of which 25.58% are adapted and 30% are not. Similarly, the majority of respondents (54%) were between 30 and 50 years old, with 55.07% adapting and 46.25% not adapting; a minority under 30 years old (16%), with 15.13% adapting and 26.25% not adapting; and an average over 50 years old (30%), with 29.79% adapting and 27.5% not adapting (Table ).
5. Results
The literature has identified climate change perception and access to climate information as potential instrumental variables. Following the falsification test, the perception of climate change is retained as a valid instrumental variable. The falsification test determines whether these variables (perception of climate change and access to climate information) have an indirect effect on sorghum productivity through their effects on adaptation. To that end, the sorghum productivity of non-adapted households was regressed on these variables (perception of climate change and access to climate information) as well as all other variables in the model.
The lack of significance of the climate change perception variable suggests that it has no direct impact on sorghum productivity, in contrast to the access to climate information variable. As a result, perception of climate change is a valid instrumental variable.
5.1. Endogenous switching model (ESR) result
As previously stated, the average productivity of sorghum in the semi-arid zone is 1107.9 kg/ha, which is lower than the average productivity of sorghum in Cameroon, which is 1261.72 kg/ha (INS, 2014). The majority of crop plots are rainfed, which could explain the low productivity. Climate change adapters’ sorghum productivity (1455.32 kg/ha) is significantly higher than that of non-adapters (407.58 kg/ha). This finding is consistent with other studies that conclude that adaptation to climate change improves sorghum productivity in Mali, Nepal, and Nigeria (Di Falco et al., Citation2012; Zhou & Turvey, Citation2014; Khanal et al., Citation2018a; Onyeneke, Citation2019; Diallo et al., Citation2020b; Dessalegn et al., Citation2022). This method, however, does not account for selection bias.
Table depicts sorghum productivity predictions under real and fictitious conditions. Cells (a) and (b) show the observed sorghum productivity in the sample (b). The adapted sorghum productivity is estimated to be 1455.32 kg/ha, compared to 407.58 kg/ha for the non-adapted. This simple comparison, however, can be deceptive, leading the researchers to conclude that the adapted produced 1047.74 kg/ha more than the non-adapted.
Table 2. Average expected sorghum productivity per hectare; treatment effects
According to the analysis by type of coping strategy (Table ), sorghum productivity is approximately 1162.49 kg per hectare for farm households that have diversified their activities, compared to 485.02 kg per hectare for those that have not. Farming households that diversified produced approximately 667.47 kg per hectare, or 139.67% more than those that did not. These findings are consistent with those of Onyeneke (Citation2019), Diallo et al. (Citation2020b), and Khanal et al, (Citation2018b), who discovered that households that diversified produced 7% more than those that did not.
Planting early seed yielded 1157.15 kg per hectare in comparison to 272.12 kg per hectare in farm households that did not. Households that planted early varieties produced approximately 885.02 kg per hectare more than those that did not. Due to the area’s lack of rainfall, households are forced to plant varieties with short germination times. These findings support those of Onyeneke (Citation2019) in Nigeria and Diallo et al. (Citation2020b) in southern Mali, who found that using improved short-cycle seeds not only increased agricultural productivity but also effectively combated rainfall variations.
Table final column displays the average effects of adaptation on sorghum productivity. These treatment effects account for the selection bias caused by the likelihood that adapted and non-adapted individuals differ systematically (Abdulai & Huffman, Citation2014). Cell (c) represents the adapted’s predicted sorghum productivity per hectare if they did not adapt, and cell (d) represents the non-predicted adapted’s sorghum productivity per hectare if they did adapt.
Adapted households produced 1000, 406 kg/ha (or 21.99%) less than non-adapted households. In addition, if households had adapted, they would have produced 457.538 kg/ha (or 11.22%) more. These findings imply that (Table ) adaptation to climate change boosts sorghum productivity significantly. These findings support those of Di Falco et al. (Citation2011), who estimate that if farmers do not adapt, they will lose 20% of their production. Furthermore, farmers would have produced 35% more if they had adapted to climate change. In Nepal, Khanal et al. (Citation2018a) finds comparable results. Onyeneke (Citation2019) and Diallo et al. (Citation2020b) do so in Nigeria and Mali.
Furthermore, in terms of the analysis by type of coping strategy, farm households that diversified their activities produced approximately 1136.14 kg less than they would have if they had not done so. Furthermore, farm households that did not diversify would have produced 909.20 kg/ha (or 187.45%) more. Households who planted early seed produced approximately 961.31 kg/ha less than those who did not. Furthermore, farm households who did not plant early varieties would have produced 1098.08 kg/ha (or 403.52%) more. Khanal et al., (Citation2018b) and Onyeneke, Citation2019) find comparable results in Nepal and Nigeria.
Many other studies, most notably Onyeneke (Citation2019); Diallo et al. (Citation2020b); Dessalegn et al. These findings imply that climate change adaptation strategies significantly increase sorghum productivity. These findings support those of Di Falco et al. (Citation2011), who estimate a 20% loss in production for adapted households if they do not adapt. Furthermore, if non-adapted households had adapted to climate change, they would have produced 35% more. In Nepal, Khanal et al (Citation2018a) discover comparable results. Onyeneke (Citation2019) and Diallo et al. (Citation2020b) do in Nigeria and Mali.
5.2. Robustness of the results using the propensity score matching (PSM) method
According to the nearest neighbour method, the results of the impact of climate change adaptation on sorghum productivity estimated by the propensity score matching method (Table ) show that adaptation is positive and significant at 5% of sorghum productivity (3 neighbours). It is also positively correlated at 1% sorghum productivity, according to the Kernel method. Adapted farm households increased productivity by 586.69 kg/ha and 845.3 kg/ha, or 55.41% and 79.84%, respectively. These findings support the findings of Di Falco et al. (Citation2011), Khanal et al (Citation2018a), Onyeneke (Citation2019), and Diallo et al. (Citation2020b), who discovered that adaptation increases agricultural productivity in Ethiopia, Nepal, and Mali, respectively.
Table 3. ATT by propensity score matching method
In terms of activity diversification, the results show that regardless of the methods used, this practise is positive and significant at 10% of farm household sorghum productivity (nearest neighbour and kernel). Farming households that diversified their activities increased their productivity by 3,801.39 kg/ha and 2,042.01 kg/ha, respectively, 79.02% and 42.44% more than farming households that did not diversify.
According to the nearest neighbour method, they are positive and significant at 5% of sorghum productivity for households that planted improved sorghum grains early (3 neighbours). According to the Kernel method, it is also positively correlated at 10% of sorghum productivity. Farm households that planted early increased their sorghum productivity by 3771.63 kg/ha and 1065.45 kg/ha, respectively, by 78.11% and 22.06%. These findings are consistent with those of Khanal et al (Citation2018a), Onyeneke (Citation2019), and Diallo et al. (Citation2020b), who show that diversification of activities and adoption of improved seeds significantly increases crop productivity (cassava, sorghum, etc.) in Nepal, Nigeria, and Mali, respectively.
The propensity score matching (PSM) method demonstrated that farming households in the semi-arid zone benefit from climate change adaptation strategies. The selection assumption on observables is, however, a limitation of this method. The ESR model has a greater impact on the adoption of climate change adaptation strategies than the SHP model. The negative sign indicates that unobservable variables that increase farm household sorghum productivity are correlated with unobservable variables that reduce climate change adaptation. As a result, low-productivity farm households are more likely to adapt to climate change. In this case, failing to account for them results in an underestimation of the impact of adaptation, which explains the larger effect observed with the ESR model.
6. Conclusion
In this study, the ESR and PSM models were used to compare sorghum productivity between climate change-adapted and non-adapted farm households in Cameroon’s semi-arid zone. The ESR and PSM models revealed that adaptation strategies (activity diversification and semi-early planting) increased sorghum productivity. The estimated impact using the ESR and PSM approaches ranged from 457.53 to 1000.40 kg/ha and from 597.41 to 721.36 kg/ha, respectively. Although the estimated impact differs slightly between the two approaches, it is worth noting that adapted households would have lost 1000.40 kg/ha if they had decided not to adapt, while non-adapted households would have gained 457.53 kg/ha if they had decided to adapt, according to the ESR estimate. As a result, diversification of activities and the use of early semis improve sorghum productivity significantly in adapted farm households. These findings are critical for policymakers developing effective policies to address the potential effects of climate change. Furthermore, while adaptation strategies can increase sorghum yields, they can be expensive to implement and conflict with other societal and environmental goals. As a result, public policies must assist rural households in effectively implementing adaptation strategies (e.g., access to climate information, information and training seminars, etc.). Furthermore, public authorities, through their extension services, should make research results more accessible (improved inputs, new agricultural practices, etc.).
Data availability
The data used in the preparation of this document are available.
Ethical approval
All precautions related to scientific ethics have been taken into account from the conception to the writing of this paper, as well as the submission to the said journal.
Disclosure statement
No potential conflict of interest was reported by the author(s).
Additional information
Funding
Notes on contributors
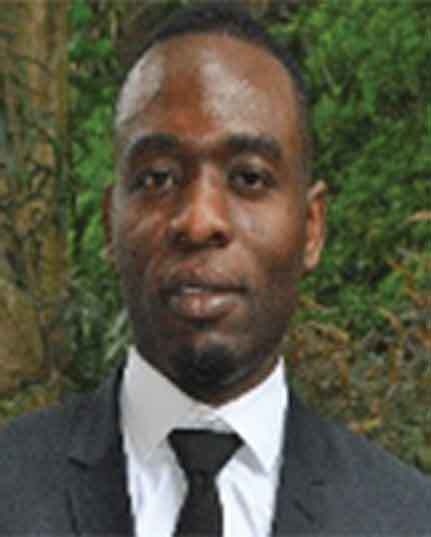
Nelson Sergeo Tagang Tene
Nelson Sergeo Tagang Tene is a rural and environmental economist at the Centre d’Étude et de Recherche en Économie et Gestion (CEREG-Cameroon) and the Centre de Recherche en Économie Appliquée (CREEA) (CREA-Senegal). His doctoral research examines the impact of climate change adaptation strategies on farm sustainability in Cameroon’s Sahelian zone. He has received awards such as the 2019 Erasmus Mundus intra-Africa mobility grant from the African Cooperation of International Mobility Programs (CAPITUM), as well as an MPhil scholarship from the Inter-University Postgraduate Programme in Economics (IUPCE) from 2016 to 2017. He is an expert in environmental economics and development economics. His research interests are in rural and environmental economics, with a focus on farm sustainability, agricultural innovation, climate change adaptation, productivity, information and communication technology (ICT), rural youth entrepreneurship, food security, impact assessment, gender, and poverty analysis. He has several publications in international peer-reviewed scientific journals, including the Cahier du CEDIMES, the University of Born’s collective work on the relationship between climate change and food security in West Africa. He contributes to survey reports, scientific reports, conference reports, and book chapters on a regular basis.
References
- Abdulai, A., & Huffman, W. (2014). The adoption and impact of soil and water conservation technology: An endogenous switching regression application. Land Economics, 90(1), 26–23. https://doi.org/10.3368/le.90.1.26
- Adger, W. N., Huq, S., Brown, K., Conway, D., & Hulme, M. (2003). Adaptation to climate change in the developing world. Progress in Development Studies, 3(3), 179–195. https://doi.org/10.1191/1464993403ps060oa
- Aryal, A., Brunton, D., & Raubenheimer, D. (2014). Impact of climate change on human-wildlife-ecosystem interactions in the Trans-Himalaya region of Nepal. Theoretical and Applied Climatology, 115(3–4), 517–529. https://doi.org/10.1007/s00704-013-0902-4
- Aryal, A., Shrestha, U. B., Ji, W., Ale, S. B., Shrestha, S., Ingty, T., Raubenheimer, D., Cockfield, G., & Raubenheimer, D. (2016). Predicting the distributions of predator (snow leopard) and prey (blue sheep) under climate change in the Himalayas. Ecology and Evolution, 6(12), 4065–4075. https://doi.org/10.1002/ece3.2196
- Asseng, S., & Pannell, D. J. (2013). Adapting dryland agriculture to climate change: Farming implications and research and development needs in Western Australia. Climatic Change, 118(2), 167–181. https://doi.org/10.1007/s10584-012-0623-1
- Bandara, J. S., & Cai, Y. (2014). The impact of climate change on food crop productivity, food prices and food security in South Asia. Economic Analysis and Policy, 44(4), 451–465. https://doi.org/10.1016/j.eap.2014.09.005
- Bergmann, G. T., Bates, S. T., Eilers, K. G., Lauber, C. L., Caporaso, J. G., Walters, W. A., Fierer, N., & Fierer, N. (2011). The under-recognized dominance of Verrucomicrobia in soil bacterial communities. Soil Biology and Biochemistry, 43(7), 1450–1455. https://doi.org/10.1016/j.soilbio.2011.03.012
- Bryan, E., Deressa, T. T., Gbetibouo, G. A., & Ringler, C. (2009). Adaptation to climate change in Ethiopia and South Africa: Options and constraints. Environmental Science & Policy, 12(4), 413–426. https://doi.org/10.1016/j.envsci.2008.11.002
- BUCREP. (2010). Yaoundé: Bureau Central des Recesements et des Etudes de Population. Population du Cameroun En 2010.
- Challinor, A. J., Watson, J., Lobell, D. B., Howden, S., Smith, D., & Chhetri, N. (2014a). A meta-analysis of crop yield under climate change and adaptation. Nature Climate Change, 4(4), 287. https://doi.org/10.1038/nclimate2153
- Coromaldi, M., Pallante, G., & Savastano, S. (2015). Adoption of modern varieties, farmers’ welfare and crop biodiversity: Evidence from Uganda. Ecological Economics, 119, 346–358. https://doi.org/10.1016/j.ecolecon.2015.09.004
- Csc. (2013): Climate Change Scenarios for the Congo Basin. [A. Haensler, D. Jacob, P. Kabat, & F. Ludwig (eds.)]. Climate Service Centre Report No. 11 21924058. 21924058.
- Dessalegn, B., Asnake, W., Tigabie, A., & Le, Q. B. (2022). Challenges to the adoption of improved legume varieties: A gender perspective. Sustainability, 14(4), 2150. https://doi.org/10.3390/su14042150
- Devkota, R. P., Pandey, V. P., Bhattarai, U., Shrestha, H., Adhikari, S., & Dulal, K. N. (2017). Climate change and adaptation strategies in Budhi Gandaki River Basin, Nepal: A perception-based analysis. Climatic Change, 140(2), 195–208. https://doi.org/10.1007/s10584-016-1836-5
- Diallo, A., Donkor, E., & Owusu, V. (2020b). Climate change adaptation strategies, productivity and sustainable food security in southern Mali. Climatic Change, 1–19. https://doi.org/10.1007/s10584-020-02684-8
- Di Falco, S., & Veronesi, M. (2013). How can African agriculture adapt to climate change? A counterfactual analysis from Ethiopia. Land Economics, 89(4), 743–766. https://doi.org/10.3368/le.89.4.743
- Di Falco, S., Veronesi, M., & Yesuf, M. (2011). Does adaptation to climate change provide food security? A micro-perspective from Ethiopia. American Journal of Agricultural Economics, 93(3), 829–846. https://doi.org/10.1093/ajae/aar006
- Di Falco, S., Yesuf, M., Kohlin, G., & Ringler, C. (2012). Estimating the impact of climate change on agriculture in low-income countries: Household level evidence from the Nile Basin, Ethiopia. Environmental and Resource Economics, 52(4), 457–478. https://doi.org/10.1007/s10640-011-9538-y
- Dugué, P., & Harmand, J. M. (1994). Projet Garoua. Report on a mission to Chad. Programme Gestion de Terroir de la Station de Bebedja (4 to 9 July 1994). https://agritrop.cirad.fr/312489/
- Easterling, W. E., Aggarwal, P. K., Batima, P., Brander, K. M., Erda, L., Howden, S. M., & Schmidhuber, J. (2007). Food, fibre and forest products. Climate Change, 273–313.
- Ellis, F. (2000). Rural livelihoods and diversity in developing countries. Oxford university press.
- Faostat, F. (2017). https://www.fao.org/faostat/en/#data. (accessed January 2018).
- Fatemi, F., Ardalan, A., Aguirre, B., Mansouri, N., & Mohammadfam, I. (2017). Social vulnerability indicators in disasters: Findings from a systematic review. International Journal of Disaster Risk Reduction, 22, 219–227. https://doi.org/10.1016/j.ijdrr.2016.09.006
- Finger, R., Hediger, W., & Schmid, S. (2011). Irrigation as adaptation strategy to climate change-a biophysical and economic appraisal for Swiss maize production. Climatic Change, 105(3–4), 509–528. https://doi.org/10.1007/s10584-010-9931-5
- Finnigan, J. (2009). Response to comment by Dr. AS Kowalski on “The storage term in eddy flux calculations”. Agricultural and Forest Meteorology, 149(3–4), 725–729. https://doi.org/10.1016/j.agrformet.2008.10.012
- Fougère, D. (2010). Econometric evaluation methods. Revue française des affaires sociales, (1), 105–128. https://doi.org/10.3917/rfas.101.0105
- Heckman, J. J., & Vytlacil, E. (2001). Policy-relevant treatment effects. American Economic Review, 91(2), 107–111. https://doi.org/10.1257/aer.91.2.107
- Huq, S., Reid, H., Konate, M., Rahman, A., Sokona, Y., & Crick, F. (2004). Mainstreaming adaptation to climate change in least developed countries (LDCs). Climate Policy, 4(1), 25–43. https://doi.org/10.1080/14693062.2004.9685508
- Ins. (2017). Yaoundé: Institut national de la statistique. 2013
- Institut National de la Statistique du Cameroun (INS), Décembre 2015.Quatrième Enquête Camerounaise Auprès des Ménages (ECAM-4) : Tendances, profil et déterminants de la pauvreté au Cameroun entre 2001-2014.
- IPCC, B. (2001). Climate Change: The Scientific Basis. Contribution of Working Group I to the Third Assessment Report of the Intergovernmental Panel on Climate Change. IPCC Working Group I Report, Cambridge.
- IPCC. (2021). Appendix. IPCC projections. In Climate (p. 149–153). EDP Sciences
- IPCC, R., & Pachauri, A. R. (2014). Climate Change 2014: IPCC.
- Islam, S., Cenacchi, N., Sulser, T. B., Gbegbelegbe, S., Hareau, G., Kleinwechter, U., Robinson, S., Nedumaran, S., Robertson, R., Robinson, S., & Wiebe, K. (2016). Structural approaches to modeling the impact of climate change and adaptation technologies on crop yields and food security. Global Food Security, 10, 63–70. https://doi.org/10.1016/j.gfs.2016.08.003
- Kassie, M., Shiferaw, B., & Muricho, G. (2011). Agricultural technology, crop income, and poverty alleviation in Uganda. World Development, 39(10), 1784–1795. https://doi.org/10.1016/j.worlddev.2011.04.023
- Kate, S., Dagbenonbakin, G., Agbangba, C., de Souza, J., Kpagbin, G., Azontondé, A., Ogouwalé, E., Tinté, B., & Sinsin, B. (2014). Perceptions locales de la manifestation des changements climatiques et mesures d’adaptation dans la gestion de la fertilité des sols dans la Commune de Banikoara au Nord-Bénin. Journal of Applied Biosciences, 82(1), 7418–7435. https://doi.org/10.4314/jab.v82i1.11
- Khanal, U., Wilson, C., Hoang, V.-N., & Lee, B. (2018a). Farmers’ adaptation to climate change, its determinants and impacts on rice yield in Nepal. Ecological Economics, 144, 139–147. https://doi.org/10.1016/j.ecolecon.2017.08.006
- Khanal, U., Wilson, C., Lee, B. L., & Hoang, V.-N. (2018b). Climate change adaptation strategies and food productivity in Nepal: A counterfactual analysis. Climatic Change, 148(4), 575–590. https://doi.org/10.1007/s10584-018-2214-2
- Khandker, S., Koolwal, G. B., & Samad, H. (2009). Handbook on impact evaluation: Quantitative methods and practices. The World Bank.
- Khonje, M., Manda, J., Alene, A. D., & Kassie, M. (2015). Analysis of adoption and impacts of improved maize varieties in eastern Zambia. World Development, 66, 695–706. https://doi.org/10.1016/j.worlddev.2014.09.008
- Koguia, W. N., Nonga, F. N., Madi, A., Leblois, A., & Laure, M. T. G. (2021). Perception of Climatic Change and Farmers’ Decision to Adapt in the Sudano-Sahelian Zone in Cameroon. Journal of Humanities and Social Sciences Studies, 3(10), 22–33. https://doi.org/10.32996/jhsss.2021.3.10.3
- Lokshin, M., & Sajaia, Z. (2004). Maximum likelihood estimation of endogenous switching regression models. The Stata Journal, 4(3), 282–289. https://doi.org/10.1177/1536867X0400400306
- Mendelsohn, R., Dinar, A., & Williams, L. (2006). The distributional impact of climate change on rich and poor countries. Environment and Development Economics, 11(2), 159–178. https://doi.org/10.1017/S1355770X05002755
- Mendola, M. (2007). Agricultural technology adoption and poverty reduction: A propensity-score matching analysis for rural Bangladesh. Food Policy, 32(3), 372–393. https://doi.org/10.1016/j.foodpol.2006.07.003
- Minepded, G. O. (2015). (Intended) Nationally Determined Contribution: Minepded Yaounde.
- Minister, P. (2009). Growth and Employment Strategy Paper. Yaounde: Republic of Cameroon, 113.
- Molua, E. L. (2006). Climatic trends in Cameroon: Implications for agricultural management. Climate Research, 30(3), 255–262. https://doi.org/10.3354/cr030255
- Molua, E. L. (2007). The economic impact of climate change on agriculture in Cameroon. World Bank Policy Research Working Paper, (4364).
- Morton, T. (2007). Ecology without nature: Rethinking environmental aesthetics. Harvard University Press.
- Nelson, R., Kokic, P., Crimp, S., Martin, P., Meinke, H., Howden, S. M., Nidumulu, U., & de Voil, P. (2010). The vulnerability of Australian rural communities to climate variability and change: Part II - Integrating impacts with adaptive capacity. Environmental Science and Policy, 13(1), 18–27. https://doi.org/10.1016/j.envsci.2009.09.007
- Ngoucheme, R. (2018). the effects of certification on the performance of cocoa-based agroforestry systems in Cameroon. [ PhD thesis in Economics], University of Dschang.
- Niehof, A. (2004). The significance of diversification for rural livelihood systems. Food Policy, 29(4), 321–338. https://doi.org/10.1016/j.foodpol.2004.07.009
- Niles, M. T., Brown, M., & Dynes, R. (2016). Farmer’s intended and actual adoption of climate change mitigation and adaptation strategies. Climatic Change, 135(2), 277–295. https://doi.org/10.1007/s10584-015-1558-0
- Onacc (National Observatory on Climate Change). (2019a). Rainfall and temperature in the East Cameroon region: Analysis of the evolution from 1950. 2015 and projections to 2090. Republic of Cameroon.
- Onacc (National Observatory on Climate Change). (2019b). Rainfall and temperature in the Central Cameroon region: Analysis of the evolution from 1950. 2015 and projections until L’horizon 2090. Republic of Cameroon.
- Onoja, A. O. (2019). 4 Economic benefits from cassava in peri-urban multiple-cropping systems in Nigeria. Multifunctional Land Uses in Africa, 61. https://library.oapen.org/bitstream/handle/20.500.12657/24946/9780367246440_text.pdf?sequence=1#page=77
- Onyeneke, R. U. (2020b). Does climate change adaptation lead to increased productivity of rice production? Lessons from Ebonyi State, Nigeria. Renewable Agriculture and Food Systems, 1–15. https://doi.org/10.1017/S1742170519000486
- Quan, S., Li, Y., Song, J., Zhang, T., & Wang, M. (2019). Adaptation to Climate Change and its Impacts on Wheat Yield: Perspective of Farmers in Henan of China. Sustainability, 11(7), 1928. https://doi.org/10.3390/su11071928
- Reid, S., Smit, B., Caldwell, W., & Belliveau, S. (2007). Vulnerability and adaptation to climate risks in Ontario agriculture. Mitigation and Adaptation Strategies for Global Change, 12(4), 609–637. https://doi.org/10.1007/s11027-006-9051-8
- Rosenbaum, P. R., & Rubin, D. B. (1983). Reducing bias in observational studies using subclassification on the propensity score. Journal of the American Statistical Association, 79(387), 516–524. https://doi.org/10.1080/01621459.1984.10478078
- Rosenzweig, C., & Parry, M. L. (1994). Potential impact of climate change on world food supply. Nature, 367(6459), 133. https://doi.org/10.1038/367133a0
- Sarker, M. A. R., Alam, K., & Gow, J. (2014). Assessing the effects of climate change on rice yields: An econometric investigation using Bangladeshi panel data. Economic Analysis and Policy, 44(4), 405–416. https://doi.org/10.1016/j.eap.2014.11.004
- Takam-Fongang, G. M., Kamdem, C. B., & Kane, G. Q. (2019). Adoption and impact of improved maize varieties on maize yields: Evidence from central Cameroon. Review of Development Economics, 23(1), 172–188. https://doi.org/10.1111/rode.12561
- Tingem, M., Rivington, M., Bellocchi, G., Azam-Ali, S., & Colls, J. (2008). Comparative assessment of crop cultivar and sowing dates as adaptation choice for crop production in response to climate change in Cameroon. The African Journal of Plant Science and Biotechnology, 2(1), 10–17.
- UNDP. (2008). Social responsibility, corruption and sustainable human development in Benin UNDP National Report 2007-2008
- Wur, C. (2013). Country Fact Sheets. Climate change scenarios in the Congo Basin. Fact Sheet - Hydrology and Energy - Republic of Cameroon - Zone 2. Final report 67p
- Yorobe, J., Jr, Rejesus, R., & Hammig, M. (2011). Insecticide use impacts of integrated pest management (IPM) farmer field schools: Evidence from onion farmers in the Philippines. Agricultural Systems, 104(7), 580–587. https://doi.org/10.1016/j.agsy.2011.05.001
- Zhang, H.-L., Zhao, X., Yin, X.-G., Liu, S.-L., Xue, J.-F., Wang, M., & Chen, F. (2015). Challenges and adaptations of farming to climate change in the North China Plain. Climatic Change, 129(1–2), 213–224. https://doi.org/10.1007/s10584-015-1337-y
- Zhou, L., & Turvey, C. G. (2014). Climate change, adaptation and China’s grain production. China Economic Review, 28, 72–89. https://doi.org/10.1016/j.chieco.2014.01.001
Appendices
Table shows the effect of adaptation on farming households’ sorghum productivity, and Table shows the probit model propensity scores. The difference test before and after matching is shown in Tables and A4. The quality indicator before and after matching is shown in Table .
Figures A1 and A2 show the standardised bias (sorghum) before and after matching, as well as the distribution of propensity scores and common support (sorghum).
Figure A1. Standardised bias before and after matching and the distribution of propensity scores and common support (sorghum).
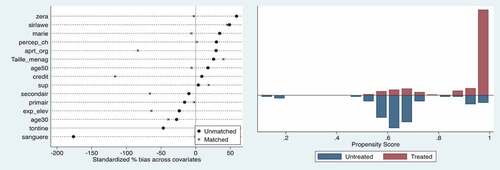
Table A1. Results of the impact of adaptation on sorghum productivity of farm households
Table A2. Propensity scores by probit model (sorghum)
Table A3. Pre-match difference test (sorghum)
Table A4. Difference test after matching (sorghum)
Table A5. Quality indicators before and after matching (sorghum)