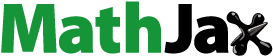
Abstract
The COVID-19 pandemic that broke out in late 2019 ushered in a rare era of global uncertainty that tested the resilience of financial and economic systems. Countries tried to implement stringent public health regulations to stop the virus’s rapid spread, frequently at the expense of economic activity. We contribute to the existing body of knowledge by providing a detailed analysis of the distinct effects of government initiatives during the COVID-19 pandemic on stock market returns. We examine monthly data from February 2020 to December 2022 using the Autoregressive Distributive Lag model, focusing on both short- and long-run patterns and trends. The findings show that the COVID-19 stringency index has a short-term positive effect on stock market returns and a negative effect in the long-run. Furthermore, the findings show negative correlations between stock market returns and global economic policy uncertainty in the long run and a negative effect in the short-run. The study recommends that investors consider spreading their investments to take advantage of short-term gains during pandemic-related restrictions; listed firms should adapt their business strategies to navigate uncertainties during challenging times and finally, policymakers should enhance communication and transparency in policy decisions to build and maintain investor confidence.
Introduction
Studies on the effect of coronavirus disease (COVID-19) have recently received greater attention. The novel coronavirus was identified in Wuhan, China and was declared an epidemic in January 2020 and a pandemic in March 2020 (Director General, World Health Organization [WHO], 2020). Within 3 months of its declaration as an outbreak, the viral infection was found in nearly every country in the world, destroying the plans of governments, individuals and business organizations (Alexandridi et al., Citation2022). Over three years after the outbreak, the virus infected 695,340,677 lives, killed 6,915,759 people and had 667,401,327 recoveries (Worldometer, Citation2023). Evidence from a variety of countries shows that the COVID-19 pandemic has had significant impacts on human health, economies and global security (Amoah et al., Citation2023; Kubatko et al., Citation2023; Lee et al., Citation2023; Ozili & Arun, Citation2023; Panchal et al., Citation2023; Qiu et al., Citation2017; Sridhar et al., Citation2023; Ullah, Citation2023; Weaver, Citation2023; Xu et al., Citation2023).
The severe consequences of the pandemic on international trade were highlighted by the World Trade Organization’s (WTO) prediction of a substantial decline of 13-32 percent in global commerce in 2020 (World Trade Organization, Citation2020). According to Aharon et al. (Citation2022), American depository receipts’ liquidity and stability were said to have been harmed by closures, restrictions and containment measures. Yang and Deng (Citation2021) also argue that stock market returns were also negatively affected by testing, contact tracing regulations and other forms of social isolationism, as found. There was widespread disruption across industries like tourism and banking as a result of government actions taken in response to the pandemic. While Aharon et al. (Citation2021) highlighted the negative impact of government initiatives on the hospitality industry in the United States, Chow and Tan (Citation2022) highlighted the significant impact of COVID-19 government interventions on multiple sectors in Malaysia. The effects of COVID-19 on global stock markets and corporate performance have also been studied extensively in the extant literature. This field of study has been the subject of a plethora of studies that have delved into various facets (Albulescu, Citation2021; Mohammed, Citation2023; Rababah et al., Citation2020; Sansa, Citation2020; Shen et al., Citation2020). In addition, there has been recent interest in how COVID-19-related government interventions affected stock market performance in countries outside of Africa (see Abdullah et al., Citation2022; Bakry et al., Citation2022; Guven et al., Citation2022; Kassamany & Zgheib, Citation2023; Li et al., Citation2023; Owjimehr & Samadi, Citation2022; Sinaga et al., Citation2022; Yang et al., Citation2023; Yh Saif-Alyousfi, Citation2022; Yu & Xiao, Citation2023).
As with the stock market, international trade opportunities have suffered from the restrictions imposed by governments during COVID-19. Exchange rates have been impacted as countries have reduced their international trade in response to restrictions. There has been some research into the connection between government intervention and exchange rates, but only a small number of studies have so far, done so (Feng et al., Citation2021; Hoshikawa & Yoshimi, Citation2021; Sunaryati & Munandar, Citation2023; Syed et al., Citation2022; Wang et al., Citation2021). The current state of knowledge is illuminated by the literature review on the effects of COVID-19 on stock markets and organizational performance in Africa. Contributing to the literature, Adenomon et al. (Citation2022) analyze how the release of COVID-19 affected the Nigerian stock market. Their research, which takes into account market volatility, investor behaviour and regulatory measures, provides a comprehensive evaluation of the stock market’s response to the pandemic. African stock markets are also studied by Takyi and Bentum-Ennin (Citation2021) and Insaidoo et al. (Citation2021), who look into the impact of COVID-19. Despite the lack of details, it is reasonable to assume that they will shed light on how the pandemic affected stock markets in various African countries, perhaps delving into variations in performance and resilience across the continent. Understanding the impact of government interventions related to COVID-19 on stock market performance is highlighted by Li et al. (Citation2023), Raifu (Citation2022) and Yh Saif-Alyousfi (Citation2022). Possible topics covered in such research include the impact of market closures, government spending programs and new regulations on Africa’s stock exchanges. Considering the pandemic, their findings can help us better comprehend the connection between government policies and market dynamics.
The global economic impact of the COVID-19 pandemic has been significant, and Ghana is no exception. Dawda and File (Citation2023) point out that when the COVID-19 pandemic broke out in Ghana, the tourism industry saw a significant drop in revenue. Tourist arrivals dropped significantly because of the travel bans, lockdowns and widespread panic caused by the virus. The tourism industry felt the effects of this recession, from hotels and restaurants to travel agencies and even local artisans. According to the available literature, the recovery of Ghana’s tourism industry hinges heavily on the virus’s containment, the success of vaccination campaigns and the regaining of travellers’ confidence. The effect of COVID-19 on the Ghanaian stock market is another topic that has received a lot of attention from academics. According to Dadzoe et al. (Citation2021), the Ghanaian stock market experienced significant volatility and uncertainty because of the pandemic. Investors suffered significant losses as stock prices fell sharply. The literature emphasizes that both global factors and local government interventions affected the stock market performance in Ghana during the pandemic.
Ghana documented its initial two cases of COVID-19 in early March 2020, prompting an immediate governmental reaction. Elaborate safety protocols, lockdowns and travel restrictions were among the stringent measures that were enforced to contain the virus’s spread. There were periodic spikes in the number of confirmed cases, which required the implementation of adaptive strategies. As of March 20, 2020, the capital city accounted for all sixteen confirmed cases of COVID-19 in Ghana (Ministry of Health, 2020). In response to the COVID-19 outbreak, the Ghanaian government proclaimed a two-week closure for the Greater Accra Metropolitan Area (where the capital city is located) and the Greater Kumasi Metropolitan Area (one of the major economic hubs in Ghana) on Saturday, March 28. The public was instructed to remain indoors, venturing outside solely for essential purposes such as banking, utilizing public restrooms, obtaining food, medication, and water (Ansah, Citation2020). Except for essential products and services, intercity travels were halted and passenger capacities on intracity vehicles were reduced to ensure social distancing.
Until March 22, only returning Ghanaians and foreign nationals with Ghanaian residence permits were granted access to the Ghanaian borders; those who exhibited symptoms were subjected to a 14-day quarantine period (Nyavi, Citation2020). Ghana has documented 141 COVID-19 cases and five fatalities as of March 28 (Ansah, Citation2020). As of the most recent updates on December 30, 2023, Ghana has documented a cumulative count of 171,889 cases of COVID-19, resulting in 1,462 fatalities and the recovery of 170,425 individuals (Worldometer, Citation2023).
The Ghana Stock Market (GSE) is an emerging market that was founded in July 1989 as a privately held corporation under the Companies Code of 1963 (Act 179) and began trading on November 12, 1990. There are now 42 stocks listed, representing 37 different firms, as well as 2 corporate bonds issued by Abonongo in 2016. The GSE ranked sixth among emerging stock markets in terms of index performance in 1993, seeing a capital appreciation of 116% (Tetteh et al., Citation2022). In 1994, the GSE outperformed all others, experiencing a remarkable increase of 124.3% in its index level. The index experienced a growth rate of 6.3% in 1995, which can be attributed in part to elevated levels of inflation and interest rates (Mlambo & Biekpe, Citation2001; Ulzen, Citation2017). The index experienced a growth rate of 42% in 1997 and its value reached 868.35 by the conclusion of 1998 (Opoku-Agyemang et al., Citation2016).
In October 2006, the Ghana Stock Exchange had a market capitalization of around 111,500 billion cedis [$11.5 billion] (Raji, Citation2012). The GSE’s market value on December 31, 2007, amounted to 131,633.22 billion cedis representing a 31.84% increase in value in 2007 (Ulzen, Citation2017). In August 2014, the GSE had a total of 38 listings and 2 corporate bonds, specifically Osae, Citation2021. The list of prominent corporations includes AngloGold Ashanti, Accra Brewery Company, Ecobank Ghana, Guinness Ghana Breweries and Unilever Ghana. The Ghana Stock Exchange (GSE) has played a crucial role as a venue for enterprises to secure funding, thereby making a significant contribution to the economic development of Ghana (Akomea-Bonsu & Sampong, Citation2013).
The Ghana Stock Exchange Composite Index is a weighted index that monitors the performance of all stocks listed on the Ghana Stock Exchange based on their market capitalization (Agyarko et al., Citation2023). The Ghanaian stock market index offers a thorough assessment of the overall condition of the stock market in Ghana. It reflects the total value of all stocks listed on the exchange, taking into account the number of shares in circulation. The index is computed based on the aggregate market value of all publicly traded equities and is continuously revised to account for fluctuations in the underlying stock prices (Lin, Citation2018). The fluctuations in stock prices and sector performances underscore the ever-changing nature of the stock market, which is shaped by a multitude of factors including economic conditions, industry trends and company-specific developments. Investors and market experts diligently observe these movements to make well-informed decisions in the ever-changing terrain of the Ghanaian stock market. The study explores the Ghanaian stock market as a result of the consistent announcement of the government of Ghana for people to stay at home, businesses to shut down and the frequent contact tracing by the Ministry of Health in anticipating that these frequent announcements would feed into the prices of stocks listed on the Ghanaian stock exchange, thereby affecting the stock market performance as proposed by the Efficiency Market Hypothesis. Moreover, the choice for the Ghanaian stock market is the fact that the exchange has received very limited attention in the existing body of literature concerning the interconnectedness of government policy initiatives and the market’s performance. This creates a loophole in knowledge on the side of investors who seek to know the dynamics of market performance in times of such uncertainties.
Several studies have been undertaken to understand the relationship between the pandemic and the performance of the stock market (Agyei et al., Citation2022; Deri et al., Citation2023; Ofori-Boateng et al., Citation2022; Tetteh et al., Citation2022). Recent research has focused on how COVID-19-related government interventions in Ghana have affected the country’s economic fortunes. Global Economic Policy Uncertainty (GEPU) and stock market performance are studied by Asafo-Adjei et al. (Citation2020) and Asafo-Adjei et al. (Citation2021). Both studies concluded that the stock market suffered because of the pandemic’s negative effects on investor confidence and volatility due to the lack of clarity surrounding global economic policies and interventions. The COVID-19 pandemic has had a major effect on the economy of Ghana. There was a noticeable drop in returns on assets and net interest margins in Ghana’s banking sector, according to the study by Musah et al. (Citation2022). As a result of the pandemic’s effect on household income, food insecurity worsened in urban areas (Bukari et al., Citation2022; Onyango et al., Citation2023). Poverty increased and fewer people were able to get the vaccines they needed (Kissi et al., Citation2022). Due to financial setbacks, hotel workers saw their pay decrease (Hervie et al., Citation2022). Sleep quality, food choices and finances were all negatively impacted for college students (Amoako et al., Citation2022). These findings stress the importance of preventative measures, targeted policies and recovery initiatives in Ghana to combat the threats posed by the pandemic and strengthen the country’s economy.
This study contributes to the existing literature in two main ways by exploring the impact of restrictions imposed by the government of Ghana, in attempting to curb the spread of the COVID-19 pandemic, on the performance of the Ghanaian stock market despite the numerous existing literature on the effect of the pandemic on economic growth and the behaviour of macroeconomic variables in Ghana. Second, this study adds to the existing body of knowledge by exploring the dynamic effect of the interventions with a specific focus on understanding the long-run and short-run effects of the pandemic on stock market performance in the Ghanaian context. Again, this study is the first to establish these dynamics in the Ghanaian context.
The subsequent sections of this paper are structured as follows; section two provides a review of existing theoretical and empirical literature in the area of study and section three presents a description of the data, sample and research methodology. In section four, we present the empirical results while section five finally concludes the paper.
Literature review
The study seeks to investigate the impact of COVID-19-related government interventions on the performance of Ghana’s stock market. In this section, we present a review of relevant theories underpinning the relationship between the study’s variables followed by a review of the empirical literature relating to the main aims of the study. The theoretical underpinnings of the study hover around the Efficient Market Hypothesis (EMH) and the Adaptive Market Hypothesis (AMH).
Theoretical review
The paper is underpinned by the efficient market theory which states that the observed price of financial securities reflects all available information in that market. On the other hand, behavioural finance shares the idea that investors’ sentiments affect their decisions and can therefore affect stock prices in financial markets (Fama, Citation1965). Most studies suggest that extreme events and disasters such as the 2001 terrorist attack on the US and the 2002 severe acute respiratory syndrome (SARS) in China have had negative impacts on investors’ portfolios and thereby investment decisions. This clearly shows that some information leads to the observation of anomalies in the financial markets, thus calling into question the market efficiency. The study is grounded on both the Efficient Market Hypothesis (EMH) and the Adaptive Market Hypothesis (AMH). The Efficient Market Hypothesis (EMH) and the Adaptive Market Hypothesis (AMH) significantly explain the study’s objectives and offer emphasis on crucial aspects of market uncertainty, financial contagion and financial stability.
First, the EMH emphasizes the premise that stock prices represent all available information (Fama, Citation1970), which makes it imperative to evaluate how government constraints affect the efficiency of the Ghanaian stock market. These limits can induce market uncertainty by questioning the EMH’s primary premise of information efficiency, potentially leading to erratic price movements and investor concerns. In addition, departures from the EMH’s assumptions may contribute to financial contagion as interconnection and linkages between assets become more obvious during periods of inefficiency. The EMH’s applicability to the study also extends beyond financial stability issues, since market disruptions produced by government actions can erode investor trust and impair market stability as a whole.
The efficient market hypothesis (EMH) establishes a connection between two primary factors: the flow of information and the values of assets (Ehiedu & Obi, Citation2022). Within the realm of government restrictions, such as lockdowns and regulatory interventions, the Efficient Market Hypothesis (EMH) posits that the stock market swiftly integrates this information into stock prices. Investors promptly respond to the new information by engaging in purchasing or selling activities, and making decisions based on their appraisals of the impact of government restrictions. As a result, the market undergoes modifications to accurately represent these assessments. In addition, the Efficient Market Hypothesis (EMH) proposes the idea of a ‘random walk’ in stock prices which suggests that future price changes are fundamentally unpredictable because all pertinent information is already reflected in current prices (Blackledge & Lamphiere, Citation2021). Hence, if government limitations are already widely acknowledged and expected, their possible influence on stock prices may have already been taken into account which makes it difficult to forecast future returns within the context of the Efficient Market Hypothesis (EMH). This study utilizes the principles of the Efficient Market Hypothesis (EMH) to evaluate the impact of government-imposed restrictions as information flow on the returns of Ghana’s stock market.
Second, the study’s adoption of the Adaptive Market Hypothesis (AMH) acknowledges that markets are not perpetually efficient and that players adjust to changing circumstances (Lo, Citation2004). This correspondence with the AMH highlights the purpose of the study, which is to investigate how government regulations influence the adaptive behaviour of market players and how this adaptation, in turn, affects the performance of the Ghanaian stock market. AMH acknowledges that periods of market uncertainty triggered by government interventions may elicit diverse adaptive responses, further emphasizing the importance of the study in determining how these adaptive strategies influence market dynamics, financial contagion dynamics and ultimately financial stability. The Adaptive Market Hypothesis (AMH) offers an intriguing framework for analyzing the impact of government restrictions on stock market returns. The Adaptive Market Hypothesis (AMH) allows us to analyze how investors adjust their tactics by changing information. This enables us to examine how market participants might adjust to uncertainty arising from government interventions. This theory analyzes cognitive biases and inefficiencies in the market (Manahov & Hudson, Citation2014), which helps us understand potential deviations from ideal market predictions during periods of restrictive measures. It is crucial to acknowledge the varying degrees of risk aversion to comprehend how alterations in the perception of risk, influenced by government actions, can influence the integration of information into stock prices. Our study focuses on the AMH’s analysis of the evolution of investment strategies, specifically examining how the flow of information impacts the adaptive behaviour of market participants in the presence of government constraints. Consequently, this impacts the volatility of stock prices.
Empirical review
Several studies have assessed the effect of government intervention on stock returns. Most of these studies have presented diverse outcomes. For instance, Zoungrana, Toé and Toé, (Citation2023) employed Wilcoxon’s signed ranks test to assess the impact of government interventions (social distancing, public health measures, movement restrictions, lockdown and governance) measured as a dummy variable on the West African Economic and Monetary Union’s (WAEMU) stock market. The study used the GARCH model for their analysis and the results of their study concluded that while social distancing and governance have a positive effect on the stock returns, lockdown, public health measures and movement restrictions negatively affected the returns of the WAEMU stock market. Focusing on 20 OECD countries for a period of 8 months from February 2020 to October 2020, using a panel regression model, Yang and Deng (Citation2021) identified that government restrictions, measured by the stringency index, government response index, containment & health index and the economic support index enlarge the adverse effect of the pandemic on returns of stocks.
Moreover, attempting to investigate the effect of COVID-19-related government restrictions on global stock market liquidity, Zaremba et al. (Citation2020) retrieved daily data from 49 countries from January to April 2020 and realized that the closure of workplaces and schools negatively affected the liquidity and returns of emerging stock markets. Additionally, Aharon et al. (Citation2022) used a large data of 387 collected from 34 American Depository Receipts (ADRs) to check the effect of government intervention on the volatility and liquidity of cross-listed securities. They find that restrictions, closures and health containment measures negatively affected the liquidity and stability of the ADRs. Similar to the study by Yang and Deng (Citation2021), Owjimehr and Samadi (Citation2022) investigated the effect of the government response index, containment and health index, stringency index and economic support index on the returns of the Iranian stock market. They analyzed data spanning from 22 Feb 2020 to 12 June 2021 using a threshold regression. They postulated that the government response index, stringency index, containment and health index negatively affected the stock returns while the economic support index had a positive impact on the returns of the selected stocks.
Many studies have been conducted to assess how restrictions during the pandemic affected African stock markets. Yh Saif-Alyousfi (Citation2022) seeks to investigate how the stringency of government policy impacted stock returns on both global and regional levels using Pooled-ordinary least squares and panel data techniques with data spanning from January 2020 to 10 May 2021. The results of the study showed that policy interventions positively affected the returns of stocks both on the global and regional levels. Moreover, using dummy variables and vaccine index as measures of government response to COVID-19, Badmus and Ojelade (Citation2022) concluded that the dummy variable and the vaccine index positively affect the stock returns of countries like Cote D’Ivoire, Zambia and Botswana except for Tunisia. In Nigeria, Bello (Citation2022) used the event study methodology to assess the effect of lockdown announcements on the performance of 38 listed firms on the Nigerian stock exchange and found that the stock market positively reacts to the lockdown announcements.
However, despite all the positive effects identified by several studies, Raifu (Citation2022) realized that the announcement of government policy interventions has a negative impact on the returns of stocks in countries like Egypt, Morocco, Nigeria, Namibia, Cote D’Ivoire, Kenya and Uganda using both time series Ordinary Least Squares and panel estimation techniques for the analysis of data retrieved from 11 countries in Africa. Also, Lawal et al. (Citation2020) results contradicted Bello (Citation2022) after using the ARDL model to analyze data from 2019 to 2020. The results of their study reveal that government restrictions during the pandemic negatively affected the returns of stocks listed in Nigeria. Moreover, Aggarwal et al. (Citation2021) note that the stringency of government restrictions negatively affects stock returns using 12 sampled countries in Africa.
Uncertainty in economic policy is the danger to the economy brought on by unclear future laws and regulations. Due to market uncertainty, this phenomenon further raises the possibility that businesses and individuals would postpone their purchases and investments (Al-Thaqeb & Algharabali, Citation2019). Several empirical studies have assessed its impact on the returns of stocks across different financial markets. Mbanyele (Citation2023) for instance took data covering 2002 to 2015 from sampled firms in Brazil and used the two-step GMM dynamic estimation model and ordinary least squares estimation and found that economic policy uncertainty adversely affects stock returns. In the UK, Gao et al. (Citation2019) developed a time-varying parameter factor-augmented vector autoregressive (TVP-FAVAR) model for its variable estimation and the results suggest that both economic policy uncertainty and the uncertainty with economic activity have greater power in elucidating UK stock returns. Based on a panel VAR model Christou et al. (Citation2017) analyzed data spanning from January 1998 to December 2014 and found a significant adverse association between EPU and the stock returns of Australia, Canada, China, Japan, Korea and the US.
Moreover, Wu (Citation2022) analyzed the effect of EPU on the returns of A-share firms listed in China from 2010 to 2019. The results of the study show that an increase in economic policy uncertainty reduces stock prices. Also, with monthly data from January 1997 to July 2020, Adam and Yacob (Citation2022) looked at the effect global economic policy uncertainty has on the returns of firms listed on the ASEAN stock exchange focusing on the Kuala Lumpur Composite Index, Vietnam Stock Index, Stock Exchange of Thailand, Philippine Stock Exchange and Jakarta Stock Exchange. They found a strong negative effect of global policy uncertainty on the returns of the various exchanges sampled. While many other studies found a negative impact of economic policy uncertainty on stock returns (Gea et al., Citation2021; Kang et al., Citation2021; Lee & Chen, Citation2021; Wang & Zhang, Citation2021; Yousuf, Citation2021; Zhang et al., Citation2023), Yu and Huang (Citation2021) realized that economic policy uncertainty significantly helps to predict the performance of the volatility of the stock index. Existing knowledge on the effect of government regulations on the performance of the stock market is complex and contradictory. Numerous studies have assessed the consequences of government initiatives during events such as the COVID-19 pandemic using a wide range of approaches and indicators, examining this link across numerous countries and regions. While some studies have reported positive effects of government interventions, such as increased stock market returns in response to certain policies such as social distancing and governance measures (Bello, Citation2022; Yh Saif-Alyousfi, Citation2022; Yu & Huang, Citation2021; Zoungrana, Toé & Toé, Citation2023), others have found negative effects, especially in the context of lockdowns, public health measures and movement restrictions (Aggarwal et al. Citation2021; Aharon et al., Citation2022; Lawal et al., Citation2020; Owjimehr & Samadi, Citation2022; Raifu, Citation2022; Yang & Deng, Citation2021; Zaremba et al., Citation2020). These disparities imply that the relationship between government limitations and stock market performance is complex and context-dependent.
The existing body of literature has extensively explored the effects of government interventions during the COVID-19 pandemic on stock market returns across various global contexts. This is evident in the research conducted by Zoungrana et al. (Citation2023), Yang and Deng (Citation2021), Zaremba et al. (Citation2020), Yh Saif-Alyousfi (Citation2022), Badmus and Ojelade (Citation2022), Bello (Citation2022), Raifu (Citation2022) and Lawal et al. (Citation2020). Zoungrana et al. (Citation2023) and Yang and Deng (Citation2021) studied the rapid responses to particular events and government actions. On the other hand, Zaremba et al. (Citation2020) and Yh Saif-Alyousfi (Citation2022) analyzed the wider effects on the overall liquidity of the global stock market and the returns of regional stocks, respectively. Badmus and Ojelade (Citation2022), Bello (Citation2022) and Raifu (Citation2022) studied the distinct aspects of COVID-19 immunization, lockdown announcements and government policy actions. They provided helpful knowledge about how these factors impact the performance of stock markets in different African countries.
Nevertheless, despite the vast amount of research available, there is still a significant lack of knowledge regarding a thorough comprehension of the immediate and prolonged effects of government interventions on stock markets, specifically in the unique setting of Ghana. Lawal et al. (Citation2020) provided great knowledge by utilizing dummy variables to represent government intervention in Nigeria. However, it is important to further investigate the potential oversight of obscure dynamics. Hence, this study seeks to fill this crucial void by thoroughly investigating the temporal aspects of government interventions, differentiating between short-term fleeting impacts and long-term associations. We employ a widely used measure by the Oxford Coronavirus Government Response Tracker (OxCGRT) as a composite measure of nine of the response metrics in the Ghanaian context. This helps us contribute significantly to the existing literature by providing a detailed understanding of the dynamic relationship between government actions during the pandemic and the subsequent behaviour of the stock market.
Regarding Ghana specifically, despite the importance of understanding the influence of government limitations on stock market performance on a worldwide scale, there is a paucity of studies on Ghana’s stock market, especially when considering both long-run and short-run effects. Given the particular economic and political circumstances of Ghana, it is vital to analyze how government interventions, such as COVID-19-related legislation, impact the country’s stock market. In addition, investors, policymakers and market participants who wish to make well-informed decisions must comprehend the short- and long-term dynamics of this relationship. By performing a Ghana-specific study, we contribute vital knowledge to the broader literature on government limits and stock market performance, filling a significant knowledge vacuum and providing pertinent advice to Ghana’s financial market participants.
Data and method
Data and variables
The study used Ghana Stock Exchange Composite Index (GSECI) data converted into log returns as the dependent variable whereas crude oil prices, global economic policy uncertainty index and government intervention measured by the COVID-19 stringency index and health containment index were the independent variables. The stringency index and the health containment index were averaged to find the monthly indexes to match with the data for other variables. For a sample period from February 2020 to December 2022, the study collects the GSEI data from the Ghana Stock Exchange (GSE), petrol prices from the Bank of Ghana (BoG), Global Economic Policy Uncertainty Index (GEPU) from the economic policy uncertainty website. While data for all other variables were available for a significant number of years, we could not retrieve data for the COVID-19 stringency index and health containment index which were part of our main variables of interest. This is because data was not available for these variables for the periods before and after our study’s sampling timeline. Hence, we selected the timeframe from February 2020 to December 2022 for the study. summarizes the variables with their measurement and the corresponding abbreviations used.
Table 1. Measurement of variables.
The study uses the Oxford COVID-19 Government Response Tracker (OxCGRT) database (Hale et al., Citation2020) to measure governments’ responses. The stringency index considers information on social distancing measures represented by 8 indicators including school closure, workplace closure, cancelling of public events, restrictions on gathering size, closure of public transport, stay-at-home requirements, restrictions on internal movement and restrictions on international travel. The Health Containment Index is comprised of three indicators: public awareness campaigns, testing policies and contact tracing. This index represents government emergency healthcare measures, such as the COVID-19 testing procedure. Each of the three indexes is a simple cumulative score of the core indicators that have been rescaled to range between 0 and 100.
Empirical methods
The study employed the ARDL model for its estimations. The choice for the ARDL model was influenced by its ability to work with a small sample size (Narayan, Citation2004; Narayan & Narayan, Citation2005) and its allowance for variables that are I(0) and I(1) (Qamruzzaman & Jianguo, Citation2018). The model also allows the study to estimate long-run and short-run relationships between the variables (Qamruzzaman & Jianguo, Citation2018). These properties of the ARDL model enable the study to analyse both the long-run and short-run connection between the GSE returns and crude oil prices, global economic policy uncertainty and government interventions. The study estimates two different equations to avoid multicollinearity among the health containment and stringency indexes. The first equation models GSE returns as the dependent variable and stringency index, global economic policy uncertainty and crude oil prices as the independent variables. The second equation models GSE returns as the dependent variable and health containment index, global economic policy uncertainty and crude oil prices as the independent variables. Pesaran and Pesaran (Citation1997) give the general form of ARDL with lags of both the dependent and independent variables:
(1)
(1)
where
represents the dependent variable at time t,
and
represent the lagged values for the dependent variable up to the selected optimal lag length,
represents the constant term,
and
represent the coefficient estimates for the lagged values of the dependent variable,
,
,
and
represent matrixes of the independent variables at their respective levels and lagged values for the selected optimal lag length,
,
and
are the coefficient estimates for the respective independent variables and
is the error term.
The conventional error correction and the unrestricted ARDL models of the study respectively for both contingency index and health containment index estimates are as follows:
(2)
(2)
where
represents a change in the dependent variable,
represents a change in the lagged values of the dependent variable,
and
are matrixes of the change in the independent variables and their respective lagged values,
,
and
are the respective lag lengths for the dependent variable and the regressors,
represents the error correction term or the cointegrating term which is the lagged OLS residual,
represents the stochastic error term,
,
,
represent the short-run dynamic coefficients,
represents the coefficient of the error correction term which measures the speed of adjustment of
to long-run equilibrium after changes in the regressors.we estimate the following error correction model (ECM)
(3)
(3)
(4)
(4)
where
represents the monthly returns of the Ghana Stock Exchange Index,
represents the monthly COVID-19 Stringency Index,
represents the monthly Global Economic Policy Uncertainty Index,
represents monthly crude oil prices,
represents the monthly COVID-19 Health Containment Index,
represents the error correction term,
,
,
and
are the short-run dynamic coefficient estimates and
is the coefficient of the error correction term.
Results and discussion
Pre-estimation tests
shows the research variables’ descriptive statistics. From the table, the variable GSEI has a mean value of 1.000488 and a median value of 0.999181 which suggests a relatively symmetrical distribution. The dataset has a minimum value of 0.993503 and a maximum value of 1.013379. The standard deviation is relatively minimal at 0.004371. This indicates that there is limited variability around the mean value. COSI has a narrow spread, as shown by its low standard deviation of 0.190650 and the relationship between its mean and median is consistent (mean = 3.787530, median = 3.765344). The small standard deviations of GEPU.
Table 2. Summary statistics.
CONT suggest that the values close to their means (5.586432 and 3.856692, respectively) are close together. Both variables have slightly positive differences between their mean and median, which points to a slight right-skew. With a mean of 7.310000 and a median of 6.115000, OILP stands out with its high mean and skewness toward higher values. The big standard deviation of 3.413655 shows that there is a lot of variation around the mean. Based on 34 observations, the data seems to be consistent across these variables.
The correlation matrix provided in shows the associations between the variables GSEI, COSI, GEPU, CONT and OILP. GSEI and COSI have a negative correlation of -0.1524, suggesting a modest inverse association between them. This agrees with the findings of Mishra et al. (Citation2022) who evidenced that in the long run, confirmed cases and stringency have a negative and significant relationship with US stock markets. Using a total of 26 emerging stock markets, Gunawan and Nainggolan, (nd) also found that the stringency index has a negative significant relationship with the stock markets’ return. While the findings of Yh Saif-Alyousfi (Citation2022) contradict the positive relationship, the finding coincides with the conclusions of several studies (Li et al., Citation2023; Rowland et al., Citation2023; Yu & Xiao, Citation2023). On the contrary, COSI and GEPU demonstrate a slight positive association of 0.1781. The correlation between GSEI and GEPU is slightly greater and negative, with a value of -0.6635, indicating a considerable inverse association. This is in line with the conclusions of Ullah et al. (Citation2023) as they revealed that both COVID-19 and EPU had a significant negative impact on both Shanghai and Shenzhen stock market returns. Moreover, Maquieira et al. (Citation2023) find that EPU negatively correlates with the stock returns of copper firms. similarly, Xu et al. (Citation2021) confirm that the monthly EPU index can significantly and negatively impact the next month’s stock returns in China.
Table 3. Correlation matrix.
CONT and COSI are moderately positively associated at 0.7726, whereas CONT and GEPU have a moderate negative correlation of -0.2794. CONT and OILP have a mild positive connection of 0.1375, whereas OILP is modestly negatively connected with COSI (-0.4898) and weakly correlated with GEPU (0.0552) and CONT (-0.3222). Apart from the relationship between the contingency index and the health containment index which is too high, the rest of the relationships are very low. To deal with multicollinearity among the contingency and the health containment indexes, the study models them in different equations and estimates their results separately. The adverse relationship between government-imposed limitations during the COVID-19 epidemic on crude oil prices can be ascribed to a convergence of multiple variables. The implementation of stringent lockdowns and travel restrictions resulted in a significant decrease in worldwide economic activities, leading to a pronounced downturn in the oil demand (Khan et al., Citation2020). The overstock problem was worsened by supply chain interruptions, as well as reduced industrial production and travel (Min, Citation2023). As storage facilities became full, it became more difficult to store items, which caused costs to decrease. The sharp decrease in crude oil prices at the beginning of the pandemic in 2020 was caused by a combination of factors including the global economic downturn, disagreements among oil-producing nations regarding production cuts, pressures from the futures market and increased market speculation (Arshizadeh et al., Citation2021; Le et al., Citation2021).
The correlation matrix indicates that the associations between GEPU and GSEI, OILP and COSI and CONT are statistically significant at the 1% significance level. Investors should keep track of the fluctuations in global economic policy uncertainty (GEPU) as it has a negative impact on stock returns. This implies that they should make necessary adjustments to their portfolios accordingly. The stock market performance tends to suffer when oil prices rise due to the increased costs and reduced consumer spending that come with it. This is depicted by the negative correlation between oil prices and stock returns. Moreover, the successful implementation of health containment measures, as reflected by a higher Health Containment Index, has a positive correlation with stock returns. This suggests that investors may stand to gain when governments and health authorities can effectively control the spread of diseases.
Unit root test
presents the Philip Perron test for unit root. The results of the Philip Perron Unit Root Test provide helpful information about the stationarity features of the variables under examination. The remarkably substantial adjusted t-statistics and low p-values demonstrate the effectiveness of the test in evaluating stationarity. The integration order (I(1)) indicates that both the returns of the Ghana Stock Exchange Index (GSEI) and Global Economic Policy Uncertainty (GEPU) variables achieve stationarity after undergoing a single differencing operation. In contrast, the COVID-19 Stringency Index (COSI) and Health Containment Index (CONT) are already in a stationary state in their original versions (I(0)). Moreover, the integration order of Crude Oil Prices (OILP) is I(1), indicating that differencing the series makes it stationary.
Table 4. Unit root test.
ARDL model selection
presents the lag selection results for both the model for the stringency index and the health containment index. The Log-likelihood (LogL) values for the Stringency Index model fall as lag rises, indicating a better model fit to the data. The likelihood ratio (LR) test shows statistical significance for the Lag 1 model, which increases LogL significantly. Using the lowest Akaike Information Criterion (AIC) and Schwarz Criterion (SC), Lag 1 is the best choice for model fit and complexity. Lag 1 has the second-lowest Hannan-Quinn Criterion (HQ), supporting its selection. Similar to the Health Containment Index model, the Lag 1 model increases LogL and passes the LR test, showing its significance. Lag 1 is always recommended by AIC, SC and HQ due to its lower values. The Log-likelihood (LogL) values for the COVID-19 Stringency Index model demonstrate a noticeable enhancement as the lags increase, with Lag 1 being particularly prominent. The Likelihood Ratio (LR) test confirms that Lag 1 is statistically significant and contributes significantly to a substantial improvement in LogL. Furthermore, Lag 1 exhibits the smallest Final Prediction Error (FPE) and achieves the lowest scores on both the Akaike Information Criterion (AIC) and Schwarz Criterion (SC). This confirms its appropriateness. The Hannan-Quinn Criterion (HQ) is marginally elevated for Lag 1 but still maintains viability. In the Health Containment Index model, Lag 1 is also notable as the most significant, as indicated by the LR test and the higher LogL value. The AIC, SC and HQ criteria all prefer Lag 1 since it has lower values which outlines its efficacy in achieving a good trade-off between model fit and complexity. However, we fixed the lag length for the model with the stringency index at (5,0,0,0) and the model with the health containment index at (1,2,2,0) to enable us to understand the short-run mechanisms among the past values of the variables.
Table 5. Lag selection results.
Cointegration tests
presents the results of the cointegration tests. The F-statistics for both models are above the upper bounds at all levels of significance indicating the existence of long-run relationships among the variables. This shows that there is cointegration between the variables. Cointegration tests evaluate the presence of persistent associations between variables (Feng et al., Citation2023). The F-statistics for both models surpass the upper limits at all levels of significance. this suggests the existence of cointegration among the variables. The F-statistic for the COVID-19 Stringency Index is 4.657, whereas for the Health Containment Index, it is 5.053. The critical levels establish thresholds for evaluating the importance of the F-statistics. At a significance level of 1%, the values for both indices continually exceed the critical boundaries, with values significantly higher than the upper bounds. This pattern is also observed at the 5% and 10% significance levels. The results confirm the presence of long-term correlations between the variables in both models. Cointegration implies that the variables exhibit a long-term association, indicating a stable and interconnected relationship (Wang et al., Citation2023). This reveals an essential understanding of the co-movements and connections between the variables.
Table 6. Cointegration tests.
Model estimates
The study investigates the impact of government interventions during the COVID-19 pandemic on stock market performance in Ghana using the ARDL model. The study included other economic variables such as global economic policy uncertainty and crude oil price in the models and looked at the existence of long and short-run relationships between the variables and the GSE returns. The empirical results in present both short-run and long-run ARDL and ECM model estimates.
Table 7. Regression results.
In the short-run, we find that Ghana’s stock exchange returns are negatively explained by their past values at 1%, 5% and 10% significant levels. This result can be explained by the existence of market reversals, overreactions and corrections in the market as well as mean reversion during shocks. Huo and Qiu (Citation2020) studied the way China’s stock market reacted to shocks from COVID-19 in 2020 and realized reversals in the market. They found that out of 22 sectors with negative cumulative abnormal returns during the outbreak, 19 recovered after one month with positive cumulative abnormal returns. This also agrees with the findings of Yong and Laing (Citation2021) in the U.S. as they noted that the short-run effect of the pandemic reverses in the long-run. Moreover, Yan (Citation2020) discovered that returns of stocks reverse every ten trading days in China owing to the lockdown of Wuhan city. Spierdijk et al. (Citation2012) document that, stocks revert very quickly to their fundamental values in times of higher economic uncertainties. Our findings also correspond with the findings of Khanthavit (Citation2020) who seeks to find how and how early the world and national markets reacted and found significant negative reactions to the COVID-19 disease based on returns on the world, French, German, Italian, Spanish, U.K., U.S., Chinese, Philippine and Thai stocks. Moreover, during the outbreak of the COVID-19 pandemic, while Allam (Citation2024) found significant short-term reversals in the Egyptian stock market, Rojo-Suárez and Alonso-Conde (Citation2024) evidenced serious reversals in the trends of the returns in the U.S. equity market, Kurtović and Markarian (Citation2024) showed that listed private equities in North America, Europe and Asia did not recover over the observed period. In Indonesia, Riadi et al. (Citation2023) demonstrated that there was an overreaction in both winner and loser stocks in the LQ 45 group on the IDX in 3 months of observation since COVID-19 was declared as a pandemic. This overreaction is then succeeded by a price reversal even though it has not given a significant return after t + 13 for both winner and loser stocks. In addition, Mohanty and Mishra (Citation2023) found in India that the market overreacted initially but started correcting itself after eight trading days as a result of the outbreak of the pandemic.
Both the stringency index and global economic policy uncertainty are negatively associated with the returns of the Ghana stock exchange and are statistically significant at 5% and 1% significant levels respectively. A percentage increase in the stringency index is associated with a -107. 4 percent fall in the returns of the market in the short run. The findings are confirmed by the conclusions from Zoungrana, Toé and Toé, (Citation2023) who found that movement restrictions negatively affected the returns of the WAEMU stock market. Moreover, the study agreed with the findings from Zaremba et al. (Citation2020) as they retrieved daily data from 49 countries for the period from January to April in the year 2020 and realized that the closure of workplaces and schools negatively affected the liquidity and returns of emerging stock markets. As an affirmation of the results of this study, Owjimehr and Samadi (Citation2022) also found a negative relationship between the stringency index and Iranian stock market performance. The results from Raifu (Citation2022) also agreed with the results of the current study as they found that government policy interventions have a negative impact on the returns of stocks in countries like Egypt, Morocco, Nigeria, Namibia, Cote D’Ivoire, Kenya and Uganda. Moreover, Aggarwal et al. (Citation2021) found a negative relationship between government intervention and stock returns in 12 African countries.
The past and current values of global economic policy uncertainty as well as the lagged values of the GSEI negatively affect the returns of the stock market and are statistically significant at a 1 percent level. This negative association between GEPU and GSEI is confirmed by the findings of several authors who also found a negative effect (Adam & Yacob, Citation2022; Christou et al., Citation2017; Gao et al., Citation2019; Gea et al., Citation2021; Kang et al. Citation2021; Lee & Chen, Citation2021; Mbanyele, Citation2023; Wang & Zhang, Citation2021; Wu, Citation2022; Yousuf, Citation2021; Zhang et al., Citation2023). Our findings contradict the results of Yu and Huang (Citation2021) who noticed a positive impact of global economic policy uncertainty on the stock market. The results, however, disagree with the findings of Yh Saif-Alyousfi (Citation2022) who noticed that policy interventions positively affected the returns of stocks both on the global and regional levels. Also, In Nigeria, Bello (Citation2022) used the event study methodology to assess the effect of lockdown announcements on the performance of 38 listed firms on the Nigerian stock exchange and found a contradicting result that the stock market positively reacts to the lockdown announcements. There is no evidence of an effect of the past and current values of the health containment index on the returns of the stock market which did not agree with several studies (Aharon et al., Citation2022; Owjimehr & Samadi, Citation2022; Yang & Deng, Citation2021; Zoungrana, Toé & Toé, Citation2023). However, we find that in the long-run, only global economic policy is statistically significant at 1 percent level.
The error correction term coefficient in the model with the stringency index is -0.578001. How quickly the system returns to its long-term equilibrium following a disturbance is represented by this coefficient. What this means is that the system will tend to return to equilibrium at a rate of about 57. 8 percent every period if there is a positive deviation from the equilibrium (the variables are not in their long-term relationship). This is statistically significance at the 1% level. The error correction term coefficient in the model including the health containment index is -0.894463 at the 1% statistical significance level. These findings lead us to the solid conclusion that both the COVID-19 stringency index and the health containment index have a negative effect on the stock market returns over the long term.
Model diagnostic tests
Heteroskedasticity and serial correlation tests
According to the Breusch-Pagan-Godfrey tests, there is no evidence of Heteroskedasticity in the models in both the short and long-runs. Moreover, the p-values for the serial correlation tests show non-statistical significance at the 1% level which shows evidence of no serial correlation as presented in Appendix A.
Model stability tests
As proposed by Brown, Durbin and Evans (1975) and suggested by Pesaran et al. (Citation2001) within the ARDL framework, this is thus confirmed by the plots of the CUSUM and CUSUMSQ tests. The CUSUM and CUSUMSQ residuals lines lie within the 5 percent critical value bounds (see Appendix B) indicating stability in the estimated model. From Appendix B, all the tests indicate that the model fits the data for the analysis. This is shown by the blue line lying in between the red lines in all the boxes. This means the models are stable in all instances.
Impulse response results
Impulse response function (IRF) analyses were also conducted to complement and serve as a robustness check on the findings from the econometric estimations. The study specifically analyzes how global economic policy uncertainties, crude oil prices and stock returns respond to the COVID-19 stringency and health containment Shocks.
Effects of COVID-19 stringency shocks
The results from the IRF as presented in Appendix C reveal that when there is a stringency shock, the returns of the market fall sharply at the initial stages, remain stable and negative during the 3rd month and gradually begin to decay back to a steady state from the 8th month. The observed pattern in the data from the Impulse Response Function (IRF) conforms to the Efficient Market Hypothesis (EMH). As per the Efficient Market Hypothesis (EMH), stock prices rapidly assimilate and mirror all accessible information. Within the framework of a stringency shock, we interpret the initial significant decline in market returns as an immediate response to the newly acquired knowledge or shock. Over time, the negative returns continue for a few months, indicating that the market adapts to the consequences of the strict shock. This is consistent with the semi-strong variant of the Efficient Market Hypothesis (EMH), which states that stock prices adjust gradually in response to new information. The following decline and restoration to a stable condition from the 8th month may suggest that market participants have completely absorbed and factored in the effects of the strict shock, thereby reinstating a state of balance. Nevertheless, the consistent and pessimistic trend found in the third month suggests that certain market inefficiencies or behavioural factors are impacting the continuation of negative returns shortly which departs from the rigid efficiency assumptions of the Efficient Market Hypothesis (EMH). Our finding is confirmed by the empirical estimations presented in and Zoungrana, Toé and Toé, (Citation2023). Moreover, Raifu (Citation2022) notes that the announcement of government policy interventions has a direct negative and an indirect positive effect on stock returns. Their IRF results equally show that initially, the stock returns react negatively to cases, deaths and GPIs, however, the negative effect flattens over time. For global economic policy uncertainty, the shock at the initial stages causes a rise in policy uncertainty up to the 3rd month, remains stable for a while and then begins to cause policy uncertainty to fall back to its steady state in the long-run. Moreover, the stringency shock causes crude oil prices to rise slowly and approach the initial values in the 10th. The behaviours of the variables depicted by the impulse response functions justify the ARDL results and that things begin to normalize in the long-run after the implementation of the stringency policy. These also explain the concept of mean reversion, market efficiency and the implications of market overreaction discussed earlier. Moreover, these suggest the weak-form market efficiency in the Ghanaian stock market.
Effects of health containment Shocks
Introducing the health containment as a shock to the system caused the returns of the stock market in Ghana to fall very sharply in the first 1 month as shown in Appendix D. The returns remain stable for the 3rd month and finally, the effect begins to decay slowly back to its steady state. The shock causes its values to decline sharply in the first 6 months and finally become stable. A one standard deviation shock of the health containment index caused the global economic policy uncertainty to rise slowly at the early stages and then begin to decrease slowly from the 2nd month up to the 8th month when it remains back to its initial state. Similar to the shock of the stringency index, at the early stage when the health containment policy was implemented, prices of crude oil fell to negative. This effect died out slowly back to the original state in the 8th month. These dynamics equally explain our regression outputs for the long-run and short-run. All the impulse response functions for all the variables lie within the 5% confidence interval which confirms the robustness of our regression and the stability of the model as shown by the CUSUM of squares tests.
Our findings reveal significant observations regarding the immediate and prolonged fluctuations of Ghana’s stock market in reaction to the COVID-19 pandemic and governmental initiatives. The current returns are influenced by the negative consequences of previous stock values in the short term which indicates the presence of market reversals, overreactions and corrections. This finding is consistent with comparable discoveries made in the literature on China and the United States. Moreover, the observed negative correlation between the stringency index, global economic policy uncertainty and stock returns at different levels of statistical significance supports previous research findings. This emphasizes the Ghanaian stock market’s susceptibility to pandemic-related constraints and uncertainties. We expand upon current understanding by examining the long-term impacts of the COVID-19 pandemic on the stock market. The long-term persistence of negative relationships between the stringency index and global economic policy uncertainty demonstrates the lasting influence of government interventions. The coefficients of the error correction term signify the progressive restoration of the system to its equilibrium state, revealing the enduring adverse impacts of both the COVID-19 stringency and health containment indices on stock returns in the long run.
Our findings have theoretical implications that enhance our knowledge of market behaviour during pandemics. We stress the significance of taking into account both short-term fluctuations and long-term equilibrium in stock markets. The confirmation of theoretical expectations supports the idea of mean reversion and the weak-form market efficiency hypothesis in the stock market of Ghana. The study provides relevant useful perspectives for investors, policymakers and market participants from a management standpoint. The adverse immediate consequences show the necessity for prudent decision-making during moments of increased uncertainty. Policymakers should aim to achieve equilibrium between containment measures and market stability to sustain investor confidence. Investors should be cognizant of the possibility of market reversals and declines that may arise from pandemic-induced shocks. In the long run, we highlight the importance of global economic policy uncertainty in influencing the direction of the stock market over time. Policymakers should take into account the wider economic landscape when conducting interventions, while investors may gain advantages from long-term plans that incorporate ongoing uncertainties. The impulse response function provides further evidence to enhance the reliability of the results by demonstrating how market variables react to shocks originating from the stringency and health containment indices.
Conclusion
This paper assessed the impact of government restrictions on the returns of the Ghana Stock Exchange Composite Index inculcating two other important variables in the models; global economic policy uncertainty and crude oil prices. The study used the Autoregressive-Distributive Lag (ARDL) model to analyze its data from February 2020 to December 2022. The study aims to find out both the long-term and short-term impacts of government restrictions on the returns of the stock market in the Ghanaian context. The outcomes of the paper indicated that the COVID-19 stringency index negatively affects the performance of the stock market in Ghana in both the short-run and long-run. This supports the findings of several authors (Adam & Yacob, Citation2022; Christou et al., Citation2017; Gao et al., Citation2019; Gea et al., Citation2021; Kang et al., Citation2021; Lee & Chen, Citation2021; Mbanyele, Citation2023; Wang & Zhang, Citation2021; Wu, Citation2022; Yousuf, Citation2021; Zhang et al., Citation2023). However, the paper finds no evidence of the impact of the health containment index on the returns of the stock market in Ghana but the existence of a negative relationship with the returns of the market in both the short run and the long run.
Based on the results of the study, we encourage investors to diversify their investments to capitalize on short-term profits during pandemic-induced restrictions. Nevertheless, they should proceed with prudence due to the possibility of enduring long-term losses linked to rigorous actions. Furthermore, we advise that publicly traded companies should modify their business strategies to effectively navigate uncertainty in difficult circumstances. Ultimately, we recommend that governments should bolster communication and transparency in policy decisions to establish and uphold investor confidence. It is advisable to introduce targeted economic stimulus measures during periods of increased restrictions to offset any potential adverse impacts on stock markets and maintain stability in the financial market. Furthermore, given the dynamic nature of the COVID-19 pandemic and its impact on the global economy, future research should investigate the developing connection between government restrictions, health containment measures and stock market returns in Ghana, taking into account more recent data and potential moderating factors.
The main limitation of the study is that the sample used for the study is too small as allowed by the ARDL model. Moreover, the study did not include other important variables such as stock market volatility, Covid 19 cases, other macroeconomic variables such as inflation, DGP, global financial crisis etc. It is recommended for future studies to include these variables in robust models such as quantile ARDL (QARDL) or even a non-linear regression model to estimate the impact of government restrictions on the performance of the Ghanaian stock exchange.
Authors’ contributions
Iddriss Askandir: Conceptualization, Data curation, Formal analysis, Writing – original draft.
Eric Amoo Bondzie: Conceptualization, Data curation, Formal analysis, Writing – original draft, review and editing.
George Tweneboah: Formal analysis, Writing – review and editing.
Disclosure statement
The authors declare that they have no known competing financial interests or personal relationships that could have appeared to influence the paper.
Data availability statement
The data that support the findings of this study are available from the corresponding author, EAB, upon reasonable request.
Additional information
Funding
Notes on contributors
Iddriss Askandir
Iddriss Askandir is a graduate student at the University of Cape Coast in Ghana, where he is currently pursuing his Master of Philosophy in Economics and a level 2 student at the Institute of Chartered Accountants Ghana (ICAG). He earned his bachelor’s degree in finance from the University of Cape Coast. His primary research interest is in the interactions between monetary policy, financial markets, and economic variables.
Eric Amoo Bondzie
Eric Amoo Bondzie is currently a senior lecturer at the Department of Economic Studies, University of Cape Coast – Ghana where he teaches both at the undergraduate and graduate levels. He holds a master’s degree in Economic Policy and Institutions from La Sapienza University of Rome and a PhD in Economics from the Catholic University of Milan all in Italy. His research areas are Macroeconomics, Monetary Economics, DSGE modelling and Time Series Econometrics.
George Tweneboah
George Tweneboah is an associate professor at the Department of Finance, School of Business, University of Cape Coast, Ghana. He has prolific publication records in well-regarded academic journals and provides daily supervision for PhD students. He boasts of a distinguished career marked by scholarly excellence, with a prolific publication record in esteemed economics and finance journals. Professor Tweneboah’s research interests are primarily cantered on various areas, including time-series modelling, exchange rate management, economic integration, financial market development, and fiscal sustainability.
References
- Abdullah, M., Wali Ullah, G. M., & Chowdhury, M. A. F. (2022). The asymmetric effect of COVID-19 government interventions on global stock markets: New evidence from QARDL and threshold regression approaches. Investment Analysts Journal, 51(4), 1–23. https://doi.org/10.1080/10293523.2022.2112665
- Abonongo, J. (2016). Modelling volatility and the risk-return relationship of selected equities on the Ghana stock exchange. [Doctoral dissertation].
- Adam, N., & Yacob, N. (2022). The impact of global economic policy uncertainty, geopolitical risk and oil price on stock market: Evidence from ASEAN countries. International Journal of Academic Research in Accounting, Finance and Management Sciences.
- Adebayo, T. S., Akadiri, S. S., & Rjoub, H. (2022). On the relationship between economic policy uncertainty, geopolitical risk and stock market returns in South Korea: A quantile causality analysis. Annals of Financial Economics, 17(01), 2250008. https://doi.org/10.1142/S2010495222500087
- Adenomon, M. O., Maijamaa, B., & John, D. O. (2022). The effects of Covid-19 outbreak on the Nigerian stock exchange performance: Evidence from GARCH models. Journal of Statistical Modelling and Analytics, 4(1), 25–38. https://doi.org/10.22452/josma.vol4no1.3
- Adu-Gyamfi, M. (2020). Investigating financial statement fraud in Ghana using Beneish M-Score: A case of listed companies on the Ghana stock exchange (GSE). Available at SSRN 3627689.
- African Financials. (2023). Ghana stock exchange share prices. https://africanfinancials.com/ghana-stock-exchange-share-prices/
- Aggarwal, S., Nawn, S., & Dugar, A. (2021). What caused global stock market meltdown during the COVID pandemic–Lockdown stringency or investor panic? Finance Research Letters, 38, 101827. https://doi.org/10.1016/j.frl.2020.101827
- Agyarko, K., Wiah, E. N., Frempong, N. K., & Odoi, B. (2023). Modelling the volatility of the Ghana stock market: A comparative study. International Journal of Statistics and Applied Mathematics, 8(3), 125–132. https://doi.org/10.22271/maths.2023.v8.i3b.998
- Agyei, S. K., Bossman, A., Asafo − Adjei, E., Asiamah, O., Adela, V., & Adorm − Takyi, C. (2022). Exchange rate, COVID-19 and stock returns in Africa: Insights from time-frequency domain. Discrete Dynamics in Nature and Society, 2022, 1–20. https://doi.org/10.1155/2022/4372808
- Aharon, D. Y., Baig, A. S., & DeLisle, R. J. (2022). The impact of government interventions on cross-listed securities: Evidence from the COVID-19 pandemic. Finance Research Letters, 46, 102276. https://doi.org/10.1016/j.frl.2021.102276
- Aharon, D. Y., Jacobi, A., Cohen, E., Tzur, J., & Qadan, M. (2021). COVID-19, government measures and hospitality industry performance. PloS One, 16(8), e0255819. https://doi.org/10.1371/journal.pone.0255819
- Akomea-Bonsu, C., & Sampong, F. (2013). Is it worthwhile investing on the Ghana stock exchange. Global Journal of Commerce & Management Perspective, 2(4), 49–60.
- Albulescu, C. T. (2021). COVID-19 and the United States financial markets’ volatility. Finance Research Letters, 38, 101699. https://doi.org/10.1016/j.frl.2020.101699
- Alexandridi, M., Mazej, J., Palermo, E., & Hiscott, J. (2022). The Coronavirus pandemic–2022: Viruses, variants & vaccines. Cytokine & Growth Factor Reviews, 63, 1–9. https://doi.org/10.1016/j.cytogfr.2022.02.002
- Allam, S. (2024). Investment Strategies and Covid-19 Pandemic: A Case of The Egyptian Stock Exchange. Scientific Journal of Financial and Commercial Studies and Research, 5(1), 371–396. https://doi.org/10.21608/cfdj.2024.324101
- Al-Thaqeb, S. A., & Algharabali, B. G. (2019). Economic policy uncertainty: A literature review. The Journal of Economic Asymmetries, 20, e00133. https://doi.org/10.1016/j.jeca.2019.e00133
- Amoah, J. O., Addai-Mununkum, T., Oduro, G. Y., Odoi, A., Ansah, J. W., Takyiakwaa, D., & Sakyi-Djan, P. (2023). Effects of COVID-19 on coastal livelihoods in the central region of Ghana. Social Sciences & Humanities Open, 7(1), 100443. https://doi.org/10.1016/j.ssaho.2023.100443
- Amoako, M., Amoah-Agyei, F., Mensah, G. O., Du, C., Sergin, S., Fenton, J. I., & Tucker, R. M. (2022). Effects of the COVID-19 pandemic on health behaviors of higher education students in Ghana: A cross-sectional study. International Journal of Environmental Research and Public Health, 19(24), 16442. https://doi.org/10.3390/ijerph192416442
- Ansah, M. (2020). Akufo-Addo announces partial lockdown of Accra, Kumasi, Tema to curb COVID-19 spread. Citinewsroom – Comprehensive News in Ghana. https://citinewsroom.com/2020/03/akufo-addo-announces-partial-lockdown-of-accra-kumasi-tema-to-curb-covid-19-spread/
- Arshizadeh, S., Gorgani, S. H., Taheri, P., Givgol, M., Shahrokhi, S., & Abdalisousan, A. (2021). The impact of COVID-19 on oil supply in the short term. Advanced Journal of Science and Engineering, 2(2), 120–135.
- Asafo-Adjei, E., Agyapong, D., Agyei, S. K., Frimpong, S., Djimatey, R., & Adam, A. M. (2020). 2020). Economic policy uncertainty and stock returns of Africa: A wavelet coherence analysis. Discrete Dynamics in Nature and Society, 2020, 1–8. (https://doi.org/10.1155/2020/8846507
- Asafo-Adjei, E., Boateng, E., Isshaq, Z., Idun, A. A. A., Owusu Junior, P., & Adam, A. M. (2021). Financial sector and economic growth amid external uncertainty shocks: Insights into emerging economies. PloS One, 16(11), e0259303. https://doi.org/10.1371/journal.pone.0259303
- Badmus, J. O., & Ojelade, I. M. (2022). Covid-19 vaccination and stock markets performance: Empirical evidence from African countries. International Journal of Business and Economics Research, 11(5) 264–275.
- Bakry, W., Kavalmthara, P. J., Saverimuttu, V., Liu, Y., & Cyril, S. (2022). Response of stock marketvolatility to COVID-19 announcements and stringency measures: A comparison ofdeveloped and emerging markets. Finance Research Letters, 46, 102350. https://doi.org/10.1016/j.frl.2021.102350
- Bello, M. (2022). Stock market reaction to COVID-19 lockdown announcement in Nigeria: An event study approach. Bayero Business Review, 6(2), 16–30.
- Blackledge, J., & Lamphiere, M. (2021). A review of the fractal market hypothesis for trading and market price prediction. Mathematics, 10(1), 117. https://doi.org/10.3390/math10010117
- Bukari, C., Aning-Agyei, M. A., Kyeremeh, C., Essilfie, G., Amuquandoh, K. F., Owusu, A. A., Otoo, I. C., & Bukari, K. I. (2022). Effect of COVID-19 on household food insecurity and poverty: Evidence from Ghana. Social Indicators Research, 159(3), 991–1015. https://doi.org/10.1007/s11205-021-02766-9
- Chow, Y. P., & Tan, Y. H. (2022). Impact of COVID-19 and government interventions on Bursa Malaysia sectors. Journal of Chinese Economic and Foreign Trade Studies, 16(2), 99–118. https://doi.org/10.1108/JCEFTS-06-2022-0039
- Christou, C., Cunado, J., Gupta, R., & Hassapis, C. (2017). Economic policy uncertainty and stock market returns in PacificRim countries: Evidence based on a Bayesian panel VAR model. Journal of Multinational Financial Management, 40, 92–102. https://doi.org/10.1016/j.mulfin.2017.03.001
- Covid19 - Ministry of Health. (2020). Ministry of Health. https://www.moh.gov.gh/covid-19/
- Dadzoe, E., Darko, E. K., Djanfosu, A. L., & Donkor, A. (2021). The Impact of COVID-19 Pandemic on the Ghana Stock Market. [Undergraduate Thesis]. UCC.
- Dawda, T. D., & File, D. J. M. B. (2023). Assessing the Impact of COVID-19 on Tourism in Northern Ghana: Role of Local Governments for Destination Recovery. In K. Dube, G. Nhamo & M. P. Swart (Eds.), COVID-19, tourist destinations and prospects for recovery: A global perspective (pp. 199–215). Springer.
- Deri, M. N., Ari Ragavan, N., Niber, A., Zaazie, P., Akazire, D. A., Anaba, M., & Andaara, D. (2023). COVID-19 shock in the hospitality industry: its effect on hotel operations within the Bono Region of Ghana. African Journal of Economic and Management Studies, 14(3), 355–378. https://doi.org/10.1108/AJEMS-07-2022-0264
- Director-General, W. H. O. (2020). WHO director-general’s opening remarks at the media briefing on COVID-19. World Health Organization.
- Ehiedu, V. C., & Obi, C. K. (2022). Efficient market hypothesis (EMH) and the Nigerian stock exchange in the midst of global financial crises. International Journal of Academic Management Science Research, 6(8), 263–273.
- Ertuğrul, H. M., Güngör, B. O., & Soytaş, U. (2020). The effect of the COVID-19 outbreak on the Turkish diesel consumption volatility dynamics. Energy Research Letters, 1(3). https://doi.org/10.46557/001c.17496
- Fama, E. F. (1965). The behavior of stock market prices. Journal of Business, 38(1), 31–105.
- Fama, E. F. (1970). Efficient capital markets: A review of theory and empirical work. The Journal of Finance, 25(2), 383–417. https://doi.org/10.2307/2325486
- Feng, Z., Chen, W., Liu, Y., Chen, H., & Skibniewski, M. J. (2023). Long-term equilibrium relationship analysis and energy-saving measures of metro energy consumption and its influencing factors based on cointegration theory and an ARDL model. Energy, 263, 125965. https://doi.org/10.1016/j.energy.2022.125965
- Feng, G. F., Yang, H. C., Gong, Q., & Chang, C. P. (2021). What is the exchange rate volatility response to COVID-19 and government interventions? Economic Analysis and Policy, 69, 705–719. https://doi.org/10.1016/j.eap.2021.01.018
- Gao, J., Zhu, S., O’Sullivan, N., & Sherman, M. (2019). The role of economic uncertainty in UK stock returns. Journal of Risk and Financial Management, 12(1), 5. https://doi.org/10.3390/jrfm12010005
- Gea, C., Vereda, L., Pinto, A. C. F., & Klotzle, M. C. (2021). The effects of economic policy uncertainty on stock market returns: Evidence from Brazil. Brazilian Review of Finance, 19(3), 53–84. https://doi.org/10.12660/rbfin.v19n3.2021.83014
- Gunawan, M. I., & Nainggolan, Y. A. Stringency Index and Stock Market Return Amidst COVID-19 Pandemic: Evidence from Emerging Stock Market Countries
- Guven, M., Cetinguc, B., Guloglu, B., & Calisir, F. (2022). The effects of daily growth in COVID-19 deaths, cases and governments’ response policies on stock markets of emerging economies. Research in International Business and Finance, 61, 101659. https://doi.org/10.1016/j.ribaf.2022.101659
- Hale, T., Webster, S., Petherick, A., Phillips, T., & Kira, B. (2020). Oxford COVID-19 government response tracker (OxCGRT). Last Updated, 8, 30.
- Hervie, D. M., Amoako-Atta, E., Hossain, M. B., Illés, C. B., & Dunay, A. (2022). Impact of COVID-19 pandemic on hotel employees in the greater Accra region of Ghana. Sustainability, 14(5), 2509. https://doi.org/10.3390/su14052509
- Hoshikawa, T., & Yoshimi, T. (2021). The Effect of the COVID-19 pandemic on South Korea’s stock market and exchange rate. The Developing Economies, 59(2), 206–222. https://doi.org/10.1111/deve.12276
- Huo, X., & Qiu, Z. (2020). How does China’s stock market react to the announcement of the COVID-19 pandemic lockdown? Economic and Political Studies, 8(4), 436–461. https://doi.org/10.1080/20954816.2020.1780695
- Insaidoo, M., Arthur, L., Amoako, S., & Andoh, F. K. (2021). Stock market performance and COVID-19 pandemic: Evidence from a developing economy. Journal of Chinese Economic and Foreign Trade Studies, 14(1), 60–73. https://doi.org/10.1108/JCEFTS-08-2020-0055
- Kang, W., de Gracia, F. P., & Ratti, R. A. (2021). Economic uncertainty, oil prices, hedging and US stock returns of the airline industry. The North American Journal of Economics and Finance, 57, 101388. https://doi.org/10.1016/j.najef.2021.101388
- Kassamany, T., & Zgheib, B. (2023). Impact of government policy responses of COVID-19 pandemic on stock market liquidity for Australian companies. Australian Economic Papers, 62(1), 24–46. https://doi.org/10.1111/1467-8454.12280
- Khan, N., Fahad, S., Naushad, M., & Faisal, S. (2020). COVID-2019 locked down effects on oil prices and its effects on the world economy. Available at SSRN 3588810.
- Khanthavit, A. (2020). World and national stock market reactions to COVID-19. ABAC Journal, 40(2), 1–20.
- Kissi, J., Owusu-Marfo, J., Osei, E., Dzamvivie, K., Akorfa Anku, V., & Naa Lamiokor Lamptey, J. (2022). Effects of coronavirus pandemic on expanded program on immunization in Weija Gbawe Municipality (Accra-Ghana). Human Vaccines & Immunotherapeutics, 18(6), 2129830. https://doi.org/10.1080/21645515.2022.2129830
- Kubatko, O., Merritt, R., Duane, S., & Piven, V. (2023). The impact of the COVID-19 pandemic on global food system resilience. Mechanism of an Economic Regulation, 1(99), 144–148.
- Kurtović, H., & Markarian, G. (2024). Tail risks and private equity performance. Journal of Empirical Finance, 75, 101457. https://doi.org/10.1016/j.jempfin.2023.101457
- Latunde, T., Akinola, L. S., & Dare, D. D, Department of Mathematics, Federal University Oye-Ekiti, Oye-Ekiti, Nigeria. (2020). Analysis of capital asset pricing model on Deutsche bank energy commodity. Green Finance, 2(1), 20–34. https://doi.org/10.3934/GF.2020002
- Lawal, N. A., Osinusi, K. B., & Badmus, J. O. (2020). COVID-19 Pandemic and Stock Market Performance in Nigeria. African Journal of Sustainable Development, 10(3), 131–145.
- Le, T. H., Le, A. T., & Le, H. C. (2021). The historic oil price fluctuation during the Covid-19 pandemic: What are the causes? Research in International Business and Finance, 58, 101489. https://doi.org/10.1016/j.ribaf.2021.101489
- Lee, C. C., & Chen, M. P. (2021). The effects of investor attention and policy uncertainties on cross-border country exchange-traded fund returns. International Review of Economics & Finance, 71, 830–852. https://doi.org/10.1016/j.iref.2020.10.015
- Lee, C. C., Lee, C. C., & Wu, Y. (2023). The impact of COVID-19 pandemic on hospitality stock returns in China. International Journal of Finance & Economics, 28(2), 1787–1800. https://doi.org/10.1002/ijfe.2508
- Li, M. L., Bouardi, H. T., Lami, O. S., Trikalinos, T. A., Trichakis, N., & Bertsimas, D. (2023). Forecasting COVID-19 and analyzing the effect of government interventions. Operations Research, 71(1), 184–201. https://doi.org/10.1287/opre.2022.2306
- Li, H., Chen, H., Xu, G., & Zhang, W. (2023). COVID-19, various government interventions and stock market performance. China Finance Review International, 13(4), 621–632. https://doi.org/10.1108/CFRI-03-2023-0068
- Lin, Z. (2018). Modelling and forecasting the stock market volatility of SSE composite index using GARCH models. Future Generation Computer Systems, 79, 960–972. https://doi.org/10.1016/j.future.2017.08.033
- Lo, A. W. (2004). The adaptive markets hypothesis: Market efficiency from an evolutionary perspective. The Journal of Portfolio Management, 30(5), 15–29. https://doi.org/10.3905/jpm.2004.442611
- Manahov, V., & Hudson, R. (2014). A note on the relationship between market efficiency and adaptability–New evidence from artificial stock markets. Expert Systems with Applications, 41(16), 7436–7454. https://doi.org/10.1016/j.eswa.2014.06.004
- Maquieira, C. P., Espinosa-Méndez, C., & Gahona-Flores, O. (2023). How does economic policy uncertainty (EPU) impact copper-firms stock returns? International evidence. Resources Policy, 81, 103372. https://doi.org/10.1016/j.resourpol.2023.103372
- Mbanyele, W. (2023). Economic policy uncertainty and stock liquidity: The role of board networks in an emerging market. International Journal of Emerging Markets, 18(1), 122–147. https://doi.org/10.1108/IJOEM-05-2020-0492
- Min, H. (2023). Assessing the impact of a COVID-19 pandemic on supply chain transformation: An exploratory analysis. Benchmarking: An International Journal, 30(6), 1765–1781. https://doi.org/10.1108/BIJ-04-2022-0260
- Mishra, R., Sharma, R., Karedla, Y., & Patel, N. (2022). Impact of COVID-19 cases, deaths, stringency and vaccinations on the US stock market. Vision: The Journal of Business Perspective, 097226292210749. https://doi.org/10.1177/09722629221074901
- Mlambo, C., & Biekpe, N. (2001). Investment Basics XLIV: Review of African stock markets. MPRA Paper, 24973.
- Mohammed, K. (2023). The effects of the quarantine imposed on Algerian society in light of the spread of The COVID-19 pandemic. Elementary Education Online, 22(2), 102–102.
- Mohanty, P., & Mishra, S. (2023). Did the Indian stock market overreact to Covid-19? The North American Journal of Economics and Finance, 70, 102072. https://doi.org/10.1016/j.najef.2023.102072
- Musah, A., Padi, A., & Ahmed, I. A. (2022). The effect of the COVID-19 pandemic on the financial performance of commercial banks in Ghana. International Journal of Accounting & Finance Review, 12(1), 21–29.
- Narayan, P. K. (2004). Fiji’s tourism demand: The ARDL approach to cointegration. Tourism Economics, 10(2), 193–206. https://doi.org/10.5367/000000004323142425
- Narayan, P. K., & Narayan, S. (2005). Estimating income and price elasticities of imports for Fiji in a cointegration framework. Economic Modelling, 22(3), 423–438. https://doi.org/10.1016/j.econmod.2004.06.004
- Nyavi, G. A. (2020). Ghana closes its borders for 2 weeks to fight Coronavirus. Graphic Online. https://www.graphic.com.gh/news/general-news/ghana-news-coronavirus-borders-closed-directive-will-last-2-weeks.html
- Ofori-Boateng, K., Agyapong, E. K., Ohemeng, W., & Annor, L. D. J. (2022). Commodity price volatility and stock market returns in an emerging economy. International Social Science Journal, 72(244), 321–337. https://doi.org/10.1111/issj.12320
- Ofori-Boateng, K., Ohemeng, W., Agyapong, E. K., & Bribinti, B. J. (2022). The impact of COVID-19 on stock returns of listed firms on the stock market: Ghana’s experience. African Journal of Economic and Management Studies, 13(1), 136–146. https://doi.org/10.1108/AJEMS-02-2021-0074
- Onyango, E. O., Owusu, B., & Crush, J. S. (2023). COVID-19 and urban food security in Ghana during the third wave. Land, 12(2), 504. https://doi.org/10.3390/land12020504
- Opoku-Agyemang, B., Ofori-Abebrese, G., & Korankye, B. (2016). Analysis of interest rate and stock price in africa: Case study of ghanaian listed firms. International Journal of Science and Research, 5(10), 1582–1587.
- Osae, P. Y. O. (2021). Corporate sustainability disclosure and financial performance of listed firms in Ghana. [Doctoral dissertation]. University of Cape Coast.
- Owjimehr, S., & Samadi, A. H. (2022). Government policy response to COVID-19 and stock market return: The case of Iran. Socioeconomic dynamics of the COVID-19 crisis: Global, regional and local perspectives, 423–439.
- Ozili, P. K., & Arun, T. (2023). Spillover of COVID-19: Impact on the global economy. In Managing inflation and supply chain disruptions in the global economy (pp. 41–61). IGI Global.
- Panchal, U., Salazar de Pablo, G., Franco, M., Moreno, C., Parellada, M., Arango, C., & Fusar-Poli, P. (2023). The impact of COVID-19 lockdown on child and adolescent mental health: Systematic review. European Child & Adolescent Psychiatry, 32(7), 1151–1177. https://doi.org/10.1007/s00787-021-01856-w
- Pesaran, M. H., & Pesaran, B. (1997). Working with microfit 4.0: Interactive econometric analysis. Oxford University Press.
- Pesaran, M. H., Shin, Y., & Smith, R. J. (2001). Bounds testing approaches to the analysis of level relationships. Journal of Applied Econometrics, 16(3), 289–326. https://doi.org/10.1002/jae.616
- Qamruzzaman, M., & Jianguo, W. (2018). Nexus between financial innovation and economic growth in South Asia: Evidence from ARDL and nonlinear ARDL approaches. Financial Innovation, 4(1), 20. https://doi.org/10.1186/s40854-018-0103-3
- Qiu, W., Rutherford, S., Mao, A., & Chu, C. (2017). The pandemic and its impacts. Health, Culture and Society, 9, 1–11. https://doi.org/10.5195/HCS.2017.221
- Rababah, A., Al-Haddad, L., Sial, M. S., Chunmei, Z., & Cherian, J. (2020). Analyzing the effects of COVID-19 pandemic on the financial performance of Chinese listed companies. Journal of Public Affairs, 20(4), e2440.
- Raifu, I. A. (2022). COVID-19 pandemic, government policy responses and stock market performance in some selected African countries. SSRN Electronic Journal, 4, 24–65. https://doi.org/10.2139/ssrn.4235242
- Raji, I. (2012). Effects of ownership structure on the performance of listed companies on the Ghana stock exchange. [Doctoral dissertation]. University of Cape Coast.
- Riadi, D. R., Garnia, E., & Tahmat, T. T. (2023). Impact of The Covid 19 Pandemic: Is There Overreaction in LQ 45 Stock? Jurnal Manajemen Dan Keuangan, 12(1), 178–194. https://doi.org/10.33059/jmk.v12i1.4727
- Rojo-Suárez, J., & Alonso-Conde, A. B. (2024). Have shifts in investor tastes led the market portfolio to capture ESG preferences? International Review of Financial Analysis, 91, 103019. https://doi.org/10.1016/j.irfa.2023.103019
- Rowland, R., Chia, R. C. J., & Liew, V. K. S. (2023). Do non-pharmaceutical policies in response to COVID-19 affect stock performance? Evidence from Malaysia stock market return and volatility. PloS One, 18(1), e0277252. https://doi.org/10.1371/journal.pone.0277252
- Sansa, N. A. (2020). The Impact of the COVID-19 on the financial markets: Evidence from China and USA. Electronic Research Journal of Social Sciences and Humanities, 2, 29–39.
- Shen, H., Fu, M., Pan, H., Yu, Z., & Chen, Y. (2020). The impact of the COVID-19 pandemic on firm performance. Emerging Markets Finance and Trade, 56(10), 2213–2230. https://doi.org/10.1080/1540496X.2020.1785863
- Sinaga, J., Wu, T., & Chen, Y. W. (2022). Impact of government interventions on the stock market during COVID-19: A case study in Indonesia. SN Business & Economics, 2(9), 136. https://doi.org/10.1007/s43546-022-00312-4
- Spierdijk, L., Bikker, J. A., & Van den Hoek, P. (2012). Mean reversion in international stock markets: An empirical analysis of the 20th century. Journal of International Money and Finance, 31(2), 228–249. https://doi.org/10.1016/j.jimonfin.2011.11.008
- Sridhar, A., Balakrishnan, A., Jacob, M. M., Sillanpää, M., & Dayanandan, N. (2023). Global impact of COVID-19 on agriculture: Role of sustainable agriculture and digital farming. Environmental Science and Pollution Research International, 30(15), 42509–42525. https://doi.org/10.1007/s11356-022-19358-w
- Sunaryati, S., & Munandar, A. (2023). The COVID-19 pandemic and the exchange rate: A lesson learned from Indonesia. Jurnal Ekonomi & Studi Pembangunan, 24(1), 1–13. https://doi.org/10.18196/jesp.v24i1.16110
- Syed, A. A. S., Fatima, K., & Zaheer, M. (2022). The impact of COVID-19 on stock market and exchange rate uncertainty in Pakistan. Business Review, 16(2), 96–108. https://doi.org/10.54784/1990-6587.1423
- Takyi, P. O., & Bentum-Ennin, I. (2021). The impact of COVID-19 on stock market performance inAfrica: A Bayesian structural time series approach. Journal of Economics and Business, 115, 105968. https://doi.org/10.1016/j.jeconbus.2020.105968
- Tetteh, J. E., Amoah, A., Ofori-Boateng, K., & Hughes, G. (2022). Stock market response to COVID-19 pandemic: A comparative evidence from two emerging markets. Scientific African, 17, e01300. https://doi.org/10.1016/j.sciaf.2022.e01300
- Tiryaki, H. N., & Tiryaki, A. (2019). Determinants of Turkish stock returns under the impact of economic policy uncertainty. Uluslararası İktisadi ve İdari İncelemeler Dergisi, (22), 147–162. https://doi.org/10.18092/ulikidince.424369
- Ullah, S. (2023). Impact of COVID-19 pandemic on financial markets: A global perspective. Journal of the Knowledge Economy, 14(2), 982–1003. https://doi.org/10.1007/s13132-022-00970-7
- Ullah, A., Zhao, X., Amin, A., Syed, A., & Riaz, A. (2023). Impact of COVID-19 and economic policy uncertainty on China’s stock market returns: Evidence from quantile-on-quantile and causality-in-quantiles approaches. Environmental Science and Pollution Research, 30(5), 12596–12607.
- Ulzen, T. (2017). 1990: The Ghana stock exchange is established—Edward A. Ulzen Memorial Foundation. Edward Ulzen memorial foundation. https://www.eaumf.org/ejm-blog/2017/11/12/november-12-1990-the-ghana-stock-exchange-is-established
- Wang, X., Wang, X., & Zhai, Y. (2023). Advancing sustainable financial management in greening companies through big data technology innovation. Environmental Science and Pollution Research International, 31(4), 5641–5654. https://doi.org/10.1007/s11356-023-30950-6
- Wang, Y., Zhang, H., Gao, W., & Yang, C. (2021). COVID-19-related government interventions and travel and leisure stock. Journal of Hospitality and Tourism Management, 49, 189–194. https://doi.org/10.1016/j.jhtm.2021.09.010
- Wang, S., & Zhang, X. (2021). The impact of macroeconomic uncertainty on China’s stock market—Take the Shanghai composite index as an example [Paper presentation]. 2021 2nd Asia-Pacific Conference on Image Processing, Electronics and Computers, (pp. 515–519). https://doi.org/10.1145/3452446.3452573
- Weaver, R. L. (2023). The impact of COVID-19 on the social enterprise sector. Journal of Social Entrepreneurship, 14(2), 177–185. https://doi.org/10.1080/19420676.2020.1861476
- World Trade Organization. (2020). E-commerce, trade and the COVID-19 pandemic. WTO Working Papers, No. 2020/05.
- Worldometer. (2023). Ghana COVID – Coronavirus statistics. https://www.worldometers.info/coronavirus/country/ghana/#graph-cases-daily
- Worldometer. (2023). Reported Cases and Deaths. https://www.worldometers.info/coronavirus/
- Wu, J. (2022). Economic policy uncertainty, investor sentiment and stock price synchronisation: Evidence from China. Mathematical Problems in Engineering.
- Xu, Y., Wang, J., Chen, Z., & Liang, C. (2021). Economic policy uncertainty and stock market returns: New evidence. The North American Journal of Economics and Finance, 58, 101525. https://doi.org/10.1016/j.najef.2021.101525
- Xu, L., Yang, Z., Chen, J., & Zou, Z. (2023). Impacts of the COVID-19 epidemic on carbon emissions from international shipping. Marine Pollution Bulletin, 189, 114730. https://doi.org/10.1016/j.marpolbul.2023.114730
- Yan, C. (2020). COVID-19 outbreak and stock prices: Evidence from China. Available at SSRN 3574374.
- Yang, C., Abedin, M. Z., Zhang, H., Weng, F., & Hajek, P. (2023). An interpretable system for predicting the impact of COVID-19 government interventions on stock market sectors. Annals of Operations Research, 1–28. https://doi.org/10.1007/s10479-023-05311-8
- Yang, H., & Deng, P. (2021). The impact of COVID-19 and government intervention on stock markets of OECD countries. Asian Economics Letters, 1(4), 18646. https://doi.org/10.46557/001c.18646
- Yh Saif-Alyousfi, A. (2022). The impact of COVID-19 and the stringency of government policy responses on stock market returns worldwide. Journal of Chinese Economic and Foreign Trade Studies, 15(1), 87–105.
- Yong, H. H. A., & Laing, E. (2021). Stock market reaction to COVID-19: Evidence from US firms’ international exposure. International Review of Financial Analysis, 76, 101656. https://doi.org/10.1016/j.irfa.2020.101656
- Yousuf, S. K. (2021). The impact of economic policy uncertainty on UK stock market. Saudi Journal of Economics and Finance, 5(3), 107–113. https://doi.org/10.36348/sjef.2021.v05i03.002
- Yu, X., & Huang, Y. (2021). The impact of economic policy uncertainty on stock volatility: Evidence from GARCH–MIDAS approach. Physica A: Statistical Mechanics and Its Applications, 570, 125794. https://doi.org/10.1016/j.physa.2021.125794
- Yu, X., & Xiao, K. (2023). COVID-19 government restriction policy, COVID-19 vaccination and stock markets: Evidence from a global perspective. Finance Research Letters, 53, 103669. https://doi.org/10.1016/j.frl.2023.103669
- Zaremba, A., Aharon, D. Y., Demir, E., Kizys, R., & Zawadka, D. (2020). COVID-19, government policy responses and stock market liquidity around the world: A note. Research in International Business and Finance, 56, 101359–101359. https://doi.org/10.1016/j.ribaf.2020.101359
- Zhang, M. (2023). The impact of economic policy uncertainty on equity market volatility. BCP Business & Management, 43, 334–341. https://doi.org/10.54691/bcpbm.v43i.4658
- Zhang, L., Chen, W., & Hu, N. (2023). Economic policy uncertainty and stock liquidity: Evidence from China. International Journal of Emerging Markets, 18(1), 22–44. https://doi.org/10.1108/IJOEM-06-2020-0625
- Zhao, N., Yao, S., Thomadsen, R., & Wang, C. B. (2022). The impact of government interventions on COVID-19 spread and consumer spending. Available at SSRN 3725711.
- Zoungrana, T. D., Toé, D. L. t., & Toé, M. (2023). Covid-19 outbreak and stocks return on the West african economic and monetary union’s stock market: An empirical analysis of the relationship through the event study approach. International Journal of Finance & Economics, 28(2), 1404–1422. https://doi.org/10.1002/ijfe.2484
Appendix
Appendix A. Heteroskedasticity and serial correlation tests
Appendix B:
Stability tests
CUSUM test and CUSUM of square tests for the Stability of the Models.
Source: Authors’ construct (2023).
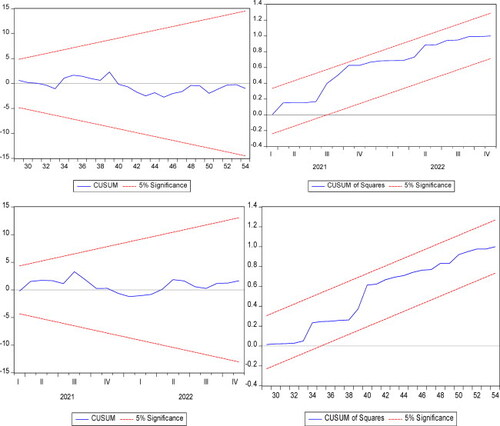