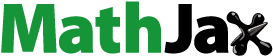
Abstract
The coronavirus disease (COVID-19) pandemic produced adverse effects which spreads globally and throughout all the nations. During the outbreak, governments announced emergency policies geared to control the situation. Subsequently, researchers have attempted to determine the advantages and disadvantages of these policies. We developed a method for evaluating COVID-19 policy in Thailand from January 2020 to March 2022. The Policy Model Consistency (PMC) Index is introduced as the primary model with 10 powerful indicators for policy evaluation. Additionally, the stock price changes while the COVID-19 policy was being enforced, as recorded in the Securities Exchange of Thailand (SET) database, are also observed with the other indicators for a measure of public confidence. All indicators are scored on a binary basis based on the policy consistency. The result of PMC-score is outputted as a contour surface graph. The PMC-surface result shows the good and adverse point of the policy to a policymaker, and it is successfully discussed to present the internal relationship of the indicators from the policymaker’s perspective. This tool benefits policymakers by facilitating early identification of policy’s strengths and weaknesses and the findings of policy angles in all related perspectives are significant to policymakers to address and improve in the next enhancement as well.
1. Introduction
In 2021, the coronavirus disease 2019 (COVID-19) pandemic spread throughout Thailand, exerting both direct and indirect effects on health and day-to-day life. The number of infected people has been dramatically increasing since April 2021, from 42 confirmed cases on March 31st to 2839 cases on April 24th (World Health Organization Thailand, Citation2022). In Thailand, the outbreak has been spread over five phases, beginning in January 2020. The current wave is attributed to the Omicron variant, first discovered in South Africa, and has increased the number of COVID-19 cases nationwide. Beginning with the first case Thai COVID-19 case in January 2021, the number of infections increased to around 23,400 per day by August 2021 (World Health Organization Thailand, Citation2022). The government announced many policies to help citizens, enhance access to currency, and slow disease spread (Office of the International Parliamentary Organization, Citation2021). For example, lockdown measures were strictly enforced to reduce face-to-face meetings and the Welfare Ministry of Thailand approved additional financial measures, including government subsidies, to support cost-of-living and daily expenses during the lockdown.
The Thai government responded to the COVID-19 pandemic by issuing urgent policies designed to slow the spread of COVID-19 as much as possible. Since then, various economic and social welfare policies have been proposed to address the pandemic’s long- and short-term societal challenges (Council of Misters of Thai government, Citation2021). Unfortunately, not all policies were successful. There are several policy deficiencies when they was distributed to an sub-operation area, such as the lack of patient beds or medical personnel and a delay of operation processes (Toraksa & Saengkrod, Citation2019). Such deficiencies caused direct issues to the community. Efficient and productive feedback is extremely significant for policymaker to improve the policy’s quality but it requires some time and resources for the study. Therefore, our research objective is to discover a policy model which can properly evaluate the overall implementation policy with less requirement of the analysis process for an emergency case. Based on the objective, our research question is following: 1) What a policy model can be used to analyze policy strengths and weaknesses with quick response? and 2) How to include the societal belief level to the model? There are several policy models applied to various fields of study, for example, a survey of transportation policy by elasticity analysis (Hsieh, Citation2020), a quantitative evaluation of agricultural policy by the Policy Modelling Consistency Index (PMC-Index) (Kuang et al., Citation2020), a survey responses analysis of air-pollution policy (Geng et al., Citation2018), and others. Among these, the PMC-Index (Ruiz Estrada, Citation2012) is suitable for Thailand’s COVID situation because its framework greatly facilitates large-scale analysis with quick responses.
To promptly structure an announced policy and reflect the public feedback intensity, this research proposes a novel data analysis model for evaluating the strengths and weaknesses of COVID-19 in Thailand by using the PMC-Index model approach. The model constructs a policy in 10 connected angles designed by a researcher based on various information, not specific only about the individual topic. Policymakers can use the model to study other policy fields as well by designing identifiers capable of evaluating the policy from different perspectives. The PMC model comprises four phases. Firstly, the parameters and variables that define the policy’s strengths and weaknesses are organized into main- and sub-variables, which are further classified based on the relevant policy field. Next, an input-output table is used to quantify all related variables. The PMC-index score is calculated from table step two. Finally, the PMC- surface is constructed from the PMC-Index score, and the policy can be efficiently evaluated using the model’s output to determine its strengths and weaknesses. The greater challenge with this approach is determining which variables exert the greatest impact on the policy.
Stock price is considered a policy-index indicator in that it represents investor’s public beliefs in government policies following their issuance. Changes in stock prices are popular indicators given their (sometimes wide) fluctuation resulting from public information (Agarwal, Citation2020). For example, suppose there is positive news about a company. The company’s stock price will generally rise because of investors’ confidence in the company’s future. These official policy announcements can be linked to breaking financial news, given that such announcements may result in fluctuating stock, which can be assessed by comparing them with the prior year. Then, the varied rate of relative stocks, compared with inflation within the same duration, is brought to identify the intensity of the changes. Since inflation is an important measurement of the consistency of economic growth, it can also impose the negative and positive situation on the nation (Eggoh & Khan, Citation2014; Gokal & Hanif, Citation2004).
According to the proposed circumstance, this research focused on the policy model related to COVID-19 policy in Thailand. The model consists of 10 designed indicators based on the PMC-Index approach, and the stock price changes are included in the indicators for evaluating investor’s point of view on the policy measurement. The organization of the article is as follows five sections. After the overview of relevant literature on the policy evaluation models and stock price significance in section 2, the methodology is presented in section 3. This section is divided into three parts: Data Preparations, Model Design, and Result Validation. Correspondingly, the findings and discussions are obtained and presented in the remainders of section 4 and 5, respectively
2. Policy evaluation and stock significance
This section describes the theories and related literature that can be applied to future work. The details are as follow:
2.1. Public policy actions under the COVID-19 situation
COVID-19 has been spreading rapidly worldwide and it has been causing massive losses. The pandemic has greatly affected the global health system and human living. Governments across many countries have been working day and night to mitigate the reproductive number of infections. Billah et al. (Citation2020) had defined the reproductive number (R) as an efficacy of virus transmission from the primary infected patient to the second infected person. For example, if R number for an area is 3.00, it means that the virus could be carried from one person to three persons. Thus, the R number have been consistently assessed and decreased in many countries, R > 1 the infection is seriously expanding and R < 1 is contracting (Vegvari et al., Citation2021).
According to emergency policy, Dzator et al. (Citation2021) studied the lockdown policy outcome based on the number of daily confirmed cases. The result significantly indicated that the interaction between policy strictness and handwashing policy positively affected daily confirmed cases in the long term. On the other hand, it was almost negative at the initial stage because the announced policy required time for the responses.
In Thailand, the first case was found on 13 January 2020 and since then, the number of infections has been increasing dramatically (World Health Organization Thailand, Citation2022). During the pandemic, the Thai government and The Ministry of Public Health of Thailand worked intensively to solve that emergency problem. They had officially announced many policies to assist people’s plight, enhance liquidity, and slow the spreading (Office of the International Parliamentary Organization, Citation2021). For example, the traveling restriction to the high-risk province, and intensive lockdown rules were also highly controlled. Toraksa and Saengkrod (Citation2019) had studied the implementation of COVID-19 policy in a controlled municipality, Chumpae Thailand. They conducted a qualitative experiment by interview with all relevant organizations. The findings presented that there were still problems about the operation process and the lack of medical personnel to handle during the outbreak. The announced policy was not prepared to cover all angles well: the gap was that the operation took time.
2.2. Basic methods of policy evaluation
Various policy models were proposed to evaluate a policy in all associated perspectives. Geng et al. (Citation2018) conducted the qualitative research to discuss the perspective of public opinion against the official transport policies in an urban of China. Four-paradigm models introduced the survey, categorized as economics, administration, technology, and public information, based on public policy responses and evaluations. The results indicated that public responses to public transportation were stronger than car-restriction policies. Identically, Hsieh (Citation2020) evaluated transportation policy from collected survey data. The model was introduced by elasticity analysis to represent the social reaction level. A policy evaluation utilizing survey model is famous for many study topics. However, the gap is that the proposed model requires a certain area of measurement and is suitable for a specific attribute. Time consuming is one of the factors to be considered.
Other policy analysis models are also proposed to help policymakers review the overall policy angles for improvement. Ruiz Estrada (Citation2012) introduced the Policy Model Consistency (PMC) Index model to evaluate the strength and weakness of a policy by creating 10 powerful. With regards to the model framework, there were four steps; (i) the classification of 10 variables which were designed by a researcher to reflect strength and weakness through 10 policy angles, (ii) use of an input-output table that arrange all variables and sub-variables into the table, (iii) measuring PMC-Index score that evaluating the score of each policy indicator, and (iv) construction a PMC-Surface that generating a surface graph by the score of all 10 indicators. The surface allowed a policymaker to discover the strengths and weaknesses of the surface graph, which showed 10 parameter scores.
Kuang et al. (Citation2020) evaluated the Chinese cultivated land protection policy using the Policy Model Consistency (PMC) Index model. The PMC-Index evaluate policies using researcher-defined criteria to generate evaluation score and final matrix surface graph. The overall value of a particular policy can then be discussed based on the consistency rating. Gao et al. (Citation2021) adopted PMC-Index framework with text-mining and machine-learning techniques to match each parameter appropriately. The proposed methodology improved the accuracy of the evaluation, and the efficiency of the overall result was also improved. Such enhancements could be applied to various policy types. However, a major challenge of this method was the complexity of natural language and domain knowledge needed to establish specific keywords to indicate the main policy approaches.
2.3. Stock price analysis
This section presents factors directly impacting the stock price from many study cases. They have significant positive and negative effects on one stock or the whole sector.
2.4. Stock price prediction from headline news
Headline news can produce significant stock market fluctuations. Thus, many researchers have studied stock price fluctuation resulting from public information disseminated on various communication platforms. Li et al. (Citation2014) proposed a method of determining which news articles most influenced investors’ trading activity and stock market fluctuations. This methodology was applied to the original predictive method, the Support Vector Regression model (SVR). The comparison result showed that the stock market responded to public information on social media. Similarly, Shah et al. (Citation2019) proposed a stock trend prediction based on sentiment scores measured from India’s news articles. They identified that relative information about a stock also significantly affected investing activities and insights. This study also used Natural Language Processing model (NLP) to calculate decision-makers’ sentiment scores after the news was released. Additionally, the researchers summarized the frequency of positive and negative words and the resultant score effects. Agarwal proposed a similar methodology by categorizing news impacts as Positive, Negative, or Neutral (Kirange & Deshmukh, Citation2016) to draw conclusions about market characteristics and social trends.
2.5. Correlation between stock price and inflation
Inflation is one of the indicators for identifying the economic growth rate. Gokal and Hanif (Citation2004) studied this long-standing debate topic about the relationship between inflation and economic growth. The reviewed empirical literature highlighted that the inflation rate significantly affected economic growth when it was maintained at a low rate and considerably influenced the short-market than the long-run market. Similarly, Eggoh and Khan (Citation2014) presented about nonlinearity of inflation and economic growth as well. The findings were analyzed by PSTM and GMM models to determine that the inflation rate and economic growth had a relationship as a nonlinearity. Furthermore, the financial system, trade openness, capital accumulation, and government size also powerfully influenced the relationship. The level of an inflation-financial system which tended to have an empirical impact on a country, was not fully described in the work because it was likely related to the characteristic of a country and other variables.
There is a well-recognized relationship between stock price changes and inflation. Farooq and Ahmed (Citation2018) collected relative stock price and inflation data to determine how investors are affected by inflation. General inflation information is often used to forecast stock price increases, and inflation news significantly impacts stock market fluctuations. Additionally, Lou et al. (Citation2021) expressed that the inflation news, stock, bond pricing, and consumption growth had a significant moment of impact on macroeconomic. The researchers summarized that bond yields had been connected to inflation and risk aversion variances while risk aversion was positively fluctuated by inflation news. Nevertheless, other variables which related to the alteration of economics were not included in the discussion to support their evaluations.
2.6. Related work
Hale et al. (Citation2021) studied COVID-19 policies using the Oxford COVID-19 Government Response Tracker (OxCGRT) to determine the effects of these policies on government actions. The researchers identified 19 government policy actions, which were subsequently discussed and analyzed. Cairney (Citation2021) assessed COVID-19 policies published by the UK government, including policy generation, information dissemination, and learning to improve the efficiency of the government’s response.
Özer (Citation2020)proposed the review of educational policy actions from the Turkish Ministry. The pandemic rate in Turkey was higher after 100 confirmed cases. Therefore, schools in Turkey were announced to be closed immediately. The prompt reaction from the ministry of education was a long-distance classroom. They closely collaborated with broadcast corporations to provide an educational program by free-accessibility. The results became an important resource for educational system during the spreading in Turkey.
The government developed and deployed many policies during the COVID-19 pandemic, some of which were successful (others were not). Policies must be efficiently deployed as quickly as possible to control the emergency situation such as the global COVID-19 pandemic. Various models to assess a policy’s overall quality have been proposed. However, it can be challenging to determine which indicators should be included to cover policy’s significant points. We sought to establish a method for assessing the long- and short-term policy effects based on standard policy perspectives, including stock market indicators. Ideally, such a model should include salient issues to guide future policy development and deployment.
3. Materials and methods
We sought to develop an effective model for evaluating policies related to COVID-19 in Thailand. To accomplish this, we use the PMC- Index model and present our work in three parts: Data Preparation, Model Design, and Result Validation.
3.1. Data preparation
The input data consisted of the Thai government’s COVID-19 policies, COVID-19 cases per day, reproduction rate, stock price, inflation rate, and Business Sentiment Index (BSI), as shown in Table . These variables were entered into a data table and divided into four main categories: Containment and Closure, Health System, Business Responses, and Miscellaneous.
Table 1. Data sources
3.2. Model design
With regards to the model design, PMC-Index model is applied to the research because the model structure can widely distribute an input-policy in many attributes on a practical surface graph. Four main phases are implemented following the PMC-model structure: Classification of Variables, Tabulating Input-Output, Calculation of PMC-Index Score, and Construction of PMC-Surface. The first part, Classification of Variables, is the first major sequence to set up indicators for evaluating policy’s perspective. This section defines the characteristics which are fitted to Thailand’s COVID-19 policies. It aims to indicate the strength and weaknesses of the policy in 10 different angles. The rest sections represent the variables table, PMC scoring, and the outputted surface graph, respectively.
3.3. Classification of variables
The PMC-Index evaluates policies by examining the relationship among internal factors (René Wies, Citation1996). Thus, each attribute is linked and evaluated for the perspective of a selected policy. Ten mains ( and sub-variables
are designed following this methodology and included in the policy structure. To determine the confidence in a policy, we include stock price fluctuations from the related market sector as an indicator, as shown in Table .
Table 2. Main- and sub-variables of the PMC- Index model
is set to define a policy type (Kuang et al., Citation2020).
classifies the coverage of policy itself, which is divided into Economy, Industries, Health, Societies, Education, and Civic Space (Gao et al., Citation2021).
is the enforcement territory, which is National level, Provincial level, district, and only a specific village. Then,
is defined as the content of policy period. There is long term (more than 5 years), medium term (3–5 years), short term (less than 3 years), and non-specific.
is the level of stock price changing within policy period compared to the inflation in the same duration. The range of difference is set as the neutral level at
3%, the intermediate level at 3–5%, and the large level at more than 5%. For instance, if the related stock price is averagely changed about 5% after 3 months policy issue and the inflation rate is averagely 3.02%, the
will be at the neutral level because it shifts around 2% when compared with the inflation. Three percentage of changing is defined as an acceptable quantity because it is in the same range of money control by Bank of Thailand (BOT) (BANK OF THAILAND, Citationn.d..). Next,
is defined as policy supports. They are divided into financial, people health and well-being, industry, transportation, agriculture, service, and technology and education.
is the reproduction rate (R number) of COVID-19. There are no exact criteria for the evaluation based on Thailand’s circumstance. The level of R number is designed based on the ideal condition, which one infection should not spread the disease to more than one person. Therefore, the level of
is designed following: the acceptable rate is defined at 0.9–1.1, high rate when more than 1.1, and less rate as lower than 0.9. Consequently,
is the policy coverage. Four different ranges: childhood (0–14), youth (15–24), adulthood (25–65), and seniority (65 and above) are set.
is expressed as the policy continuity divided into continuation, discontinuation, and phase development. Lastly,
is defined as the public communication channel, government website, social media, and newspaper (Kuang et al., Citation2020).
3.4. Tabulating input-output
The main- and sub-variables are arranged in the input-output table to calculate the PMC -Index score in the next section. This is the beginning of the quantitative analysis.
3.5. Calculation of PMC-Index score
Once a policy is selected for evaluation, all sub-variables (n) in the table are considered to have the same significance or weight (importance) because the single value of the main-variables () is measured based on the PMC- Index. Consequently, we use a binary system (0,1) to score each sub-variable as the formula one for the matching context of policy.
Secondly, the value of the main- variables in each category was calculated by Equationequation (2)
(2)
(2) , where
is the total number of sub-variables.
Thirdly, the policy’s PMC- Index score is determined using formula three, calculated by summing all 10 main variables.
Based on the PMC-index model feature, if the PMC- Index score is 9–10, that policy is “Perfect”, 7–8.99 “Good”, 5–6.99 “Acceptable”, and 0–4.99 “Low” consistency. Given the variables specific to Thailand’s COVID-19 responses, the maximum possible PMC-Index score would be 7.12. The resultant four criteria levels are 0–1.77 “Low”, 1.78–3.55 “Acceptable”, 3.56–5.34 “Good”, and 5.35–7.12 “Perfect”.
3.6. Construction of PMC-surface
Finally, the scores were entered into the formula four matrix to calculate PMC-surface. We exclude from the equation because it consisted of a header used only to identify policy type and it was likely less significant compared to other variables. The PMC- Index score is a stereo image to analyze a policy’s strengths and weaknesses.
4. Results
This section clarifies the final result’s contribution and discusses policy strengths and weaknesses relative to surface score. The matching linkage values of sub-variables express the policy’s coverage field, the degree to which the policy’s stated objective was achieved, and the uncovered area. Furthermore, stock market fluctuations that followed the policy announcement likely reflected the public’s opinion. We also discuss the number of COVID-19 cases and the business sentiment index.
4.1. PMC-Index scoring and surface
After obtaining the required data, we extracted and scored policy’s contents based on PMC sub-variables, as with the Containment and Closure Policy example shown in Table . Then, each indicator’s score was calculated to the PMC- surface to construct an outstanding policy point (Figure ). Finally, the level of effectiveness of the policy will be evaluated by the PMC-Score and the PMC-surface according to the criteria.
Figure 1. The PMC-Surface of Containment and Closure policy. (a) curfew policy (b) country Closure policy (c) Business shutdown policy.
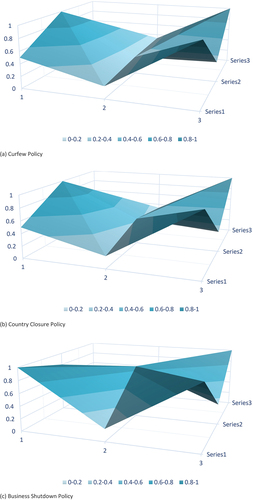
Table 3. The PMC-Index score of the containment and closure policies in three phases
5. Conclusions
Announcing business closure was the most effective policy, scoring 6.11. This policy was issued after the issuing of two curfew policies with the highest virus reproduction rate, . However, after the issuance and enforcement of the business shutdown policy, the stock
and business sentiment index levels continued to change positively. This likely reflects a strong belief in the business closure policy than the curfew or country entrance restriction policies. Support for business shutdowns was outstanding,
. These findings suggest that the curfew and country closure policy could be strengthened by describing the policies in greater detail by the government.
The PMC- scores for all evaluated policies are summarized in Table . The policy period, Business Sentiment Index (BSI), reproduction rate (R), and the percent of stock variations (P) are also addressed in the discussion session. When the government eased up on and
in the closure and containment group, the reproduction rate increased. Expansion of policy coverage areas may improve the overall score. Policymakers need to know which policies effectively control the outbreaks while simultaneously driving economic activity; such policies would invariably be favoured over those that only address one outcome (i.e., infection control or economic activity, though not both). Consequently,
has a more-significant positive change when
supports more outcomes. Improving the overall PMC- Index score suggests adequate coverage of policy’s supported areas. The relationship between
and
is also important since the PMC- Index score will improve as coverage improves. Implementing only broadly supported policy may reduce the conflict between policy measurement and policy areas. The idea of policy coverage can be applied to other policy types. Stock price changes reflect the public’s opinion of an announced policy. Such opinions should be sought out by policymaker early on.
Table 4. The PMC-Index score of all policy groups
This study proposes a modified policy model evaluating COVID-19 policies implemented by the Thai government during the recent COVID-19 global pandemic. We grouped the selected policies into four categories: Containment and Closure, Health system, Economic Responses, and Miscellaneous. All items were analyzed using 10 PMC-variables; each variable’s PMC-Score was calculated. Finally, the average PMC-Score and PMC-Surface were generated. The results reflect public’s opinion of policy by observing stock price changes after the policy was announced. Consideration of variables such as reproduction rate and BSI will support a policy’s comprehension and effectiveness. In accordance with a similar field of study, our result is reflected to cover policy’s environment from more different angles than the traditional qualitative study. For example, the survey research about the transportation policy correlated to social reaction (Hsieh, Citation2020) or the Urban’s resident survey of pollution policy in China’s sub-area (Geng et al., Citation2018) are designed to cover the policy implementation in deep detail. Then, their results revealed public’s opinions related to policy contents. Finally, the weakness, strength, and policy coverage are efficiently performed. However, the model is not suitable for the other areas of study. It cannot apply the indicators to other policy areas because the parameters were specifically built. Compared with our designed model, the 10 powerful indicators can be applied to other policy areas, such as economics, health system, and emergency policy. A wide diversity of the policy domain is as important as the policy responses. The other PMC research has also modified the 10-variables by using different techniques. Thus, adopting the PMC-Index as the policy evaluation of land protection policies has presented the PMC-score based on the 10 policy schemes (Kuang et al., Citation2020).
Nevertheless, the level of public concern is not also observed with the valid 10 indicators. As a result, our findings can improve their methodology by scoring the level of public trust, which is calculated by the fluctuation of the stock market after a policy is announced. Furthermore, our result dramatically benefits the public belief’s score from the study which applied text mining techniques to evaluate social interaction (Gao et al., Citation2021). The noise of information is out of the concerns based on our proposed work.
Since the emergency, such as COVID-19 outbreaks, quick and effective responses from a policy or emergency measurement are highly required for the next phase of development. Our empirical finding is a novel policy model for policymakers to measure a policy’s strengths and weaknesses with a short-time process. The policy indicators are designed to cover every angle of a general policy, such as policy areas, policy improvement, policy support, etc. Additionally, stock price changes are also included as one of the powerful variables to assist policymakers in measuring the public responses. The responses reflect the policy strength after an announcement from the investor’s perspective. When a policy has a low score for this parameter, it can be roughly noticed that it may leak somewhere. The results are scored by the PMC-Index model and displayed on the final PMC-surface graph. The comprehension of a policy is all presented on the surface by the essential information which can refine the strengths and weaknesses well. This model is expected to help policymakers to issue a practical policy for the country even in an emergency, such as the COVID-19 pandemic. The loss could be lowered, and the situation can be recovered within a short period as the model’s objective. However, the results should be considered with several theories, specifically, the scoring process and stock fluctuation factors, for example, Efficient Market Hypothesis (EMH) (Naseer & Bin Tariq, Citation2015), Random Walk Model (Naseer & Bin Tariq, Citation2015), Financial Behavior Theory (Ozmete & Hira, Citation2011), and so on. They describe that the stock market prices are consistent and ideally varied by the released information. According to our findings, the market fluctuations could be systematically analyzed, together with those models, to define the fluctuation with respect to an announced policy narrowly. The forms of the stock market can also help us evaluate the relationship between an obtained policy and investors’ behaviors. Furthermore, other data analysis techniques should be further applied to the evaluation and discussion section. The techniques, such as text-mining and descriptive analysis, should limit human bias and allow variables to be weighed appropriately. Additionally, further studies with the real test should be conducted to confirm the validity of our factors.
List of Abbreviation
BSI | = | Business Sentiment Index |
ETID | = | Economic and Trade Indices Database |
NLP | = | Natural Language Processing |
PMC | = | Policy Model Consistency |
SVR | = | Support Vector Regression |
Disclosure statement
No potential conflict of interest was reported by the authors.
Additional information
Notes on contributors
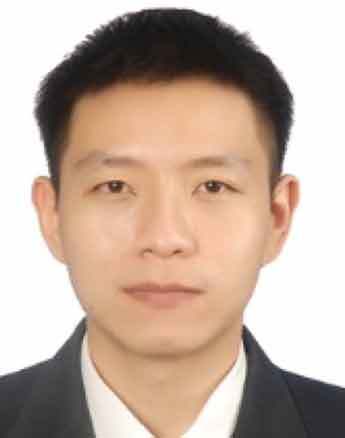
Adisorn Yimsuk
Adisorn Yimsuk, Graduate with a Master’s degree in IT Management from Mahidol University, Thailand. He was born in Thailand, 13 December 1992. His research interest are social issues, data management, and social policy.
References
- Agarwal, A. (2020). Sentiment analysis of financial news. Proceedings - 2020 12th International Conference on Computational Intelligence and Communication Networks, CICN 2020 (pp. 312–13). https://doi.org/10.1109/CICN49253.2020.9242579
- Bank of Thailand. (n.d.). Bank of Thailand | Central Bank of Thailand. https://www.bot.or.th/English/Pages/default.aspx
- Billah, M. A., Miah, M. M., Khan, M. N., & Flacco, M. E. (2020). Reproductive number of coronavirus: A systematic review and meta-analysis based on global level evidence. PLoS ONE, 15(11), 1–17. https://doi.org/10.1371/journal.pone.0242128
- Cairney, P. (2021). The UK government’s COVID-19 policy: Assessing evidence-informed policy analysis in real time. British Politics, 16(1), 90–116. https://doi.org/10.1057/s41293-020-00150-8
- Council of Misters of Thai government. (2021). Labor market and businesses remedy measures (Section 33, Section 39, Section 40, and Section 9).
- Dzator, J., Acheampong, A. O., Dzator, M., Paolucci, F., Yawe, B. L., Asmah, E. E., Andoh, F. K., Kabagenyi, A., & Gillespie, J. (2021, November). Policy stringency, handwashing and COVID-19 cases: Evidence from global dataset. Health Policy and Technology, 11(2), 100574. https://doi.org/10.1016/j.hlpt.2021.100574
- Eggoh, J. C., & Khan, M. (2014). On the nonlinear relationship between inflation and economic growth. Research in Economics, 68(2), 133–143. https://doi.org/10.1016/j.rie.2014.01.001
- Farooq, O., & Ahmed, N. (2018). Does inflation affect sensitivity of investment to stock prices? Evidence from emerging markets. Finance Research Letters, 25(June 2017), 160–164. https://doi.org/10.1016/j.frl.2017.10.019
- Gao, Y., Li, Y. Y., & Wang, Y. (2021). Modular policy evaluation system: A policy evaluation framework based on text mining. 2021 IEEE 6th International Conference on Big Data Analytics, ICBDA 2021 (204–209). https://doi.org/10.1109/ICBDA51983.2021.9403142
- Geng, J., Long, R., Chen, H., & Li, Q. (2018). Urban residents’ response to and evaluation of low-carbon travel policies: Evidence from a survey of five eastern cities in China. Journal of Environmental Management, 217, 47–55. https://doi.org/10.1016/j.jenvman.2018.03.091
- Gokal, V., & Hanif, S. (2004). Relationship between inflation and economic growth. Working Paper, Reserve Bank of Fiji. (Vol. 04, Issue December 2004).
- Hale, T., Angrist, N., Goldszmidt, R., Kira, B., Petherick, A., Phillips, T., Webster, S., Cameron-Blake, E., Hallas, L., Majumdar, S., & Tatlow, H. (2021). A global panel database of pandemic policies (Oxford COVID-19 government response tracker). Nature Human Behaviour, 5(4), 529–538. https://doi.org/10.1038/s41562-021-01079-8
- Hsieh, H. S. (2020). Transport policy evaluation based on elasticity analysis with social interactions. Transportation Research Part A: Policy and Practice, 139(March 2019), 273–296. https://doi.org/10.1016/j.tra.2020.07.011
- Kirange, D. K., & Deshmukh, R. R. (2016). Sentiment analysis of news headlines for stock price prediction. An International Journal Of Advanced Computer Technology, 1–6. https://doi.org/10.13140/RG.2.1.4606.3765
- Kuang, B., Han, J., Lu, X., Zhang, X., & Fan, X. (2020). Quantitative evaluation of China’s cultivated land protection policies based on the PMC-Index model. Land Use Policy, 99(2020), 105062. https://doi.org/10.1016/j.landusepol.2020.105062
- Li, Q., Wang, T., Li, P., Liu, L., Gong, Q., & Chen, Y. (2014). The effect of news and public mood on stock movements. Information Sciences, 278, 826–840. https://doi.org/10.1016/j.ins.2014.03.096
- Lou, J., Wong, T. W., Fung, K. W. T., & Shaende, J. J. N. (2021). Stock and bond joint pricing, consumption surplus, and inflation news. Research in International Business and Finance, 58(June), 101426. https://doi.org/10.1016/j.ribaf.2021.101426
- Ministry of Public Health of Thailand. (2018). Royal Thai Government Gazette. Royal Thai Government, 136, 5–133. https://www.thaigov.go.th/
- Naseer, M., & Bin Tariq, Y. (2015). The Efficient market hypothesis: A critical review of the literature capital budgeting view project the Efficient market hypothesis: A critical review of the literature view project. IUP Journal of Financial Risk Management, 12(4), 48–63. https://www.researchgate.net/publication/289952213
- Office of the International Parliamentary Organization. (2021). The Summary of Measures and Operations of Thai Government on COVID-19 situation. (Issue March 2021).
- Özer, M. (2020). Türkiye’de COVID-19 Salgını Sürecinde Milli Eğitim Bakanlığı Tarafından Atılan Politika Adımları. Kastamonu Eğitim Dergisi, 28(3), 1124–1129. https://doi.org/10.24106/kefdergi.722280
- Ozmete, E., & Hira, T. (2011). Conceptual analysis of behavioral theories/models: Application to financial behavior. European Journal of Social Sciences, 18(2011), 386–404.
- René Wies. (1996 September). Policy definition and Classification: Aspects, criteria, and examples policy definition and Classification: Aspects, criteria, and examples 1 introduction: Differences in policy definitions. Proceeding of the IFIP/IEEE InternationalWorkshop on Distributed Systems: Operations & Management (pp.1–12). https://www.researchgate.net/profile/Rene_Wies/publication/2784392_Policy_Definition_and_Classification_Aspects_Criteria_and_Examples/links/00b4952eb61a7acb04000000.pdf
- Ritchie, H., Mathieu, E., Rodés-Guirao, L., Appel, C., Giattino, C., Ortiz-Ospina, E., Hasell, J., Macdonald, B., Beltekian, D., & Roser, M. (2020). Coronavirus Pandemic (COVID-19). Our World in Data. https://ourworldindata.org/coronavirus
- Ruiz Estrada, M. A. (2012). The Policy Modeling Research Consistency Index (PMC-Index). SSRN Electronic Journal, 1–13. https://doi.org/10.2139/ssrn.1689475
- Shah, D., Isah, H., & Zulkernine, F. (2019 May). Predicting the effects of news sentiments on the stock market. Proceedings - 2018 IEEE International Conference on Big Data, Big Data 2018 (pp. 4705–4708). https://doi.org/10.1109/BigData.2018.8621884
- The Stock Exchange of Thailand. (n.d.). SETTRADE.COM - Leading Technology for professional investors. Retrieved August 19, 2022, from https://classic.settrade.com/settrade/home
- Thailand Ministry of Commerce. (n.d.). Ministry of Commerce, Thailand. Retrieved November 2, 2022, from https://www.moc.go.th/en/page/item/index/id/6
- Toraksa, S., & Saengkrod, W. (2019). Translating policy to practice in the control and prevention of covid-19: Case study of chumpae municipality. Journal of Modern Learning Development, 7(7), 248–262.
- Vegvari, C., Abbott, S., Ball, F., Brooks-Pollock, E., Challen, R., Collyer, B. S., Dangerfield, C., Gog, J. R., Gostic, K. M., Heffernan, J. M., Hollingsworth, T. D., Isham, V., Kenah, E., Mollison, D., Panovska-Griffiths, J., Pellis, L., Roberts, M. G., Scalia Tomba, G., Thompson, R. N., & Trapman, P. (2021). Commentary on the use of the reproduction number R during the COVID-19 pandemic. Statistical Methods in Medical Research, 31(9), 1675–1685. https://doi.org/10.1177/09622802211037079
- World Health Organization Thailand. (2022). COVID-19 Situation, Thailand Contents COVID-19 Update. (Issue 12 January 2022).