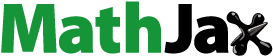
Abstract
Crime imposes significant direct and indirect costs on society. While a great wealth of literature focuses on crime as a result of prevailing economic conditions, one cannot exclude the possibility that the relationship between crime and economic growth may go the other way around. This study employs an Autoregressive Distributed Lag (ARDL) approach to empirically examine the extent to which homicides affect economic growth. The case study was conducted in India, and longitudinal annual data from 1990 to 2019 were employed. The bounds cointegration test reveals that crime rate, proxied by homicide rate, along with investment, FDI, exports, and enrolment Granger cause real per capita GDP in the long run. The homicide rate will have a significantly negative impact on economic growth in the short run. A one percentage increase in homicide rates leads to 0.25% decrease in economic growth. The findings point to urgent attention to the reduction of crime rates by increasing the opportunity cost of criminal activities and reducing the damaging effects of homicides on economic growth.
1. Introduction
While a great wealth of literature focuses on economic determinants of crimes, one cannot exclude the possibility that crimes have an impact on economic growth as well. Crime, in its broadest sense, is any act of deviation that is punishable upon conviction as per the laws of the state (Raj & Rahman, Citation2023). Broadly, crime can be categorized into crime against persons, crime against property, economic crimes, such as money laundering, corruption, drug trafficking, customer frauds, tax evasions, company frauds, cybercrimes, etc. Crimes against persons include offenses, such as murders, kidnapping and abduction, assaults, and rape, in which victims are always individuals. Crimes against property are offenses that are targeted towards victims by offenders to obtain material gains. Crimes including burglary, robbery, dacoity, housebreaking, thefts, vandalism, etc., are examples of property (usually private property) crimes. Economic crimes, usually not involving violence of any sort, are offenses, which are committed during the course of some kinds of business activities. Economic offences not only inflict pecuniary losses on individuals but also damage the national economy and have security implications as well (NCRB, n.d.). Crime imposes significant direct and indirect costs on society. Direct costs derive values from lost human lives, incapacitation of victims, foregone earnings of both the victims and perpetrators, medical expenses in cases of injury, prevention costs, such as policing costs, incarceration costs, judicial expenses, private spending on security personnel, installation of CCTV cameras for surveillance purposes, and so on. Although it is challenging to quantify the costs of incapacitated victims or lost human lives, other direct costs can be roughly quantified in monetary terms. The total of these costs can be viewed as a loss of social welfare and consequently a negative effect on the economy. The impact of direct costs on society is immediate costs attributable to a specific crime and its prevention. Second-order additional costs resulting from criminal activities are termed indirect costs. Such costs include reductions in investment due to fear of crime, lost productivity arising from physical or psychological trauma, loss of confidence in the rule of law, reduction in manhours worked, and reduction in economic activities arising from limited movement. These costs are detrimental because they have long-running structural implications on the economy. The perceived loss of security brought on by the undermining of law and diminished protection of private property rights creates unfavorable conditions for entrepreneurial activities. Criminal activity also hinders a state’s ability to attract skilled workers, domestic and foreign investment, decreases firm competitiveness, and increases business uncertainty, all of which decrease the ease of doing business in an area with high crime rates (Brand & Price, Citation2000; Dutta & Husain, Citation2009; Goulas & Zervoyianni, Citation2012; Greenbaum & Tita, Citation2004; Pellegrini & Gerlagh, Citation2004; Sandler & Enders, Citation2008). Some industries, particularly the hospitality sector, are highly sensitive to crime rates. Within the context of the developing world, the rising crimes encountered by medium- and small-sized firms have direct negative consequences on investment (Islam, Citation2014).
Scholars have paid much attention to understanding the determinants of crime; however, the role that criminal activity plays in affecting economic growth remains largely neglected (Detotto & Otranto, Citation2010). The present study underscores the effects of crime on the economic performance of a nation. Specifically, we tested the hypothesis of a negative impact of crime on economic growth. To this end, this study employs the auto-regressive distributed lag (ARDL) bounds test of cointegration to investigate into interrelationships between crime and economic growth controlling for other factors that are determinants of growth. The case study was conducted in India, and longitudinal annual data from 1990 to 2019 were employed. Due to the diversity of its ethnic and religious groups, socioeconomic conditions, and demographics, India offers an interesting case study to illustrate the relationships between crime and economic variables. According to the Global Peace Index Report 2022,Footnote1 India is placed in the list of top 30 countries affected by violence, and it is estimated that crime costs India 6% of its Gross Domestic Product (GDP) annually. The estimated cost represents a sizable economic burden of crime for developing economies such as India. The Criminal Procedure Code (CrPC) of India identifies two crime categories: cognizable and non-cognizable. Additionally, cognizable offenses are further divided into crimes that fall under the Indian Penal Code (IPC), or under the Special and Local Laws (SLL). This study considers only the total cognizableFootnote2 IPC offenses. The information on crime records is gathered from the annual publications of the National Crime Records Bureau (NCRB), India.
Given the significant costs that criminal activity imposes on the economy, it is imperative to conduct an empirical investigation into understanding the relationship between crime and economic growth in India. Does crime impede India’s economic growth, even after accounting for other factors that stimulate growth? If so, what kind of policy framework should be designed to minimize the damaging effects of crime rates on economic growth? An incentive to reform and modernize the current criminal justice system will come from an empirical study of the impact of crime rates on growth. The purpose of the current study is to provide empirical evidence concerning the effects of crime rates on economic growth for India. This study hypothesizes that crime’s negative effects on individuals, communities, and societal welfare can impede economic growth. The rest of this paper is as follows: Following this is a discussion of theoretical and conceptual issues. A section on research methods and the empirical model will be developed further in this section, which will then be followed by empirical results and discussions. The final section concludes the paper with policy recommendations.
2. Theoretical issues
Protection of private property rights and contract enforcement are the fundamental theoretical mechanisms by which the rule of law, particularly crime, exerts influence on economic development. Early contract theorists, most notably Hobbes,Footnote3 described the rule of law in terms of the provision of security. It makes little sense to discuss the protection of property or enforcement of contracts if economic agents do not feel safe about their personal lives. The incentives people have to invest and trade when their rights and lives are secure are at the heart of the connection between property rights and economic growth (Haggard & Tiede, Citation2011). It has been well established empirically that robust property rights protection and contract enforcement are positively associated with long-run economic performances (Barro, Citation2000; Hanushek, Citation2013; Keefer, Citation2007; Asoni, Citation2008; Lee & Son, Citation2016). Freedom from violence is one of the five fundamental freedoms listed by Sen (Citation2000) as being necessary for a society to advance in the direction of sound economic development. He argued that the state must endeavor to create laws that ensure everyone has the right to protective security and that their lives and property will be protected. Institutionalists have long debated the role of right and just institutions in promoting equitable economic development (North, Citation1991; Posner, Citation1981).
Initial theories of crime construed crime as a social construct and examined the effects of social deprivation and poverty on crime rates in a society (Shaw & McKay, Citation1942; Cloward & Ohlin, Citation1960; Merton, 1968). The connection between criminal activity and economics was first established by Fleisher’s (1963) study on the association between unemployment and juvenile delinquency. Becker’s (Citation1968) theory of criminal behavior based on rational choice provides a theoretical framework for the economics of crime. In his choice-based microeconomic framework, Becker advanced the theory that, like any rational economic agent, criminals weigh the financial benefits of committing crimes against those of lawful employment, considering the likelihood of being apprehended and convicted as well as the severity of punishment, before engaging in illegal activity. Simply put, criminals are utility maximizers, and their decision to commit a crime is driven by rational considerations as opposed to social anomalies and unique personality traits. Ehrilch (Citation1973) extended Becker’s paradigm by incorporating income distribution, unemployment, income levels, and schooling and their effects on criminal propensity. The findings of this study highlighted that the distribution of income and income levels have a greater impact on criminal behavior than unemployment. Additionally, schooling as a proxy for educational attainment has a negative impact on delinquent behaviors. Becker’s (Citation1968), and Ehrilch’s (Citation1973) theories serve as the cornerstone for empirical investigations of the economics of crime.
Numerous studies have emerged in the literature on the economics of crime since Becker’s (Citation1968) theoretical underpinnings of criminal behavior. Masih and Masih (Citation1996) investigate the association between socioeconomic variables and several crime typologies for the Australian economy from 1960 to 1993. The study concludes that urbanization and bad economic conditions positively impact criminal activity, however, it fails to find the direction of temporal causality otherwise. In their study on Italian provinces, Forni and Paba (Citation2000) report a strong negative impact of homicides on economic performance. Peri (Citation2004) reports that homicide rates had a negative impact on economic output and employment in Italian provinces for the period 1951 – 1999. Narayan and Smyth (Citation2004), within an Autoregressive Distributed Lag (ARDL) framework, analyze the relationship between real wage, unemployment, and seven different types of crime in the Australian economy (1964–2001). The authors find that real income is granger caused by homicides, vehicle thefts, and unemployment in the long run. Cardenas (Citation2007) investigate the potential reasons for the decline in the economic growth of Columbia from 1971 to 1999. His study reveals that the decline in labor productivity, due to rising criminal activities brought on by rapid expansion in drug-trafficking activities is the primary reason for slowing Columbian economy. Within an ARDL framework using Italian regional data, L. Mauro and Carmeci (Citation2007) find negative impact of crime rates on output levels in the long run. Tang (Citation2009) examines long run linkages among crime rates, inflation, and unemployment in the Malaysian economy. The findings of his study reveal that inflation and unemployment are positively associated with crime rates. Although the cointegration tests indicate a unidirectional causality running from economic variables to crime rates, however, no evidence of a reverse causality was found in his study. Habibullah and Baharom (Citation2009) estimate interrelationships between various crime typologies and real gross national product for the Malaysian economy (1973–2003). They report a long run granger causality running from economic variable to crime rates. Using a large sample of cross-country panel dataset (1991–2005), Chatterjee and Ray (Citation2009) find no conclusive evidence of a negative relationship between economic growth and crime rates, even after controlling for institutional quality and human capital as other growth determinants. Detotto and Otranto (Citation2010), using a state-space model, find evidence for the damaging effects of criminal activities on economic growth in the Italian economy. In a cross-sectional country examination for the period 1991 – 2007, Goulas and Zervoyianni (Citation2012) report that crime rates impact economic growth severely in the areas experiencing higher-than-average macroeconomic uncertainty. Simply put, macroeconomic uncertainty matters to a great extent in amplifying the negative effects of crime on economic growth. Jennings et al. (Citation2012) look into unintended consequences of neoliberal and monetarist economics policy stance on property crime rates in England and Wales for the period 1961 to 2006. Specifically, they test the effects of changes in socioeconomic variables (inequality, welfare spending, unemployment, and incarceration) arising out of the changed policy stance on property crime rates. The findings of the study suggest that increases in the unemployment rate led to an increase in property crime rates in England and Wales over the study period. Additionally, no significant association between crime rates and inequality is reported. Detotto and Pulina (Citation2013) investigate the interdependencies between economic variables and crime rates in Italy from 1970 to 2004. The findings of the study highlight the crowding-out effect of robberies and homicides on growth rate of Gross Domestic Product (GDP) for the Italian economy. Moreover, the econometric results indicate evidence of a short-run bi-directional causality between growth rate and property crimes. Naddeo (Citation2014) employed instrumental variable estimation method on a panel data on 19 Italian regions to empirically analyze the relationship between crime rate and economic growth. A two stage least squares (2-SLS) regression was run using effective abortion rate as an instrumental variable for crime rates. He finds a significantly negative impact of crime rates on both the level of per capita GDP and growth rates across the Italian regions. Using Ordinary Least Squares (OLS) on disaggregated level data on firms, Islam (Citation2014) found that violent crime has a significant negative impact on small and medium-sized businesses. The results show that increased criminal activity severely distorts investment necessary for economic growth in developing economies. Draca and Machin (Citation2015) look into the interrelationships between economic incentives and crime rates in the United Kingdom. They find that economic incentives exert influence over crime rates through effects on labor market opportunities and returns from illegal activities. Mulok et al. (Citation2016), using bounds test approach within an ARDL framework, reported strong evidence of long run cointegration between crime and economic growth in Malaysia from 1980 to 2013. Using the ARDL framework for the Nigerian economy in the period 1970–2013, Adekoya and Razak (Citation2017) report a significant negative impact of crime on economic growth for the Nigerian economy. Further, the study concludes that criminal activity impedes economic growth by placing a significant burden on the nation brought on by the costs of prosecution and punishment. Using the Generalized Methods of Moments (GMM) estimator, Anser et al. (Citation2020) examined the relationship between growth-inequality-poverty (GIP) triangle and crime rates for a panel of 16 countries in the period 1990–2014. They found that despite the fact that crime rates substantially increase income inequality, there is no correlation between crime rates and per capita income. Using a systematic literature review approach, Jonathan et al. (2021) conclude that crime hinders economic growth and instills fear and tension. The effect of crime on economic growth in emerging economies has so far received little scholarly attention. Kumar (Citation2013), using the panel Generalized Method of Moments estimator (System GMM), investigates the dynamic relationship between crime and economic growth in the Indian economy (1991–2011). This study includes homicide and robbery rates as proxies for crime and real net state domestic product per capita as indicators of economic growth. He finds a statistically significant and negative impact of crime on economic growth for the Indian states in the period 1991–2011. Within an Autoregressive Distributed Lag (ARDL) framework, A. Ahmad et al. (Citation2014) analyze the empirical relationship between crime and economic growth for the Pakistani economy using time series data from 1980 to 2011. The results of the cointegration test show that crime exerts a significantly negative impact on economic growth in the long run, whereas the impact is negative but insignificant in the short run. Debnath and Das (Citation2017) conduct an empirical investigation into dynamic linkages between crime rates and economic growth for the Indian economy in the period 1982 to 2013. The results of cointegration tests present evidence of a bi-directional causality between crime rates and economic growth. Moreover, while economic growth is found to be positively associated with violent crime rates in the long run, violent crime rates appear to have significant negative impact on economic affluence in the long run. Parida et al. (Citation2017) investigate into impact of crime and economic development on tourism in India. The results obtained from the econometric analysis reveal that criminal activity negatively affects the inflow of tourists in short run for the Indian economy. Raj and Rahman (Citation2023) look into relationships between crime and deterrence variables for the Indian economy between 2001 and 2014. Using panel data on 20 Indian states, system generalized methods of moments (GMM) was used to arrive at empirical results. The findings of the study highlight that increases in the likelihood of deterrence variables like arrest rates have not led to declines in crime rates in the Indian economy over the study period.
From the literature review, it is clear that scholars have not arrived at a consensus on the crime-growth nexus. Mixed evidence on the interrelationships between crime and economic growth depends primarily on the econometric method used and the country analyzed. However, most of the studies have been conducted in the context of advanced economies. The empirical relationship between economic variables and crime rates is an expanding field of research within a time-series framework (Detotto & Pulina, Citation2013). Crime rate is considered as one of the explanatory variables along with other determinants of growth in time-series models. The cointegration technique for time series is used to determine the existence of long-run relationships between various time-series variables. Several cointegration techniques such as the Johansen cointegration test (Johansen, Citation1991), Granger test (Engle & Granger, Citation1987), and Phillips-Ouliaris test (Phillips & Ouliaris, Citation1990) have been used in the previous research. The ARDL based bound test for cointegration proposed by Pesaran et al. (Citation2001) has advantages over the above-mentioned traditional techniques. First, unlike other tests, the ARDL test does not require that all variables be integrated in the same order; instead, it can be used with variables that are integrated in orders of zero, one, or even fractionally integrated (Belloumi, Citation2013). Second, the ARDL test is very useful, particularly for small sample sizes. Third, the ARDL technique produces unbiased estimates for the long-run coefficients in the model (Harris & Sollis, Citation2003). Additionally, because of its built-in dynamic tool, the ARDL model typically solves the simultaneity/endogeneity problem of socioeconomic variables (Adekoya & Razak, Citation2017).
In light of the existing literature, this study is a novel attempt to establish the temporal causality between crime and economic growth in the Indian economy. To the best of our knowledge, no such empirical studies have examined the relationship between crime rates and economic growth while accounting for other factors that determine growth in the Indian economy. This study employs the ARDL bound test of cointegration to conduct an empirical investigation into understanding the relationship between crime and economic growth in India. Even though maintenance of law and order is a state’s responsibility in the federal structure of the Union of India, we firmly believe that the formulation of policies to reduce the effects of crime on growth would greatly benefit from an empirical analysis of the cointegration between crime and economic growth, even at the national level.
3. Data sources and research methods
3.1. Sample
The empirical analysis is conducted using gross domestic product (GDP), gross fixed capital formation (GFCF), exports, foreign direct investment (FDI), enrolment in secondary school (9–12), and crime rates data pertaining to the period 1990 to 2019. The data series on GDP, GFCF, and exports were obtained from national account statistics of the Ministry of Statistics and Planning Implementation (MOSPI). The nominal time-series data on these variables were converted to real values (constant at 2011–12 prices) using a GDP deflator. Time-series data on FDI have been taken from the Reserve Bank of India’s (RBI) database on the Indian economy. Data on secondary school enrolment were collected from various publicationsFootnote4 by the Ministry of Education, India. The variables are expressed in natural logarithms; hence, the first difference between these variables gives their corresponding growth rates. Real per capita GDP is a measure of economic growth. The significance of physical and human capital accumulations as important determinants of economic growth has long been established (Acemoglu et al., Citation2005; Barro, Citation2000; Benhabib & Spiegel, Citation1994). Machineries, buildings, warehouse supplies, vehicles, computers, and other items that are required as inputs in the production of a good or service are referred to as physical capital. On the other hand, human capital comprises the economic value of a worker’s experience and skills. Unlike physical capital, such capital is an intangible asset that and enters the production process. Education, health, skills, intelligence, and other assets that contribute to capacity building in the economy are human capital. It is perceived to be crucial for enhancing productivity and has positive qualitative effects on growth. Real gross-fixed capital formation is a proxy for measuring the accumulation of physical capital in the economy. Human capital is a multidimensional concept, and it can be challenging to estimate a country’s human capital. Enrolment in secondary education can be used as a proxy to measure the availability of human capital, although it is not a crude measure of human capital. The argument for using secondary school enrollment as a proxy variable runs on the grounds that higher educational attainment raises skills and capabilities and is associated with increased returns in the labor market (Dreze & Khera, Citation2000; Dutta & Husain, Citation2009). Hence, we have used total enrolment in secondary school (class 9−12) as a proxy for human capital accumulation in this study. Balassa (1978); Ghatak et al., (Citation1997); Love and Chandra, (2004); Kaushik et al., (Citation2008) have pointed out the impact of exports and inward foreign direct investment on economic growth. Following the literature, this study uses real exports of goods and services, and inward FDI as other growth determinants.
The available literature on the crime and growth nexus highlights the twin problems of underreporting and underestimation of official crime records. Of all the crime typologies, homicides suffer the least from the twin problems mentioned above. In this study, we have considered homicide rateFootnote5 as a proxy variable for measuring crime rates. Therefore, the crime rate enters the growth function as an additional key determinant, as previously mentioned, the direct and indirect channels through which crime exerts its detrimental effects on the economy. Theoretically, the expected sign of the coefficient of crime rate is negative, as it results in a loss of social welfare and, hence, a reduction in economic growth. The time-series data on homicide rates are obtained from annual reports published by the National Crime Records Bureau (NCRB), the only source of official crime statistics in India. A brief summary of the variables used in this study is presented in Table . The Jarque-Bera test of normality confirms that all variables were normally distributed. At the outset, we start with a preliminary correlation analysis between the real per capita GDP and homicide rates for the study period. The correlation coefficient of −0.97 indicates a strong negative association between the two variables. However, a correlation alone does not imply causation, as such, it cannot be used to prove causality.
Table 1. Descriptive Summary of Variables
4. Methodology
An ARDL bounds cointegration technique was employed to empirically investigate the short-run interactions and long-run relationships among the variables under consideration (real GDP per capita, GFCF, FDI, exports, enrollment, and homicide rate). Denoting real GDP per capita as G; GFCF as I; FDI as F, Exports as T; enrolment as E; and homicide rate as C, the vector Yt can be written as Yt = (Gt, It, Ft, Tt, Et, Ct)’. The implementation of the ARDL bounds test involves the following three steps.
Step 1: Conduct unit root tests to determine the order of integration of the variables. This step ensures that none of the variables is integrated of order greater than 1.
Step 2: Estimation of unrestricted error correction models. In this step, the decision on cointegration or no cointegration is made using the bounds test.
Step 3: If the cointegration in the equation is established, an error correction model is estimated. If no cointegration is found, only the short-run ARDL model is then estimated.
The unit root tests confirmed that none of the variables considered in this study were integrated in the order of more than 1. Hence, we proceed to Steps 2 and 3 accordingly. The unconditional error correction model can be written as:
is the first difference operator and ln(.) is the natural logarithm operator,
are the white noise error terms in EquationEquation 1
(1)
(1) . p denotes the lags of the dependent variables and q denotes the lags of the independent variables.
The bounds test of cointegration under the null hypothesis of no cointegration is based on non-standard asymptotic distribution of the joint F-statistic. As a first step in the ARDL bounds test approach, each EquationEquation 1(1)
(1) is estimated using the ordinary least squares (OLS) method. The bounds test determines the existence of a long-run cointegrating relationship between the variables under the null hypothesis (Ho:
against the alternate hypothesis (H1:
for i = 1, 2, 3, 4, 5, and 6. For a given level of significance, two sets of critical values are determined (Pesaran et al., Citation2001). One critical value (lower bound) is determined under the assumption that the variables are stationary at levels (integrated of order zero), and the other critical value (upper bound) is determined under the assumption that all variables are stationary at the first difference (integrated of order one). The decision rule is as follows: (1) Do not reject the null hypothesis of no cointegration when the calculated F-statistic from the estimated equation falls below the lower bound. (2) Reject the null hypothesis when the calculated F-statistic is greater than the upper bound. (3) If the calculated F-statistic falls within the lower and upper bounds, the cointegration test is inconclusive. For the equations in which the bounds test rejects the null hypothesis of no cointegration, an error correction model is estimated. In the event of no cointegration among the variables, only the short-run ARDL model is estimated.
After the cointegration test, the restricted error correction models are estimated, along with the long-run relationship equations. The EquationEquations (7)(7)
(7) –(Equation12
(12)
(12) ) present the restricted error correction models.
In the above equations, ) are the short-run dynamic coefficients and is the speed of adjustment to adjust to long-run equilibrium in the case of short-run shocks to the system. In the EquationEquations (7)
(7)
(7) –(Equation12
(12)
(12) ), ECT refers to the error correction term and is obtained as residuals from the estimation of the corresponding conditional long-run ARDL equation. It enters the equation with a lag period (ECT (−1)).
5. Empirical results and discussion
Augmented Dickey Fuller (ADF) and Phillips-Perron (PP) tests were conducted to determine the integration order of the variables used in this study. The ARDL bounds test of cointegration produces spurious results in the presence of variables that are integrated of order higher than one. The null hypothesis under both tests assumes that the series has a unit root. Hence, rejection of the null hypothesis implies that the series is stationary. Table presents the results obtained from the unit root tests.
Table 2. Unit root tests
The unit root tests’ results confirm that all variables except FDI are non-stationary at their levels. Hence, FDI is considered to be stationary at level ((I (0)) and the remaining variables become stationary at their first differences (I (1)). After unit root tests, bounds test of cointegration is performed for Equationequations (1)(1)
(1) –(Equation6
(6)
(6) ). The use of the ARDL bounds test of cointegration is guided by a short data span. Hence, appropriate lag selection is imperative for such studies. Based on Akaike Information Criterion (AIC), a lag order of 1 was chosen for the conditional vector error correction models in this study. The results of the ARDL bounds test of cointegration are presented in Table .
Table 3. Results of Bounds test of Cointegration
The results confirm the existence of a long-run relationship between the explanatory variables (GFCF, FDI, exports, enrollment, and homicide rates) and economic growth, when real per capita GDP is the dependent variable. A long run cointegrating relationship is observed between gross-fixed capital formation and the explanatory variables (real per capita GDP, FDI, exports, enrolment, and homicide rates) at the 1% significance level. Additionally, evidence of long-run relationships among the variables was found when the dependent variables were exports, and enrolment. The cointegration tests reveal no evidence of a cointegrating relationship among the variables when the homicide rate is the dependent variable. Therefore, error correction models are estimated for EquationEquations (1)(1)
(1) –(Equation5
(5)
(5) ), and only the short-run ARDL model is estimated for Equationequation (6)
(6)
(6) .
In Table , we present the estimated long-run coefficients for the restricted error correction models. There is evidence of long-run bi-directional causality between real per capita GDP and real gross-fixed capital formation, between real gross-fixed capital formation and exports, between FDI and exports, between exports and enrolment, and between enrolment and FDI. In addition, the results show evidence of unidirectional causality running from homicide rates to exports, real per capita GDP to FDI, exports, and enrolment in the long run. Surprisingly, FDI inflows are found to be negatively associated with enrolment in secondary schools. This finding can be explained in two ways: First, the finding might have arisen due to the use of aggregated FDI data instead of a disaggregated FDI inflow by industry type. Second, the ability of the labor force to effectively leverage its education into creating earning opportunities in the labor market seems to be more important in attracting FDI than simply the level of educational attainment. The estimated coefficients in the long-run conditional ARDL equations are elasticities because the variables are expressed in natural logarithms. In EquationEquation 1(1)
(1) , only real gross-fixed capital formation is statistically significant in the long run. Real GDP per capita increases by 0.79% for one percentage increase in level of investment proxied by GFCF. The variables FDI and enrolment, though statistically insignificant, are positively associated with real per capita GDP in the long run. Homicide rates were found to exert adverse impacts on real per capita GDP in the long run. In Equationequation (2)
(2)
(2) , real per capita GDP and exports have a significant positive impact on GFCF in the long run. A one percentage increase in real per capita GDP and exports led to an increase in domestic investment by 0.97% and 0.38%, respectively. Homicide rates and investment (GFCF) were found to be negatively associated, though statistically insignificant, in the long run. Surprisingly, FDI seems to be negatively associated with investment in the long run. This corroborates the findings of other studies such as Adams (Citation2009) and Lean and Tan (Citation2011) that have argued for the crowding out effect of FDI on domestic investment. In Equationequation (3)
(3)
(3) , all variables except the homicide rate are statistically significant in the long run. The positive association between real per capita GDP and inward FDI conforms to the findings of Fabry and Zeghni (2002), who argued that countries witnessing outstanding growth tend to experience large FDI inflows. The results from Equationequation (4)
(4)
(4) reveal that all variables are statistically significant in the long run. Homicide rates negatively impact real exports of goods and services in the long run. A one percentage increase in the homicide rate leads to a decrease in real exports by 0.51% in the long run. The finding that homicide rates negatively impact real exports supports the findings of Anderson et al. (2022), which found a significant negative relationship between homicide rates and bilateral international trade.
Table 4. Estimated long-run coefficients from restricted error correction models
A statistically significant error correction term (ECT (−1)) determines the presence of long-run Granger causality running from the independent variables to the dependent variable in the error correction models. The negative sign of the coefficients in the error correction terms implies a monotonic convergence to long run cointegrating equilibrium. In Table , we present the short-run coefficients from estimating the restricted error correction models.
Table 5. Estimated coefficients (short run) from Error correction models
The coefficients of the error correction term indicate the adjustment speed of the system to return to long-run equilibrium in the event of any short-term imbalances. For instance, the coefficient in EquationEquation (1)(1)
(1) , which is equal to 0.24, denotes that approximately 24% of the disequilibrium caused by the shock of the previous year returns to the long-run equilibrium in the current year. All other coefficients of the error correction term can be interpreted on similar lines. As shown in Table , homicide rates have a significant negative impact on real per capita GDP and real exports of goods and services in the short run, at the 1% and 10% levels of significance, respectively. The finding that homicide rates have a negative impact on real per capita GDP in the short run corroborates the findings of previous studies (A. Ahmad et al., Citation2014; Detotto & Pulina, Citation2013; Kumar, Citation2013; Peri, Citation2004). Real gross-fixed capital formation has a significant positive impact on real per capita GDP in the long-run and in the short-run. This finding is consistent with the arguments that domestic investment has been the primary driver of economic growth in the Indian economy. There is an evidence of short-run bi-directional causality between the real value of exports of goods and services and domestic investment (GFCF). The findings suggest that real exports and domestic investment have a reinforcing relation between them. A higher level of domestic investment increases the nation’s capacity for exports, and, in turn, this increased level of exports has positive spillovers to domestic investment. The short-run results indicate that the existing economic situation (real per capita GDP) is an important factor for attracting inward FDI.
Post-estimation, a range of diagnostic tests were run to check the stability of all the estimated models and the robustness of their results. The diagnostic checks ensure that the coefficients and variances of disturbance terms remain time invariant (Pesaran and Pesaran, 2009). A Breusch-Godfrey serial correlation test was performed to check for correlations among the residuals obtained from the models, the test rejects the presence of serial correlations up to two lags at 1% level of significance. The Jarque-Bera test for normality of residuals does not reject the null hypothesis of normality; hence, the residuals obtained from the models are normally distributed. The evidence of heteroskedastic residuals is rejected from the ARCH heteroskedasticity test and the Breusch-Pagan-Godfrey test. Thus, the residuals obtained from the models are homoscedastic and non-auto correlated. The RESET test suggested by Ramsey (Citation1969) was used to check for functional form misspecification of the models. The test results do not reject the null at the 1% level of significance under the null hypothesis of no misspecification for all the models. To test the model’s structural stability, the cumulative sum of recursive residuals (CUSUM) test and the cumulative sum of the square of recursive residuals (CUSUMQ) tests were conducted. The structural coefficients were within the confidence intervals for the entire study period. We have only presented the CUSUM and CUSUMQ tests for EquationEquation (1)(1)
(1) in Figures . The diagnostic tests are presented in Table .
Table 6. Results of Diagnostic Tests
6. Conclusions
This study investigates the empirical relationships between crime rate and economic growth, controlling for other determinants of growth, in the Indian economy from 1990 to 2019. To this end, a bounds test of cointegration within an ARDL framework is employed to analyze long run as well as short-run dynamic relationships among the variables. The diagnostic tests revealed that the results obtained from the models were robust and stable. A fresh understanding of the interdependencies between economic growth and crime rates was advanced in this study. Crime rate, proxied by homicide rates, along with investment, FDI, exports, and enrolment granger cause real per capita GDP in the long run. The negative impact of homicides on economic growth was more pronounced in the short run. A one percentage increase in the growth rate of homicide rates led to 0.25% decrease in economic growth in the Indian economy. Moreover, a unidirectional causality was found running from homicide rates to real values of exports both in the long run and the short run. Given that developing countries place a strong focus on growing their relative export share in the global trade, the finding that homicide rates and real exports are inversely related to each other is significant. Our findings corroborate the hypothesis of a significantly negative impact of crime on economic growth. Crime acts as a deterrent to economic activities, decreases the ease of doing business, and discourages investment—all factors that contribute to economic growth. Homicides threaten social cohesion in a society in addition to having negative effects on economic development. A high level of homicide rates is an indicator of the extent of violent crimes in a society. It undermines citizens’ confidence in the rule of law, erodes trust in law enforcement agencies, and in certain extreme circumstances can even lead to outmigration of individuals. The negative consequences of homicides can be severe for developing countries that already reel under the pressure from poor infrastructure and low-income levels. For a developing country such as India, the loss of economic growth due to criminal activities is distortionary in nature. The level of investment, as measured by real gross-fixed capital formation, is found to have a significantly positive impact on real per capita GDP for the Indian economy, both in the short run and long run. Homicide rates are found to be negatively associated with the investment level in the long run. An increase in homicide rates crowds out public investment because resources that could have been used for productive purposes are instead diverted for protective purposes.
The empirical findings of a significant negative impact of homicides on real per capita GDP and other determinants of economic growth demand urgent attention from policymakers to frame policies that reduce criminal activity in India. From a policy standpoint, some suggested actions include the following: First, it is necessary to implement policies that raise the opportunity costs associated with crime. Examples include improving law enforcement agencies’ access to better tools and facilities, increasing the probability of detection of crimes, and increasing the conviction rate of homicides. India lags far behind in conviction rate for homicides. Some poorer states like Bihar and Uttar Pradesh have conviction rates in the range of 10–12%. Increases in the likelihood of detection and conviction rates for homicides that increase the opportunity costs of crime will go a long way toward lowering criminal activity in the Indian economy. Second, given that each state in India’s federal system is responsible for maintaining law and order, it is important to identify the states that have witnessed exceptionally high homicide rates. An inter-state comparison might help states with high homicide rates benefit from the efforts of those states that have successfully reduced their homicide rates. From a policy perspective, it would be interesting for future research to conduct an empirical examination of (i) the macroeconomic factors affecting homicide rates in India and (ii) the efficacy of deterrence variables on homicide rates in the Indian economy.
Ethical Approval And Informed Consent Statements
This article does not include any studies with human participants performed by any of the authors.
Disclosure statement
No potential conflict of interest was reported by the authors.
Data availability statement
Data is available through the official website of the NCRB (National Crime Records Bureau), Government of India. Retrieved data on 22 January 2023. www.ncrb.gov.in
Notes
1. The report can be accessed via the following link: https://www.visionofhumanity.org/maps/#/.
2. Cognizable offenses are such offenses in which the investigating authority can arrest the accused without an arrest warrant. Such crimes are usually serious in nature and come within the jurisdiction of courts.
3. Thomas Hobbes, one of the great philosophers, proposed the two natural laws that form the foundation of the society. Individuals enter into contract with a sovereign to protect and uphold these natural laws. Early social contract theories first appear with the development of his theory of natural law.
4. The erstwhile Ministry of Education had an annual publication “Education in India”, the enrolment data has been taken from these publications. The enrolment data for recent years (2003 onward) come from “Educational Statistics at Glance” from the digital archives of National Institute of Educational Planning and Administration.
5. Homicide rate is defined as number of homicides per 100,000 population and is calculated as:(Total number of homicides/Estimated mid-year Population) * 100,000.
References
- Acemoglu, D., Johnson, S., & Robinson, J. (2005). Institutions as fundamental cause of long-run growth. Handbook of Economic Growth, 1A, 386–16.
- Adams, S. (2009). Foreign Direct investment, domestic investment, and economic growth in Sub-Saharan Africa. Journal of Policy Modeling, 31(6), 939–949. https://doi.org/10.1016/j.jpolmod.2009.03.003
- Adekoya, A. F., & Razak, N. A. (2017). The dynamic relationship between crime and economic growth in Nigeria. International Journal of Management and Economics, 53(1), 47–64. https://doi.org/10.1515/ijme-2017-0004
- Ahmad, A., Ali, S., & Ahmad, N. (2014). Crime and economic growth in developing countries: Evidence from Pakistan. Journal of Basic and Applied Scientific Research, 4, 31–41.
- Anser, M. K., Yousaf, Z., Nassani, A. A., Alotaibi, S. M., Kabbani, A., & Zaman, K. (2020). Dynamic Linkages between poverty, inequality, crime, and social expenditures in a panel of 16 countries: Two-step GMM estimates. Journal of Economic Structures, 9(1). https://doi.org/10.1186/s40008-020-00220-6
- Asoni, A. (2008). Protection of Property Rights and Growth as Political Equilibria. Journal of Economic Surveys, 22(5), 953–987. https://doi.org/10.1111/j.1467-6419.2008.00554.x
- Baharom, M. (2009). Crime and economic conditions in Malaysia. International Journal of Social Economics, 1071–1081.
- Barro, R. (2000). Rule of law, democracy and economic performance. Index of Economic Freedom.
- Becker, G. (1968). Crime and Punishment. The Journal of Political Economy, 76(2), 169–217. https://doi.org/10.1086/259394
- Belloumi, M. (2013). The relationship between trade, FDI, and economic growth in Tunisia. Economic Systems.
- Benhabib, J., & Spiegel, M. M. (1994). The role of human capital in economic development evidence from aggregate cross-country data. Journal of Monetary Economics, 34(2), 143–173. https://doi.org/10.1016/0304-3932(94)90047-7
- Brand, S., & Price, R. (2000). The economic and social cost of Crime. Home Office Research Studies.
- Cardenas, M. (2007). Economic growth in Columbia: A reversal of fortune? Ensayos sobre Política Económica, (53), 220–259. https://doi.org/10.32468/Espe.5305
- Chatterjee, I., & Ray, R. (2009). Crime, Corruption and Institutions. Monash Economics Working Papers 20-09, Monash University, Department of Economics.
- Cloward, R., & Ohlin, L. (1960). Delinquency and Opportunity. The Free Press.
- Debnath, A., & Das, S. (2017). Relationship between crime and economic affluence in India: An empirical study from 1982 to 2013. Journal of Criminological Research, Policy and Practice, 3(1), 27–37.
- Detotto, C., & Otranto, E. (2010). Does Crime affect Economic Growth. KYKLOS, 63(3), 330–345. https://doi.org/10.1111/j.1467-6435.2010.00477.x
- Detotto, C., & Pulina, M. (2013). Does more crime mean fewer jobs and less economic growth? European Journal of Law and Economics, 36(1), 183–207. https://doi.org/10.1007/s10657-012-9334-3
- Draca, M., & Machin, S. (2015). Crime and Economic Incentives. Annual Review of Economics, 7(1), 389–408. https://doi.org/10.1146/annurev-economics-080614-115808
- Dreze, J., & Khera, R. (2000). Crime, gender, and society in India: insights from homicide data. Population and Development Review, 26(2), 335–352. https://doi.org/10.1111/j.1728-4457.2000.00335.x
- Dutta, M., & Husain, Z. (2009). Determinants of crime rates: Crime Deterrence and Growth in post-liberalized India. MPRA Paper 14478. Germany: University Library of Munich.
- Ehrilch, J. (1973). Participation in Illegitimate activities: A theoretical and empirical investigation. The Journal of Political Economy, 81(3), 521–565. https://doi.org/10.1086/260058
- Enamorodo, T., Lopez-Calva, L. F., & Rodriguez-Castelan, C. (2014). Crime and growth convergence: Evidence from Mexico. Economics Letters, 125(1), 9–13. https://doi.org/10.1016/j.econlet.2014.07.033
- Engle, R. F., & Granger, C. W. (1987). Co-Integration and Error Correction: Representation, Estimation, and Testing. Econometrica, 55(2), 251–276. https://doi.org/10.2307/1913236
- Forni, M., & Paba, S. (2000). THE sources of local growth: Evidence from italy. Giornale degli Economisti e Annali di Economia, 59(1), 1–48.
- Ghatak, S., Milner, C., & Utkulu, U. (1997). Exports, export composition and growth: Cointegration and causality evidence for Malaysia. Applied Economics, 29(2), 213–223. https://doi.org/10.1080/000368497327272
- Goulas, E., & Zervoyianni, A. (2012). Economic growth and crime: does uncertainty matter? Applied Economics Letters, 20(5), 420–427. https://doi.org/10.1080/13504851.2012.709596
- Greenbaum, R. T., & Tita, G. E. (2004). The Impact of Violent Surges on Neighborhood Business Activity. Urban Studies.
- Haggard, S., & Tiede, L. (2011). The Rule of law and economic Growth: Where are we? World Development, 39(5), 673–685. https://doi.org/10.1016/j.worlddev.2010.10.007
- Hanushek, E. A. (2013). Economic growth in developing countries: The role of human capital. Economics of Education Review, 37, 204–212. https://doi.org/10.1016/j.econedurev.2013.04.005
- Harris, R., & Sollis, R. (2003). Applied time series modeling and forecasting. Wiley.
- Islam, A. (2014). Economic growth and crime against small and medium sized enterprises in developing economies. World Bank Policy Research Working Paper No 6768.
- Jennings, W., Farrall, S., & Bevan, S. (2012). The economy, crime and time: An analysis of recorded property crime in England & Wales 1961–2006. International Journal of Law, Crime and Justice, 40(3), 192–210. https://doi.org/10.1016/j.ijlcj.2012.03.004
- Johansen, S. (1991). Estimation and Hypothesis Testing of Cointegration Vectors in Gaussian Vector Autoregressive Models. The Econometric Society, 1551–1580.
- Kaushik, K. K., & Klein, K. K. (2008). Export growth, export instability, investment and economic growth in India: A time series analysis. Journal of Developing Areas, 41(2), 155–170.
- Keefer, P. (2007). Insurgency and Credible Commitment in Autocracies and Democracies. World Bank Policy Research Working Paper No. 4185.
- Kumar, S. (2013). Crime and Economic Growth: Evidence from India. MPRA Paper 48794. Germany: University Library of Munich.
- Lean, H., & Tan, W. (2011). Linkages Between Foreign Direct Investment, Domestic Investment and Economic Growth in Malaysia. Journal of Economic Cooperation and Development, 2(4), 75–96.
- Lee, D. J., & Son, J. C. (2016). Economic Growth and Income Inequality: Evidence from Dynamic Panel Investigation. Global Economic Review, 45(4), 331–358. https://doi.org/10.1080/1226508X.2016.1181980
- Masih, A. M., & Masih, R. (1996). Temporal causality and the dynamics of different categories of crime and their socioeconomic determinants: Evidence from Australia. Applied Economics, 1093–1104.
- Mauro, L., & Carmeci, G. (2007). A poverty trap of crime and unemployment. Review of Development Economics, 11(3), 450–462. https://doi.org/10.1111/j.1467-9361.2006.00350.x
- Mulok, D., Kogid, M., Lily, J., & Asid, R. (2016). The Relationship between crime and economic growth in Malaysia: re-examine using bound test approach. Malaysian Journal of Business and Economics, 3(1), 15–26.
- Naddeo, A. (2014). How crime affects the economy: Evidence from Italy. Munich Personal RePEc Archive.
- Narayan, P. K., & Smyth, R. (2004). Crime rates, male youth unemployment and real income in Australia: Evidence from Granger Causality Tests. Applied Economics, 2079–2095.
- North, D. C. (1991). Institutions. Journal of Economic Perspectives, 5(1), 97–112. https://doi.org/10.1257/jep.5.1.97
- Parida, Y., Bhardwaj, P., & Chowdhury, J. (2017). Effects of economic development and crime on tourism in India. Anatolia, 29(1), 63–73. https://doi.org/10.1080/13032917.2017.1384742
- Pellegrini, L., & Gerlagh, R. (2004). Corruption’s Effect on Growth and Its Transmission. Kyklos, 57(3), 429–456. https://doi.org/10.1111/j.0023-5962.2004.00261.x
- Peri, G. (2004). Socio-cultural Variables and Economic Success: Evidence from Italian Provinces. Topics in Macroeconomics, 4(1), 1–34. https://doi.org/10.2202/1534-5998.1218
- Pesaran, M. H., Shin, Y., & Smith, R. J. (2001). Bounds Testing Approach to the analysis of level relationships. Journal of Applied Econometrics, 16(3), 289–326. https://doi.org/10.1002/jae.616
- Phillips, P. C., & Ouliaris, S. (1990). Asymptotic Properties of Residual Based Tests for Cointegration. Econometrica, 58(1), 165–193. https://doi.org/10.2307/2938339
- Posner, R. A. (1981). The Economics of Privacy. The American Economic Review, 71(2), 405–409.
- Raj, P., & Rahman, M. (2023). Revisiting the economic theory of crime : A state-level analysis in India. Cogent Social Sciences.
- Ramsey, J. (1969). Tests for Specification Errors in Classical Linear Least Squares Regression Analysis. Journal of the Royal Statistical Society, 31(2), 350–371. https://doi.org/10.1111/j.2517-6161.1969.tb00796.x
- Sandler, T., & Enders, W. (2008). Economic Consequences of Terrorism in developed and developing countries: An Overview. In P. Keefer & N. Layza (Eds.), Terrorism, Economic Development and Political Openness (pp. 17–47). Cambridge University Press.
- Sen, A. (2000). Development as Freedom. Anchor Books.
- Shaw, C. R., & McKay, H. D. (1942). Juvenile Delinquency and Urban Areas. The University of Chicago Press.
- Tang, C. (2009). The Linkages among Inflation, Unemployment. International Journal of Economics & Management, 7(18), 50–61.