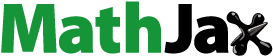
Abstract
To foster maize productivity and advance agricultural development, the Government of Ghana (GoG) has enacted several flagship programmes as a response to the pressing need to improve the sector. Planting for food and jobs (PFJ) is one such programme. This study investigates the impact of PFJ participation on maize productivity and income amongst maize farmers in northern Ghana. The study sampled 384 maize farmers. Cross-sectional data were collected and analysed using the probit model, propensity score matching, and Dolby robust estimator. The estimation shows that PFJ participation had a positive effect on maize productivity and farmers’ income. It was also observed that PFJ participation was influenced by gender, farming experience, education, access to credit, cooperative membership, and farm distance. Limited fertilizer access and restricted market access were the major challenges that militated against PFJ programme participation. Thus, Government of Ghana (GoG), through the Ministry of Food and Agriculture (MoFA), prioritised the provision of subsidised fertiliser to farmers who would otherwise struggle to purchase enough fertiliser to increase productivity and income. This could greatly reduce the impact of the crowding out phenomenon on the PFJ programme.
REVIEWING EDITOR:
1. Introduction
Agriculture has been widely acknowledged as a critical component in the fight against poverty in Africa (Adu et al., Citation2018). The agricultural sector has made significant contributions to the United Nations Sustainable Development Goals (SDGs) of ending poverty and hunger (FAO, Citation2023b). These positive global outlooks have prompted Ghana to invest in sustainable agriculture. Currently, the agricultural sector is a vital part of Ghana’s economy, accounting for 18.5% of the country’s GDP in 2019 and employing 52% of the labor force (FAO, Citation2023a; GSS, Citation2020). Despite its substantial contributions to Ghana’s economic development, the agricultural sector faces several challenges. One of the most significant challenges is the low yield of staples and cash crops (Ali et al., Citation2021). Other challenges include limited access to credit, unpredictable weather patterns, low soil fertility, pest, and disease incidence, and inadequate utilisation of improved agricultural technology. Within this context, the Government of Ghana (GoG), through successive administrations, has implemented various policies and programmes aimed at revitalising the agricultural sector. These policies, as outlined by Appiah-Twumasi (Citation2019), include the Food and Agricultural Sector Development Policy (FASDEP I, 2002), which sought to transform the agricultural sector, as well as FASDEP II (2007), which revised FASDEP I, and the Medium-Term Agriculture Sector Plan (METASIP 2010–2015), which served as an investment approach for implementing FASDEP II. To optimise the implementation of these policies, the Government also initiated the Fertilizer Subsidy Programme (FSP), Block Farm Programme, and the Planting for Food and Jobs (PFJ) Programme.
The inception of the Planting for Food and Jobs (PFJ) programme in 2017 was aimed at addressing the declining agricultural output and providing sustainable employment opportunities for farmers. The programme is supported by five key pillars, namely subsidized seed and fertilizer supplies, marketing options after harvest, free extension services, and an E-Agriculture database system to track farmers’ progress. Currently, the programme focuses on five major crops: rice, maize, sorghum, soybeans, and vegetables (onion, tomato, and chili pepper). However, the Ghanaian agricultural minister reported that ∼70% of the target farmers were not interested in participating in the PFJ programme (Tekuni et al., Citation2021). Similarly, Pauw (Citation2022) noted that as of 2020, only 1.74 million out of an estimated 2.6 million agricultural households had participated in the programme. Therefore, understanding the factors that drive farmers’ participation in the PFJ programme is a critical research question.
Tanko et al. (Citation2019) undertook a study to examine the effect of the Planting for Food and Jobs (PFJ) programme on the productivity of rice farmers in Northern Ghana. The findings of their research indicated that the PFJ programme had a positive influence on rice production. However, as the programme was still relatively new and farmers did not have a complete understanding of it at the time, the study’s results and estimates of impact may have been misleading. As the PFJ programme, which was scheduled to run from 2017 to 2020, has now ended, a comprehensive impact assessment can now be conducted on agricultural productivity. It is important to note that minor changes in the policy over the years may have affected farm outcomes, which could differ from those observed when the policy was first introduced in 2017.
In Ghana, the majority of studies conducted on the Planting for Food and Jobs (PFJ) programme have focused on rice production (see Tanko et al., Citation2019; Zoogah & Nakuja, Citation2020), with little attention given to maize production. Ansah et al. (Citation2020) and Lambongang et al. (Citation2019) have investigated the factors affecting PFJ participation among maize farmers in the Northern region of Ghana. Despite this, there is a research gap regarding the scope and time frame of the PFJ programme. The programme has ended, and changes in policy over the years may have impacted farm outcomes differently from when the programme was first introduced in 2017. Therefore, the present study aims to provide a more comprehensive impact assessment on agricultural productivity. The findings of this research will inform stakeholders on how to support the programme and identify solutions to challenges for effective implementation to increase productivity, food security, and the welfare of smallholder maize farmers.
The study focuses on the impact of the PFJ programme participation on maize productivity in northern Ghana. Specifically, it
Assess the factors influencing maize farmers’ participation in the PFJ programme in Northern Ghana
Evaluate the impact of PFJ participation on maize productivity and welfare (i.e. Income/acre) of maize farmers in Northern Ghana
Identify the challenges affecting PFJ participation amongst maize farmers.
2. Literature review
2.1. Agricultural projects participation: the determining factors known so far
The determinants of agricultural project participation, such as Ghana’s PFJ could be largely grouped into social, economic, political, physical, and environmental factors. Farmer’s participation in agricultural projects directly affects technology awareness, adoption, livelihoods, poverty, environment, agricultural sector performance, and the economy at large (Etwire et al., Citation2013). Considering these contemporaneous benefits, researchers have been motivated to conduct empirical studies on the determinants of agricultural projects. Whilst Ismaila and Tanko (Citation2021) identified that nepotism significantly affects PFJ participation positively, Lambongang et al. (Citation2019) reported that farmer’s experience is an imperative determinant of PFJ.
In a similar vein, Wiredu et al. (Citation2013) found a positive relationship between rice development projects participation and socio-economic indices including household size, access to credit, off-farm income, and market price while Ansah et al. (Citation2020) also revealed that age is an imperative determinant of PFJ participation. This is because as the farmer ages, he/she gains much influence than the young in agricultural projects, such as PFJ. In the Ghanaian context, adults’ (particularly, male adult) suggestions are highly appreciated than the young due to the traditional values and norms. In so doing, older individuals would have the propensity of gaining political leverage which would increase their chances of being included in the PFJ programme. Albeit, men have access to productive resources, such as land, the aged have robust political alliance and social networks which makes them influential relative to the young. Conversely, increasing farm size decreases agricultural project participation (Nwaobiala, Citation2014).
In addition, Awotodunbo et al. (Citation2022) in their study on cocoa farmer’s participation in the Agricultural Transformation Agenda (ATA) programme in western Nigeria found that years of experience, marital status, cooperative membership, sex, household size, and years of formal education significantly influenced ATA participation. Their results further revealed that ∼90% of cocoa farmers participated in 8 out of 12 ATA programme identified. This indicates a high level of participation. Farm distance and number of extension visit received are critical factors to agricultural projects developments in South Africa (Afful & Mafsikaneng, Citation2018). Our investigation to identify important factors determining maize farmer’s participation in PFJ is placed within this context.
2.2. Impact assessment of agricultural projects on productivity and income
Numerous studies have assessed the impact of agricultural projects on agricultural productivity and farmer’s well-being. Some studies (Adeyeye et al., Citation2021; Attipoe et al., Citation2021; Danso-Abbeam et al., Citation2018) have investigated the economic impact of agricultural project participation in Sub-Saharan Africa (SSA). A recent study by Mokgomo et al. (Citation2022) showed that albeit the proportion of households participating in the government agricultural support programme in South Africa had marginally decreased from 16 to 14% in 2013 and 2016, respectively, the impact on agricultural productivity and income had improved and food insecurity reduced. Davis et al. (Citation2012) also found that Farmer Field Schools (FFSs) participation in Kenya, Uganda, and Tanzania increased productivity and income aggregately. However, differential impacts were observed on a country level. In detail, Kenya’s crop productivity increased by 80%, Tanzania’s agricultural income beyond 100% whereas the marginal impact of FFSs in Uganda was the resultant effect of their National Agricultural Advisory Services. Martey et al. (Citation2021) offered viewpoints on the effects of a novel comprehensive agricultural training programme (CATP) on productivity, income, and cowpea adoption in northern Ghana. The study revealed that CATP participation, respectively increased productivity, cowpea income, and climate-smart cowpea adoption by 15, 24, and 75% points relative to their average levels. Nakano et al. (Citation2018) noted that after agricultural training in Tanzania, key rice farmers observed an increase in yield from 3.1 to 5.3 tons/hectare whereas for every hectare ordinary farmer had yield increase from 2.6 to 3.7 tons/hectare.
Agricultural projects play an indispensable role in community development. Its function in evaluating and distributing agricultural knowledge and technology based on research to rural people leads to advancements in the agricultural sector (Maulu et al., Citation2021).
Notwithstanding, empirical studies existing on the impact of PFJ participation on maize productivity and farmer’s income are limited in Ghana. Given the extreme poverty and food insecurity in the area, northern Ghana makes an intriguing study location. Second, the region has enjoyed a great deal of development initiatives with the focus of abolishing poverty and promoting sustainable environment (Martey et al., Citation2019). In this study, we focus on the maize productivity and maize farmer’s income using propensity score matching (PSM) and inverse probability weighted regression adjustment (IPWRA). To the best of our knowledge, as compared to earlier studies (see Tanko et al., Citation2019; Zoogah & Nakuja, Citation2020), the robust estimating approaches deployed in this study offer a deeper understanding of the function that PFJ participation plays in farm productivity and income.
3. Materials and methods
3.1. Study area
Northern Ghana has five regions namely; Northern, Upper East, Upper West, Savannah, and North-East regions (GSS, Citation2021). However, the study focused on the Northern, Upper-East, and Upper West regions. Given the extreme poverty and food insecurity in the area, northern Ghana makes an intriguing study location. Second, the region has enjoyed a great deal of development initiatives with the focus of abolishing poverty and promoting sustainable environment (Martey et al., Citation2019). These three regions combined covers about 41% of Ghana’s total land area (SRID, Citation2016). The area has a generally dry climate with just one rainy season that lasts from May to October (GSS, Citation2013). Between 750 and 1050 mm of rain are measured annually. Maximum temperatures are experienced around the end of the dry season (March-April), and minimum temperatures are experienced in December and January (GSS, Citation2013). The main economic pursuits are agriculture, forestry, and hunting (MoGCSP, Citation2014). Maize, millet, rice, sorghum, groundnut, cowpea, and yam are the main agricultural products cultivated by farmers (Adzawla & Alhassan, Citation2021). shows map of the study area.
3.2. Sampling and data collection methods
In this study, the multistage sampling approach was employed to sample 384 maize farmers in Northern Ghana. Six districts out of the three regions were purposively selected based on high quantity of maize produced and farmers’ access to farm inputs (Refer to ). Out of the six districts, a total of 12 villages were simple randomly selected with two villages from each district for the survey. This was done by listing the villages with high number of maize farmers and assigning numbers to these villages. Randomly, these numbers were picked, resulting in the selected villages. Consequently, 384 maize farmers were simple randomly selected with the lottery method for the study ().
Table 1. Estimated maize production in the study area.
Table 2. Regional distribution of sampled maize farmers.
The list of registered maize farmers in all the 12 villages in the six districts was sourced from the District Department of Agriculture. From the list, considering the PFJ programme, an estimated number of 4523 maize farmers from the Karaga and Kpandai districts of the Northern region, 3811 in the Bolgatanga East and Binduri districts in the Upper East region and 1087 maize farmers in the Nadowli-Kaleo and Wa East districts in the Upper West region have been registered. A total population of 9421 maize farmers constitute the sampling framework from which the sample size was extrapolated. Employing Yamane’s (Citation1967) sample size determination approach ( where
sample size, N = Population of maize farmers aware of PFJ programme, e = margin of error (5%), the calculated sample size is ∼384.
Nonetheless, due to mis-specified data from 14 maize producers the sample size was reduced to 370 for analysis. Financial constraint affected the collection of additional data above the minimum sample size of 384. Besides, the central limit theorem indicates that a sample size ≥30 is appropriate for a standard normal deviation (Amrago & Mensah, Citation2023). In so doing, the sample size of 370 is an adequate representation of the target population in the study site, and that the results could be generalised. reports the districts and the corresponding communities chosen.
Table 3. Districts with their respective villages sampled for the study.
A cross-sectional survey was carried out amongst the selected maize farmers in northern Ghana from 10 January to 15 February 2023. The farmers were interviewed with a structured questionnaire. This questionnaire contained questions relating to maize farmers socio-economic characteristics, benefits of PFJ, the influencing factors of PFJ participation, maize productivity, maize income, constraints to PFJ participation, amongst others. The questionnaire was pre-tested on 30 maize farmers and the response was instrumental in refining the instrument for the actual data collection. The data collection tool used was a structured questionnaire through a face-to-face interview.
In , two districts were selected from each region to foster geographic diversity and representation. The same number of farmers from each village ensured consistency and study comparability. These reduced possible biases associated with village size variations.
3.3. Ethical declarations
The University of Energy and Natural Resources’ Committee for Human Research and Ethics (CHRE) officially declares that the research proposal with the title ‘Planting for Food and Jobs Participation and Its Impact on Maize Productivity in Ghana: Implications for Research and Policy’ has been granted a waiver of permission in compliance with our ethical standards and values. The approval was waived on this justification: The CHRE carried out thorough analyses and reviews of the research methodology and design. It was found that the research’s potential dangers to the participants are low at best. The safety, privacy, and anonymity of the participants have all been guaranteed throughout the research procedure by taking all essential safeguards.
3.4. Methods of data analysis
In this research endeavor, descriptive and inferential statistics were utilised. The mean was employed to determine the central position of the variables present in the dataset. The propensity score matching and inverse probability weighted regression adjustment techniques were implemented to assess the influence of PFJ participation on maize productivity and farmers’ income. Before the commencement of the study, participants were informed about the objectives, methods, potential risks, and benefits, as well as their rights as participants. They voluntarily and formally consented to participate after receiving thorough information. No data was collected or analysed until after they had given their informed consent.
3.4.1. Conceptual framework and estimation strategies
A sequential decision-making process is used to conceptualize the participation in Planting for Food and Jobs (PFJ) and its impact on maize productivity and maize income, where the decision to participate predetermines productivity and income. PFJ participation is a behavioral response and thus modeled in the framework of random utility theory. In the random utility framework (RUF), farmers encounter a set of constraints and alternatives (Asfaw et al., Citation2012; Kangile et al., Citation2021; Ward et al., Citation2016). The farmer as a rational decision maker opts to participate in PFJ if the utility obtained from participation () maximizes that of non-participation (
) such that
represents the difference between the two mutually exclusive utilities. Following Martey et al. (Citation2019), the utility difference is expressed as:
(1)
(1)
However, because the two utilities are unobservable, they can be described in the latent variable model below as a function of observable elements:
(2)
(2)
where
is a binary response variable for PFJ participation if
and non-participation if
;
is a vector of regressors, such as socio-economic and institutional characteristics
is a vector of parameters to be estimated and
is the stochastic error.PFJ participation is expected to affect maize productivity and maize income. The outcome variable is expressed as a linear function of observed variables and the dichotomous response variable,
expressed as:
(3)
(3)
where
signifies the outcome variables (maize productivity and maize income),
is the already defined PFJ participation variable,
and
are parameters to be estimated and
is an error term. Because the farmers were not randomly allocated, estimates of
will be biased. Participants are usually different from non-participants due to selection bias and this could dampen the effect on the outcome variables (Janvry et al., Citation2011). Ordinary least squares (OLS) estimation of Equationequation (3)
(3)
(3) results in inaccurate estimates. Redressing this drawback, we employ the propensity score matching (PSM) and inverse probability weighted regression adjustment (IPWRA) models. The selection bias rejects the use of the OLS. Owing to this deficiency, the PSM is explored. The PSM eliminates selection bias and produces accurate estimates by adjusting for observable confounding variables.
3.4.2. Factors influencing maize farmers’ participation in the PFJ programme
In this study, maize farmer’s decision to participate in the PFJ programme is a qualitative choice. The study of qualitative choice is concerned with the proper formulation, estimation, and application of models for the probability of events, where the ‘event’ is often a person’s decision among a variety of options (Greene, Citation2003). In our case, the maize farmer is faced with the option of participating in the PFJ programme (Y = 1) or otherwise (Y = 0). In this situation, where the dependent variable (i.e. PFJ participation, Y) is dichotomous in nature, Woo et al. (Citation2022) suggest the use of the probit or logit model. Albeit the probit and logit models are commonplace for a binary dependent variable, the standard normal distribution function of probit is worthy of analysis. Besides, Botlhoko and Oladele (Citation2013), Etwire et al. (Citation2013), Nxumalo and Oladele (Citation2013), and Wiredu et al. (Citation2013) had used the probit model to investigate the factors influencing agricultural projects in South Africa and Ghana, respectively. To this end, we employ the probit model to underscore the factors influencing PFJ participation. Inferring from Osabuohien (Citation2014), the probit model is specified as:
(4)
(4)
Where, PFJ is farmer i participation in PFJ or otherwise; represents the standard normal cumulative distributive function;
denotes a set of PFJ participation influencing factors and
is an error term.
3.4.3. Impact of PFJ participation on maize productivity and maize income
The propensity score matching is a widely employed non-experimental method in the evaluation of literature, as evidenced by Lee (Citation2013). This approach, which has gained credibility due to Rosenbaum and Rubin (Citation1983), involves matching participants and non-participants based on their propensity scores following programme participation (Pufahl & Weiss, Citation2009). The validity of propensity score matching rests on the assumptions of conditional independence and common support. Specifically, conditional independence assumes that, given a set of observable covariates X, non-treatment outcomes are independent of the participation status. In simple terms, unobserved factors do not influence participation whereas the common support presumes that in the propensity score distribution, treatment observations have similar observations ‘nearby’. Unfortunately, no straight forward method exists to verify the conditional independence assumption. Given that, Mensah et al. (Citation2022) suggested the use of the Rosenbaum sensitivity analysis to observe the sensitivity of the estimates to bias resulting from unobserved variables. The common support assumption is validated with a propensity score distribution graph.
The average treatment effect (ATE) and average treatment effect on the treated (ATT) have been the common evaluation parameters in PSM analysis. Yet in this study, the ATT is used to estimate the impact of PFJ participation on maize productivity and maize income via four matching algorithms, namely: nearest-neighbour, radius, stratification, and kernel matching. Albeit the compendium of these matching methods makes the results robust, we further employ the inverse probability weighted regression adjustment (IPWRA) as robustness check to the impact estimate. Since the ATE estimate includes the impact on people for whom the programme was never designed, Heckman (Citation1997) warns that it could not be important to policy-makers. As a result, following Wonde et al. (Citation2022), the ATT is given as:
(5)
(5)
Where E is the average value, and
are the potential outcomes for participants and non-participants, respectively, and D denotes the binary treatment variable (Farmer participated in the PFJ programme).
If ATT has a positive (negative) value, it means that programme participants’ mean maize productivity and income (Y, the outcome variable) are higher (lower) than those of programme non-participants.
4. Results
4.1. Descriptive statistics
reports the differences in the outcome and independent variables based on participation status. Participants refer to maize farmers who engaged in the planting for food and jobs programme. They represent 59% of the sampled farmers. The results indicate significant variations between participants and non-participants in terms of maize productivity. Likewise, concerning farmers’ income, the differences between participants and non-participants were statistically significant. On the independent variables, participants are significantly distinguishable with regard to sex, farming experience, marital status, education, farm size, access to credit, cooperative membership, extension service, off-farm income, and farm distance. In comparison to non-participants, we found that participants have more years of farming experience. The positive difference indicates that more years of farming facilitates PFJ participation. The total farm size available and education reinforce the critical role that physical resources and human capital play in PFJ participation.
Table 4. Descriptive statistics of variables by participation status.
To this end, understanding how these factors (i.e. farm size, education amongst others) critically influence PFJ participation, we employ the probit regression model to quantitatively measure the influence of each factor on PFJ participation. This is demonstrated in section 3.2.
4.2. Factors influencing PFJ programme participation
The findings presented in indicate that several demographic and institutional factors have a significant influence on participation in the PFJ programme. Specifically, the results demonstrate that sex, farming experience, and education positively impact participation, while access to credit and cooperative membership also exhibit a positive influence. However, it is worth noting that access to credit has a negative effect on PFJ participation. Additionally, the data suggests that farm distance negatively affects farmers’ participation in the programme.
4.3. Impact of PFJ participation on maize productivity and maize income
shows the impact of PFJ participation on maize productivity and maize income. Inferring from , all the four matching algorithms thus the nearest neighbour matching, radius matching, kernel matching, and the stratification matching revealed a positive impact of PFJ programme participation on maize productivity and maize income. Likewise, the IPWRA. In general, the impact was above Gh¢ 3000.00 (USD 253.00) for maize productivity and Ghc 14,000.00 (USD 1182.00) for maize income.
Table 5. PSM estimates of PFJ participation.
4.4. Challenges affecting PFJ participation
presents the challenges militating against PFJ participation in the study site. The results indicate that limited fertilizer access (47%) is the most pressing challenge impeding the growth of the PFJ programme. This is followed by restricted market access (36%) and delayed input delivery (10%). The least pressing challenge reported was the low number of extension services (8%) to aid deliver experiential guidance.
5. Discussions
5.1. Factors influencing PFJ programme participation
displays the outcomes of the probit regression model, which was employed to examine the factors affecting participation in the PFJ programme. The results indicate that sex, farming experience, education, access to credit, cooperative membership, and farm distance significantly affects the participation in the PFJ programme. Specifically, sex, farming experience, education, and cooperative membership demonstrated a positive effect on participation in the PFJ programme, while access to credit and farm distance had an inverse association.
Table 6. Determinants of PFJ programme participation.
In detail, the gender variable’s positive effect reveals that male-headed households are more likely to participate in the PFJ programme than female-headed households by 10.6%. Households headed by women may have less access to the resources needed to participate in the PFJ Programme, such as land, credit, and inputs (Lambongang et al., Citation2019). This could be attributed to cultural customs or barriers to obtaining these resources. Besides, women in female-headed families may have conflicting obligations, such as child care and housework, which can limit their time for the PFJ programme participation. Men in male-headed households, however, might have the flexibility to participate in agricultural pursuits. This observation is consistent with Abdul-Hanan and Anang (Citation2018) who found that gender had a positive effect on the spraying programme in Ghana. The reason for this finding is that communities in northern Ghana are usually patriarchal in structure and this can have implications for the participation of women in such programmes when they are competing for the same resources with their male counterparts.
The positive effect of farming experience suggests that farmers with more experience will probably have established connections with consumers and marketing outlets, which will make it seamless for them to commercialise their produce. Due to their improved ability to capitalize on new market opportunities, they may have an advantage when participating in the PFJ Programme. These experienced farmers are also well equipped to control the risk associated with farming; weather fluctuation, pest and disease, and price volatility. With such, they are capable of managing the risk posed by implementing new farming methods and technologies. By a percentage increase of 1.8, we found that experienced farmers are likely to participate in the PFJ programme. Our result follows that of Mthombeni et al. (Citation2022) in South Africa.
From the result, we further found that the propensity of farmer participation in the PFJ programme increases with the years of education. Farmers with education may be more knowledgeable about agricultural technologies, such as improved seed varieties, irrigation systems, and pest control strategies. In so doing, they are likely to participate in the PFJ programme by 5.3%. The result is not surprising, as education has often been the greatest hallmark for agricultural projects participation. Notwithstanding, Etwire et al. (Citation2013) reported an inverse relationship between education and agricultural projects in the northern region of Ghana. Access to credit had a significant, yet negative effect on the PFJ programme participation. Thus, farmers are less likely to participate in the PFJ programme with increasing access to credit. The result seems counterintuitive; however, this could be that farmers invest in their farms by expanding their farms or acquiring new farm inputs. Moreover, with seamless credit access, farmers could decide not to participate in the PFJ programme which is likely to have restrictions and controls. Alternatively, they could have more opportunities to invest in other alternative investment vehicles, such as small business, mutual funds, bonds, stocks, and annuities. These investments could fetch them an attractive regular income, as such their demotivation to participate in the PFJ programme. The result contradicts the findings of Jamilu et al. (Citation2015). In the context of Nigeria, they found that access to credit positively influences agricultural project participation.
Moving forward, cooperative member farmers are more likely to participate in the PFJ programme than non-cooperative member farmers by 2%. We envisage that cooperative members have access to information on the PFJ Programme through their cooperative. The cooperative’s ability to inform its members about the programme and its advantages could increase participation. Finally, the increasing farm distance in minutes reduces the likelihood of farmer participation in the PFJ programme by 0.4%. The reason could be that the stress associated with carrying out farming activities in terms of transportation of inputs and outputs decreases with increasing proximity between home and the farm. In fact, because of the near proximity of their residences to their fields, farmers who are closer to their operations are able to fully utilize their internal resources. In simple terms, further distance translates to increased commuting time and transportation costs. This makes it logistically challenging for farmers to attend the PFJ programme activities regularly. Particularly, this can be a burden upon farmers who may have already tight schedules and limited resources. The travel inconvenience could discourage participation, limiting farmers access to valuable resources, support, and information offered by the PFJ programme. Likewise, Lambongang et al. (Citation2019) revealed that farm distance negatively affects PFJ participation in the Bunkpurugu-Yunyoo district of Ghana.
Nevertheless, the propensity scores established from the probit model in are used for the matching in the PSM analysis. As a result, we conduct a thorough investigation to evaluate the impact of PFJ participation on the outcomes (Refer to ).
Table 7. Variables measurement and hypothesised effect in the probit model.
5.2. Impact of PFJ participation on maize productivity and maize income
The propensity score distribution of PFJ participants and non-participants for maize productivity and farmers’ income is presented in and , respectively.
Albeit the majority of participants and non-participants shared a support region, it was found that some participants were outside the region. By excluding farmers in the off-support region, matches were limited to farmers who fall into the participants and non-participants groups. The wide distribution of the common support region validates PSM use.
highlights the PSM estimates of the impact of PFJ participation on maize productivity and farmers’ income. All the matching algorithms demonstrated a significant and positive impact of PFJ participation on maize productivity and income. Participants of the PFJ programme reported ∼3911.398 kg/acre increase in their maize productivity on average relative to non-participants (). The Dolby robust estimator, thus the IPWRA was used to check the robustness of the results. The results showed that PFJ participants recorded 3815.936 kg/acre in maize productivity (). This indicates that on several estimation techniques, the results are robust. In addition, the IPWRA overlap assumption was not violated because both estimated densities have their respective masses overlapping and neither plot show severe probability mass near 0 or 1 (). The finding is no different from the study of Hailu et al. (Citation2014), Mokgomo et al. (Citation2022), and Prah et al. (Citation2023) in Ghana, South Africa, and Northern Ethiopia, respectively. They found that agricultural project participation had a significant positive impact on farm productivity. Conversely, in the northern region of Ghana, rice yield decreases as fertilizer prices increase (Tanko et al., Citation2016). Care must be taken when interpreting the data as the results is subject to hidden bias (Refer to Appendix A). That is, we cannot confidently report that the estimated maize productivity impact was solely due to the PFJ participation.
Another metric to assess the impact of the agriculture industry is farm income. The propensity score estimate demonstrated that participating in the PFJ programme had a positive effect on income. According to the matched sample, farmers with PFJ participation had a higher income of 14,767.04 Ghana Cedis (US$ 1247.21) than farmers without PFJ participation. The finding is in line with previous research by Martey et al. (Citation2021) and Samanta (Citation2023) stating that agricultural project participation is imperative for increasing farm income.
Agricultural initiatives, such as extension services, can bolster the resilience of small-scale farmers by offering advisory assistance that facilitates well-informed decisions for improved farm income (Baiyegunhi et al., Citation2019). However, Martey et al. (Citation2015) reported that exposure to agricultural development projects does not necessarily lead to higher farm income in Ghana. Risk-averse farmers, who have experienced limitations in the past and face external constraints, may choose not to immediately apply the advice they receive through agricultural mentorship programmes. Nonetheless, the long-term effects of participation may become evident and impact farm income. The finding of 14,845.43 Ghana Cedis (US$ 1253.84) on the IPWRA estimate corroborates the estimates of the four matching algorithms, indicating that the impact estimate on maize income is robust to various estimation methods. The overlap plot shown in suggests that the estimated densities have considerable overlap, implying the absence of overlap violation. The Rosenbaum sensitivity analysis (refer to Appendix A) confirms that the result is not influenced by unobserved heterogeneity, as the Sig+ was significantly different from zero in gamma 1–2. Therefore, the result can be interpreted without caution.
5.3. Challenges affecting PFJ participation
Albeit the PFJ programme participation had positive impacts on maize productivity and farmer’s income, having a comprehensive understanding of the challenges limiting the programme potential outcomes is imperative for Ghana’s agricultural development. In so doing, we present the result of these challenges in . Explicitly, limited access to fertilizer was a significant (47%) bottleneck to the PFJ programme progression. This, Pauw (Citation2022) referred to as the crowding out challenge. Crowding out is frequently observed as a significant challenge in the underperformance of subsidy programmes in Sub-Saharan Africa’s production effects (Jayne et al., Citation2018). Crowding out focuses on farmers who would have bought commercial fertilizer in the absence of the subsidy. When farmers transition from commercial to subsidized fertilizer, the net infusion of fertilizer into the system decreases; this reduces marginal productivity benefits without reducing programme costs (Pauw, Citation2022).
Table 8. Challenges militating against PFJ participation.
Restricted market access was also an imperative challenge to the PFJ programme development. This can be the result of poor storage facilities, insufficient transportation infrastructure, or trade restrictions. With debilitating market access, farmers are less motivated to participate in the PFJ programme, as they have poor commercialization capacity. Likewise, Lambongang et al. (Citation2019) reported that limited market access was a challenge among maize farmers in the Bunkpurugu-Yunyoo District of Ghana. However, delayed input delivery and inadequate extension services were not that of a major challenge to the PFJ programme participation as their respective percentages were 9 and 8.
6. Conclusions and recommendations
The study focuses on the impact of the PFJ programme on maize productivity and income amongst maize farmers in Northern Ghana using an econometric model that controls for selection bias and a Dolby robust estimator for robustness check. The results indicate that gender, farming experience, education, access to credit, cooperative membership, and farm distance influence the participation in the PFJ programme in northern Ghana, which suggests that these characteristics should be considered while promoting PFJ programme to maize farmers to ensure maximum participation. The result suggests that PFJ participation significantly increased maize productivity and income. The findings show that PFJ participation has the capacity to improve farmers’ wellbeing; hence, previous and ongoing support for the PFJ programme should be strengthened to help maize farmers gain more farm input with a ripple impact on the populace. Limited fertilizer access and restricted market access were the major challenges militating against the PFJ programme participation. As such, the Government of Ghana (GoG), through the Ministry of Food and Agriculture (MoFA) should improve collaboration with fertilizer firms to streamline distribution channels and enhance rural market accessibility.
6.1. Implications for practice
The study indicated that the PFJ programme had a favorable impact on maize productivity and income. Therefore, it is suggested that the Ministry of Food and Agriculture (MoFA), Agricultural and Rural Development Association (ARA), Farmers Organisation Network in Ghana (FONG), and other relevant Ghanaian agricultural organizations could support the development of the PFJ programme by providing the Government of Ghana (GoG) with experiential advice on initiating phase 2 of the programme. As productivity is directly linked to income, the well-being of farmers would be sustainably maintained, contributing to the global sustainable development goals (SDGs) of ending poverty (SDG 1) and achieving zero hunger (SDG 2).
The challenge of limited access to fertiliser was a significant obstacle for farmers participating in the Planting for Food and Jobs (PFJ) programme. In light of this, it is recommended that the Government of Ghana, through the Ministry of Food and Agriculture, prioritise providing subsidised fertiliser to farmers who would otherwise struggle to purchase enough fertiliser to increase productivity and income. This could greatly reduce the impact of the crowding out phenomenon on the PFJ programme.
6.2. Economic/commercial impact
GoG through MoFA should encourage collaborations between the public and private sectors to aid in the development of infrastructure, the adoption of advanced farming techniques, and the launch of agribusiness projects. These collaborations may enhance financial support for the agricultural industry and generate job possibilities.
6.3. Limitations
We recognize that the use of cross-sectional data for the impact evaluation may provide challenges because it is unable to capture pertinent time-variant characteristics. Given that the sample represents the main maize-producing areas in Northern Ghana, the welfare effect cannot be applied to all farmers in that region. We emphasize that the results should be viewed carefully in light of these flaws.
6.4. Future research
Future research lines should investigate PFJ participation impact on the poverty status of maize farmers using per capita expenditure, poverty headcount ratio, and per capita food expenditure as proxy variables for poverty. From a policy standpoint, expanding access to farm inputs is essential for promoting the participation in the PFJ programme and improving welfare results.
Disclosure statement
No potential conflict of interest was reported by the author(s).
References
- Abdul-Hanan, A., & Anang, B. (2018). Factors influencing participation of cocoa farmers in the government spraying programme in Ghana. Asian Journal of Agricultural Extension, Economics & Sociology, 22(2), 1–17. https://doi.org/10.9734/AJAEES/2018/38842
- Adeyeye, B., Amodu, L., Odiboh, O., Oyesomi, K., Adesina, E., & Yartey, D. (2021). Agricultural radio programmes in indigenous languages and agricultural productivity in North-Central Nigeria. Sustainability, 13(7), 3929. https://doi.org/10.3390/su13073929
- Adu, M. O., Yawson, D. O., Armah, F. A., Abano, E. E., & Quansah, R. (2018). Systematic review of the effects of agricultural interventions on food security in northern Ghana. PLOS One, 13(9), e0203605. https://doi.org/10.1371/journal.pone.0203605
- Adzawla, W., & Alhassan, H. (2021). Effects of climate adaptation on technical efficiency of maize production in Northern Ghana. Agricultural and Food Economics, 9(1), 1–18. https://doi.org/10.1186/s40100-021-00183-7
- Afful, D. B., & Mafsikaneng, A. N. (2018). Assessment of factors influencing farmers’ participation in the comprehensive agricultural support programme in Tshwane Metropolitan Municipality, South Africa. South African Journal of Agricultural Extension, 46(2), 79–88. https://doi.org/10.17159/2413-3221/2018/v46n2a465
- Ali, E. B., Agyekum, E. B., & Adadi, P. (2021). Agriculture for sustainable development: A SWOT-AHP assessment of Ghana’s planting for food and jobs initiative. Sustainability, 13(2), 628. https://doi.org/10.3390/su13020628
- Amrago, E. C., & Mensah, N. O. (2023). Trade credit from agrochemical vendors as an alternative source of finance for cabbage producers in the Bono East Region of Ghana. Agricultural Finance Review, 83(1), 43–82. https://doi.org/10.1108/AFR-11-2021-0155
- Ansah, I. G., Lambongang, M., & Donkoh, S. A. (2020). Ghana’s planting for food and jobs programme: A look at the role of capability in farmers’ participation. Journal of Human Development and Capabilities, 21(2), 161–182. https://doi.org/10.1080/19452829.2020.1745162
- Appiah-Twumasi, M. A. R. K. (2019). Farmer innovations in agricultural financing and economic efficiency of maize production in the Northern Region of Ghana [Doctoral dissertation]. http://hdl.handle.net/123456789/2267
- Asfaw, S., Shiferaw, B., Simtowe, F., & Lipper, L. (2012). Impact of modern agricultural technologies on smallholder welfare: Evidence from Tanzania and Ethiopia. Food Policy, 37(3), 283–295. https://doi.org/10.1016/j.foodpol.2012.02.013
- Attipoe, S. G., Cao, J. M., Opoku-Kwanowaa, Y., & Ohene-Sefa, F. (2021). Assessing the impact of non-governmental organization’s extension programs on sustainable cocoa production and household income in Ghana. Journal of Integrative Agriculture, 20(10), 2820–2836. https://doi.org/10.1016/S2095-3119(21)63607-9
- Awotodunbo, A. A., Musa, M. W., Omokore, D. F., & Sanni, A. A. (2022). Factors influencing level of participation of cocoa farmers in agricultural transformation agenda in Ondo and Ekiti States, Nigeria. Journal of Agriculture and Applied Biotechnology, 1(1), 7–13. https://doi.org/10.52493/j.jaab.2022.1.47
- Baiyegunhi, L. J. S., Majokweni, Z. P., & Ferrer, S. R. D. (2019). Impact of outsourced agricultural extension program on smallholder farmers’ net farm income in Msinga, KwaZulu-Natal, South Africa. Technology in Society, 57, 1–7. https://doi.org/10.1016/j.techsoc.2018.11.003
- Botlhoko, G. J., & Oladele, O. I. (2013). Factors affecting farmers participation in agricultural Projects in NgakaModiriMolema district north west province, South Africa. Journal of Human Ecology, 41(3), 201–206. https://doi.org/10.1080/09709274.2013.11906568
- Danso-Abbeam, G., Ehiakpor, D. S., & Aidoo, R. (2018). Agricultural extension and its effects on farm productivity and income: insight from Northern Ghana. Agriculture & Food Security, 7(1), 1–10. https://doi.org/10.1186/s40066-018-0225-x
- Davis, K., Nkonya, E., Kato, E., Mekonnen, D. A., Odendo, M., Miiro, R., & Nkuba, J. (2012). Impact of farmer field schools on agricultural productivity and poverty in East Africa. World Development, 40(2), 402–413. https://doi.org/10.1016/j.worlddev.2011.05.019
- Etwire, P. M., Dogbe, W., Wiredu, A. N., Martey, E., Etwire, E., & Robert, K. (2013). Factors influencing farmer’s participation in agricultural projects: The case of the agricultural value chain mentorship project in the northern region of Ghana. Journal of Economics and Sustainable Development, 14(10), 36–43.
- FAO. (2023a). Retrieved June 2, 2023, from fromhttps://www.fao.org/ghana/fao-in-ghana/ghana-at-a-glance/en
- FAO. (2023b). Retrieved June 2, 2023, from https://www.fao.org/sustainable-development-goals/overview/fao-and-the-2030-agenda-for-sustainable-development/poverty-eradication/en/
- Ghana Statistical Service. (2013). 2010 Population and housing census, regional analytical report, northern region.
- Ghana Statistical Service. (2020). Retrieved June 2, 2023, from https://www.statsghana.gov.gh/
- Ghana Statistical Service. (2021). Ghana 2021 population and housing census. General report volume 3A.
- Greene, W. (2003). Econometric analysis (5th ed.). Pearson Education, Inc.
- Hailu, B. K., Abrha, B. K., & Weldegiorgis, K. A. (2014). Adoption and impact of agricultural technologies on farm income: Evidence from Southern Tigray, Northern Ethiopia. International Journal of Food and Agricultural Economics, 2(4), 91–106. https://doi.org/10.22004/ag.econ.190816
- Heckman, J. (1997). Instrumental variables: A study of implicit behavioral assumptions used in making program evaluations. The Journal of Human Resources, 32(3), 441–462. https://doi.org/10.2307/146178
- Ismaila, S., & Tanko, M. (2021). Exploring relative deprivation theory in the rice industry: Planting for food and jobs (PFJ) in northern Ghana. Technology in Society, 65, 101556. https://doi.org/10.1016/j.techsoc.2021.101556
- Jamilu, A. A., Atala, T. K., Akpoko, J. G., & Sanni, S. A. (2015). Factors influencing smallholder farmers participation in IFAD-community based agricultural and rural development project in Katsina State. Journal of Agricultural Extension, 19(2), 93–105. https://doi.org/10.4314/jae.v19i2.8
- Janvry, A. D., Dustan, A., & Sadoulet, E. (2011). Recent advances in impact analysis methods for ex-post impact assessments of agricultural technology: Options for the CGIAR (p. 40). ISPC. https://hdl.handle.net/10568/128915
- Jayne, T. S., Mason, N. M., Burke, W. J., & Ariga, J. (2018). Taking stock of Africa’s second-generation agricultural input subsidy programs. Food Policy, 75, 1–14. https://doi.org/10.1016/j.foodpol.2018.01.003
- Kangile, J. R., Kadigi, R. M., Mgeni, C. P., Munishi, B. P., Kashaigili, J., & Munishi, P. K. (2021). Dynamics of coffee certifications in producer countries: Re-examining the Tanzanian status, challenges and impacts on livelihoods and environmental conservation. Agriculture, 11(10), 931. https://doi.org/10.3390/agriculture11100931
- Lambongang, M., Ansah, I. G. K., & Donkoh, S. A. (2019). Participation and yield effect of Ghana’s planting for food and jobs programme in Bunkpurugu-Yunyoo district. UDS Online Database, 2(1), 86–98. http://hdl.handle.net/123456789/2752
- Lee, W. S. (2013). Propensity score matching and variations on the balancing test. Empirical Economics, 44(1), 47–80. https://doi.org/10.1007/s00181-011-0481-0
- Martey, E., Dogbe, W., Etwire, P. M., & Wiredu, A. N. (2015). Impact of farmer mentorship project on farm efficiency and income in rural Ghana. Journal of Agricultural Science, 7(10), 79. https://doi.org/10.5539/jas.v7n10p79
- Martey, E., Etwire, P. M., & Mockshell, J. (2021). Climate-smart cowpea adoption and welfare effects of comprehensive agricultural training programs. Technology in Society, 64, 101468. https://doi.org/10.1016/j.techsoc.2020.101468
- Martey, E., Kuwornu, J. K., & Adjebeng-Danquah, J. (2019). Estimating the effect of mineral fertilizer use on Land productivity and income: Evidence from Ghana. Land Use Policy, 85, 463–475. https://doi.org/10.1016/j.landusepol.2019.04.027
- Maulu, S., Hasimuna, O. J., Mutale, B., Mphande, J., & Siankwilimba, E. (2021). Enhancing the role of rural agricultural extension programs in poverty alleviation: A review. Cogent Food & Agriculture, 7(1), 1886663. https://doi.org/10.1080/23311932.2021.1886663
- Mensah, N. O., Bazimwomit, T. K., Kambilige, S. P., Amrago, E. C., Asare, J. K., Donkor, A., & Osei Tutu, F. (2022). Impact of formal credit on tomato trader’s welfare in the upper east region of Ghana: A propensity score matching estimation. World Journal of Entrepreneurship Management and Sustainable Development, 18(3), 345–361. https://doi.org/10.47556/J.WJEMSD.18.3.2022.3
- Ministry of Gender, Children and Social Protection (MoGCSP) (2014). Child Protection Baseline Research Report, Northern Regional Profile.
- Mokgomo, M. N., Chagwiza, C., & Tshilowa, P. F. (2022). The impact of government agricultural development support on agricultural income, production and food security of beneficiary small-scale farmers in South Africa. Agriculture, 12(11), 1760. https://doi.org/10.3390/agriculture12111760
- Mthombeni, D. L., Antwi, M. A., & Oduniyi, O. S. (2022). Factors influencing access to agro-processing training for small-scale crop farmers in Gauteng province of South Africa. Agriculture & Food Security, 11(1), 1–7. https://doi.org/10.1186/s40066-022-00370-9
- Nakano, Y., Tsusaka, T. W., Aida, T., & Pede, V. O. (2018). Is farmer-to-farmer extension effective? The impact of training on technology adoption and rice farming productivity in Tanzania. World Development, 105, 336–351. https://doi.org/10.1016/j.worlddev.2017.12.013
- Nwaobiala, C. U. (2014). Socio-economic factors influencing farmers’ participation in community-based programme in Abia and cross river states of Nigeria. Journal of Agricultural Extension, 18(1), 48–61. https://doi.org/10.4314/jae.v18i1.5
- Nxumalo, K. K. S., & Oladele, O. I. (2013). Factors affecting farmers’ participation in agricultural programme in Zululand district, Kwazulu Natal Province, South Africa. Journal of Social Sciences, 34(1), 83–88. https://doi.org/10.1080/09718923.2013.11893120
- Okoli, V. B. N., Okereke, C. N., Onubuogu, G. C., & Esiobu, N. S. (2014). Analysis of participating and non-participating commercial agriculture development project (CADP) farmers in pineapple production in Awgu LGA, Enugu State, Nigeria. Global Advanced Research Journal of Agricultural Science, 3(8), 259–270.
- Oladejo, J. A., Olawuyi, S. O., & Anjorin, T. D. (2011). Analysis of women participation in agricultural production in Egbedore local government area of Osun State, Nigeria. International Journal of Agricultural Economics and Rural Development, 4(1), 1–11.
- Osabuohien, E. S. (2014). Large-scale agricultural land investments and local institutions in Africa: The Nigerian case. Land Use Policy, 39, 155–165. https://doi.org/10.1016/j.landusepol.2014.02.019
- Pauw, K. (2022). A review of Ghana’s planting for food and jobs program: Implementation, impacts, benefits, and costs. Food Security, 14(5), 1321–1335. https://doi.org/10.1007/s12571-022-01287-8
- Prah, S., Asante, B. O., Aidoo, R., Mensah, J. O., & Nimoh, F. (2023). Impact of agricultural policy intervention on yield and profitability of maize farmers: The case of Planting for Food and Jobs (PFJ) programme in Ghana. Cogent Food & Agriculture, 9(1), 2249928. https://doi.org/10.1080/23311932.2023.2249928
- Pufahl, A., & Weiss, C. R. (2009). Evaluating the effects of farm programmes: Results from propensity score matching. European Review of Agricultural Economics, 36(1), 79–101. https://doi.org/10.1093/erae/jbp001
- Rosenbaum, P. R., & Rubin, D. B. (1983). The central role of the propensity score in observational studies for causal effects. Biometrika, 70(1), 41–55. https://doi.org/10.1093/biomet/70.1.41
- Samanta, D. (2023). Estimating impact of technological adoption in farming in Bihar: A propensity score matching approach. International Journal of Social Economics, 50(7), 1007–1016. https://doi.org/10.1108/IJSE-09-2022-0606
- SRID (2016). Agriculture in Ghana. Facts and figures (2016) Ministry of Food and Agriculture–Statistics, Research and Information Directorate (SRID) October 2017.
- Tanko, M., Iddrisu, A., & Alidu, A. (2016). Determinants of rice yield in Northern region of Ghana, the role of policy. Asian Journal of Agricultural Extension, Economics & Sociology, 9(2), 1–11. https://doi.org/10.9734/AJAEES/2016/22922
- Tanko, M., Ismaila, S., & Sadiq, S. A. (2019). Planting for food and jobs (PFJ): A panacea for productivity and welfare of rice farmers in Northern Ghana. Cogent Economics & Finance, 7(1), 1693121. https://doi.org/10.1080/23322039.2019.1693121
- Tekuni, N., Kyere, G. H., Anang, S. A., & Mensah, N. O. (2021). Robustness of food security and policy trust on smallholder participation in Ghanas planting for food and jobs programme. African Journal of Agricultural Research, 17(12), 1517–1523.
- Tologbonse, E. B., Jibrin, M. M., Auta, S. J., & Damisa, M. A. (2013). Factors influencing women participation in women in agriculture (WIA) programme of Kaduna state agricultural development project, Nigeria. International Journal of Agricultural Economics and Extension, 1(7), 47–54.
- USAID. (2022). The market for maize, rice, soy and warehousing in northern Ghana.
- Ward, P. S., Bell, A. R., Parkhurst, G. M., Droppelmann, K., & Mapemba, L. (2016). Heterogeneous preferences and the effects of incentives in promoting conservation agriculture in Malawi. Agriculture, Ecosystems & Environment, 222, 67–79. https://doi.org/10.1016/j.agee.2016.02.005
- Wiredu, A. N., Asante, B. O., Annin, K., Dogbe, W., Attoh, C., & Al-Hassan, R. M. (2013). Factors influencing participation in rice development projects the case of smallholder rice farmers in northern Ghana. International Journal of Development and Economic Sustainability, 1(2), 13–27.
- Wonde, K. M., Tsehay, A. S., & Lemma, S. E. (2022). Training at farmers training centers and its impact on crop productivity and households’ income in Ethiopia: A propensity score matching (PSM) analysis. Heliyon, 8(7), e09837. https://doi.org/10.1016/j.heliyon.2022.e09837
- Woo, H. S., Berns, J. P., & Solanelles, P. (2022). How rare is rare? How common is common? Empirical issues associated with binary dependent variables with rare or common event rates. Organizational Research Methods, 26(4), 655–677. https://doi.org/10.1177/10944281221083197
- Yamane, T. (1967). Statistics: An introductory analysis (No. HA29 Y2 1967).
- Zoogah, A. T., & Nakuja, T. (2020). Modernizing extension services to improve rice productivity: Lessons from Ghanas planting for food and jobs program. Journal of Agricultural Extension and Rural Development, 14(4), 110–115.