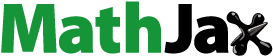
Abstract
The current study revisited social capital and knowledge sharing processes in virtual communities among university students. The conceptual framework was developed in the form of a hierarchical component model. Two dimensions of social capital (relational and cognitive) as second-order variables reflected sequencing relationships among social capital, outcome expectations, knowledge sharing, and learning performance. Social capital theory was used in support of the study’s conceptual framework. The target populations were Vietnamese and Bangladeshi students at Vietnam National University Ho Chi Minh City and Comilla University, respectively. The sample included a total of 465 participants recruited through purposive and quota sampling in the two target populations. We used partial least squares structural equation modelling (PLS-SEM) to estimate the hierarchical component model. Our findings suggest that the cognitive dimension of social capital, and outcome expectations (i.e., personal and community-related) had significantly positive impacts on knowledge sharing behaviour; and subsequently that knowledge sharing behaviour had a significantly positive impact on learning performance. Moreover, a multi-group analysis was conducted to deliver new insights when making comparisons among different sub-samples. The study provides theoretical contributions to the fields of social capital and knowledge sharing through delivering related best practices among university students in virtual communities, and outlines promising directions for further research.
1. Introduction
One of the most recent breakthroughs in information technology has enabled people easy access to the internet, thereby allowing them to virtually contact to people across the globe. Virtual communities are created by individual participation and interaction with others, alongside knowledge sharing and exchange. The 4.0 era has made virtual community a main platform for knowledge sharing where people can post, comment, discuss and give advice and recommendations to gain their common interests. By integrating from several previous studies, Liang et al. (Citation2016) indicated that virtual communities that are regarded as an innovation for organizational knowledge sharing satisfy members’ needs of knowledge sharing and information exchange. Originally, virtual community is defined as a place that connects people with others to interact and communicate through the technology (Romm et al., Citation1997). Moreover, the concept of virtual community is proposed by Chiu et al. (Citation2006) as a social network that people with common interests can share their knowledge and experiences by involving social interactions. Various studies have explored and investigated the effects of virtual communities in different activities, such as in economics, marketing, education, communities of practice in organisations (Nguyen et al., Citation2006), and professional virtual communities (Chang & Chuang, Citation2011; Chiu et al., Citation2006; Yli-Renko et al., Citation2001). However, Chiu et al. (Citation2006) observed that the amount of mutual knowledge is limited as the results that people are not willing or not confident to share their own experiences. People tend to share things less than what they really have. Determining motivations for knowledge sharing in virtual communities is necessary for the improvement of social assets.
Social capital is defined as the collective benefits embedded in the networking which are generated by the social interaction and stimulate the cooperation among individuals to achieve mutual goals (Bourdieu, Citation2011). The collective benefits are closely related to the relationship of “mutual acquaintance and recognition” (Bourdieu, Citation2011) because it can gather individuals in the community to collaborate and cooperate for common goals (Putnam, Citation1995). Various social capital constructs are presented in an offline environment (Nahapiet & Ghoshal, Citation1998; Singh et al., Citation2021; Lefebvre et al., Citation2016; Sheng & Hartono, Citation2015) and recently applied in online environment to determine how social capital facilitates the information exchange process (Chiu et al., Citation2006; Chang & Chuang, Citation2011). Although there are several empirical research studying the concept social capital by various measurements, variables that are applied to measure the social capital take in form of unidimensional. Therefore, a need for improved measurement is required in virtual community. The work of Meek et al. (Citation2019) has applied the multidimensional variables in online brand community, including shared vision, shared language, a norm of reciprocity and social trust. In this study, the approach is re-used to determine social capital’s effects on the knowledge sharing process in a multidimensional form.
Under virtual community, the social capital theory is used to determine the close relation between social capital and knowledge sharing, which explains how the process of knowledge sharing is facilitated by the aspects of social capital (Zhang et al., Citation2017; Chiu et al., Citation2006; Chang & Chuang, Citation2011; Yan et al., Citation2019). In the trend of online learning, the need for increased student interaction in online classes is enforced to improve students’ learning performance because there is a lack of interactivity among students (Strang, Citation2011). Eid and Al-Jabri (Citation2016) suggested that social network sites are places where students can communicate and interact with their peers for learning purposes. It found that knowledge sharing on social network sites gives better outcome learning, which social media (i.e., Whatsapp, Facebook and Instagram) facilitate the lesson-related discussion among students and promote learning performance.
Chiu et al. (Citation2006) and Chang and Chuang (Citation2011) used social capital theory to explain how social capital, individual motivations, and outcome expectations induce knowledge sharing behaviour in virtual communities. Subsequently, Eid and Al-Jabri (Citation2016) argued how the use of social network tools and knowledge sharing among students can enhance their learning performance. Building on previous research, the current study revisits the social capital and knowledge sharing processes of students in tertiary education. Our aim is to develop a second-order factor model for observing knowledge sharing processes with three distinct dimensions of social capital.
This study makes substantial contribution to social capital and knowledge sharing. It explores social capital and its abstract sub-dimensions (e.g., relational, in terms of trust, reciprocity, and identification; and cognitive, regarding shared language and shared vision) in the measurement framework. Second-order variables and a hierarchical component model were used to build the conceptual framework. The conceptual framework reflects the mechanism of knowledge sharing processes with social capital, outcome expectations, and personal involvement for improving the learning performance of tertiary education students.
2. Literature review
2.1. Virtual communities
Schaefer (Citation2022) stated that a group composed of various people who share similar norms, values, and expectations can expect to socially interact on a regular basis. There are two group types: primary and secondary. Primary groups are characterised by their small sizes, greater levels of intimacy, and face-to-face interactions. Meanwhile, secondary groups are characterised by their large sizes, formal relationships, little social intimacy, and mutual understanding.
A virtual community is a social network without limitations of space and time; in which members not only mutually interact and communicate due to their common interests and characteristics, but also create long-term relationships by sharing contents to maintain a sustainable virtual community (Chang & Chuang, Citation2011).
2.2. Knowledge sharing processes
Knowledge-created from the accumulation of information, experience, and theory encompasses both explicit knowledge (i.e., tangible knowledge delivered through systematic and structured processes) and implicit knowledge (i.e., intangible knowledge shared through impersonal means; Chang & Chuang, Citation2011).
Knowledge sharing processes are undertaken to exchange information, skills, and expertise among members of a certain community/organisation in order to maximise the effectiveness and benefits attached to such individuals or groups (Bukowitz & Williams, Citation2000; Grant, Citation1996). Communication tools for virtual communities are used within knowledge sharing processes to perform social networking free from time and space constraints (Chang & Chuang, Citation2011; Chiu et al., Citation2006; Eid & Al-Jabri, Citation2016).
Charband and Jafari Navimipour (Citation2018) systematically reviewed knowledge sharing in education using three concrete mechanisms (the motivation/forces of knowledge sharing, using tools for knowledge sharing, and innovation/high performance resulting from it). The authors identified social capital as a specific enabler of knowledge sharing. Eid and Al-Jabri (Citation2016) applied social cognitive theory to explain the sequencing relationships among social networking sites (e.g., online discussions, content creation, file sharing, etc.), knowledge sharing, and learning performance of tertiary students. Indeed, Saudi students used different social networking tools (e.g., WhatsApp, YouTube, and Facebook) in knowledge sharing processes in order to improve their study outcomes.
2.3. Social capital and knowledge sharing behaviour
Bourdieu (Citation2011) defined social capital as the collective benefits created not only by social networks and reciprocal trust, but also from mutual acquaintance and recognition. Indeed, social capital can induce coordination and cooperation among community members so as to obtain mutual benefits (Putnam, Citation1995). Adopting Bourdieu (Citation2011) and Putnam (Citation1995), Nahapiet and Ghoshal (Citation1998, p. 243) defined social capital as “the sum of actual and potential resources embedded within, available through, and derived from the network of relationships possessed by an individual or social unit”.
Moreover, Nahapiet and Ghoshal (Citation1998) introduced three distinct dimensions of social capital: the structural dimension (i.e., the overall pattern of relationships and social interaction ties between community members), the relational dimension (i.e., the nature of the connections between individuals reflected through trust, norms, obligations, expectations, and identification), and the cognitive dimension (i.e., resources in the forms of a shared language and vision which offers the same group representation, interpretation, and meaning system among members).
Various studies have used social capital theory to explain how social capital embedded in a social network induces knowledge sharing processes. For instance, Tsai and Ghoshal (Citation1998) showed how social capital stimulates resource exchange and production innovation in organisations, Yli-Renko et al. (Citation2001) demonstrated the impacts of social capital and knowledge acquisition on exploitation in young technology-based factories, and Chang and Chuang (Citation2011) and Wasko and Faraj (Citation2005) noted social capital’s effects on knowledge sharing and contribution.
Nahapiet and Ghoshal (Citation1998) suggests that social interaction is an aspect of organizational structure because its role is gathering individuals in an organization. Recalling Nahapiet and Ghoshal (Citation1998), Meek et al. (Citation2019) suggested that social interaction is hierarchical in an organization because people use social interaction ties to develop their profile and career prospect. However, the hierarchy does not exist in virtual communities, community members have the same position so they do not need to develop their social relationships with others, as Meek et al. (Citation2019) discussed in online brand community. Although time-consuming, social interaction are symbolic of the strength and frequency of social relationships between members of a virtual community (Chiu et al., Citation2006). Social interaction ties in virtual communities allow individuals to access, share, and exchange knowledge (Chang & Chuang, Citation2011). Yli-Renko et al. (Citation2001) argued that, the larger the number of social interactions, the greater the intensity, frequency, and breadth of knowledge and experience that is exchanged. Consequently, social capital in the form of social interaction ties has a significant impact on knowledge sharing behaviour.
As such, we propose the following hypothesis:
H1: The structural dimension of social capital has a significantly positive impact on knowledge sharing behaviour.
Members of a virtual community with similar accepted values, norms, and principles (i.e., inter-personal trust) are more likely to engage in social exchanges, cooperative interactions, and knowledge sharing (Chiu et al., Citation2006, as cited in Chang & Chuang, Citation2011). Trust can facilitate the knowledge sharing when people perceive the shared information is important and reliable (Jones & Taylor, Citation2012). Virtual community includes many individuals who don’t know each other in reality and face some difficulties in maintaining the relationships (Lin et al., Citation2020). People can contribute knowledge sharing aggressively when they feel comfortable to share their own experiences. Hence, the trust has the ability to create the friendly environment for the improvement of knowledge sharing.
Reciprocity refers to a strong sense of fairness in the way knowledge exchanges are perceived as mutual by members of a virtual community (Chiu et al., Citation2006). Members are encouraged and motivated to contribute more of their own knowledge if this has been reciprocated by the community in the past (Chang & Chuang, Citation2011). Moghavvemi et al. (Citation2017) argued that due to limited human resources (i.e., time, energy, and knowledge), people are willing to share their own resources only if they perceived their contribution is worth. Therefore, knowledge sharing happened continuously or not depends on the reciprocity as it impacts the expectations of future returns between members in the community (Meek et al., Citation2019; Nahapiet & Ghoshal, Citation1998).
Identification represents an individual’s willingness to be a part of a virtual community, as well as their own positive feelings towards it. For instance, individuals with their own valuable knowledge have an intention to hoard it unless they are recognised as group members by others in the community, or their knowledge contribution meets their needs or interests. The perception of social unity and togetherness of the community enhances an individual’s motivation to share their knowledge, and facilitate the depth and breadth of shared knowledge (Chiu et al., Citation2006).
Accordingly, we advance the following:
H2: The relational dimension of social capital has a significantly positive impact on knowledge sharing behaviour.
A shared language can enhance group understanding given the fact that each member can communicate and share ideas among others with similar backgrounds/practical experiences (Chang & Chuang, Citation2011; Chiu et al., Citation2006). Integrating from Nahapiet and Ghoshal (Citation1998), Chiu et al. (Citation2006) showed that “shared codes and language facilitate the common understanding of collective goals and the proper ways of acting in virtual communities” in which enables community members to get close relationships with others. Hence, shared languages can motivate people to engage in knowledge sharing and exchanges. In the context of Meek et al. (Citation2019) argued that the gap of members’ similarities can be mitigated by shared language because it provides the common communication ways for members to interact with each other. The higher interaction is, the more efficient the knowledge sharing is fostered.
A shared vision refers to “the collective goals and aspirations of the member in an organisation” (Tsai & Ghoshal, Citation1998, p. 467). Chiu et al. (Citation2006) stated that virtual communities are positive places wherein groups of people can be brought together through common interests and goals. Such interests and visions can motivate individuals within the virtual community to engage in knowledge sharing processes for their own purposes and achievements.
Based on the above, we formulated the following hypothesis:
H3: The cognitive dimension of social capital has a significantly positive impact on knowledge sharing behaviour.
2.4. Outcome expectations, participation involvement, and knowledge sharing behaviour
Chiu et al. (Citation2006) explained individual motivation to spend time and effort on sharing knowledge with other members of a virtual community. Their study was conducted in terms of personal recognition (i.e., personal outcome expectations) and community recognition (i.e., community-related outcome expectations). Indeed, individuals engage in knowledge sharing process for various reasons, such as knowledge enrichment and friendship (Andrews, Citation2002), reflecting individual talents (Butler et al., Citation2007), and knowledge accumulation and development (Bock & Kim, Citation2002; Kolekofski & Heminger, Citation2003; Lesser, Citation2000).
Chang and Chuang (Citation2011) argued that people are willing to participate in virtual community when they perceived that the more benefits are received, and suggested that the level of knowledge sharing depends on the level of individuals involvement. Hence, participation involvement can moderate the causal relationship between individual motivations and knowledge sharing behaviour.
We thus propose the following hypotheses:
H4: Personal outcome expectations have a significantly positive impact on knowledge sharing behaviour
H4a: Participation involvement significantly moderates the causal relationship between personal outcome expectations and knowledge sharing behaviour.
H5: Community-related outcome expectations have a significantly positive impact on knowledge sharing behaviour.
2.5. Knowledge sharing behaviour and learning performance
Knowledge sharing processes yield various benefits for both individuals and organisations. These include: expertise development, employee capabilities, more effective learning and performance, innovation, creativity, and optimised resource use (Ahmad & Karim, Citation2019; Henttonen et al., Citation2016; Masa’deh et al., Citation2016). Moreover, knowledge sharing processes and social network tools can help students improve their learning performance through online discussions and file sharing (Eid & Al-Jabri, Citation2016).
Accordingly, we propose our final hypothesis:
H6: Knowledge sharing behaviour has a significantly positive impact on learning performance.
3. The conceptual framework
Social capital theory has been used to explain the influences of social capital on knowledge sharing (Chang & Chuang, Citation2011; Wasko & Faraj, Citation2005). Outcome expectations (i.e., personal, and community-related) is proved to induce people to share their knowledge and join virtual communities (Chiu et al., Citation2006). Similarly, Wasko and Faraj (Citation2005) and Chang and Chuang (Citation2011) established a significantly causal relationship between individual motivations (i.e., reputation, the joy of helping/altruism) and knowledge sharing in order to explain people’s willingness to participate in both knowledge sharing and virtual communities. Interestingly, participation involvement plays a moderating role in the causal relationship between individual motivations and knowledge sharing (Chang & Chuang, Citation2011). Various previous studies have evidenced significantly positive impacts of knowledge sharing on individual performance, learning, and creativity levels (Ahmad & Karim, Citation2019; Eid & Al-Jabri, Citation2016; Henttonen et al., Citation2016; Masa’deh et al., Citation2016).
As well as to investigate sequencing relationships among social capital, knowledge sharing behaviour, and learning performance alongside other intervening variables (e.g., personal and community outcome expectations, and participation involvement). In essence, social capital in tertiary education has been gradually established in such forms as social interaction ties, trust, reciprocity, identification, shared language, and shared visions within student communities. Remarkably, the structural social capital is considered as the outcome of relational and cognitive dimensions (Meek et al., Citation2019). Community members can strengthen their personal relationships by having trust and reciprocity, and simultaneously developing shared language and shared vision. Other aspects of knowledge sharing behaviours relate to community and personal outcome expectations, with participation involvement throughout the entire process. Regarding community-related outcome expectations, students gain mutual benefits by sharing knowledge, such as achieving community goals and building up the knowledge sharing base of their virtual communities. In terms of personal outcome expectations, knowledge sharing benefits students by granting them greater recognition and respect, and strengthening community cooperation. Lastly, knowledge sharing processes help students improve their learning performance.
The figure below introduces the conceptual framework in the form of a hierarchical component model with two second-order variables (the relational and cognitive dimensions of social capital).
4. Methodology
4.1. Data collection
This research included two target populations. The first was comprised of current students at the Vietnam National University Ho Chi Minh City (VNU-HCM), which is a higher education institution providing graduates for Vietnam’s (and its southern regions in particular) socio-economic development. It is formed of eight member universities offering courses in science and social science, technology and engineering, economics and law, and the environment and sustainability, among other fields. We approached two of the VNU-HCM’s eight member universities in VNU-HCM, namely the University of Technology (with a capacity of 20,000 students), and the International University (comprised of 10,000 students). The second population was current students at Comilla University (Bangladesh)—a renowned public university affiliated with the University Grants Commission—which has as its central mission the production of knowledgeable, enlightened, creative, and competent graduates. It is home to approximately 10,000 students.
The mass survey was conducted in 2021 by students in VNU-HCM (Vietnam) and Comilla University (Bangladesh) recruited through the non-probability sampling approach (i.e., convenient sampling, snowball sampling, quota sampling).
We developed the measurement scale shown in Appendix 1 from previous studies. A total of 51 items (listed in Appendix 1) were used to measure the constructs and test the hypotheses detailed above. There were two question types in the survey: demographic information, and a questionnaire answered on a five-point Likert scale. The main survey for Vietnamese students was translated into Vietnamese from the original English, and adjusted into a Vietnamese context. The main survey for Bangladeshi students was conducted in English and adjusted to a Bangladeshi context.
Some studies have posited the weakness of random sampling (i.e., its impossibility and practical unfeasibility), and have thus instead supported the purposive strategy for collecting representative samples in target populations (e.g., Radević et al., Citation2021). Accordingly, we applied purposive and quota sampling approaches for recruiting our respondents (465 in total). The total sample was spread almost equally across the universities (i.e., each contributed approximately 33.3% of the total). As two of the universities were in Vietnam, our sample contained more Vietnamese students (70% approx.) than Bangladeshi ones (roughly 30%). The gender ratio was approximately equal (50% male and 50% female). The majority of the sample were studying Science & Engineering (55%), and 45% were reading Social Science & Art. Most of respondents were in their second, third, and fourth years of their bachelor’s degrees (almost 90%, see, Table ).
Table 1. Demographics of respondents
4.2. Assessment method
Theoretically, partial least squares structural equation modelling (PLS-SEM)—using “total variance to develop linear combinations of indicators to form composite variables that empirically represent the conceptual variables”—are an appropriate statistical method with which to estimate various casual relationships among latent variables in the complex conceptual framework, and to analyse small sample sizes (Hair & Sarstedt, Citation2019).
J. F. J. Hair et al. (Citation2019) criticised the abuse of “extremely small samples relative to the underlying population”. Instead of this, minimum sample size in PLS structural modelling should be calculated in terms of the number of items in a latent variable, statistical power, and probability of error (J. F. Hair et al., Citation2017). In this study, we calculated sample size using Yamane’s (Citation1967) formulation. With target populations of 40,000 students and an applied significant value of 5%, the sample size should be 396. Thus, collected data from 465 valid cases is sufficient for satisfying the requirements of minimum sample size.
Source: Yamane (Citation1967)
Note: n = sample size, N = population size, e = significant value.
The conceptual framework was built in the form of a hierarchical component model. The second-order constructs (i.e., the relational and cognitive dimensions of social capital) were established by first-order constructs (i.e., trust, reciprocity, and identification for the former, and shared language and vision for the latter). We applied reflective-reflective specification of higher-order constructs for building the hierarchical component model. Higher-order constructs support the reduction of path model linkages, resulting in model parsimoniousness (Sarstedt et al., Citation2019). The PLS algorithm (traditional/standard PLS) and bootstrapping have been recommended for estimating the hierarchical component model with the repeated indicator approach (Wong, Citation2019).
We adopted the repeated indicators approach to validate the higher-order constructs with reflective-reflective specification with critical considerations recommended by Sarstedt et al. (Citation2019) (e.g., the measurement model reflects the concrete relationships between the second-order variable and its lower-order components; the lower-order components are not counted in the structural model).
This study applied traditional/standard PLS to analyse the reflective model, as per the guidelines proposed by Cheah et al. (Citation2018). To assess the measurement model, we evaluated the indicator loading, construct reliability, and convergent validity by factor loadings (i.e., exceeding 0.708), composite reliability (CR, ranging between 0.7 to 0.95), and average variance extracted (AVE, exceeding 0.5), respectively. Additionally, discriminant validity was evaluated by the Heterotrait-Monotrait Ratio (HTMT) and Fornell-Larcker criteria (J. F. J. Hair et al., Citation2019). Recalling the work of Jöreskog (Citation1971), J. F. J. Hair et al. (Citation2019) recommend estimating construct reliability by CR rather than Cronbach’s alpha. Indeed, CR can weight the individual indicators based on their loadings, thereby overcoming limitation of Cronbach’s alpha.
We collected primary data from individual respondents in a single one-time survey. Accordingly, common method bias was a potential problem we had to consider, as it could have influenced the proposed impacts in the conceptual framework. With respect to Kock (Citation2017), all VIFs resulting from a full collinearity test were controlled (i.e., equal to or less than 3.3) to eliminate common method bias in the model.
To assess the structural model, we estimated the various causal relationships in the conceptual framework using path coefficients, coefficient of determination (R2/R-squared), and effect size (f2) (J. F. J. Hair et al., Citation2019). Indeed, the quality of the structural model was reflected through classification on R-squared values (i.e., small: 0.02; medium: 0.13; large: 0.26) (Wetzels et al., Citation2009), and classification on effect size (f2) values (i.e., no effect: less than 0.02; small: 0.02; medium: 0.15; large: 0.35) (Cohen, Citation1988, as cited in J. F. J. Hair et al., Citation2019).
We used SmartPLS v. 3.3.2 software to test all of our hypotheses in the conceptual framework (see, Figure ).
5. Results
5.1. Testing measurement model
Table shows that the reliability and convergent validity of all latent constructs in the reflective measurement model achieved all thresholds recommended by J. F. J. Hair et al. (Citation2019). It is vital for the process of item selection in the measurement model to reach acceptable ranges for indicator loading (i.e., factor loading) and Cronbach’s alpha. There were five deleted items in the reflective measurement model (SCS2, KSB6, KSB8, POE4, and POE11). Essentially, two second-order latent constructs measured by their first-order latent constructs in the measurement model achieved acceptable ranges of indicator loadings, construct reliability, and convergent validity (i.e., factor loadings, CR, and AVE, respectively).
Table 2. Reliability and convergent validity of latent constructs
In the PLS-SEM, discriminant validity reflecting “the extent to which a construct is distinct from other constructs” was assessed by the HTMT Ratio. Table shows the measurement model achieved discriminant validity of HTMT85 (i.e., a more conservative threshold value of 85, the construct are conceptually more distinct), as recommended by Henseler et al. (Citation2015), as cited in J. F. J. Hair et al. (Citation2019). In addition, Fornell-Larcker Criterion shows a direct comparison of the AVEs of two constructs to the shared variance between the two constructs” (J. F. J. Hair et al., Citation2019, p. 776) (see Table ).
Table 3. Discriminant validity (Fornell-Larcker Criterion)
Table 4. Discriminant validity (Heterotrait-Monotrait Ratio)
5.2. Testing structural model
Following Wetzels et al. (Citation2009), we found that the structural model had a larger effect with an R-squared value of 0.381 for learning performance, meaning that all of the explanatory variables in the structural model can explain 38.1% of learning performance variability. As such, the predictive power of our structural model was supported (see, Table ).
Table and Figure show that four of our hypotheses were supported, all of which had significantly positive causal relationships (H3, H4, H5, and H6), while two had to be rejected due to their insignificant causal relationships (H1 and H2).
Figure 2. Theoretical model estimation.
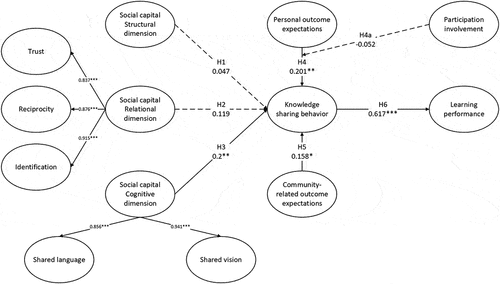
Table 5. Results of hypothesis testing
The cognitive dimension of social capital had a significantly positive impact on knowledge sharing behaviour (β = 0.2, p-value ≤ 0.01, supporting H3). Personal and community-related outcome expectations had significantly positive impacts on knowledge sharing behaviour (β = 0.201, p-value ≤ 0.01, supporting H4; β = 0.158, p-value ≤ 0.05, supporting H5). Knowledge sharing behaviour had a significantly positive impact on learning performance (β = 0.617, p-value ≤ 0.001, supporting H6). However, the structural and relational dimensions of social capital had insignificant impacts on knowledge sharing behaviour (thus rejecting H1 and H2).
In hierarchical component models, second-order variables are measured by their lower-order constructs. Trust, reciprocity, and identification were significantly (p ≤ 0.001) reflective-reflective lower-order constructs of the relational dimension of social capital (β = 0.837; β = 0.876; β = 0.915, respectively). Shared language and shared vision were significantly (p ≤ 0.001) reflective-reflective lower-order constructs of the cognitive dimension of social capital (β = 0.856 and β = 0.941, respectively).
According to J. F. J. Hair et al. (Citation2019, p. 780), “the effect size represents the change in the R-squared value when a specified exogeneous construct is omitted from the model”. There were two positive causal relationships with small effects of exogenous construct (i.e., the cognitive dimension of social capital and community-related outcome expectations influenced knowledge sharing behaviour). Personal outcome expectations had a medium effect on knowledge sharing behaviour. Knowledge sharing behaviour had a large effect on learning performance. All reflective-reflective specification of second-order constructs had large effects (reflected through the causal relationships between second-order constructs and their first-order constructs) (see Table ).
Table shows participation involvement insignificantly moderated the causal relationship between personal outcome expectations and knowledge sharing behaviour, although both participation involvement and personal outcome expectations had significant positive impacts on knowledge sharing with small effects.
Table 6. Result of moderating effect
5.3. Multigroup analysis
Table reveals new insights on knowledge sharing processes with multi-group analysis. In all sub-samples, second-order variables were significantly (p-value ≤ 0.001) measured by their lower-order constructs (i.e., the relational dimension of social capital was measured by trust, reciprocity, and identification; the cognitive dimension was measured by shared language and shared vision). In terms of the respective countries, the structural, relational, and cognitive dimensions of social capital had significant positive impacts on knowledge sharing behaviour among Vietnamese students, but only insignificant impacts on their Bangladeshi counterparts. We found a significantly positive causal relationship between community-related outcome expectations and knowledge sharing behaviour, but only among Bangladeshi students. Regarding the courses studied, only the cognitive dimension of social capital had a significantly positive impact on knowledge sharing behaviour among Social Science & Art students, whereas there was an insignificantly causal relationship between these two in Science & Engineering students. Moreover, we identified a significantly positive causal relationship between participation involvement and knowledge sharing behaviour among two certain sub-samples, namely Bangladeshi students, and Social Science & Art students.
Table 7. Multigroup analysis
6. Discussion
This study revisited the causal relationship between social capital and knowledge sharing. Chiu et al. (Citation2006) and Chang and Chuang (Citation2011) considered two facets of knowledge sharing (i.e., quantity and quality), and proved its positive causal relationships with different types of social capital. However, some insignificant causal relationships have also been identified, such as social interaction and trust on quantity of knowledge sharing (Chang & Chuang, Citation2011), trust and shared language on the quantity of knowledge sharing (Chiu et al., Citation2006), and reciprocity and identification on the quality of knowledge sharing (Chiu et al., Citation2006). Building on Chiu et al. (Citation2006) and Chang and Chuang’s (Citation2011) grounded theory on social capital, we developed a conceptual framework in the form of a hierarchical component model with second-order variables (e.g., the relational and cognitive dimensions of social capital). We found there to be significantly reflective-reflective specifications on such second-order variables in the measurement model. We conceptualised knowledge sharing behaviour in terms of quality and behaviour, as per Kang et al. (Citation2008) and Masa’deh et al. (Citation2016). We also identified the significantly positive causal relationship between the cognitive dimension of social capital and knowledge sharing. Contrary to our expectations, the structural and relational dimensions of social capital had insignificant relationships to knowledge sharing behaviour. These findings aligns with those of Chang and Chuang (Citation2011), and are partially consistent with Chiu et al. (Citation2006). From this standpoint, this study contributes a new explanation on the causal relationship between social capital and knowledge sharing. Social capital should be multidimensionally conceptualised with a foundation in social capital theory.
Similar to Chiu et al. (Citation2006), this study proved the significantly positive causal relationships between outcome expectations (both personal and community-related) and knowledge sharing behaviour. However, where it differs is that Chiu et al. (Citation2006) only found this positive relationship with community-related outcomes. Accordingly, by revisiting this topic, we have been able to provide additional empirical results.
Furthermore, we also introduced a new moderator (participation involvement) intervening the causal relationship between personal outcome expectations and knowledge sharing. Contrary to our expectations, participation involvement was an insignificant moderator interrupting said causal relationship. However, participation involvement was a significant driver of knowledge sharing in this study, which is consistent with Chang and Chuang (Citation2011).
Various outcomes from the knowledge sharing process were investigated, such as organisational and learning performance, and innovation. In line with Eid and Al-Jabri (Citation2016), we confirmed that knowledge sharing processes within student virtual communities can improve learning performance.
This paper also provides practical implications on knowledge sharing processes among university students in virtual communities. Knowledge sharing behaviours in this context concern the existence of in-group feelings, shared languages (in terms of understandable writing and conversation), and a shared vision (experiences) among members. Knowledge sharing processes can also occur when personal and community outcome expectations are fulfilled. Intrinsic and extrinsic rewards (e.g., bonus points and compliments, respectively) tend to be motivators for student involvement in the knowledge sharing process. Knowledge contribution conducted by group members and appropriate strategies formulated by group owners can yield active status, social recognition, reputation, and general success to the social environment of a virtual community. Membership policies attract more loyal and enthusiastic group members. Lastly, knowledge sharing processes with social capital and outcome expectations can provide students with both academic and non-academic benefits.
7. Conclusion
The study revisited social capital and knowledge sharing processes among Vietnamese and Bangladeshi students in tertiary education. As a novel contribution to the topic, we developed a hierarchical component model with second-order constructs to explain such observed phenomena. Using a new explanation, we support the notion that knowledge sharing processes created by (cognitive) social capital can improve the learning performance of students in virtual communities. Social capital, personal and community-related outcome expectations, and participation involvement are the main drivers of knowledge sharing behaviour; while increased learning performance is one of the crucial benefits/outcomes from such knowledge sharing processes. The observed phenomenon reflects knowledge sharing process among students in virtual community.
This study has two limitations in research design. Social network analysis would be applied to measure structural and relational dimensions of social capital and its impacts. Observations should be independent to avoid unbiased effect coefficients and contribute to generalizability from research findings.
Further research can investigate whether the structural and cognitive dimensions of social capital can induce knowledge sharing behaviour in different contexts (e.g., in Western countries). The concepts of knowledge sharing and outcome expectations could be intensely reviewed and developed in the form of multidimensional constructs in future research.
Disclosure statement
No potential conflict of interest was reported by the author(s).
Additional information
Funding
Notes on contributors

Han Nu Ngoc Ton
Han Nu Ngoc Ton (Ph.D) is the lecturer and researcher at School of Business, International University, Vietnam National University, Ho Chi Minh City. Her research interests are development and sustainable development, public policy, development economics, gender equality, child marriage, sociology, knowledge sharing, social housing, neighborhood governance, event management. Email address: [email protected]
References
- Ahmad, F., & Karim, M. (2019). Impacts of knowledge sharing: A review and directions for future research. Journal of Workplace Learning, 31(3), 207–32. https://doi.org/10.1108/JWL-07-2018-0096
- Andrews, D. C. (2002). Audience-specific online community design. Communications of the ACM, 45(4), 64–68. https://doi.org/10.1145/505248.505275
- Blasco-Arcas, L., Buil, I., Hernández-Ortega, B., & Sese, F. J. (2013). Using clickers in class. The role of interactivity, active collaborative learning and engagement in learning performance. Computers & Education, 62, 102–110. https://doi.org/10.1016/j.compedu.2012.10.019
- Bock, G. W., & Kim, Y.-G. (2002). Breaking the myths of rewards. Information Resources Management Journal, 15(2), 14–21. https://doi.org/10.4018/irmj.2002040102
- Bourdieu, P. (2011). “The forms of capital” (1986). in. In I. Szeman & T. Kaposy (Eds.), Cultural theory: An anthology (1st) ed.) (pp. 81–91). Wiley–Blackwell. https://books.google.com.vn/books?id=O5uGEAAAQBAJ&printsec=frontcover#v=onepage&q&f=false
- Bukowitz, W. R., & Williams, R. L. (2000). The knowledge management fieldbook. Financial Times-Prentice Hall.
- Butler, B., Sproull, L., Kiesler, S., & Kraut, R. (2007). Community effort in online groups: Who does the work and why. In S. P. Weisban (Ed.), Leadership at a distance: Research in technologically-Supported work (pp. 171–194). Taylor & Francis Group.
- Chang, H. H., & Chuang, -S.-S. (2011). Social capital and individual motivations on knowledge sharing: Participant involvement as a moderator. Information & Management, 48(1), 9–18. https://doi.org/10.1016/j.im.2010.11.001
- Charband, Y., & Jafari Navimipour, N. (2018). Knowledge sharing mechanisms in the education. Kybernetes, 47(7), 1456–1490. https://doi.org/10.1108/K-06-2017-0227
- Cheah, J. H., Memon, M. A., Chuah, F., Ting, H., & Ramayah, T. (2018). Assessing reflective models in marketing research: A comparison between PLS and PLSC estimates. International Journal of Business and Society, 19(1), 139–160. https://www.ijbs.unimas.my/index.php/volume-11-20/volume-19-no-1-2018/435-assessing-reflective-models-in-marketing-research-a-comparison-between-pls-and-plsc-estimates
- Chiu, C.-M., Hsu, M.-H., & Wang, E. T. G. (2006). Understanding knowledge sharing in virtual communities: An integration of social capital and social cognitive theories. Decision Support Systems, 42(3), 1872–1888. https://doi.org/10.1016/j.dss.2006.04.001
- Cohen, J. (1988). Statistical Power Analysis for the Behavioral Sciences (2nd) ed.). Routledge.
- Eid, M. I. M., & Al-Jabri, I. M. (2016). Social networking, knowledge sharing, and student learning: The case of university students. Computers & Education, 99, 14–27. https://doi.org/10.1016/j.compedu.2016.04.007
- Grant, R. M. (1996). Toward a knowledge-based theory of the firm. Strategic Management Journal, 17(S2), 109–122. https://doi.org/10.1002/smj.4250171110
- Hair, J. F. J., Black, W. C., Babin, B. J., & Anderson, R. E. (2019). Multivariate Data Analysis (8th) ed.). Cengage Learning EMEA.
- Hair, J. F., Jr, Hult, G. T. M., Ringle, C., & Sarstedt, M. (2017). A primer on partial least squares structural equation modeling (PLS-SEM). Sage publications.
- Hair, J. F., & Sarstedt, M. (2019). Factors versus Composites: Guidelines for Choosing the Right Structural Equation Modeling Method. Project Management Journal, 50(6), 619–624. https://doi.org/10.1177/8756972819882132
- Henseler, J., Ringle, C. M., & Sarstedt, M. (2015). A new criterion for assessing discriminant validity in variance-based structural equation modeling. Journal of the Academy of Marketing Science, 43(1), 115–135. https://doi.org/10.1007/s11747-014-0403-8
- Henttonen, K., Kianto, A., & Ritala, P. (2016). Knowledge sharing and individual work performance: An empirical study of a public sector organisation. Journal of Knowledge Management, 20(4), 749–768. https://doi.org/10.1108/JKM-10-2015-0414
- Jones, T., & Taylor, S. F. (2012). Service loyalty: Accounting for social capital. Journal of Services Marketing, 26(1), 60–75. https://doi.org/10.1108/08876041211199733
- Jöreskog, K. G. (1971). Simultaneous factor analysis in several populations. Psychometrika, 36(4), 409–426. https://doi.org/10.1007/BF02291366
- Kang, Y.-J., Kim, S.-E., & Chang, G.-W. (2008). The impact of knowledge sharing on work performance: An empirical analysis of the public employees’ Perceptions in South Korea. International Journal of Public Administration, 31(14), 1548–1568. https://doi.org/10.1080/01900690802243607
- Kock, N. (2017). Partial least squares path modeling. In Hengky, L., & Richard, N. (Eds.), Common method bias: A full collinearity assessment method for PLS-SEM (pp. 245–257). Springer International Publishing. https://doi.org/10.1007/978-3-319-64069-3_11
- Kolekofski, K. E., & Heminger, A. R. (2003). Beliefs and attitudes affecting intentions to share information in an organizational setting. Information & Management, 40(6), 521–532. doi.00068-X. https://doi.org/10.1016/S0378-7206(02)
- Lefebvre, V. M., Sorenson, D., Henchion, M., & Gellynck, X. (2016). Social capital and knowledge sharing performance of learning networks. International Journal of Information Management, 36(4), 570–579. https://doi.org/10.1016/j.ijinfomgt.2015.11.008
- Lesser, E. L. (2000). Leveraging social capital in organizations. In E. L. Lesser (Ed.), Knowledge and social capital: Foundations and applications (pp. 3–16). Butterworth-Heinemann. https://books.google.com.vn/books?id=Qh94ajh1kLAC&printsec=frontcover&source=gbs_atb#v=onepage&q&f=false
- Liang, C., Chang, -C.-C., Rothwell, W., & Shu, K.-M. (2016). Influences of organizational culture on knowledge sharing in an online virtual community. Journal of Organizational and End User Computing, 28(4), 15–32. https://doi.org/10.4018/JOEUC.2016100102
- Lin, X., Xu, X., & Wang, X. (2020). Users’ knowledge sharing on social networking sites. Journal of Computer Information Systems, 1–10. https://doi.org/10.1080/08874417.2020.1736690
- Masa’deh, R., Obeidat, B. Y., & Tarhini, A. (2016). A Jordanian empirical study of the associations among transformational leadership, transactional leadership, knowledge sharing, job performance, and firm performance. Journal of Management Development, 35(5), 681–705. https://doi.org/10.1108/JMD-09-2015-0134
- Meek, S., Ryan, M., Lambert, C., & Ogilvie, M. (2019). A multidimensional scale for measuring online brand community social capital (OBCSC). Journal of Business Research, 100(October2018), 234–244. https://doi.org/10.1016/j.jbusres.2019.03.036
- Moghavvemi, S., Sharabati, M., Paramanathan, T., & Rahin, N. M. (2017). The impact of perceived enjoyment, perceived reciprocal benefits and knowledge power on students’ knowledge sharing through Facebook. The International Journal of Management Education, 15(1), 1–12. https://doi.org/10.1016/j.ijme.2016.11.002
- Nahapiet, J., & Ghoshal, S. (1998). Social capital, Intellectual capital, and the organizational advantage. Academy of Management Review, 23(2), 242–266. https://doi.org/10.5465/amr.1998.533225
- Nguyen, L., Torlina, L., Peszynski, K., & Corbitt, B. (2006). Power relations in virtual communities: An ethnographic study. Electronic Commerce Research, 6(1), 21–37. https://doi.org/10.1007/s10660-006-5986-9
- Putnam, R. D. (1995). Bowling alone: America’s declining social capital. Journal of Democracy, 6(1), 65–78. https://doi.org/10.1353/jod.1995.0002
- Radević, I., Dimovski, V., Lojpur, A., & Colnar, S. (2021). Quality of healthcare services in focus: The role of knowledge transfer, Hierarchical organizational structure and trust. Knowledge Management Research & Practice, 1–12. https://doi.org/10.1080/14778238.2021.1932623
- Romm, C., Pliskin, N., & Clarke, R. (1997). Virtual communities and society: Toward an integrative three phase model. International Journal of Information Management, 17(4), 261–270. doi.00004-2. https://doi.org/10.1016/S0268-4012(97)
- Sarstedt, M., Hair, J. F., Cheah, J.-H., Becker, J.-M., & Ringle, C. M. (2019). How to specify, estimate, and validate higher-Order constructs in PLS-SEM. Australasian Marketing Journal, 27(3), 197–211. https://doi.org/10.1016/j.ausmj.2019.05.003
- Schaefer, R. T. (2022). Sociology: A Brief Introduction (14th) ed.). McGraw-Hill Education.
- Sheng, M., & Hartono, R. (2015). An exploratory study of knowledge creation and sharing in online community: A social capital perspective. Total Quality Management & Business Excellence, 26(1–2), 93–107. https://doi.org/10.1080/14783363.2013.776769
- Singh, S. K., Mazzucchelli, A., Vessal, S. R., & Solidoro, A. (2021). Knowledge-based HRM practices and innovation performance: Role of social capital and knowledge sharing. Journal of International Management, 27(1), 100830. https://doi.org/10.1016/j.intman.2021.100830
- Strang, K. D. (2011). Asynchronous knowledge sharing and conversation interaction impact on grade in an online business course. Journal of Education for Business, 86(4), 223–233. https://doi.org/10.1080/08832323.2010.510153
- Tsai, W., & Ghoshal, S. (1998). Social capital and value creation: The role of intrafirm networks. Academy of Management Journal, 41(4), 464–476. https://doi.org/10.5465/257085
- Wasko, & Faraj. (2005). Why should I share? Examining social capital and knowledge contribution in electronic networks of practice. MIS Quarterly, 29(1), 35. https://doi.org/10.2307/25148667
- Wetzels, M., Odekerken-Schröder, G., & Van Oppen, C. (2009). Using PLS path modeling for assessing hierarchical construct models: Guidelines and empirical illustration. MIS Quarterly, 33(1), 177. https://doi.org/10.2307/20650284
- Wong, -K.-K.-K. (2019). Mastering partial least squares structural equation modeling (PLS-SEM) with smartPLS in 38 hours. iUniverse.
- Yamane, T. (1967). Statistics, An Introductory Analysis (2nd) ed.). Herper and Row.
- Yan, J., Leidner, D. E., Benbya, H., & Zou, W. (2019). Social capital and knowledge contribution in online user communities: One-way or two-way relationship? Decision Support Systems, 127(March), 113131. https://doi.org/10.1016/j.dss.2019.113131
- Yli-Renko, H., Autio, E., & Sapienza, H. J. (2001). Social capital, knowledge acquisition, and knowledge exploitation in young technology-based firms. Strategic Management Journal, 22(6–7), 587–613. https://doi.org/10.1002/smj.183
- Zhang, X., Liu, S., Deng, Z., & Chen, X. (2017). Knowledge sharing motivations in online health communities: A comparative study of health professionals and normal users. Computers in Human Behavior, 75, 797–810. https://doi.org/10.1016/j.chb.2017.06.028