Abstract
Combinatorial knowledge dynamics and recombinant innovation have received increasing attention. So far, however, empirical studies have insufficiently focused on how firms utilize multi-scalar sources to facilitate the combination of analytical and synthetic knowledge, which is a gap we would like to fill with the help of this paper. Through interviews with high-end medical device companies from Shanghai, this paper analyzes the role of proximity in firms’ combinatorial knowledge base (CKB) creation from a multi-scalar perspective, offering insights into the geography of knowledge. Moreover, cutting-edge technologies and complex innovation in the manufacturing field are often observed in the high-end medical device industry. Unravelling the creation of CKB in such an industry in Shanghai could help us better understand emerging economies.
1. Introduction
Within economic geography, combinatorial knowledge dynamics and recombinant innovation activities have been receiving increased attention (Grillitsch et al., Citation2018; Mewes, Citation2019; Nieth & Benneworth, Citation2020). The related studies have shown that in the process of knowledge evolution or KB combination, more and more actors from different fields and spatial scales participate in the innovation projects, which in turn leads to a more complex knowledge interaction process (Isaksen & Trippl, Citation2017; Mazzucchelli et al., Citation2019). This complexity can be understood as the complex geography of knowledge interaction, namely multi-scalar combinatorial knowledge creation. However, there is a limited understanding of how do firms utilize innovative sources at different spatial scales to promote combinatorial knowledge creation.
Since places are embedded in a socio-political structure, characterized by specific institutional and economic contexts (Strambach, Citation2017; Trippl et al., Citation2018), multiple factors explain combinatorial knowledge dynamics. In this sense, analyzing the characteristics of combinatorial knowledge interaction from a multiple perspective is necessary.
The proximity framework is expected to reflect the relationships between interacting partners from a multiple perspective and hence is widely applied in knowledge creation and innovation networks (Balland et al., Citation2015; Boschma, Citation2005; Musil & Eder, Citation2016). However, till now, studies on the role of proximity in combinatorial knowledge creation and recombinant innovation are not satisfactory.
On the one hand, in quantitative research, although scholars have long been interested in the impacts of proximity on collaborations (Hansen, Citation2015; Marek et al., Citation2017), they rarely distinguish the types of innovative products according to their different knowledge fields. However, recently several researchers swift to analyze recombinant knowledge from a proximity perspective. For example, Li et al. (Citation2021) examined how geographical proximity facilitates unrelated technological combinations and breakthrough discoveries in the green industry.
On the other hand, in the qualitative study, although Capone and Lazzeretti (Citation2018) identified the impacts of proximity on KBs, which has not been done to the same extent in the CKB literature, particularly for the CKB with codified and tacit attributes. Combinatorial knowledge base (CKB), a combination of knowledge bases (KBs), offers a theoretical lens for this topic (Boschma, Citation2018). In comparison to the studies evaluating recombinant knowledge based on patents, the CKB concept offers a broader view to understand complex knowledge creation dynamics that underlie complex innovation processes (Asheim et al., Citation2017). A typical case is the CKB based on analytical and synthetic knowledge, which prevails in advanced manufacturing industries.
Moreover, although the CKB concept provides insights into combinatorial knowledge dynamics and innovation studies, so far, the empirical literature on CKB mainly focuses on cases regarding advanced and lagging cities/regions of industrialized countries (Northern Europe and Central Europe; Southern Europe) (Bennat & Sternberg, Citation2020; Grillitsch et al., Citation2017; Marques, Citation2019). However, multi-scalar CKB in advanced and lagging cities/regions of emerging economies and developing countries has been hardly studied so far. In comparison to industrialized countries, emerging economies and developing countries have a shorter history in combining KBs and cross-domain R&D. Therefore, it is worthwhile to analyse how advanced and lagging cities of emerging economies and developing countries utilize innovative sources at different spatial scales to develop cutting-edge technologies in terms of CKB. However, few scholars zoom in on this point.
To fill these gaps, this paper aims at analyzing the role of proximity in CKB regarding analytical and synthetic knowledge from a multi-scalar perspective in the high-end medical device industry in Shanghai, through interviews with local companies. This study will respond to the question: how do firms utilize multi-scalar innovative sources to develop CKB characterized by analytical and synthetic knowledge? So why choose the Shanghai high-end medical device industry? The medical device industry is a multi-disciplinary, knowledge-intensive and capital-intensive high-tech industry (Davey et al., Citation2011; Zhao et al., Citation2018), where joint R&D prevails (Bergsland et al., Citation2014). The reason why we choose the medical device industry is so we can expect a combination of several knowledge bases, as well as knowledge interaction across space in this industry. In order to analyze and understand the case, we construct a new framework in which multi-scalar CKB, as well as several proximities, are taken into account.
To conclude, this paper investigates the role of proximity in firms’ CKB creation at different spatial scales (Boschma, Citation2018), providing insights into the geography of knowledge (Shearmur et al., Citation2016). Furthermore, although firms’ CKB and related innovation activities have been addressed in existing studies regarding the food and beverage industry, cutting-edge technologies and complex innovation in the manufacturing field are often observed in the high-end medical industry. In such an industry, how CKB is created and how it happens in developed cities in emerging countries are interesting questions. By answering these questions, this paper contributes to studies on emerging economies.
This paper is structured as follows. In the next section, we elaborate on the literature about the role of proximity in the combination of knowledge (bases). Section 3 presents the case study, which consists of the specific industrial and regional-industrial context, the methodological approaches, and the empirical analysis of the medical device industry in Shanghai. Concluding remarks are presented in Section 5.
2. Theoretical analysis
In this section, we will review the literature about DKB and proximity in Section 2.1, and discuss the potential linkages between firms’ CKB and proximity in diversify innovation projects in Section 2.2.
2.1. DKB and proximity
According to Boschma (Citation2018, p. 24), the DKB literature elaborated “ … the nature of knowledge sharing and its geographical extent which were claimed to vary between analytical, synthetic and symbolic knowledge bases … ”, and is hence a good example of context-sensitive theorizing in economic geography (Gong & Hassink, Citation2020). The original theoretical literature states that analytical knowledge plays a vital role in the process of science-based industries characterized by radical innovation, such as the pharmaceutical and biotechnology industries (Asheim & Gertler, Citation2005). In contrast, synthetic knowledge mainly refers to incremental innovation for artefact engineering and construction industries (Asheim & Gertler, Citation2005; Bathelt et al., Citation2004; Yew, Citation2021). Symbolic knowledge is strongly related to cultural codes within the cultural and creative industries, in which innovative activities are based on the combination of existing knowledge (Manniche & Larsen, Citation2013). If we compare the three different kinds of knowledge, analytical knowledge is the easiest to absorb or transfer. In comparison to other distinctions and approaches such as high- vs. low-tech and codified vs. tacit knowledge, the KB approach is strongly connected to knowledge creation and innovation processes taking place within industries (Asheim & Gertler, Citation2005).
The literature on proximity started to grow in the 1990s with the aim to explore the coordination of industrial activities. The French proximity school first pointed out, in addition to the geography of actors, a non-geographical dimension, namely organizational proximity, should be embodied in the proximity analysis (Bellet et al., Citation1993; Rallet & Torre, Citation1998). In their view, not only the physical distance between collaborators but also the close relationship of actors has the potential to facilitate or hinder knowledge collaborations (See Table ).
Table 1. The definition of five dimensions of proximity
Over time they added the institutional dimension to the proximity framework in order to explain the phenomenon of how institutional environments affect, shape and reshape knowledge interactions and innovative networks between agents (Carrincazeaux et al., Citation2001). Additionally, other dimensions of proximity, such as social, cognitive, technological and interpersonal proximity, have been receiving increased attention and are widely applied in economic geography as well as related social science domains (Guan and Yan, Citation2016; Sabbado et al., Citation2021).
Scholars have tried to summarize, integrate and compare different dimensions of proximity. Boschma (Citation2005) summarized and integrated the above-mentioned literature through establishing a comprehensive analytical framework, where five types of proximities are included, i.e., geographical, organizational, institutional, social and cognitive proximity. Moreover, some researchers have been much concerned with the relationship between different dimensions of proximity such as substitution and overlap (Hansen, Citation2015). Last but not least, Balland et al. (Citation2015, p. 8) explored the co-evolution of proximity and knowledge network, elaborating that five types of dimensions may vary over time because of “the processes of learning, decoupling, institutionalization, integration and agglomeration”. Overall, proximity provides an in-depth understanding of knowledge interactions and innovation networks (Boschma, Citation2005; Davids & Frenken, Citation2018; Marek et al., Citation2017).
The role of proximity in industry-level DKB has prevailed in economic geography and innovation studies in the last decade (Mattes, Citation2012). Analytical knowledge, characterized by science models and codified attributes, is easy to disseminate across space and thus is not sensitive to geographical distances (Asheim & Gertler, Citation2005). Organizational and cognitive proximity is crucial in non-local knowledge transfer because science knowledge diffusion needs professional research staff with a professional education background. For example, Huawei Corp. in Shenzhen (a latecomer firm in China) actively collaborates with the Technische Universität München (TUM) and the RWTH Aachen in Germany because these two organizations specialize in high-quality electronics (Liefner et al., Citation2019).
Synthetic knowledge creation depends stronger on face-to-face communication and local buzz, where geographical proximity matters (Asheim et al., Citation2007), and collaborators share values in the same institutional environment. Such close communications are often built via social ties and cognitive proximity (Mattes, Citation2012). At the non-local level, organizational proximity plays a decisive role in cross-region or cross-country synthetic knowledge interactions because of the geographically sensitive character of synthetic knowledge per se.
Symbolic knowledge is strongly associated with territorial culture and arts and relies on geographical proximity. Culture, values, consumers’ preferences, and recognition work together to create symbolic knowledge. Therefore, institutional and cognitive proximity may affect the related innovation process. However, for globalization-oriented products, the impact of formal and informal institutions on symbolic knowledge creation becomes less than that of non-global products (Mattes, Citation2012).
The role of proximity in firm-level KBs in the different innovation projects and different stages is visible. Davids and Frenken (Citation2018) explored Unilever’s Becel diet margarine, finding that institutional and cognitive proximity matters in the research stage as scientific research and academic collaborations (analytical KB) are prevalent. In the developmental stage, institutional, geographical, and organizational proximity affect knowledge production and practices (synthetic KB). In the marketing stage of products, the territorial cultural elements dominate related innovation processes, influenced mainly by geographical and cultural proximity.
The DKB approach and proximity analysis are criticized because the generation of KB only refers to one kind of actor specializing in scientific analyses or engineering or art and culture and is more static (Asheim et al., Citation2011). Although Davids and Frenken (Citation2018) advanced knowledge dynamics in the firm evolutionary process, they still zoom in on how proximity contributes to single KB in different innovation projects and different stages. However, within the same stage, how proximity promotes combinations of KBs in the same project has not been sufficiently investigated.
The following section will discuss the potential linkages between firms’ CKB and proximity in the same innovation projects in the same stage.
2.2. Firms’ CKB, proximity and innovation process
CKB is the combination of intra-KB or inter-KB leading to innovation (Asheim et al., Citation2017; Manniche, Citation2012), which offers a more nuanced insight into knowledge flows and innovation characteristics of regional industries. They can help to understand under which conditions combinations between intra-KB or inter-KB matter most for innovation in regional firms and industries (Bennat & Sternberg, Citation2020; Marques, Citation2019).
Intra-KB combinations include combinations of analytical KBs, combinations of synthetic KBs, and combinations of symbolic KBs. Intra-KB combinations are codified or tacit because of only one type of KB (Asheim et al., Citation2007, Citation2011). The codified or tacit of CKBs attribute could reflect the geographical sensitivity.
Inter-KB combinations often refer to three types: combinations of analytical and synthetic KBs, combinations of symbolic and synthetic KBs, and combinations of analytical, synthetic, and symbolic KBs. In addition to combinations of symbolic and synthetic KBs with tacit attributes, other inter-KB combinations may have mixed attributes because two types of KBs with different attributes are involved.
Knowledge similarities and heterogeneity between firms within the same industry lead to vertical and horizontal knowledge links (Turkina et al., Citation2021). One firm may participate in several innovative projects and have different knowledge linkages.
In the same stage, the whole attribute of CKBs with codified and tacit characteristics in the same innovative project is vague. Little literature analyzes the potential linkages between CKBs with codified and tacit characteristics and proximity.
Combinations of analytical and synthetic KBs are highly agglomerated in metropolitan areas and industrialized regions (Balland et al., Citation2020; Strambach, Citation2017). Firms benefit from strong and comprehensive innovation systems, a large-sized market and skilled labor, multiple position mobility (multiple working experiences in different organizations), and various innovation collaboration projects (Balland et al., Citation2020; Broekel & Youn, Citation2019; Grillitsch & Hansen, Citation2019).
At the local level, geographical proximity plays a significant role in collaborations based on analytical & synthetic knowledge combinations because innovation activities including synthetic knowledge are done by face-to-face communication and local buzz (Asheim et al., Citation2017).
Knowledge interactions are a social-spatial process (Boschma, Citation2005), which means innovation processes regarding combinations of analytical and synthetic knowledge are also embedded in local social networks and institutional environment (Strambach, Citation2017; Wagner & Growe, Citation2022).
Note that the regional institutional environment reflects how complex innovation and the combination of knowledge (bases) are treated. Firm-level actors generally prefer to choose partners who follow the same rules, regulations and policies (formal institutions) when these partners could offer related R&D requirements. In addition, if collaborators share common norms, values and culture (informal institutions), they are also a top priority for firm-level actors.
At the non-local level, for collaborations with synthetic and analytical knowledge, organizational proximity may be an essential alternative to geographical proximity (Figueiredo et al., Citation2020). On the one hand, establishing sub-organizations is beneficial to absorbing cross-region or cross-county synthetic knowledge because synthetic knowledge is susceptible to geographical distances. On the other hand, the recombinant process of analytical and synthetic knowledge is always accompanied by high uncertainty (Bennat & Sternberg, Citation2020). If interacting collaborators are in the same organizational branches and follow the same “organizational arrangements,” they could work together with a high-level synergy and reduce uncertainties (Capone & Lazzeretti, Citation2018). In other words, organizational closeness can potentially reduce the risk of non-local collaborations and accelerate knowledge combinations between subsidiaries.
At the non-local level, combining knowledge creation is also very cognitively demanding due to complex R&D process for analytical knowledge and mutual technical support for synthetic knowledge (Tödtling & Grillitsch, Citation2015). In such an innovation process, both interacting partners absorb knowledge from each other and finally contribute to recombinant knowledge across space (Franco & Esteves, Citation2020).
Organizations in different metropolitan areas often interact mutually and complement each other and hence are the main contributors to knowledge combination and cross-space diffusion. These metropolitan regions may be in the same or different countries.
For cross-country CKB flows, social proximity may matter because social ties are linked to trust and friendship (Broekel & Boschma, Citation2012; Martin et al., Citation2017) which shape, strengthen and solidify the combination of analytical and synthetic knowledge. Such a solidify tie bring about a greater sense of identity among actors in advanced knowledge creation processes and smaller frictions than collaborations without social ties.
3. Case study
After describing the literature about the role of proximity in the combination of knowledge (bases), we will now analyze CKB across space and proximity in the high-end medical device industry in Shanghai. We will first introduce the industrial and regional-industrial context, then we will describe the methods, which will be followed by the empirical analysis.
3.1. Research context
The medical device industry is a multi-disciplinary, knowledge-intensive industry (Davey et al., Citation2011; Zhao et al., Citation2018), which refers to among others mathematics, physics, biology, engineering, and electronics. Therefore, in the process of R&D, cross-disciplinary collaborations are the main way of product innovation. Moreover, enterprises in this industry are mainly funded by themselves or venture investments.
According to international regulations, medical devices can be divided into three categories (Kramer et al., Citation2012). Class I refers to medical devices that are safe and effective. Class II refers to medical devices for which safety and effectiveness can be controlled. Class III (the most stringent device management) includes those that are potentially dangerous to the human body, and the safety and effectiveness, therefore, must be strictly controlled (Kramer et al., Citation2012). In our paper, we are only concerned with high-end medical devices referring to Class II and Class III medical devices, for reasons, we will explain below.
Concerning the regional context, Shanghai, in addition to being the country’s centre for international economy, finance, trade and shipping (Shen & Li, Citation2014), is characterized by a high concentration of rich innovation sources, including organizations such as universities and public and private research institutes (Chen et al., Citation2017). In order to build Shanghai into a global technology innovation centre (Du, Citation2018), the Shanghai government has developed policies to facilitate advanced knowledge creation and innovation, contributing to the development of local high-tech industries (Cao et al., Citation2019; Zeng et al., Citation2011). In 2019, the Shanghai Bureau of Statistics claimed that six key manufacturing industries contributed to 2327.915 billion yuan industrial output value, which accounts for more than 67.6% of the city’s total. These six industries include electronic information, automobile, petrochemical and fine chemical manufacturing, fine steel, biopharmaceutical manufacturing (including medical devices) and complete equipment/complete set of equipment, such as industrial machinery.
Shanghai is one of the earliest cities in China developing medical device industries, having a strong historical endowment. After the establishment of new China in 1949, Shanghai Medical Instrument Factory and Shanghai Precision Instrument Factory jointly successfully developed the first 200 mA medical X-ray machine in China in 1952, which can be seen as a milestone in the history of medical devices in China (China Medical Device Industry Association www.camdi.org/news/8416). After a long period of slow growth, more recently the Shanghai high-end medical device industry has developed into a strong agglomeration (See Table ).
Table 2. The number of main agglomeration areas relevant to the Shanghai high-end medical device industry
Over time, the Shanghai medical device industry cluster has become a significant contributor to the innovativeness of the national medical device industry. First, the number of Shanghai’s medical device company patents granted has exceeded 10,000 by the end of 2019, ranking second in the whole country, which accounted for about 6% of the nation’s total (see Table ). Secondly, the number of Shanghai’s very innovative medical devices was 44 by the end of 2019, which accounted for about 20% of the nation’s total (see Table ). The latter has gone through a special review organized by the China Food and Drug Administration, which will be introduced in the last paragraph in Section 5.1.
Table 3. Detailed information about medical device industry in the end of 2019
As Figure shows, seven areas are listed in the biomedical plan scheme in which five districts, including Pudong, Minhang, Fengxian, Songjiang and Xuhui districts, refer to high-end medical device industries. Our survey was conducted in these districts. According to the data from Zhongcheng medical device big data platform, by the end of 2019, 972 manufacturing medical device enterprises have been identified, of which 363 are regarded as innovative (see Table ).
Figure 1. Shanghai industrial planning map for biological medicine offered by the Shanghai government (see https://map.Sheitc.sh.gov.cn/#/map/hydt).
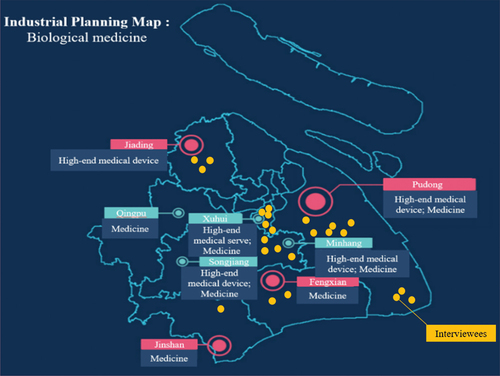
Recently, work on the Shanghai high-end medical device industry mainly focuses on two aspects. First, some work analyzes whether institutional entrepreneurship effectively promotes the development of the Shanghai high-end medical device industry. For example, Wang et al. (Citation2021) evaluated the effects of a special review process. Such a review is compulsory for medical device companies in China before launching a new product on the market. Only companies with patents and advanced products can apply for this special review process, which speeds up the time to the market. Xu et al. (Citation2019) analyzed the role of Marketing Authorization Holder (MAH), which is a national policy aiming at unbounding product registration and production license, which positively affected the high-end medical device industry in Shanghai. Secondly, some work focuses on the effects of “‘ … an industrial technological innovation alliance … ’” on the Shanghai high-end medical device industry (Shi, Citation2016, p. 12, in Chinese, translation by the author). Shi (Citation2016) showed that the establishment of this alliance supports enterprises to effectively obtain needed innovation resources, improving their innovation ability, which is beneficial to the technological innovativeness of the Shanghai high-end medical device industry.
4. Methods
In order to analyze the CKB, we decided to carry out a case study. Case studies are a preferred method to respond to “how” and “why” questions (Eisenhardt, Citation1989; Yin, Citation2003). Since we try to find answers to how and why questions concerning CKB at different spatial scales, we decided to use a qualitative case study as our method. The fieldwork was conducted in Shanghai from August 2020 to November 2020, and from September 2021 to October 2021. As Table shows, in total 24 experts were interviewed in this period (See Table ), consisting of 17 experts in enterprises, including six CEOs and 11 R&D staff (indicated as CR), three scholars and experts from universities and public research institutes (SE), and four officials (OF). CEOs and R&D staff(CR)from high-end medical device firms have rich experiences in R&D projects and participate in firms’ combining knowledge base collaborations as a leader or research staff. They are familiar with the detailed information on collaborations, as to why they choose those collaborators, and they can clearly describe how the collaboration develops. Scholars and experts (SE) come from universities and research institutions, having more than 10 years of working experience. They are familiar with the industry-university-research collaborations. In addition, these people have a macro knowledge of Shanghai’s advantages, other cities’ advantages in China and foreign advantages in this field. Official staff (OF) work in cluster organizations or the shanghai science & technology council, which endeavor to facilitate local knowledge creation and innovative activities, particularly in high-end manufacturing industries, through communicating with firms at certain times and providing relevant help for them. Therefore, they could offer related information to this point. These actors not only respond to our questions according to their own experiences but also introduce the general conditions. Based on these responses from interviewees and the general stories offered by these actors, data analysis and basic research consults have been done. The interviews lasted on average 45 minutes. The interview data were complemented with a large number of secondary data from various sources, including mainstream media reports, industrial reports and professional magazines (Table ).
Table 4. Detailed information around the survey of Shanghai high-end medical device industry
Table 5. Complementary materials
After introducing the survey and the related secondary sources, we will elaborate on the definition of proximity dimensions in this paper. First, we argue that within Shanghai geographical proximity means the distance between partners is less than 25 km; At the national level, geographical proximity matters if crucial partners are in Jiangsu Province and Zhejiang Province; At the international level, geographical proximity matters if crucial partners are in Japan, South Korea, North Korea, and Mongolia. Secondly, for what refers to the identification of cognitive, social, institutional, and organizational proximity, we followed closely the definitions by Davids and Frenken (Citation2018, p. 27) (see Table ).
4.1. Empirical analysis
In the following, we will present the results of our empirical analysis. The empirical analysis is introduced based on three spatial scales, namely, intra-Shanghai, Shanghai-other cities in China and Shanghai-global studies. According to the data statistic analysis, we found that the proportion of collaborators from Shanghai, other cities in China and international cities are about 3:1:2, which means Shanghai’s high-end medical device development is highly dependent on local and local-global sources. Detailed collaboration analyses are as follows.
4.1.1. Intra-shanghai analysis
After the end of the 20th Century, the central government has paid more attention to the development of medical devices and encouraged enterprise innovation through a related set of industrial policies. Partly because of this support, the number of medical device companies has been growing in Shanghai, locating in new districts, such as Pudong, Minhang, Fengxian, and Songjiang. Moreover, the number of public research institutes increased rapidly, significantly supporting industrial development.
These preconditions pay the way for national policy upgrading to develop the high-end medical device industry since 2013. National policies and regulations primarily refer to opinions on deepening the reform of the review and approval system to encourage innovation in drugs and medical devices (issued in 2017), the Twelfth Five-Year Plan for the Medical Device Technology Industry (work from 2011 to 2015) and Special Plan for Scientific and Technological Innovation of Medical Devices in the Thirteenth Five-Year Plan (work from 2016 to 2020).
Within Shanghai, there are by now about 50 universities, colleges and research institutes that refer to related disciplines or technologies, including biotechnology, medical science, artificial intelligence, mechanical engineering and electronics. The disciplines or technologies primarily relevant to analytical and synthetic knowledge (Cheong et al., Citation2020; Wu, Citation2007).
Among these, the R&D collaborations of the high-end medical device industry mainly include software engineering, electronic engineering and human factor engineering characterized by the combination of synthetic and analytical KB. Space agglomeration characteristics of this type of CKB are mostly influenced by high geographical embeddedness and tacit features of synthetic KB.
Geographical proximity facilitates knowledge interactions concerning software engineering, electronic engineering, and human factor engineering. An essential prerequisite for planning the Shanghai high-end medical device industrial park is its proximity to relevant universities, research institutes, and university-affiliated hospitals in Shanghai. Integrating industry, academia, research, and medicine provides favorable conditions. Given the requirement for face-to-face interaction in composite CKBs, companies tend to prioritize industrial parks near their partners, such as universities and research institutes (2019 Shanghai High-End Medical Device Industry Report).
“Knowledge interaction between software engineering and electrical engineering is crucial for CKB-related R&D during collaboration, necessitating frequent face-to-face communication. The two research groups typically provide detailed reports on CKB, share research progress, and discuss and prepare new plans for the next phase,” explained the R&D staff. Geographical proximity is vital in reducing unnecessary time costs for collaborators and contributes positively to local synergy.
“During R&D collaborations in human factors engineering, the primary purpose of communication between engineers and doctors is to enhance medical devices. Physicians document user needs, and occasionally, they may propose their solutions. As engineers, we evaluate the feasibility of the doctors’ proposed solutions, or we propose solutions based on user feedback and subsequently assess their feasibility with the doctor,” explained the two engineers from the startup.
“In this industry-academia-research-medicine collaboration, doctors working in hospitals and academics affiliated with universities and research institutes usually serve as the authors of research papers, while the company applies for the patents. Sometimes both partners become co-authors of the paper and co-holders of the patent,” explained a well-known team from Shanghai Jiaotong University.
Moreover, Shanghai’s institutions reflect how the combination of analytical and synthetic KB in the high-end medical device industry is treated (See Figure ). On the one hand, Shanghai’s inclusive innovative culture (informal institutions) plays a positive role in cross-domain R&D activities regarding software engineering, electronic engineering and human factor engineering (Action Plan for Promoting the High-Quality Development of Shanghai’s Biomedical Industry). Against this context, inventors are encouraged to do it whereas CEOs invest heavily in these fields.
On the other hand, preferential policies, regulations and relevant rules (formal institutions) given by cluster organizations or parks have become key advantages for Small and Medium enterprises (SMEs), large firms and start-ups to explore how analytical KB is combined with synthetic KB. In other words, institutional proximity is key in the process of KB combination.
For example, “The China (Shanghai) Pilot Free Trade Zone (CSPFTZ), to which many enterprises would like to move, gives key advantages concerning a reduction of import fees, financial subsidies of enterprise income tax, and value-added tax and individual tax adjustment, to medical device companies with CKB”, an official staff explained (Meng & Zeng, Citation2019).
Fudan Fenglin Science Park is another park giving advantages to SMEs and start-ups (Wu, Citation2007). According to the CEO of a firm located in Fudan Fenglin Science Park said, “Our company produces advanced combinatorial knowledge, received specialized financial subsidies, 300,000 yuan, from the Shanghai government and Fudan Fenglin Science Park in 2020, for being successfully listed as a Shanghai high-tech enterprise in 2020. According to him, being based in Fudan Fenglin Science Park helped us to receive such a big financial subsidy.”
Last, social ties reflect innovation cooperation networks, where social embeddedness based on trust and friendship contributes to the combination of inter-KB. Interviewees specializing in products’ R&D acknowledged that”finding reliable partners with similar cognitive levels through acquaintances can effectively reduce the risk of collaboration” (Sabbado et al., Citation2021).
An expert from Fudan University added, “Most CEOs and managers grew up or studied in Shanghai. Their friends, alumnus or relatives working in hospitals or engaging in high-end medical device industries provided some related knowledge resources in joint R&D and production. It means that social proximity facilitates CKB in the Shanghai high-end medical device industry. It is worth noting that collaborators are often very familiar with one type of KB, such as analytical or synthetic KB. Through mutual discussions and communications, both of them progress together and CKB is developed and then patented.”
4.1.2. Shanghai-other cities analysis in China
In order to utilize R&D sources of other cities in China to resolve problems relevant to implantable medical devices and diagnostic equipment mainly based on the combination of analytical and synthetic knowledge, the Shanghai high-end medical device industry actively seeks collaborations with firms or organizations outside the Shanghai area in China.
In such a process, high organizational proximity is viewed as a significant driver. KB combination is always accompanied by high risks and uncertainties, which can be reduced if partners follow the same organizational arrangements.
One R&D manager from Weichuang Co., Ltd. explained that “we have to choose intra-firm collaborations if those branches could meet their R&D requirements. Especially for large companies in Shanghai, it is a very common way to acquire some related medical device companies in other Chinese cities as their subsidiaries, thus promoting intra-company cooperation regarding CKB” (Shanghai high-end medical device industry report in 2019). He added, “For example, in 2012, Weichuang, a medical device company located in Zhangjiang High-Tech Park, Pudong, Shanghai, specializing in orthopedic and implantable medical devices acquired D-Pulse Medical (Beijing) Co., Ltd. with patents related to the stent” (Wang et al., Citation2021).
Note that at this level, regular communication and sending people to the cooperation unit for longer periods are easier to observe. Concerning the allocation of R&D benefits, it also follows the three forms observed within Shanghai mentioned above.
In addition, cognitive proximity makes the combination of analytical and synthetic KB possible. Highly qualitative products with CKB are easier to create if actors have decreased cognitive distances because of the same industrial and knowledge background.
For example, the Shenzhen Lianying High-end Medical Equipment Innovation Institute, established in 2019, is a private, non-profit organization jointly sponsored by Shanghai Lianying Medical Technology Co., Ltd. and the Shenzhen Institute of Advanced Technology, Chinese Academy of Sciences. It contributes to Shanghai Lianying’s R&D on software engineering and electronic engineering characterized by combinations of analytical and synthetic KBs.
“Although the geographical location of most collaborators predominantly refers to Shanghai, Beijing, Tianjin, Zhejiang Province, Jiangsu Province and Guangdong Province, geographical proximity varies, which means cognitive proximity is more important than geographical proximity at this level”, an expert working in the medical device consultation department summarized based on her rich consultation experiences.
4.1.3. Shanghai-global cooperation
Although the Shanghai high-end medical device industry has recently developed quickly, medical device products from America, Germany, Japan, Switzerland and the Netherlands have been imported to Shanghai and China for a long time. In specific fields, Shanghai’s industrial development has depended highly on international sources (Shanghai high-end medical device industry report in 2019).
Research staff specializing in R&D of high-end medical devices acknowledged that”Shanghai-international interactions primarily include optical engineering (optical engineering consists of a combination of analytical and synthetic KBs) and electronic engineering. Concerning optical engineering, collaborators mainly come from cities in Germany, Japan and Switzerland, which have advanced optical engineering knowledge in this aspect, while American collaborators are the biggest partners in R&D in electronic engineering, as they are renowned in this field” (Wang, Citation2008).
In these international interactions, social proximity and cognitive proximity are often linked to each other and matter together in R&D projects regarding optical and electrical engineering. “The social relationships are primarily built via universities where we once studied, as well as previous institutes where we worked. The same education background and working environments lead to similar cognitive levels”, CEOs of medical device companies explained. To some extent, cognitive proximity can be replaced by social proximity.
Earlier collaborations or peer recommendations play an important role in facilitating current interactive learning. If the behavior of both partners is in line with their mutual expectations during earlier collaborations, they usually continue to work together in the future.
Entrepreneurs with overseas studying backgrounds acknowledged that “Collaborating with alumni, laboratories and universities via regular communication and sending people to collaborating organizations where they once studied is a common interactive mode. Note that collaboration at the international level is fundamentally based on the communication between optical engineers, electronic engineers and mechanical engineers, referring to concrete technical resolve schemes, whereas the doctor-engineer collaborations are few”.
In addition to cognitive and social proximity, the international level also plays a role in organizational proximity. For example, in 2013, Weichuang acquired Wright’s OrthoRecon business, which consists of hips and knee implant products, and set up Weichuang’s global orthopedics headquarters in Arlington, Tennessee, USA. Then it acquired the stent-related assets of Johnson & Johnson Cordis in 2014. The organizational integration aims at building technological relatedness between Shanghai headquarters and branches. Furthermore, “the dual-embeddedness cooperation mode also prevails, which means the subsidiaries actively collaborate with other companies or institutes in their geographic scope and hence get the patents relevant to CKB and then offer help for R&D activities in Shanghai headquarters”, one scholar specializing in International R&D activities. “This approach is breaking a ban on the export of cutting-edge technology from several countries (i.e., the USA) to China. Optical devices and electronics are the main objects of the export ban”, he added.
5. Conclusions and policy implications
5.1. Conclusions
Economic geographers have long shown an interest in knowledge flows at different spatial scales. So far, however, at least two weaknesses could be identified in the literature, namely, a limited understanding of multi-scalar CKB in the healthcare industry and a story associated with advanced cities in developing countries. By empirically investigating the Shanghai high-end medical device industry, we are able to fill these gaps and hence contribute to the literature on the geography of knowledge.
Moreover, we find that Shanghai companies conduct R&D activities at different spatial levels through different dimensions of proximity regarding the combination of analytical and integrative types of knowledge. At the local level, geographical, institutional, and social proximity play an active role in software engineering, electrical engineering, and human factors engineering R&D. At the national level, organizational and cognitive proximity play a role in software engineering and electronic engineering cooperation. At the international level, Shanghai’s high-end medical device companies rely heavily on electrical engineering from the USA and optical engineering from Japan, Germany, Switzerland, and the Netherlands.
Based on our findings and the existing CKB literature, we identify several similarities and differences between industrialized and emerging countries. On the one hand, the similarities include three aspects: (1) The combination of inter-KBs makes firms more innovative (Tödtling & Grillitsch, Citation2015); (2) Local, national, and international innovative sources matter together in facilitating combinations of different types of KBs (Plechero & Grillitsch, Citation2022); (3) Policies and intra-firm integration are crucial in facilitating KB combinations at the firm level (Marques, Citation2019; Strambach, Citation2017). On the other hand, differences refer to three aspects: (1) At the local level, social networks primarily constructed by friends, colleagues, families, and alums contribute to cross-domain R&D; (2) At the national level, in China, some innovative advantages outside Shanghai have been developed into affiliates of Shanghai headquarters. Inflows of research institutes are central strategies for CKBs in most regions in emerging economies; (3) At the international level, social ties work for local-global CKB cooperation in China, whereas social linkages are rarely emphasized in industrialized studies.
Finally, three remaining interesting issues require future research. First, the analytical framework developed in this paper to analyze the Shanghai high-end medical device industry to study proximities could be applied more widely in other empirical studies on CKB, industrial clusters, and global value chains. Secondly, within Shanghai, more research could be done on other kinds of industries and the potential combinations of KBs in order to compare features and characteristics of combinatorial knowledge flows. Thirdly, future research could be carried out on the medical device industry in other cities in China or abroad, in order to compare the results and to be able to generalize and have a deeper understanding of underlying mechanisms explaining combinatorial knowledge interaction across different geographical contexts.
5.2. Policy implications
Based on the empirical results, this study offers the following policy implications to promote industrial development in Shanghai. Firstly, the Shanghai government should actively form new optical departments and create new affiliated entities for the application of optical knowledge in the high-end medical device industry (the CKB regarding optical engineering). Reconfiguring and integrating optical R&D sources at the system level offer firms more opportunities to engage in innovative optical projects. Secondly, at the international level, stable collaborations are often highly dependent on regional cognitive proximity on the one hand, but on the other hand, such a solid tie also results from extra-regional trust and friendship (Broekel & Boschma, Citation2012; Martin et al., Citation2017). Therefore, international linkages can be strengthened from inside and outside. Thirdly, the downstream-pulling-upstream model dominated the Shanghai high-end medical device industry in the past several years. It is necessary to shift to the upstream-pushing-downstream model to accelerate regional industrial development via technological changes in the next years.
Author contributions
This study was conceptualized by Shuaijun Xue and Robert Hassink; Shuaijun Xue conducted the fieldwork in China, and wrote the manuscript in Germany; Robert Hassink edited and revised the article. All authors read and approved the final manuscript.
Acknowledgments
An earlier version of the paper was presented at the Northern German Colloquium on Economic Geography in 2021. We are grateful for constructive comments received.
Disclosure statement
No potential conflict of interest was reported by the author(s).
Additional information
Funding
References
- Asheim, B. T., Boschma, R., & Cooke, P. (2011). Constructing regional advantage: Platform policies based on related variety and differentiated knowledge bases. Regional Studies, 45(7), 893–17. https://doi.org/10.1080/00343404.2010.543126
- Asheim, B., Coenen, K., & Vang, J. (2007). Face-to-face, buzz and knowledge base: Socio-spatial implications for innovation and innovation policy. Environment and Planning C: Government and Policy, 25(5), 655–670. https://doi.org/10.1068/c0648
- Asheim, B. T., & Gertler, M. S. (2005). The geography of innovation: Regional innovation systems. In J. Fagerberg, D. Mowery, & R. Nelson (Eds.), The oxford handbook of innovation (pp. 291–317). Oxford University Press. https://doi.org/10.1093/oxfordhb/9780199286805.003.0011
- Asheim, B., Grillitsch, M., & Trippl, M. (2017). Introduction: Combinatorial knowledge bases, regional innovation, and development dynamics. Economic Geography, 93(5), 429–435. https://doi.org/10.1080/00130095.2017.1380775
- Balland, P. A., Boschma, R., & Frenken, K. (2015). Proximity and innovation: From statics to dynamics. Regional Studies, 49(6), 907–920. https://doi.org/10.1080/00343404.2014.883598
- Balland, P. A., Jara-Figueroa, C., Petralia, S. G., Steijn, M. P., Rigby, D. L., & Hidalgo, C. A. (2020). Complex economic activities concentrate in large cities. Nature Human Behaviour, 4(3), 248–254. https://doi.org/10.1038/s41562-019-0803-3
- Bathelt, H., Malmberg, A., & Maskell, P. (2004). Clusters and knowledge: Local buzz, global pipelines and the process of knowledge creation. Progress in Human Geography, 28(1), 31–56. https://doi.org/10.1191/0309132504ph469oa
- Bellet, M., Colletis, G., & Lung, Y. (Eds.). (1993). Économie de proximités. In Revue d’Économie Régionale et Urbaine (Vol. 3).
- Bennat, T., & Sternberg, R. (2020). Knowledge bases in German regions: What hinders combinatorial knowledge dynamics and how regional innovation policies may help. European Planning Studies, 28(2), 319–339. https://doi.org/10.1080/09654313.2019.1656168
- Bergsland, J., Elle, O. J., & Fosse, E. (2014). Barriers to medical device innovation. Medical Devices (Auckland, NZ), 7, 205. https://doi.org/10.2147/MDER.S43369
- Boschma, R. (2005). Proximity and innovation: A critical assessment. Regional Studies, 39(1), 61–74. https://doi.org/10.1080/0034340052000320887
- Boschma, R. (2018). A concise history of the knowledge base literature: Challenging questions for future research. In New avenues for regional innovation systems-theoretical advances, empirical cases and policy lessons (pp. 23–40). Springer. https://doi.org/10.1007/978-3-319-71661-9_2
- Broekel, T., & Boschma, R. (2012). Knowledge networks in the Dutch aviation industry: The proximity paradox. Journal of Economic Geography, 12(2), 409–433. https://doi.org/10.1093/jeg/lbr010
- Broekel, T., & Youn, H. (2019). Using structural diversity to measure the complexity of technologies. PloS One, 14(5), e0216856. https://doi.org/10.1371/journal.pone.0216856
- Cao, X., Zeng, G., & Ye, L. (2019). The structure and proximity mechanism of formal innovation networks: Evidence from Shanghai high‐tech ITISAs. Growth and Change, 50(2), 569–586. https://doi.org/10.1111/grow.12294
- Capone, F., & Lazzeretti, L. (2018). The different roles of proximity in multiple informal network relationships: Evidence from the cluster of high technology applied to cultural goods in Tuscany. Industry and Innovation, 25(9), 897–917. https://doi.org/10.1080/13662716.2018.1442713
- Carrincazeaux, C., Lung, Y., & Rallet, A. (2001). Proximity and localisation of corporate R&D activities. Research Policy, 30(5), 777–789.
- Chen, C., Fan, X., Li, S., & Tan, M. (2017). Effectiveness, problems and countermeasures of promoting the integration of industry, education and research in Shanghai. Science Development. 09(in Chinese), 31–38.
- Cheong, S. T., Li, J., Ung, C. O. L., Tang, D., & Hu, H. (2020). Building an innovation system of medical devices in China: Drivers, barriers, and strategies for sustainability. SAGE Open Medicine, 8, 2050312120938218. https://doi.org/10.1177/2050312120938218
- Davey, S. M., Brennan, M., Meenan, B. J., & McAdam, R. (2011). Innovation in the medical device sector: An open business model approach for high-tech small firms. Technology Analysis & Strategic Management, 23(8), 807–824. https://doi.org/10.1080/09537325.2011.604152
- Davids, M., & Frenken, K. (2018). Proximity, knowledge base and the innovation process: Towards an integrated framework. Regional Studies, 52(1), 23–34. https://doi.org/10.1080/00343404.2017.1287349
- Du, D. (2018). Global Science and Technology innovation Centers: World Trends and China’s practice Science. Science, 70(6), 15–18 + 69.
- Eisenhardt, K. M. (1989). Building theories from case study research. The Academy of Management Review, 14(4), 532–550. https://doi.org/10.2307/258557
- Figueiredo, P. N., Larsen, H., & Hansen, U. E. (2020). The role of interactive learning in innovation capability building in multi-national subsidiaries: A micro-level study of biotechnology in Brazil. Research Policy, 49(6), 103995. https://doi.org/10.1016/j.respol.2020.103995
- Franco, M., & Esteves, L. (2020). Inter-clustering as a network of knowledge and learning: Multiple case studies. Journal of Innovation & Knowledge, 5(1), 39–49. https://doi.org/10.1016/j.jik.2018.11.001
- Gong, H., & Hassink, R. (2020). Context sensitivity and economic-geographic (re)theorising. Cambridge Journal of Regions, Economy & Society, 13(3), 475–490. https://doi.org/10.1093/cjres/rsaa021
- Grillitsch, M., Asheim, B., & Trippl, M. (2018). Unrelated knowledge combinations: The unexplored potential for regional industrial path development. Cambridge Journal of Regions, Economy & Society, 11(2), 257–274. https://doi.org/10.1093/cjres/rsy012
- Grillitsch, M., & Hansen, T. (2019). Green industry development in different types of regions. European Planning Studies, 27(11), 2163–2183. https://doi.org/10.1080/09654313.2019.1648385
- Grillitsch, M., Martin, R., & Srholec, M. (2017). Knowledge base combinations and innovation performance in Swedish regions. Economic Geography, 93(5), 458–479. https://doi.org/10.1080/00130095.2016.1154442
- Guan, J. C., & Yan, Y. (2016). Technological proximity and recombinative innovation in the alternative energy field. Research Policy, 45(7), 1460–1473.
- Hansen, T. (2015). Substitution or overlap? The relations between geographical and non-spatial proximity dimensions in collaborative innovation projects. Regional Studies, 49(10), 1672–1684. https://doi.org/10.1080/00343404.2013.873120
- Isaksen, A., & Trippl, M. (2017). Exogenously led and policy-supported new path development in peripheral regions: Analytical and synthetic routes. Economic Geography, 93(5), 436–457. https://doi.org/10.1080/00130095.2016.1154443
- Kramer, D. B., Xu, S., Kesselheim, A. S., & Moher, D. (2012). How does medical device regulation perform in the United States and the European Union? A systematic review. PLoS Medicine, 9(7), e1001276. https://doi.org/10.1371/journal.pmed.1001276
- Laursen, K., Reichstein, T., & Salter, A. (2011). Exploring the effect of geographical proximity and university quality on university–industry collaboration in the United Kingdom. Regional Studies, 45(4), 507–523. https://doi.org/10.1080/00343400903401618
- Liefner, I., Si, Y. F., & Schäfer, K. (2019). A latecomer firm’s R&D collaboration with advanced country universities and research institutes: The case of Huawei in Germany. Technovation, 86, 3–14. https://doi.org/10.1016/j.technovation.2019.03.002
- Li, D., Heimeriks, G., & Alkemade, F. (2021). Recombinant invention in solar photovoltaic technology: Can geographical proximity bridge technological distance? Regional Studies, 55(4), 605–616. https://doi.org/10.1080/00343404.2020.1839639
- Manniche, J. (2012). Combinatorial knowledge dynamics: On the usefulness of the differentiated knowledge bases model. European Planning Studies, 20(11), 1823–1841. https://doi.org/10.1080/09654313.2012.723423
- Manniche, J., & Larsen, K. T. (2013). Experience staging and symbolic knowledge: The case of Bornholm culinary products. European Urban and Regional Studies, 20(4), 401–416. https://doi.org/10.1177/0969776412453146
- Marek, P., Titze, M., Fuhrmeister, C., & Blum, U. (2017). R&D collaborations and the role of proximity. Regional Studies, 51(12), 1761–1773. https://doi.org/10.1080/00343404.2016.1242718
- Marques, P. (2019). Intra-and inter-firm dynamics in combinatorial knowledge bases. European Urban and Regional Studies, 26(2), 186–204. https://doi.org/10.1177/0969776418779116
- Martin, R., Aslesen, H. W., Grillitsch, M., & Herstade, S. J. (2017). Regional innovation systems and global flows of knowledge. In A. Isaksen, R. Martin, & M. Trippl (Eds.), New avenues for regional innovation systems – theoretical advances, empirical cases and policy lessons (pp. 127–147). Springer. https://doi.org/10.1007/978-3-319-71661-9_7
- Mattes, J. (2012). Dimensions of proximity and knowledge base: Innovation between spatial and non-spatial factors. Regional Studies, 46(8), 1085–1099. https://doi.org/10.1080/00343404.2011.552493
- Mazzucchelli, A., Chierici, R., Abbate, T., & Fontana, S. (2019). Exploring the microfoundations of innovation capabilities. Evidence from a cross-border R&D partnership. Technological Forecasting and Social Change, 146, 242–252. https://doi.org/10.1016/j.techfore.2019.06.003
- Meng, G., & Zeng, D. Z. (2019). Structural transformation through free trade zones: The case of Shanghai. Transnational Corporations Journal, 26(2), 95–116. https://doi.org/10.18356/70a11ff7-en
- Mewes, L. (2019). Scaling of atypical knowledge combinations in American metropolitan areas from 1836 to 2010. Economic Geography, 95(4), 341–361. https://doi.org/10.1080/00130095.2019.1567261
- Musil, R., & Eder, J. (2016). Towards a location sensitive R&D policy. Local buzz, spatial concentration and specialisation as a challenge for urban planning–empirical findings from the life sciences and ICT clusters in Vienna. Cities, 59, 20–29. https://doi.org/10.1016/j.cities.2016.05.023
- Nieth, L., & Benneworth, P. (2020). Challenges of knowledge combination in strategic regional innovation processes-the Creative Science Park in Aveiro. European Planning Studies, 28(10), 1922–1940. https://doi.org/10.1080/09654313.2019.1699908
- Plechero, M., & Grillitsch, M. (2022). Advancing innovation in manufacturing firms: Knowledge base combinations in a local productive system. European Planning Studies, 31(6), 1–23. https://doi.org/10.1080/09654313.2022.2122705
- Rallet, A., & Torre, A. (1998). Which need for geographical proximity in innovation networks at the era of global economy. University of Paris Dauphine.
- Sabbado, L., Daniel, M., Ruiller, C., Fromont, E., & Crambert, R. (2021). The role of proximity relations in the integration process into the network: An analysis of CEOs’ life narratives. Industry and Innovation, 28(7), 1–21. https://doi.org/10.1080/13662716.2021.1891868
- Shearmur, R., Carrincazeaux, C., & Doloreux, D., (Eds.). (2016). Handbook on the geographies of innovation. Edward Elgar Publishing. https://doi.org/10.4337/9781784710774
- Shen, K., & Li, L. (2014). Research on the relationship between innovation-driven development and the construction of “four centers” in Shanghai. Shanghai Economic Research. 10(in Chinese), 3–15. https://doi.org/10.19626/j.cnki.cn31-1163/f.2014.10.001
- Shi, T. (2016). Establishment and effectiveness of industrial Technology innovation Strategic alliance in Shanghai medical device field. Science and Technology Innovation and Productivity. 05(in Chinese), 12–15.
- Strambach, S. (2017). Combining knowledge bases in transnational sustainability innovation: Microdynamics and institutional change. Economic Geography, 93(5), 500–526. https://doi.org/10.1080/00130095.2017.1366268
- Tödtling, F., & Grillitsch, M. (2015). Does combinatorial knowledge lead to a better innovation performance of firms? European Planning Studies, 23(9), 1741–1758. https://doi.org/10.1080/09654313.2015.1056773
- Trippl, M., Grillitsch, M., & Isaksen, A. (2018). Exogenous sources of regional industrial change: Attraction and absorption of non-local knowledge for new path development. Progress in Human Geography, 42(5), 687–705. https://doi.org/10.1177/0309132517700982
- Turkina, E., Van Assche, A., & Doloreux, D. (2021). How do firms in co-located clusters interact? Evidence from Greater Montreal. Journal of Economic Geography, 21(5), 761–782. https://doi.org/10.1093/jeg/lbaa019
- Wagner, M., & Growe, A. (2022). Patterns of knowledge bases in large city regions in Germany: Comparison of cores and their surrounding areas. Geografiska Annaler: Series B, Human Geography, 105(3), 1–21. https://doi.org/10.1080/04353684.2022.2141131
- Wang, H. (2008). A study on the development of China´ s laser & optoelectronic technology and industry: With city cases of Wuhan. Studien zur Wirtschaftsgeographie.
- Wang, A., Shen, W., Wu, Y., & Wen, D. (2021). Shanghai: Serve the Healthy development of medical device industry and guarantee the safety of people’s equipment by institutional mechanism innovation. Shanghai Medical Journal, 44(2), 69–72. https://doi.org/10.19842/j.cnki.issn.0253-9934.2021.02.001
- Wu, W. (2007). Cultivating research universities and industrial linkages in China: The case of Shanghai. World Development, 35(6), 1075–1093. https://doi.org/10.1016/j.worlddev.2006.05.011
- Xu, L., Lin, S., Hu, L., Yang, Y., Wu, Z., Huang, J., & Jiang, H. (2019). Reference and innovation: Research on the construction of medical device registrant system. Journal of Chinese Medical Device, 43(3), 192–196.
- Yew, J. L. K. (2021). Family firms, enterprise development and tacit knowledge transformation: Small and Medium enterprises (SMEs) innovation in Malaysia. Global Business Review, 09721509211047648. https://doi.org/10.1177/09721509211047648
- Yin, R. K. (2003). Designing case studies. In Case study research: Design and methods (pp. 19–56). Sage.
- Zeng, G., Liefner, I., & Si, Y. (2011). The role of high-tech parks in China´s regional economy: Empirical evidence from the IC industry in the Zhangjiang high-tech Park, Shanghai. Erdkunde, 65(1), 43–53. https://doi.org/10.3112/erdkunde.2011.01.04
- Zhao, J., Wu, G., Xi, X., Na, Q., & Liu, W. (2018). How collaborative innovation system in a knowledge-intensive competitive alliance evolves? An empirical study on China, Korea and Germany. Technological Forecasting and Social Change, 137, 128–146. https://doi.org/10.1016/j.techfore.2018.07.001