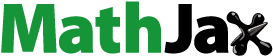
Abstract
Psycho-computing refers to the study of the interaction between humans and computers, with the aim of understanding how people use computer applications in line with their needs and improving their experience. To achieve this goal, it is necessary to have a set of observable and measurable indicators of the behavioral practices of computer application users. Accordingly, a psycho-computing traits scale was developed that extracts six main dimensions of the behavioral practices of computer users and computer science students. To ensure the validity and reliability of the scale, it was applied to a survey sample of 150 students. Subsequently, the final version of the scale was applied to an experimental sample of 722 students to verify its psychometric properties. The results of the study indicate that the psycho-computing traits scale exhibits sound psychometric properties in terms of construct validity, as evidenced by the results of the Rasch model assumptions for the data obtained from its application. Moreover, the confirmatory factor analysis results demonstrate that the goodness of fit indicators align with the main dimensions of the scale. The study’s findings also suggest that the psycho-computing traits scale exhibits high reliability, as indicated by the results of the Rasch model for both person and item reliability. Therefore, the scale can be considered a valid tool for evaluating the psycho-computing traits of computer users and computer science students.
1. Introduction
In recent years, the rapid advancement of computer technology has become a common part of our everyday lives. When it comes to education, incorporating technology requires thoughtful planning and decision making within the educational system to choose modern tools that can enhance learning effectively. According to Al-Hila (Citation2001), integrating technology in education isn’t just about the tools themselves; it’s also about the human aspect involved. Various personal traits play a role in how individuals use computers and the objectives they aim for when using them, as highlighted by Saleh & Al-Ali (Citation2023). Additionally, Lury (Citation2002) points out that an individual’s unique personality traits greatly influence how they adopt and utilize computer technology. Antoncic’s (Citation2009) research further links personality traits with the adoption of computer technology in various contexts.
Extensive research has examined into exploring the intricate connection between an individual’s personality traits and their use of computer technology. It’s widely recognized that this link is complex and can be shaped by various factors, such as the specific technology in use, differences among users and the context in which technology is applied. Turel & Serenko (Citation2012) emphasize the importance of further investigation to deepen our understanding of this relationship.
Engaging with computer technology and examining into its sciences is intertwined with individuals gaining insight into their own traits, understanding the different aspects of these traits and realizing how they intersect with computer usage. Understanding how personality traits and computer technology influence each other is crucial for enhancing self-efficacy in using computers. However, when looking at the admission processes for computer science majors at universities, it’s clear that the focus remains largely on students academic achievements across various subjects. The significance of considering a wide range of personality traits alongside technical practices and how they impact each other, often goes unnoticed. As a result, many students may enter these programs without guidance on what studying computer science entails, its prerequisites and how these requirements align with their own abilities and characteristics (Chong & Chen, Citation2016; Koc, Citation2017).
This lack of understanding results in students enrolling in computer science programs without considering the important role personality traits play and how they can affect their future studies. Hence, it is crucial to acknowledge that psychological aspects and traits have a significant influence on computer usage and performance. The field of computer science requires a careful balance between an individual’s personality characteristics and their proficiency with computers. Saleh & Al-Ali (Citation2023) highlight the significance of exploring these fields to understand the psychological elements that enhance efficient computer utilization and task accomplishment. Grasping these elements and how they interact is vital for enhancing computer education and ensuring individuals possess the right mix of personality attributes and technical competencies.
A common scenario we often encounter in our daily lives is when we seek technical help or assistance for tasks like setting up our devices or taking care of computer hardware and accessories. Interestingly, it’s not uncommon to find individuals offering these services who haven’t gone through formal computer science education at traditional academic institutions. Instead, they’ve gained practical expertise, maintenance skills and programming knowledge through hands on experience. Despite their non traditional educational background, they provide top notch services that help them succeed and stand out in the industry. Sometimes, they even outperform graduates of computer science programs in delivering these specialized services. This exceptional performance can be attributed to their unique blend of personal qualities that complement their technical skills (Saleh & Al-Ali, Citation2023).
It’s crucial to pinpoint reliable signs that can effectively measure a student’s interest in pursuing a computer science degree. These signs can vary based on the specific area of focus within the field of computer science. For example, indicators for studying medicine would include strong performance in foundational sciences, a genuine passion for healthcare, empathy, problem solving abilities and good communication skills. On the other hand, indicators for studying engineering would require a solid understanding of scientific and mathematical principles, proficiency in technical tools and software and strong problem solving skills (Ackerman et al., Citation2013). Therefore, it should be conduct a thorough study to analyze both general and specific signs that influence students choices and specialization in the realm of computer science. This research endeavor would help establish specific criteria that support well informed decision making in this field. Setting clear and precise benchmarks for assessing the skills and capabilities necessary for computer science majors is extremely important. These benchmarks are vital in guiding students towards making educated educational decisions, ultimately improving their academic performance overall. Furthermore, utilizing these evaluation criteria assists in matching students skills with the academic standards, ultimately decreasing dropout rates within particular fields of study (Daempfle, Citation2003; Geisinger et al., Citation2013; Paura & Arhipova, Citation2016).
The field of psycho-computing focuses on examining how humans and computers interact dynamically, influencing each other in predicting future outcomes. This interaction considers how personality traits affect computer usage and vice versa, how computers can impact personality traits. Existing research mainly centers around scales that assess personal characteristics like the Cattell Model of personality traits or academic competencies in computer use such as self efficacy scales for distance learning. However, there is a lack of a comprehensive scale in literature that combines specific behavioral indicators of computer use practices with essential computer skills and related personality traits. A study by Saleh & Al-Ali (Citation2023) emphasizes the need for scales that encompass various personality characteristics affecting computer use to better understand the behavioral practices involving computer technology. The goal is to establish a foundation for examining the compatibility and mutual influence between different aspects of psycho-computing, which can then be used as criteria for guiding and counseling computer users and students studying computer sciences.
The main goal of this research is to review the existing literature and educational technology frameworks to identify the fundamental frameworks and dimensions underlying the psychological and computational characteristics of computer users and students studying computer science. This forms the basis for creating a comprehensive assessment tool that combines personal traits with computer related practices. Such a scale would effectively cater to the requirements of digital environments by offering a holistic evaluation mechanism. The current research challenge stems from the lack, to the best of our knowledge, of concrete markers that link the behavioral patterns of computer users with their corresponding personality traits. Therefore, there is an immediate necessity to develop a specific measure that integrates clear and discernible indicators, aligning computer competencies with individual personality attributes. Hence, the primary objective of this study is to design and implement an assessment tool for assessing psychological traits related to computers. Furthermore, this study aims to validate the psychometric properties of this tool among individuals using computers and students studying computer science. To achieve these goals, we will explore the following research inquiries;
What are the primary dimensions that form the basis of measuring the psycho-computing traits among computer users and computer science students?
What is the proposed approach for developing and constructing a measurement tool for assessing psycho-computing traits among computer users and computer science students?
What are the procedures involved in developing a measurement tool for psycho-computing properties among computer users and computer science students, and how can its psychometric properties be validated?
2. The significance of study
The importance of developing and implementing a scale to evaluate psycho-computing traits in individuals using computers and computer science students is clear for several reasons;
Incorporating Personality Traits with Computer Practices; constructing a scale that merges personality traits with computer related activities helps in pinpointing potential indicators that can predict the success of future computer users and computer science students. This aids in gaining a deeper insight into personal characteristics linked to achievement in computer related fields.
Supportive Tool for Guidance and Counseling; constructing and developing a scale for assessing psycho-computing traits acts as a standardized and beneficial resource for guidance and counseling procedures. It offers guidance to computer users and computer science students, assisting them in personal and professional growth by aligning their strengths and interests with suitable educational paths and career choices.
Continuous Evaluation and Skill Improvement; Constructing and developing such a scale allows for ongoing evaluation, enabling the identification of strengths and areas needing improvement among computer users and computer science students. This, subsequently, empowers them to devise targeted plans for development programs aimed at enhancing their skills in areas requiring enhancement.
3. Literature review
The research, by Ko and colleagues in 2012 suggests that people with levels of neuroticism may be more prone to developing addictions to computer technology like Internet and video games. They might also show signs of Internet addiction, such as use and withdrawal symptoms. On the hand Meier et al.s study from 2016 implies that individuals with neuroticism levels could experience negative emotions while using technology, such as anxiety, frustration and confusion. However a study by So & Mak, (Citation2019) presents contrasting results indicating that technology usage can positively impact the well being of neurotic individuals especially through online social support. These studies examine into aspects related to particular technological features. While exploring these dimensions is crucial it’s essential to recognize that they only represent facets and are not comprehensive in studying the concept of psycho computing across all its dimensions.
In Tsingilis, Citation2019 study the relationship between personality traits and computer and Internet use was investigated. The research highlighted how traits like conscientiousness and agreeableness can influence individuals inclination, towards technology use and addiction.
Barroso et al. (Citation2017) explored how personality traits influence software quality. By using the Big Five personality model they found that developers individual traits have an impact, on the quality of their programs. This highlights the importance of considering characteristics in software development. In a study, Durak et al. (Citation2021) investigated the connection between thinking, lifelong learning skills and personality traits in university students. Their results revealed that extraversion, agreeableness and openness play roles in predicting reasoning with agreeableness showing the strongest impact. Computational thinking was also identified as a predictor of learning competencies. Furthermore, Seibert et al. (2021) conducted research, on how the Big Five personality traits influence technology acceptance. They focused on openness, extraversion, agreeableness, conscientiousness, neuroticism and willingness to take risks. Many of the reviewed studies found evidence linking each personality trait to technology acceptance using the Technology Acceptance Model (TAM) as a measurement framework. Although these studies may not cover all aspects comprehensively they have greatly enhanced our understanding of how the Big Five personality model relates to computer usage.
In a research study, by Paryudi et al. (Citation2021) they set out to create a model that explores how demographic information relates to personality traits. Their approach involved crafting a data model that remains consistent over an individuals lifetime featuring categories within each demographic variable. The model showed accuracy levels. Following criteria the researchers opted for a model that combines age groups and gender as components. This resultant model categorized individuals into six age groups based on gender; males, teenage females, adult males, adult females, aged males and middle aged females. Across all age groups and genders the findings indicated low extroversion levels but high agreeableness and conscientiousness traits were prevalent. Notably it was noted that teenagers of both genders displayed stability compared to other age groups and genders who exhibited higher emotional stability. Furthermore the study unveiled intelligence levels among middle aged males in contrast to other demographic categories with lower intelligence levels observed. Additionally a collaborative research effort between Stanford University and the University of Cambridge sought to assess computers effectiveness, in identifying personality traits compared to individuals friends and family members.
When people made judgments they relied on what they knew about the individual while computers analyzed signals. The research showed that, by looking at a persons Facebook ‘likes’ computers could predict their personality traits accurately than friends and family members. The computers predictions were based on the content the person showed interest in on Facebook, such, as articles, videos and artists. The goal was to see how well the computers predictions matched the individuals personality traits across five dimensions; openness, conscientiousness, extraversion, agreeableness and neuroticism. This study suggests that machines might have an understanding of us than previously believed, showcasing technologys ability to uncover our personality traits (Parker, Citation2015). These studies focus on exploring the modeling of data and personality traits relationship and examining computers accuracy in evaluating our personalities. Are limited in their scope beyond theoretical and analytical significance.
A recent research study, by Saleh & AlAli (Citation2022) aimed to explore how personality traits, characteristics and technological skills relate to each other. The study involved using the five factor personality scale to evaluate competence in a group of preparatory year students at the University of King Faisal. The results revealed a connection between students personality traits, their dimensions and their technological skills. Specifically extraversion openness to experiences, agreeableness and conscientiousness were identified as factors influencing students shared abilities. Moreover the study demonstrated that it was possible to predict personality traits based on students common skills indicating a strong link, between individual personality traits and technological proficiency. Upon reviewing this study it becomes evident that it has produced results. Notably it underscores the importance of considering personality traits when predicting an individuals tech capabilities. However one potential drawback of the research is the use of tools to measure psycho computing adaptability; while psychological aspects were assessed using the five factor model of personality computer competencies were evaluated through an examination.
After examining into the literature and prior research it is clear that the main focus has been, on pinpointing the characteristics and aspects of personality and how they impact the use of technology and performance in different tasks. Some studies have also examined into the possibility of identifying personality traits based on behavior. A recent study aimed to correlate technological traits with facets of personality and computer skills by using the five-factor personality scale and assessments of technical proficiency. What sets this study apart is its examination of dimensions of personality traits. Additionally it seeks to understand the patterns displayed by computer users to establish reliable indicators that combine individual traits with their computer related practices. These endeavors are paving the way, for developing a measure of psycho computer characteristics tailored specifically for individuals who use computers including students studying computer science.
4. Theoretical framework
Computer science students need to have a variety of skills to succeed in their field. These include being able to work with amounts of data having expertise in creating intelligence methods being skilled, at developing e learning tools knowing about robotics and industrial automation technologies being able to design voice and image recognition systems excelling in developing virtual and augmented reality applications understanding computer network systems being familiar with cloud computing technologies and having the ability to create web applications (Kim et al., Citation2013).
In a sense personal qualities can play a role in how someone can use computers and technology effectively. Understanding these traits and how they impact technology use in settings, like schools and universities is important. Therefore developing training programs and educational materials that focus on cultivating these traits to improve students proficiency in using technology is essential (Jackson et al., Citation2006).
The realm of Computing Psychology examines into the relationship, between individuals and computers a domain within the field of psychology. Its primary focus lies in exploring how psychological aspects influence computer usage and understanding the impact of technology on behavior thinking processes and social engagements (Groth-Marnat, Citation2003). Psycho computing aims to grasp how people interact with computers and create user interfaces for software, apps and technological resources that cater to their requirements thereby enhancing their user experience (Helander, Citation2014).
The implementation of established standards to aid computer users and students in the realm of computer science has become a venture. Notably there have been endeavors to identify strategies for developing standards that can assess self efficacy and self learning adequately through online platforms. For example Shen et al. (Citation2013) introduced a self efficacy assessment specifically tailored for self learning. Zimmerman & Kulikowich (Citation2016) devised the Online Learning Self Efficacy Scale (OLSES) while AlAli & Saleh (Citation2022) created a measure of self efficacy, for distance learning.
Hence the present direction focuses on creating and formalizing a scale that covers the computational characteristics of individuals using computers and computer science learners. This includes outlining its parameters and different facets recognizing linked cues and confirming its psychological measurement qualities. This research aims to tackle these issues and support progress in this area.
Psycho computing is a blend of psychology and computer science aimed at improving how people interact with devices. It involves studying the social factors that shape technology use creating user programs and websites analyzing user behavior data and developing smart systems that adapt to human actions (Dix et al., Citation2003; Myers, Citation1998). Understanding users needs designing interfaces enhancing interactions, between users and computers and using insights to enhance products are key aspects of psycho computing. By utilizing techniques in this field user experience can be enhanced, leading to increased market value, for products or applications. Designers and developers working on user interfaces should prioritize incorporating psycho computing strategies to optimize outcomes and enhance user satisfaction (Deaton, Citation2003; Pirrelli et al., Citation2020).
This research examines into the Psycho-computing characteristics scale aiming to identify indicators that connect computer user behaviors with personality traits. The scale is built on dimensions as detailed below;
4.1. Cognitive computing
Cognitive computing is an interdisciplinary field that combines philosophy of knowledge, with computing and communication technologies to create systems and tools that assist individuals in analyzing information enhancing communication and improving interaction with their surroundings (Appel et al., Citation2017; Noor, Citation2014). In essence cognitive computing focuses on developing systems for tasks requiring in depth data analysis and understanding as enhancing communication, between humans and computers. Computer technology also allows people to communicate online enabling sharing of knowledge, ideas, comments and notes (Raghavan et al., Citation2016; Raj, Citation2014). This study focuses on computing – the skills and abilities individuals utilize when using a computer – influenced by their mental makeup.These abilities involve utilizing computer technology to save information and tasks for retrieval, in situations using computer technology for cognitive processes like brainstorming ideas and problem solving in various ways and merging human skills with computer capabilities to efficiently and precisely accomplish tasks, in record time.
4.2. Neuroticism computing
Several research studies have examined into the connection between computer technology and neurotic personality traits. While a direct correlation remains unconfirmed, some findings suggest that individuals with higher levels of neuroticism might be more susceptible to the negative impacts of technology use. For example, one study revealed that those with elevated neuroticism levels reported increased feelings of anxiety and stress when engaging with technology, leading to heightened negative emotions while using social media. Another study indicated that individuals with higher neuroticism tendencies often turn to technology as a coping mechanism for dealing with negative emotions (Chen et al., Citation2022; Ko et al., Citation2012; Meier et al., Citation2016).
On the flip side, certain research has proposed that utilizing technology can actually benefit the mental well being of individuals exhibiting high levels of neuroticism. A study highlighted that online social support had a notably positive influence on the mental health of those with elevated neurotic traits compared to their less neurotic counterparts (Ragu-Nathan et al., Citation2008; So & Mak, Citation2019).
This investigation focuses on exploring how neuroticism intersects with computing practices as a personality trait characterized by a propensity towards negative emotions, instability and limited resilience in everyday technological interactions. Neuroticism can be seen as the opposite of extraversion, showcasing a person’s pessimistic outlook on life, suggesting a lack of confidence in expressing themselves, reduced energy and enthusiasm, a tendency towards feeling down and challenges in connecting with others. For instance, individuals with neurotic tendencies might exhibit behaviors like ignoring others viewpoints and resisting external influences while engaging on social media platforms. They may hold firm to their own beliefs regardless of new information or updates found online.
4.3. Extraversion computing
Extraversion is a personality trait known for being sociable, outgoing and assertive, which might have some connections to how people engage with computer technology. However, it’s important to understand that the link between extraversion and technology use isn’t straightforward and can depend on individual variations and the specific situations where technology is used.
One research study discovered that extroverted individuals tend to use computer mediated communication tools like email and instant messaging more often to stay connected socially and engage in social interactions. On the other hand, introverts lean towards face to face conversations and less mediated forms of communication (Chong & Chen, Citation2016). Another study examined into how computer technology could influence behaviors linked to extraversion. It revealed that people who interacted with strangers through computer mediated communication showed increased levels of extraverted behaviors such as assertiveness and positive emotions compared to those engaging in face to face interactions (Hamburger & Ben-Artzi, Citation2000; Koc, Citation2017).
In this particular research, extraversion computing refers to individuals with an extroverted nature who incorporate their computing habits into social relationships to fulfill various needs.An outgoing individual tends to be spontaneous, enjoys having a good time, is quick to grasp concepts, has a positive outlook, is energetic, mobile and thrives on socializing and engaging with others. Instances of digital habits for an extroverted character involve leveraging intuitive and implicit knowledge when navigating communication networks, engaging thoughtfully with concepts and discussions on various communication channels and maintaining a hopeful attitude while being motivated to put in more effort and activity to address challenges and accomplish objectives with the help of technology.
4.4. Openness computing
Openness is considered one of the key personality traits among the ‘Big Five’ that are used to explain individual differences in character. It signifies a person’s capacity for imagination, creativity and eagerness to explore new things. People with high levels of openness often show a broad range of interests, a passion for acquiring knowledge and a fondness for diversity and innovation.
Research indicates that individuals with high openness scores generally hold a positive view towards technology and are more inclined to embrace new technological advancements compared to those scoring lower on the openness scale. They also tend to see technology as a means of fostering creativity, learning and self expression (Correa et al., Citation2010). This inclination might stem from the fact that technology offers fresh avenues for examining into and expressing creative thoughts (Wang & Jackson, Citation2019). Furthermore, individuals with higher levels of openness are more likely to participate in online activities involving creativity such as blogging, content creation or engaging in virtual communities (Gosling et al., Citation2011).
Within this context, openness computing refers to an individual trait characterized by curiosity and an enthusiasm for learning about both internal and external worlds. Individuals exhibiting this trait tend to have rich experiences in various aspects of life.
Practices linked to open computing involve eagerly welcoming and looking forward to different technological advancements and changes, along with finding fulfillment in various digital content and products like texts, movies and digital elements that cater to one’s curiosity and thirst for knowledge.
4.5. Dynamic computing
The interconnectedness of dynamic computing and motivation plays a significant role in how we engage with technology in both our professional and personal lives. By enhancing dynamic computing, offering technical assistance and improving usability and reliability, individuals are more likely to feel motivated to continue utilizing technology in their daily routines. Conversely, encountering negative technological experiences can deter individuals from relying on or using technology in their work and daily activities. Therefore, it is essential to provide adequate training and technical support to optimize technology usage, enhance user satisfaction and foster continued motivation for its use (Lee & Lee, Citation2004).
In this research, dynamic computing refers to an individual’s computational behaviors and habits that align with their motives and are assessed based on physical abilities, skills or necessities. Examples of behaviors linked with dynamic computing encompass efforts to comprehend the prerequisites for learning before and after sessions through online platforms for distance education to cultivate positive expectations for success. Additionally, leveraging educational opportunities within learning networks can bolster competitiveness and increase the likelihood of achieving success.
4.6. Mastery computing
People who excel in mastering computer technology tend to see it as a tool that can be harnessed to reach their goals. They are likely more confident in their ability to pick up new technical skills and are open to experimenting with new software or hardware. On the other hand, those with lower mastery proficiency might hesitate to embrace new tech or feel daunted by the complexities of modern computing (Huang et al., Citation2021).
Overall, it appears that individuals scoring higher on the mastery trait are more inclined to embrace and make use of computer technology for accomplishing their objectives (Mehta & Chopra, Citation2020). In this research, mastery computing refers to a person’s computer habits characterized by keen attention, avoidance of indifference, commitment ability, relentless pursuit of goals and willingness to invest effort for personal gain. Examples of practices linked with mastery computing include feeling a sense of control over the learning process via learning networks and leveraging immediate and delayed feedback for accurate responses while correcting mistakes through learning networks.
5. Methodology
This study employed a method called quantitative descriptive survey, which is a systematic scientific approach used to explore and understand a specific phenomenon or issue by collecting organizing and analyzing standardized data. This type of research is known for its ability to produce highly accurate results as it involves a large sample size (Saleh et al., Citation2023). In order to assess technical behaviors that reflect personality traits, the researchers created a Psycho computing scale with six dimensions; cognitive computing, extraversion computing, neuroticism computing, openness computing, dynamic computing and mastery computing.
5.1. Population and sampling
The current study was carried out during the 2022/2023 academic year at selected universities in Saudi Arabia. Five Saudi Universities distributed among five regions in the Kingdom were purposively chosen due to the possibility of obtaining a representative sample from them. The initial stage of constructing the scale involved a pilot sample of approximately 150 male and female students from Saudi universities. Subsequently, the study sample comprised 301 male and 421 female students from diverse scientific and humanities faculties, were chosen randomly, with an age range of 19-23 years.
5.2. Procedures
After coming up with the initial research idea, a deep dive into relevant scientific literature was carried out to gather a thorough understanding of the research topic. Following this, a study tool was created and underwent a rigorous evaluation process. To ensure the trustworthiness, accuracy and consistency of the study tool, all necessary approvals were secured, including ethical clearance from the Scientific Research Ethics Committee at King Faisal University. Furthermore, the required permissions for execution were obtained (Reference Number; KFU REC 2022 DEC ETHICS420). Once all approvals were in order, the data collection phase began following established protocols. Purposeful sampling was employed to choose appropriate universities for the study, from which a random sample was selected. The developed study tool was then distributed among the chosen participants and later collected from them. After completing the data collection phase, the gathered data was diligently entered into a computer database. Statistical software was used to analyze the data, ensuring appropriate analytical techniques were applied. The data analysis process was carried out carefully to extract valuable insights and make accurate conclusions based on the collected information.
5.3. The scale
The researchers conducted a literature review and analyzed personality trait scales to identify the main dimensions for the psycho-computing traitsscale. They then examined individuals’ computer competencies and practices to create indicators that blend behavioral practices with associated personality traits. The resulting scale consists of six dimensions. The construction and refinement procedures for the psycho-computing properties scale are presented in .
Table 1. The proposed perception to construct a scale of psycho-computing characteristics.
presents the step-by-step process involved in constructing and developing the psycho-computing traitsscale, which are as follows:
Establishing the primary objective of the scale, which is to assess an individual’s psycho-computing traitspractices.
Identifying the dimensions of the scale through an extensive review of relevant literature, including books, internet sources, expert opinions, and academic resources. The scale consists of six dimensions: cognitive computing, extraversion computing, neuroticism computing, openness computing, dynamic computing, and mastery computing.
Formulating items for the scale based on previous research studies, the researchers’ experiences, and input from faculty members. The dimensional scale comprises a total of 58 items.
Developing a five-point response scale for each item, ranging from ‘completely applicable’ to ‘not completely applicable’.
The study ensured the validity of the scale through a rigorous process. Firstly, eleven experts from various Saudi universities examined the scale items and provided their feedback. The researchers used this feedback to modify items that contained ambiguous or unclear wording, correct grammatical errors, and eliminate items that were inappropriate or duplicated based on the opinion of at least 30% of the experts, as presented in Appendix A. To further enhance the validity and reliability of the scale, the researchers piloted it with 150 undergraduate students and incorporated their responses and feedback into the final version. For evaluating the construct validity of the scale, the researchers employed the Rasch model analysis, a powerful tool for objective assessment of psychological and educational measurement, by using Winsteps software version 3.68.2. The use of the Rasch model analysis ensured that the scale was well-suited to measuring the intended construct in an objective manner (AlAli & Al-Barakat, Citation2022).
To understand the results of the Rasch model measurement analysis, certain aspects must be considered. Firstly, the degree of fit of an item or a person is known as infit, and is measured by the square transformation of the residuals (the difference between the predicted and observed values). MNSQ values for infit that range from 0.4 to 1.5 and standardized fit statistic (Zstd) values between -2 and 2 are considered appropriate. Two items were omitted in each of the first, third, and sixth dimensions, One item was omitted in each of the second and fourth dimensions, and Four items were omitted in the fifth dimension, because its MNSQ value of infit and outfit was greater than 1.5, and the Zstd value was not within an acceptable range as shown in Appendix B (AlAli & Abunasser, Citation2022).
Secondly, item polarity analysis (point measure correlation PTMEA) or consistency of the items is important in understanding whether the items move in the same direction as the constructs being measured. Negative indicators suggest that some items or individuals respond differently than the construct, and data should be re-examined to improve or remove the problematic items. If the PTMEA value ranges between 0.2 and 1. Four items were not appropriate, and the rest of the items were appropriate as shown in Appendix B.
Thirdly, dimensionality refers to whether the instrument is measured in only one direction and one dimension, and is essential in confirming the instrument’s content and construct validity. The dimensionality criterion should be more than 40%, and the unexplained variance in the first contrast should be less than 15. Hence, dimensionality data result was inappropriate to Rasch model as shown in Appendix C (AlAli & Saleh, Citation2022).
Fourthly, item separation refers to the ability of all participants to answer items of varying degrees of difficulty. Higher separation values suggest more diversity among persons on the trait, while lower separation values suggest item repetition and less diversity among persons. The reliability analysis was conducted with 70 items for the Psycho-computing scale among 150 students, and the criterion for accepting reliability in the Rasch model is that it exceeds 0.50. Acceptable separation should be more than 2. The results of the reliability analysis show that the person reliability was very high at a value of 0.94, the person separation was 3.94, the item reliability was 0.91, and the item separation was 3.15, all of which are acceptable. Details of the data analysis and results for each aspect are presented in Appendix D (Saleh & AlAli, Citation2022). The ultimate version of the scale included a total of 58 items and was administered to a sample of 722 students in the study.
5.4. Data analysis
In order to validate the construct of the study, several indicators were examined, including Macdonald’s Omega and Composite Reliability, as well as convergent and discriminant validity. To achieve this, Rasch model analysis was conducted using Winsteps software version 3.68.2. This method enabled the estimation of individual ability, as well as the difficulty and discrimination coefficients for each item. Furthermore, the model generated a statistical coefficient to assess the accuracy of ability estimation for each individual. Confirmatory factor analysis (CFA) were also utilized to evaluate factor validity, using SPSS version 26, and Amos statistical software version 25. CFA is a type of structural equation modeling (SEM) that examines patterns in data and is used to assess construct validity, categorize method impacts, and test the latent structure of a test instrument during its development process. It is also an essential analytical tool for other aspects of psychometric evaluation.
6. Results
Our research aimed to answer the question of what the procedures are for developing a Psycho-computing scale and verifying its psychometric properties in Saudi environments. To achieve this, we followed specific steps in developing the scale and used various methods to verify its construct validity.
6.1. Indicators and coefficients for establishing construct validity: a comprehensive overview
The reliability of questionnaires is often determined using Macdonald’s Omega and Composite Reliability (CR). The results presented in reveal that the values of Macdonald’s Omega and CR fall within the range of 0.873-0.926 and 0.870-0.926, respectively, which align with the recommended values (>0.7), signifying that the scale possesses substantial internal consistency. Moreover, the values of Average Variance Extracted (AVE) range between 0.504-0.595, which is greater than 50%. Concerning the discriminant validity coefficients (square root of the AVE or convergent validity and its coefficients), they must exceed the intercorrelations between the latent factors or factors. By comparing the last column, we observe that it is greater than the minimum value of the loading factor. Therefore, these findings demonstrate that the scale is both reliable and valid (AlAli & Saleh, Citation2022).
Table 2. Some indicators and coefficients of construct validity.
6.2. Assessing construct validity using the rasch model
To establish the validity and reliability of the Psycho-computing scale, the following procedures were conducted. The validity of the Psycho-computing scale was assessed using MNSQ values for the infit. The results indicated that the scale demonstrated an appropriate degree of validity. The MNSQ values for the scale fell within the acceptable range of 0.4 to 1.5. Additionally, the item polarity analysis based on PTMEA values was consistent, with values ranging from 0.2 to 1. The scale also demonstrated a suitable standardized fit statistic (Zstd) value, falling within the recommended range of -2 to 2, as presented in below.
Table 3. Item Fit Analysis for Psycho-computing scale.
Moreover, the Psycho-computing scale was found to be consistent with calibration measurement analysis. The results were consistent with the dimensionality analysis, as more than 40% of the raw variance was explained by the measures, and the unexplained variance in the 1st contrast was less than 15%. Hence, the dimensionality data results were found to be appropriate according to the Rasch model, as presented in .
Table 4. Item dimensionality of Psycho-computing scale.
The reliability of the Psycho-computing scale was assessed using person reliability, which measures the degree of reliability of individuals responding to the Psycho-computing scale, as presented in . Additionally, item reliability was calculated to indicate the reliability of the scale’s items. The study’s findings demonstrated that the scale’s items exhibited an appropriate degree of reliability, as illustrated in .
Table 5. Person and Item separation and reliability for Psycho-computing scale.
6.3. Assessment of construct validity through factor analysis
To test the construct validity of the scale, exploratory factor analysis (EFA) and confirmatory factor analysis (CFA) were conducted using SPSS and Amos statistical software.
6.3.1. Exploratory factor analysis (EFA)
Principal components analysis was utilized to conduct exploratory factor analysis on the responses obtained from the final scale, which consisted of 58 items. An oblique rotation, specifically the Promax method, was performed on the extracted factors that had eigenvalues greater than one as illustrated in .
Table 6. The results of the exploratory factor analysis using Promax method.
illustrates the presence of six factors with eigenvalues greater than 1, accounting for an explanatory variance ratio of 98.134%. The eigenvalue of the first dimension was 3.554, with an explanatory variance ratio of 59.238.
The development of the scale required knowledge of the loading values of the scale items on their respective dimensions in the exploratory factor analysis, as illustrated in . The criterion for item adoption was that loading factors should not be less than 0.40 [59]. indicates that the loading factors of the items exceeded 0.40 within their respective dimensions. Consequently, the scale retained its six dimensions and item composition, comprising the first dimension (10 items), the second dimension (10 items), the third dimension (10 items), the fourth dimension (10 items), the fifth dimension (8 items), and the sixth dimension (10 items).
6.3.2. Confirmatory factor analysis (CFA)
Confirmatory factor analysis was employed to establish the factorial construct validity of the scale, the final version of the scale was administered to the study sample to conduct confirmatory factor analysis of the scale items within their respective dimensions. The adopted model depicted the relationship of the scale’s 58 items distributed over six dimensions, as illustrated in .
displays the loading degree of each item in its respective dimension. The findings revealed that each item achieved a high degree of loading in its dimension. Furthermore, the results indicated a strong correlation among the dimensions of the scale. presents the indicators of the internal construct validity, which demonstrate the validity of the scale items’ internal construction. This was conducted to confirm the results of the confirmatory factor analysis of the adopted model for the relationship between the scale items and their dimensions. The table also demonstrates that the model aligns with the relationship between the scale items and the data, confirming that all indicators meet the study’s criteria. These findings suggest the stability of the model for the relationships among the scale items.
Table 7. Confirmatory factor analysis outcomes regarding the adopted model’s fit to the relationship between scale items and their respective dimensions.
7. Discussion
The Psychology of Computing field focuses on studying the interaction between humans and computers. It is crucial to establish concrete indicators that combine computer usage proficiency with the associated personality traits of learners. Recognizing this urgency, the present research aimed to construct and develop a scale called the psycho-computing traitsscale and assess its psychometric properties. Through an extensive review and analysis of existing literature, this study identified six primary dimensions that formed the basis of the measure for assessing the psycho-computing traitsof computer users and computer science students. These dimensions include cognitive computing, neurotic computing, extroverted computing, dynamic computing, and mastery computing.
Consequently, the first research question, which inquired about the main dimensions underlying the scale of psycho-computing characteristics, was successfully answered. The development process of the psycho-computing traitsscale involved several steps. Initially, the purpose of the scale was defined, followed by the identification of the six dimensions. Next, a group of experts from Arab universities reviewed and refined the scale, eliminating any inappropriate items. Subsequently, the scale was administered to an exploratory sample, and procedures were conducted to establish its validity and reliability. As a result, a proposed conceptualization of the scale was achieved, addressing the second research question regarding the development and construction of a measure for assessing the psycho-computing traitsof computer users and computer science students.
The findings indicate that the Psycho-computing Characteristics Scale exhibits appropriate psychometric properties in relation to several scales. Specifically, the results of indicators such as McDonald’s Omega and Composite Reliability demonstrate that the scale possesses strong internal consistency. Additionally, the values of Average Variance Extracted (AVE) in relation to coefficients of discriminatory validity and the square root of AVE pertaining to convergent validity suggest that the measure is both reliable and valid. Furthermore, the indicators of construct validity also demonstrate that the Psycho-computing Characteristics Scale exhibits sound psychometric properties, as evidenced by the results of the Rasch model assumptions for the data obtained through administration of the scale. Additionally, the results of the confirmatory factor analysis demonstrated a high level of agreement between the indicators and the main dimensions of the scale. These outcomes signify that the measure possesses global validity and is well-suited to the collected data. This outcome can be attributed, in part, to the nature of the study sample, which consisted of undergraduate students known for their level of awareness, ability to engage with the scale items, and responsiveness. Furthermore, the clear formulation of the scale’s vocabulary elements and their correlation with the computer practices of the learners contributed to the positive outcomes. Based on these results, it is feasible to rely on the proposed scale for evaluating the psycho-computing traitsof university-level students.
The findings suggest that the psycho-computing traitsscale exhibits high reliability, which is consistent with the results of the Rasch model for both person and item reliability. This reliability can be attributed to the consistency among the items in measuring the intended trait among students, as well as the students’ diligence in providing accurate responses. Moreover, the scale demonstrates comprehensiveness and coherence across its various dimensions, as evidenced by the strong correlation coefficients between them. The results further confirm that there is a correlation between the items of the scale and their respective dimensions, thereby enhancing the accuracy of measurement and facilitating the assessment of individuals’ compatibility with the requirements of educational and practical settings. Consequently, the study successfully addressed the third research question, which pertained to the procedures involved in developing a scale of psycho-computing traitsfor computer users and computer science students, as well as verifying its psychometric properties.
The present study aimed to develop a version of a psycho-computing traitsscale, which is founded on observable indicators of learners’ computer use behavior that integrate fundamental computer proficiency skills and associated personality traits. The results of this study are consistent with the work of AlAli & Saleh (Citation2022), which advocated for the use of standardized scales to assess the efficacy of computer technology utilization in distance learning. While the present study shares the same objective as Saleh & Al-Ali (Citation2023) research, which aimed to explore the psycho-technological compatibility between personality traits and technological competencies by applying the Five-Factor Model of personality and evaluating technological competencies, it differs in that it proposes a novel model for assessing psycho-computing traitsbased on observable and measurable computer indicators and practices that combine computer use efficiency with its associated personality traits.
8. Limitations and future directions
The utilization of the Psych-computing Characteristics Scale has thus far been confined to undergraduate students, limiting its current applicability to this specific educational stage. However, there exists the potential to extend the application of this scale to other age groups or pre-university levels, provided that the scale’s components are adapted and simplified appropriately for the targeted age group. Furthermore, it is noteworthy that the scale’s application has been primarily limited to Arab environments. Considering the variability in computer use competencies and the associated personal traits across different environments and cultures, it would be beneficial to broaden the scale’s application to encompass diverse settings beyond the Kingdom of Saudi Arabia. Such an expansion would enhance the scale’s reliability and validity by encompassing a wider range of computer psychological measurements.
Future research endeavors in this domain could encompass the development of training programs centered around diverse computer applications to enhance positive practices and address negative practices among users. Additionally, the creation of programs based on personal traits could be explored to enhance students’ technological skills. Furthermore, investigating the impact of genetic factors on computer use efficiency and devising effective solutions to enhance computer performance among individuals affected by genetic diseases that impede their computing abilities would be of great value. Lastly, studying the influence of personality traits such as introversion and shyness on computer efficiency and developing efficacious strategies to enhance the computer efficiency of individuals with these traits would also be worth investigating.
9. Conclusion
Through an extensive review of pertinent literature and studies investigating the multifaceted impacts of human-computer interaction processes, this research endeavor was undertaken with the objective of creating a comprehensive scale to measure computer psychological characteristics. By critically examining philosophical frameworks and approaches in this domain, six fundamental dimensions of behavioral practices among computer users and computer science students were identified. Leveraging these dimensions as a framework, a scale encompassing psycho-computing traitswas meticulously developed. Rigorous measures were employed to ensure the scale’s psychometric properties, including assessing its construct validity using the Rasch model, as well as exploring its factor validity through both exploratory factor analysis (EFA) and confirmatory factor analysis (CFA). The results demonstrated a high level of reliability and validity for the scale, thus successfully establishing a robust and valid instrument for evaluating psycho-computing characteristics.
Authors’ contributions
S.S. contributed to building the theoretical framework, methodology and interpretation of the results, and R.A. contributed to building tools, statistical analysis and re-view. All authors have read and agreed to the published version of the manuscript.
Acknowledgments
The authors thank the Deanship of Scientific Research at King Faisal University, Saudi Arabia for the financial support under Annual research grant number GRANT3645.
Disclosure statement
This manuscript has not been published or presented elsewhere, in part or in entirety, and is not under consideration by another journal. There are no conflicts of interest to declare. On behalf of all authors, the corresponding author states that there are no conflicts of interest.
Data availability statement
The authors declare that all other data supporting the findings of this study are available within the article and its supplementary information files. Informed consent was obtained from all individual participants included in the study.
Additional information
Funding
Notes on contributors
Shoeb Saleh
Shoeb Saleh Supervisor of the Development Department at the National Center for Giftedness and Creativity Research - King Faisal University in the Kingdom of Saudi Arabia. Assistant Professor of Educational Technology, Faculty of Education, Sohag University, Egypt. My research interests: Educational technology and its cognitive theories - evaluating distance learning systems in light of some variables - organizing and managing knowledge in distance learning environments - preparing codified scientific standards in the field of educational computers. Evaluating the various learning outcomes of gifted programs - ways to develop digital support for gifted students.
Rommel AlAli
Rommel AlAli Assistant Professor of Measurement and Evaluation. Supervisor of the Scientific Research Department at the National Center for Giftedness and Creativity Research - King Faisal University in the Kingdom of Saudi Arabia. My research interests: Constructing and developing scales and tests, evaluating educational programs and learning outcomes, modern theory of measurement, psychometric properties of scales, tests, questionnaires and all tools.
References
- Ackerman, P. L., Kanfer, R., & Beier, M. E. (2013). Trait complex, cognitive ability, and domain knowledge predictors of baccalaureate success, STEM persistence, and gender differences. Journal of Educational Psychology, 105(3), 1–28. https://doi.org/10.1037/a0032338
- AlAli, R., & Abunasser, F. (2022). Can the leadership capabilities of gifted students be measured? Constructing a scale according to Rasch Model. Educational Administration: Theory and Practice, 28(03), 109–126.
- AlAli, R., & Al-Barakat, A. (2022). Using structural equation modeling to assess a model for measuring creative teaching perceptions and practices in higher education. Education Sciences, 12(10), 690. https://doi.org/10.3390/educsci12100690
- AlAli, R., & Saleh, S. (2022). Towards constructing and developing a self-efficacy scale for distance learning and verifying the psychometric properties. Sustainability, 14(20), 13212. https://doi.org/10.3390/su142013212
- Al-Hila, M. (2001). Educational and information technology. Al-Ain, University Book House.
- Antoncic, B. (2009). The entrepreneur’s general personality traits and technological developments. World Academy of Science, Engineering and Technology, 53(3), 236–241.
- Appel, A. P., Candello, H., & Gandour, F. L. (2017). Cognitive computing: Where big data is driving us. Handbook of big data technologies (pp. 807–850). Springer International Publishing AG. https://doi.org/10.1007/978-3-319-49340-4_24
- Barroso, A. S., Da Silva, J. S. M., Souza, T. D., Cezario, B. S. D. A., Soares, M. S., & Do Nascimento, R. P. (2017). Relationship between personality traits and software quality-big five model vs. object-oriented software metrics [Paper presentation]. Iceis, In (3), 63-74. https://doi.org/10.5220/0006292800630074
- Chen, W., Wang, X., Sun, S., Liu, Q., & Guo, Z. (2022). The relationship between neuroticism and mobile phone use among college students in love: The masking effect of self-emotional assessment. Frontiers in Psychology, 13, 942520. https://doi.org/10.3389/fpsyg.2022.942520
- Chong, E., & Chen, G. (2016). Personality traits and computer-mediated communication: A meta-analysis. Computers in Human Behavior, 63, 657–666.
- Correa, T., Hinsley, A. W., & de Zúñiga, H. G. (2010). Who interacts on the Web? The intersection of users’ personality and social media use. Computers in Human Behavior, 26(2), 247–253. https://doi.org/10.1016/j.chb.2009.09.003
- Daempfle, P. A. (2003). An analysis of the high attrition rates among first year college science, math, and engineering majors. Journal of College Student Retention: Research, Theory & Practice, 5(1), 37–52. https://doi.org/10.2190/DWQT-TYA4-T20W-RCWH
- Deaton, M. (2003). The elements of user experience: user-centered design for the Web. Interactions, 10(5), 49–51. https://doi.org/10.1145/889692.889709
- Demkanin, P. (2008). One small, but significant step in science education in Slovakia. In Effective Use of ICT in Science Education (pp. 120–140). University of Edinburgh.
- Dix, A., Finlay, J., Abowd, G. D., & Beale, R. (2003). Human-computer interaction. Pearson Education.
- Durak, H. Y., Saritepeci, M., & Dunya, B. A. (2021). Examining the relationship between computational thinking, lifelong learning competencies and personality traits using path analysis. Bartın University Journal of Faculty of Education, 10(2), 281–292. https://doi.org/10.14686/buefad.888374
- Geisinger, B., Raman, D. R., & Raman, D. (2013). Why they leave: Understanding student attrition from engineering majors.
- Gosling, S. D., Augustine, A. A., Vazire, S., Holtzman, N., & Gaddis, S. (2011). Manifestations of personality in online social networks: A self-report study. Personality and Social Psychology Bulletin, 37(4), 514–528.
- Groth-Marnat, G. (2003). Handbook of psychological assessment. John Wiley & Sons.
- Hamburger, Y. A., & Ben-Artzi, E. (2000). The relationship between extraversion and neuroticism and the different uses of the Internet. Computers in Human Behavior, 16(4), 441–449. https://doi.org/10.1016/S0747-5632(00)00017-0
- Helander, M. G. (Ed.). (2014). Handbook of human-computer interaction. Elsevier.
- Huang, K., Li, J., Hu, W., & Luo, Y. (2021). Exploring the impact of extraversion on technology acceptance: A comparison of Chinese and US samples. Journal of Business Research, 134, 139–152. https://doi.org/10.1016/j.jbusres.2021.01.047
- Jackson, L. A., von Eye, A., Biocca, F. A., Barbatsis, G., Zhao, Y., & Fitzgerald, H. E. (2006). Personality, cognitive style, demographic characteristics and internet use: Findings from the HomeNetToo project. CyberPsychology & Behavior, 9(5), 487–499.
- Kim, D. J., Benbasat, I., & Cenfetelli, R. T. (2013). An integrative framework of technology acceptance: A systematic review and meta-analysis. Information Systems Research, 24(4), 1043–1069.
- Ko, C. H., Yen, J. Y., Yen, C. F., Chen, C. S., & Chen, C. C. (2012). The association between internet addiction and psychiatric disorder: A review of the literature. European Psychiatry: The Journal of the Association of European Psychiatrists, 27(1), 1–8. https://doi.org/10.1016/j.eurpsy.2010.04.011
- Koc, M. (2017). Computer-mediated communication, perceived social support, and personality: A meta-analysis. Computers in Human Behavior, 70, 535–547.
- Lee, J. M., & Lee, J. H. (2004). Approximate dynamic programming strategies and their applicability for process control: A review and future directions. International Journal of Control, Automation, and Systems, 2, (3), 263–278.
- Lury, C. (2002). Cultural rights: Technology, legality and Personality. Routedge. https://doi.org/10.4324/9780203422892
- Mehta, R., & Chopra, A. (2020). The role of mastery in technology acceptance and usage. Journal of Computer Information Systems, 60(1), 23–33. https://doi.org/10.1080/08874417.2018.1550252
- Meier, A., Reinecke, L., & Meltzer, C. E. (2016). “Facebocrastination”? Predictors of using Facebook for procrastination and its effects on students’ well-being. Computers in Human Behavior, 64, 65–76. https://doi.org/10.1016/j.chb.2016.06.035
- Myers, B. A. (1998). A brief history of human-computer interaction technology. Interactions, 5(2), 44–54. https://doi.org/10.1145/274430.274436
- Noor, A. K. (2014). Potential of cognitive computing and cognitive systems. Open Engineering, 5(1), 75–88. https://doi.org/10.1515/eng-2015-0008
- Parker, B. C. (2015). New Stanford research finds computers are better judges of personality than friends and family. https://news.stanford.edu/2015/01/12/personality-computer-knows-011215/
- Paryudi, I., Ashari, A., & Mustofa, K. (2021). Modelling relationship between demographic data and personality traits. Turkish Journal of Computer and Mathematics Education (TURCOMAT), 12(14), 2247–2255.
- Paura, L., & Arhipova, I. (2016). Student dropout rate in engineering education study program. Eng. Rural Dev, 2016, 641–646.
- Pirrelli, V., Marzi, C., Ferro, M., Cardillo, F. A., Baayen, H. R., & Milin, P. (2020). Psycho-computational modelling of the mental lexicon. Word Knowledge and Word Usage, 337, 23–82. https://doi.org/10.1515/9783110440577-002
- Raghavan, V. V., Gudivada, V. N., Govindaraju, V., & Rao, C. R. (2016). Cognitive computing: Theory and applications. Elsevier.
- Ragu-Nathan, T. S., Tarafdar, M., Ragu-Nathan, B. S., & Tu, Q. (2008). The consequences of technostress for end users in organizations: Conceptual development and empirical validation. Information Systems Research, 19(4), 417–433. https://doi.org/10.1287/isre.1070.0165
- Raj, P. (Ed.). (2014). Handbook of research on cloud infrastructures for big data analytics. IGI Global. https://doi.org/10.4018/978-1-4666-5864-6
- Saleh, S., & AlAli, R. (2022). Digital learning tools (Institutional-Open) and their relationship to educational self-effectiveness and achievement in online learning environments. Social Space, 22(3), 226–256.
- Saleh, S., & Al-Ali, R. (2023). Psycho-technological compatibility as one of the guidelines for students of computer science. Information Sciences Letters, 12(5), 1891–1909. https://doi.org/10.18576/isl/120532
- Saleh, S., AlAli, R., Wardat, Y., Al-Qahtani, M., Soliman, Y., & Helali, M. (2023). Structural relationships between learning emotion and knowledge organization and management processes in distance learning environments: “An applied study”. European Journal of Investigation in Health, Psychology and Education, 13(9), 1569–1589. https://doi.org/10.3390/ejihpe13090114
- Seibert, D., Godulla, A., & Wolf, C. (2021). Understanding how personality affects the acceptance of technology: A literature review. Media and Communication, 1–24.
- Shen, D., Cho, M. H., Tsai, C. L., & Marra, R. (2013). Unpacking online learning experiences: Online learning self-efficacy and learning satisfaction. The Internet and Higher Education, 19, 10–17. https://doi.org/10.1016/j.iheduc.2013.04.001
- So, R. H. Y., & Mak, W. W. S. (2019). Online social support: The interplay between social support and neuroticism in relation to depression, anxiety, and stress. Journal of Health Psychology, 24(10), 1413–1423. https://doi.org/10.1177/1359105316687631
- Tsingilis, N. (2019). Personality traits and excessive computer and internet usage. International Journal of Social Science Research, 7, 1–1. https://doi.org/10.5296/ijssr.v7i2.14676
- Turel, O., & Serenko, A. (2012). The benefits and dangers of enjoyment with social networking websites. European Journal of Information Systems, 21(5), 512–528. https://doi.org/10.1057/ejis.2012.16
- Wang, X., & Jackson, L. A. (2019). Exploring the relationships between personality, media preferences, and media effects. Personality and Individual Differences, 138, 36–41.
- Zimmerman, W. A., & Kulikowich, J. M. (2016). Online learning self-efficacy in students with and without online learning experience. American Journal of Distance Education, 30(3), 180–191. https://doi.org/10.1080/08923647.2016.1193801
Appendix A
Table A. Number of items omitted in the psycho-computing traits scale based on experts.
Appendix B
Table B. Item fit analysis for psycho-computing traits scale (initial).
Appendix C
Table C. Item dimensionality of psycho-computing traits scale.
Appendix D
Table D. Person and item separation and reliability for psycho-computing traits scale.
Appendix E
Table E. The psycho-computing traits scale.