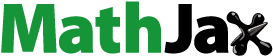
Abstract
Road infrastructure is used by various types of vehicles among which heavy vehicles imposes the most critical loading, causing damage in pavement structure, which ultimately leads to an increased maintenance and rehabilitation costs. During the design of road pavements, each type of vehicle is converted into equivalent standard axle load (ESAL) to consider their impact on road structure. This research study was conducted to quantify the number of overloaded vehicles from the data collected on a critical national highway and to compute the impact of overloaded axle loads on road pavement in terms of ESALs. The variation of truck factor with asphalt layer thickness and subgrade modulus was also studied. The truck factor was calculated according to modified French Pavement Design Guide equation. It was found that the impact of axle configuration on the pavement is significant, i.e. truck factor for 2-axle vehicles was almost 3.33 times the 3-axle vehicles and 5.45 times the 6-axle semi-trailers. It was also observed that the truck factor reduced by almost 47% with a twofold increase in asphalt layer thickness. The subgrade modulus had a very minimal effect on the truck factor.
PUBLIC INTEREST STATEMENT
Vehicle overloading is a major cause of pavement deterioration in developing countries. It reduces the pavement design life and causes an increased cost of maintenance and rehabilitation. This article aims at quantifying the number of overloaded vehicles and their impact on road structure in terms of equivalent standard axle loads (ESALs), which is used for determining the pavement thickness during the design of road. A modified ESALs equation was used to incorporate the effect of number of axles and their configuration on the resulting ESALs value. It was found that the impact of axle configuration on the pavement is significant, i.e. truck factor for 2-axle vehicles was almost 3.33 times the 3-axle vehicles and 5.45 times the 6-axle semi-trailers. It was also observed that the truck factor reduced by almost 47% with a twofold increase in asphalt layer thickness. Based on the results obtained, it is advised to the government agencies to encourage the use of semi-trailers and restrict the axle loads to legal loads as set by the national highways authority.
1. Introduction
A road structure is subjected to various types of loading during its life. These include traffic load and environmental actions producing stresses and strains in road structure. Traffic loads include various types of vehicles movement ranging from small vehicle loads such as those of motorbikes and motor cars to heavy vehicles such as trucks and multi-axle trailers. The response of pavement structure to these loads depends on the stiffness of subgrade, type of pavement, pavement thickness and type of traffic the pavement carries.
It is the overloaded vehicles which cause more damage to the pavement. The magnitude of damage depends on axle configuration (single, tandem or tridem), number of axles in the vehicle (large number of axles ensures distribution of axles load over a large length of pavement), suspension system, tire pressure and the magnitude of overload. The damaging effects of overloaded axles on a pavement include fatigue, which reduces the design life of a pavement, and rutting, which causes the serviceability problem in the pavement. The latter can be structural or non-structural depending upon the design of different pavement layers, anticipated loads and ground moisture conditions.
The impact of heavy truck loading on the pavement damage was studied by Zaghloul and White (Citation1994), which concluded that heavier loading reduces pavement life and increases cost of maintenance. To study the impact of overloaded axles on road pavement, Timm, Tisdale, and Turochy (Citation2005) devised new model to describe the distribution of axle loads, although it being just a theoretical model. Behiry and Ebraham (Citation2012) studied the effect of overloaded axles on the performance of pavement by computing the tensile strain under the asphalt layer and compressive strain on the subgrade using BISAR software, in which it was found that the tensile and compressive strains increased by increasing the axle loads and decreased with increasing the modulus of asphalt layer. Fatigue damage was found to increase by increasing axle loads and decrease by increasing the modulus of asphalt layer.
Andras Gulyas (Citation2012) studied the axle load pattern in Hungary and found that axle loads and gross vehicle weight (GVW) had increased in Hungary in the past 10 years. About 30 weigh-in-motion (WIM) stations were installed there, back in 1996. The data obtained from those WIM stations was analyzed, which showed that overloading mainly occurred at the second axle of semi-trailers with five axles (1 + 1 + 3), which is basically the driving axle. Hadiwardoyo, Sumabrata, and Berawi (Citation2012) recommended a correction factor for ESAL to consider the additional impact due to overloaded axles that will lead to an increased pavement life and prevent early damage of road pavement. It was also concluded that two axles truck had more damaging effect on road pavement as compared to 3-, 4-, 5- or 6-axle trucks and semi-trailers, the reason being load distribution on axles. Hence, larger the number of axles allow more uniform distribution of load on the pavement, and thus have lesser damaging effects.
Kubo, Paiva, Ferreira, and Larocca (Citation2015) studied the effect of shock absorber on road fatigue by using the well-known quarter car model. The analysis was carried out for new, used and failed shock absorbers and for the first and second axle of a truck. It was found that new shock absorbers had less impact on road pavement. The second axle, which is usually the power axle, had a more damaging effect on the road structure. Oliveira, Paiva, and Ferreira (Citation2016) studied the effect of different axle combinations including single axle–single wheel, single axles with dual wheels, dual and triple tandem axles with dual wheels, for 120%, 135%, 150% and 170% of Brazilian legal axle loads. It was found that axles overload decreases the pavement life through fatigue development in road structure. Since a road structure is designed for a particular number of standard axles [usually expressed in Million Standard Axles (MSA)], the ESALs are higher for overloaded axles, and thus a pavement reaches its design life earlier than it was designed for. Albayati and Saadi (Citation2017) studied the impact of overloaded axles on pavement life for both summer and winter season. It was concluded that the allowable single axle load is 9 tons and 15 tons during summer and winter season, respectively. The current allowable single axle load with dual tires is 13 tons, and this could reduce the pavement life by one-quarter of its designed life.
According to AASHTO (Citation1993), during the design of road pavement, different types of vehicles are converted into ESALs. The current practice involves calculation of ESALs based on mechanistic approach, compared with the previously used empirical approach, which involved the ratio of road life for a standard axle to the actual axle load [Pais, Amorim, and Minhoto (Citation2013)]. Based on axle configuration (single, tandem or tridem), and magnitude of loading on the axles, the conversion of different types of vehicles present in the traffic stream depends upon the truck factor. It is calculated for all axles of a vehicle and the sum of truck factors for all individual axles gives the ESAL for a vehicle.
2. Problem statement & objective
Vehicle overloading is a major issue in Pakistan, which causes premature failure of road pavements. Since no previous work has been done, there is a need to quantify the percentage of overloaded vehicles in the country and its impact on road pavements in terms of ESALs, which is ultimately used in pavement design.
Moreover, this study is an effort to find ESALs for the observed axle load data using the modified ESAL equation presented by Pais et al. (Citation2013). This equation differs from the traditional ESAL equation (AASHTO Citation1993), since it also considers the effect of asphalt layer thickness, subgrade modulus and axle configuration in addition to axle load as well. The resulting truck factor would, therefore be coupled with the above-mentioned variables out of which the axle configuration is most important.
3. WIM data acquisition
For quantification of axle overloads, data obtained from WIM station must include information such as the type of vehicle passing, number of axles in the vehicle and the load carried by each axle of the vehicle. The data was obtained from the WIM station located on National Highway N-5 near Attock, Punjab, installed by National Highways Authority (NHA), as shown in Figure and Figure . This highway is 1,819 km long and is existing since 1913, being one of the most strategic highways of the nation. The WIM station installed on this highway covers heavy traffic moving from the southern terminal of the highway at Karachi to its northern terminal at Torkham, bordering Afghanistan. The data were collected for a period of 3 months, i.e. January to March 2012 and analyzed in this study.
4. Methodology
Figure shows schematic plan of the research study. Data obtained from the WIM station were analyzed for the following parameters:
Percentage of overloaded vehicles.
Percentage of each type of vehicle in traffic stream.
Once the above-mentioned characterization was done, truck factors for each vehicle class were calculated using equation provided by Pais et al. (Citation2013). The model was selected based on similarity between axle configurations that exists between proposed model and study vehicles. Truck factors were calculated for the following conditions:
Legal axle loads.
Average-observed axle loads.
Peak-observed axle loads.
The variation of truck factor with asphalt layer thickness and subgrade modulus for legal axle loads.
5. Analysis of WIM data
Based on axle configuration, there exist different types of heavy vehicles in Pakistan. Table illustrates various classes of vehicles which are the most common among different axle configurations that currently exists in the study region. Class B2 and BH3 are single unit tractors while class S123, S113 and S112 are semi-trailers. The table also illustrates the maximum allowable load for each axle as permitted by NHA. Axle load data from the WIM station was checked against the maximum allowed axle loads for computing percentage of overloaded vehicles. A vehicle is supposed to be declared as overloaded when at least one axle has a load exceeding the maximum allowable load.
Table 1. Vehicle classes and allowed maximum load
A total of 101,585 heavy vehicles passed the WIM station during the data collection period, in which a larger number of class B2 vehicles were observed to pass compared with class S113, as shown in Figure . During this period, 41.4% of the recorded trucks were B2 followed by class BH3 at 34.5% and class S122 with the lowest of 2.24%.
Figure 4. Number and type of vehicles observed on the WIM station during the data collection period.
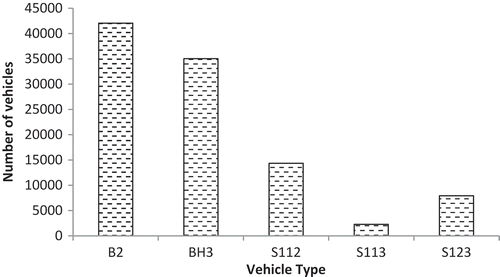
Table illustrates the percentage of overloading for each axle configuration of the observed heavy vehicles. Maximum overloading percentage was observed for class B2 vehicle which is 257.43% greater than the allowed GVW, indicating a huge amount of overloading in this vehicle class. A relatively smaller overloading percentage was observed for class S123, which is almost 200% of the permissible GVW, which still represents a much high amount of overloading.
Table 2. Percentage of overloading based on legal and observed GVW
Figure shows the ratio of average observed loads to maximum loads for each axle of different classes of vehicles. For class B2 vehicle, the difference between first and second axle was found significantly high, while the difference between ratios of different axles for the remaining vehicle classes was relatively lesser. For B2 and S113 vehicle, the average load was found about 56% and 74% of the maximum loads, respectively, while for remaining vehicle classes (i.e. BH3, S112 and S123) the average load was found to be about 80–90% of maximum observed load. Figure shows the comparison of legal GVW with maximum and average observed GVW. For class S112 vehicle, there was a very slight difference between the legal and average observed GVW. Maximum difference was observed between legal and maximum observed GVW for the class S123 vehicle, in which the maximum GVW was 1.7 times the legal GVW. The percentage overloaded vehicles for each class is shown in Figure . Many vehicles of class B2 were found overloaded, i.e. about 34.45% of the total overloaded vehicles. Class S113 vehicles had a very small number of overloaded vehicles, which was only 1.84% of the total overloaded vehicles. This is justified by the small number of S113 vehicles, which were observed in the WIM data. The total number of vehicles which was overloaded during the study period was 78.74%. This means that more than 50% of the heavy vehicles passing the WIM station were overloaded, and might have damaged the pavement, causing early fatigue and thus have ultimately reduced the life of the road structure.
6. Truck factor calculation
Since the traffic stream is composed of various types of vehicles, it is necessary to convert them into ESALs, which is the relationship between the measured axle load and the standard axle load of 80 kN and is given by Equation 1 (Laboratoire Central des Ponts et Chaussées Citation1994).
where;
k = Coefficient that depends on type of axle (single, tandem or tridem).
α = Coefficient that represents the mode of distress (fatigue, rutting).
The French pavement design guide has specified the value of “k”, but it only incorporated the effect of axle configuration; however, Pais et al. (Citation2013), developed an equation for “k” involving thickness of asphalt layer, modulus of subgrade and axle configuration in addition to axle load, as given by Equation 2. This study calculated truck factors using Equation (2). The model was selected based on similarity between axle configurations that exist between proposed model and study vehicle. The use of Equation (2) provides the benefit of evaluating the truck factor while incorporating the effect of axle configuration as well.
where
ESub = Subgrade modulus (MPa).
h = Thickness of asphalt layer (cm).
AP = Axle parameter as defined in Table .
Table 3. Axle parameter (AP) Pais et al. (Citation2013)
Equation 2 is valid for pavements with asphalt layer stiffness modulus of 5000 MPa and a granular layer of 20 cm, because the indices of Esub, h and e were calculated based on these values [Amorim, Pais, Vale, and Minhoto (Citation2014)]. The minimum asphalt layer stiffness modulus and granular thickness are 2500 MPa and 10 cm according to Amorim et al. (Citation2014). For other values of asphalt layer stiffness and granular layer thickness, relationships are provided by Amorim et al. (Citation2014).
According to Pais et al. (Citation2013), a value of 4 for α is representative of pavement failure mode, i.e. fatigue failure. Archilla and Madanat (Citation2000) obtained a value of 3.89 for α for tandem axles and rutting. Prozi and Madanat (Citation2003) using a recursive nonlinear model, obtained a value of 4.2, which is near to 4, as considered in this study. Since, the pavements in study region suffers from fatigue failure, therefore, value of α taken is 4.
It must be kept in mind that each pavement distress has a different α value. The same is true when different pavement distresses occur in combination, and this is the main limitation of the above model used for analysis of truck factor.
7. Results and discussion
Truck factors were computed for the various classes of vehicles as illustrated in Table . These were computed according to the above proposed model for the following scenarios.
Truck factor for legal axle loads.
Truck factor for average observed axle loads.
Truck factor for maximum observed axle loads.
The effect of asphalt layer thickness on truck factor.
The effect of subgrade modulus on truck factor.
Five different asphalt layer thicknesses (i.e. 10 cm, 15 cm, 20 cm, 25 cm, 30 cm, and 35 cm) and four different subgrade moduli (i.e. 20 MPa, 40 MPa, 60 MPa, and 80 MPa) were used to assess their effect on the truck factor.
Figure shows the variation of truck factor for various loading conditions and, for all classes of vehicles. The highest truck factor was observed for class B2 followed by S112. It is justified by the severe axle overloading and the presence of two single axles in the vehicle (showing the effect of AP value). The single axle–single wheel stresses the road pavement on two different areas, while single axle–dual wheels stresses the pavement on four different areas thereby, reducing the intensity of stress. The difference between the truck factor for legal axle load and average axle load for class B2, S112 and S123 was found minimal. The average axle loads observed for BH3 class vehicle were almost 4.5 times the legal axle loads. For class B2, S113 and S123 the average axle loads were 2.1–3.1 times the legal axle loads. Similarly, for class S112, the average axle loads were found to be less than the legal axle loads.
It can be concluded from the above discussion that the axle configuration and axle load are the most important variables while calculating the truck factor. The former helps in distributing the vehicle load over a larger length of pavement and prevents the overstressing of pavements at the point of contact between road and tire (larger number of tires and the presence of dual wheels). The impact of axle load value on truck factor is very clear from Figure . Both the maximum and average loads observed for each vehicle exceeded the legal axle loads.
Figures and 1 show the variation of truck factor with asphalt layer thickness and subgrade modulus, respectively. By increasing the thickness, the truck factor was reduced for all classes of vehicles. The reduction in truck factor for class B2, S112 and S113 followed almost same reduction pattern. The truck factor is reduced by almost 45–47% by increasing the asphalt layer thickness from 10 cm to 20 cm for all vehicle classes. This is justified by the presence of two single axles in the vehicle which affects the value of truck factor (in terms of AP value in the equation). The value of truck factor for class BH3 and S123 was lower than the rest because of higher AP value. The increase in subgrade modulus had a negligible effect on truck factor. The reason being, the load distribution follows a triangular pattern while flowing downward from asphalt layer to subgrade. By the time, the stresses reach the subgrade; it has reduced significantly.
8. Conclusions
This paper aimed at quantifying the number of overloaded vehicles and the impact of overloaded axles on road structure in terms of ESALs using the modified ESALs equation by incorporating the effect of asphalt layer thickness, subgrade modulus and axle configuration in addition to the axle load.
The traffic data obtained from WIM station have showed a higher percentage of passes of class B2 and a lower percentage of class S122. A large number (about 34.45%) of the class B2 were found to be overloaded.
It has also been concluded that the truck factor is higher for class B2 and lower for class S123 vehicles. This conclusion has justified the effect of axle configuration on the resulting truck factor value. It has also encouraged the use of 4-, 5- and 6-axle semi-trailers instead of 2- and 3- axle trucks. The reason being the presence of 2- and 3-axle groupings (tandem, tridem) and dual tires ensures better distribution of load over the pavement. Thus, the use of AP value during ESALs calculation must be considered.
It is also concluded that the effect of overloaded vehicles on road pavement can be reduced by increasing the asphalt layer thickness. For class BH3 and S123, with the increase in asphalt layer thickness from 10 cm to 20 cm, the truck factor is reduced by almost 49% and 48%, respectively. The increase in subgrade modulus has showed a very minimal effect on the truck factor. The optimum asphalt layer thickness suggested is 10 cm, as it is usually recommended in pavement design of highways in the study region.
The results obtained in this paper have some limitations such as the value of exponent “α” representing pavement failure mode. Another disadvantage of this model is that since it was developed for granular layer of 20 cm and asphalt stiffness of 5000 MPa (considering normal field conditions), this model will not be applicable if these values are changed.
Correction
This article was originally published with errors, which have now been corrected in the online version. Please see Correction (https://doi.org/10.1080/23311916.2019.1613330)
Acknowledgements
The authors would like to thank NHA for providing the axle load data for the study period. The authors would also like to show their gratitude to the University of Engineering and Technology Peshawar for providing a research-oriented environment.
Additional information
Funding
Notes on contributors
Rawid Khan
Dr. Rawid Khan has done PhD in Transportation Engineering from University of Nottingham, UK and currently serving as Assistant Professor in the Department of Civil Engineering, University of Engineering and Technology Peshawar. He has supervised many Masters and PhD students. The key research areas of his lab include:
Traffic Safety
Vehicle and Pavement Interaction
Traffic studies; modeling of traffic scenarios using microscopic and macroscopic simulation models
Pavement Materials
In addition to this, he also provides his services to key National Highway Agencies and Departments.
The research conducted in the present paper is an effort to quantify the magnitude of overloading and find the variables, which reduces pavement life. The research will be continued further for conducting a cost-based analysis of traditional and proposed pavement design method.
References
- Albayati, A. K. , & Saadi, A. (2017, May). Influence of axle overload on the performance of local flexible pavement. 7th Scientific Engineering and 1st International Conference “Recent Trends in Engineering Sciences and Sustainability (pp. 17–18). Baghdad.
- American Association of State Highway and Transportation Officials . (1993). AASHTO guide for design of pavement structures . Washington, D.C.
- Amorim, S. I. R. , Pais, J. C. , Vale, A. C. , & Minhoto, M. J. C. (2014). A model for equivalent axle load factors. International Journal of Pavement Engineering , 16(10), 881–893. doi:10.1080/10298436.2014.968570
- Archilla, R. , & Madanat, S. (2000). Development of a pavement rutting model from experimental data. ASCE Journal of Transportation Engineering , 126(4), 291–299. doi:10.1061/(ASCE)0733-947X(2000)126:4(291)
- Behiry, A. E. , & Ebrahim, A. (2012). Fatigue and rutting lives in flexible pavement. Ain Shams Engineering Journal , 3(4), 367–374. doi:10.1016/j.asej.2012.04.008
- Gulyas, A. (2012). Axle load trends in hungary and their effects on pavement structural design. Procedia - Social and Behavioral Sciences , 48, 888–896. doi:10.1016/j.sbspro.2012.06.1066
- Hadiwardoyo, S. P. , Sumabrata, R. J. , & Berawi, M. A. (2012). Tolerance limit for trucks with excess load in transport regulation in Indonesia. MAKARA of Technology Series , 16(1). doi:10.7454/mst.v16i1.1336
- Kubo, P. , Paiva, C. , Ferreira, A. , & Larocca, A. (2015). Influence of shock absorber condition on pavement fatigue using relative damage concept. Journal of Traffic and Transportation Engineering (English Edition) , 2(6), 406–413. doi:10.1016/j.jtte.2015.10.001
- Laboratoire Central des Ponts et Chaussées (LCPC) . (1994). French design method for flexible pavements . Paris: Laboratoire Central des Ponts et Chaussées.
- Legal axle loads, national highways authority, Pakistan. (2018). Retrieved from http://www.nha.gov.pk
- Oliveira, L. P. , Paiva, C. L. , & Ferreira, A. (2016, May). Impact assessment in the pavement life cycle due to the overweight in the axle load of commercial vehicles. 4th international conference on road and rail infrastructure (pp. 23–25). Sibenik.
- Pais, J. , Amorim, S. , & Minhoto, M. (2013). Impact of traffic overload on road pavement performance. Journal of Transportation Engineering , 139(9), 873–879. doi:10.1061/(ASCE)TE.1943-5436.0000571
- Prozzi, J. , & Madanat, S. (2003). Incremental nonlinear model for predicting pavement serviceability. Journal of Transportation Engineering , 129(6), 635–641. doi:10.1061/(ASCE)0733-947X(2003)129:6(635)
- Timm, D. , Tisdale, S. , & Turochy, R. (2005). Axle load spectra characterization by mixed distribution modeling. Journal Transportation Engineering , 131(2), 83–88. doi:10.1061/(ASCE)0733-947X(2005)131:2(83)
- Zaghloul, S. , & White, T. D. (1994). Guidelines for permitting overloads. I: Effect of overloaded vehicles on the Indiana highway network. Journal of Transportation Engineering , 2013(139), 873–879. Purdue Univ., West Lafayette.