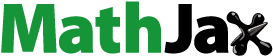
Abstract
The multivariate control charts are not only used to monitor the mean vector but also can be used to monitor the covariance matrix. The multivariate variability charts are used to guarantee the consistency of products in the subgroup. Many researchers have been studied the multivariate control chart for variability. Nevertheless, those conventional methods have several drawbacks because it is developed based on the determinant of the covariance matrix and not free of the measurement unit. To overcome such issues, this paper proposes the multivariate control chart for variability based on trace of the squared correlation matrix. Kernel Density Estimation is used to improve estimated control limit. The kernel density estimation method is used to calculate the control limit. Through simulation studies, the performance of the proposed chart is evaluated using the average run length (ARL). The control limits of the proposed chart are produced in control ARL at about 370 for α = 0.00273. Meanwhile, the proposed chart demonstrated better performance to detect the shift for the large value of quality characteristics and sample size. The proposed chart also produces a better performance than the conventional generalized variance chart when used to monitor the real case data.
PUBLIC INTEREST STATEMENT
A control chart is one of the improvement tools to monitor how a process changes over time. A good control chart will provide precise conclusion if the special causes of variation affect the actual process. The multivariate variability charts are used to guarantee the consistency of products in the subgroup. However, the conventional methods have several drawbacks because it is developed based on the determinant of the covariance matrix and not free of the measurement unit. This paper proposes the multivariate control chart for variability based on trace of squared correlation matrix to overcome such issues. Kernel density estimation is nonparametric techniques used to improve estimated control limit. Furthermore, the proposed control chart draws a correct decision to monitor real problem compared to the conventional method.
1. Introduction
One of the most powerful tools in statistical process control (SPC) is the control chart, which has been widely used in industries and services. Based on the type of monitored quality characteristics, there are two kinds of control charts: variable for interval or ratio scale (Page, Citation1961; Roberts, Citation1959; Shewhart, Citation1924) and attribute for categorical scale (Ahsan, Mashuri, & Khusna, Citation2017; Wibawati, Purhadi, & Ahsan, Citation2018; Wibawati, Purhadi, & Irhamah, Citation2016). At present, the demands of the consumers for the quality of the product are increasing, both in terms of the quality level and in terms of quality characteristics number. The quality of a product is not only determined by one characteristic but also by more than one quality characteristics. Therefore, to monitor the quality of a product, the multivariate control chart is needed in order to monitor several quality characteristics simultaneously. The multiple quality characteristics should be monitored together using a multivariate control chart. The recent development for multivariate control chart includes Hotelling’s T2 control chart (Abu‐Shawiesh, Kibria, & George, Citation2014; Ahsan, Mashuri, Kuswanto, Prastyo, & Khusna, Citation2018a; Alfaro & Ortega, Citation2009; Ali, Syed Yahaya, & Omar, Citation2013; Alkindi & Prastyo, Citation2016), MCUSUM control chart (Arkat, Niaki, & Abbasi, Citation2007; Issam & Mohamed, Citation2008; Khusna, Mashuri, Ahsan, Suhartono, & Prastyo, Citation2018; Noorossana & Vaghefi, Citation2006), and MEWMA control chart (Chen, CHENG, & Xie, Citation2005; Khusna, Mashuri, Suhartono, & Ahsan, Citation2018; Pirhooshyaran & Niaki, Citation2015).
The multivariate control charts are not only used to monitor the mean vector but also can be used to monitor the covariance matrix. The control charts are not only used to monitor the multivariate mean but also used to monitor multivariate variability. Multivariate mean vector charts are useful in seeing product uniformity between subgroups of observation, while the multivariate variability charts are used to ensure the uniformity of products in the subgroup.
Many definitions of multivariate variability measures are found in the literature, as stated by Djauhari (Citation2005b) such as total variance (TV) (Chatterjee & Hadi, Citation2009), volume ellipsoid (VE) (Croux & Haesbroeck, Citation1999; Grambow & Stromberg, Citation1998), generalized variance (GV) (Alt & Smith, Citation1988; Montgomery, Citation2009; Tang & Barnett, Citation1996). Several variants of GV can also be found in the literature includes effective variance (EV) (Peña & Rodrı́guez, Citation2003), generalized standard deviation (GSD) (Alt & Smith, Citation1988), relative generalized variance (RGV) (Tang & Barnett, Citation1996), vector variance (VV) (Suwanda & Djauhari, Citation2003), and correlation matrix chart (Sindelar, Citation2007).
The advantage of using the correlation matrix chart (Sindelar, Citation2007) in monitoring variability process is not affected by units of measurement from the quality characteristics because it uses a correlation matrix, while the other charts based on covariance matrices or successive difference covariance matrices are not free of the measurement unit. However, the chart developed based on the determinant of the correlation matrix has weaknesses because of the matrix determinant nature. The first weakness is the quality characteristics with a small variance will result in decreasing value of multivariate variability. Second, a quality characteristic is a linear combination of the other variables, if it is used then the multivariate variability becomes small. To overcome these weaknesses, Suwanda and Djauhari (Citation2003), Huwang, Yeh, and Wu (Citation2007), and Djauhari (Citation2010) proposed the trace operator.
Mashuri, Haryono, Wibawati, Khusna, and Ahsan (Citation2016) have succeeded in developing a Trace Rho 2 chart based on the squared correlation matrix using the trace operator, for the simplify henceforth it is called Tr(R2). Besides having a relatively good performance, this chart has two other advantages, which are free of unit measurement and can avoid the weaknesses of the determinant properties of a matrix. Also, there are two essential advantages of trace operators compared to determinant. First, trace statistics can deal with determinant weaknesses, that is, trace values will not produce small value. Even if one of the correlations between variables is very small or near to zero. Second, the trace of a matrix can still be calculated and will not be zero, even though the covariance matrix is definite negative.
Although it has some advantages, this chart has never been applied to monitor the actual production process and its exact distribution is still unknown. To overcome this issue, kernel density estimation (KDE) can be employed to calculate the control limit (Ahsan, Mashuri, Kuswanto, Prastyo, & Khusna, Citation2018b; Chou, Mason, & Young, Citation2001; Phaladiganon, Kim, Chen, Baek, & Park, Citation2011; Phaladiganon, Kim, Chen, & Jiang, Citation2013).
Based on the aforementioned reasons, this paper focuses on developing the multivariate variability control chart based on trace squared of correlation matrix R. The KDE method is employed to estimate the control limit of the proposed chart. The performance of the proposed chart is also evaluated using the average run length (ARL) criteria for several numbers of quality characteristics p and number of sample size n. Section 2 presents some of related work to this study. In Section 3, a brief review of the proposed Tr(R2) statistics and KDE method are presented. The methodology of simulation study is exhibited in Section 4. Section 5 contains the illustrative example of Tr(R2) control chart. Finally, Section 6 is devoted to the conclusion and future research.
2. Related works
Many works studied the utilization of a control chart to monitor the shift in variance. In univariate case, the simplest method is the Shewhart sample range (R) and sample standard deviation (S) charts. Riaz (Citation2008) proposed Q chart based on interquartile range (IQR), for monitoring changes in process dispersion under normality assumption. For non-normal distribution, Abbasi and Miller (Citation2012) inspected the performance of some univariate variability charts such as R, S, IQR, Downton’s estimator, median absolute deviation (MAD), as well as Sn and Qn. Kang, Lee, Seong, and Hawkins (Citation2007) developed coefficient of variation (CV) chart using rational groups of observations. Castagliola, Celano, and Psarakis (Citation2011) developed new method to monitor the CV by means of 2 one-sided EWMA charts of the coefficient of variation squared and later improved by Yeong, Khoo, Tham, Teoh, and Rahim (Citation2017). Calzada and Scariano (Citation2013) developed synthetic coefficient of variation (SynCV) chart. An adaptive Shewhart control chart implementing a variable sample size strategy in order to monitor the coefficient of variation is developed by Castagliola, Achouri, Taleb, Celano, and Psarakis (Citation2015). For short-run production, Amdouni, Castagliola, Taleb, and Celano (Citation2015) introduced an adaptive Shewhart control chart using a variable sample size (VSS). Variable sampling interval (VSI) Shewhart chart can also be used to monitor the coefficient of variation in a short production run context (Amdouni, Castagliola, Taleb, & Celano, Citation2017). For detecting small and moderate shifts, Khaw, Khoo, Yeong, and Wu (Citation2017) proposed variable sample size and sampling interval (VSSI) feature to improve the performance of the basic CV chart. The side sensitive group runs chart for the CV (SSGR CV) chart is developed. The chart surpasses the other charts under comparison, for most upward and downward CV shifts (W C Yeong et al., Citation2017).
The most popular multivariate variability control chart is the GV control chart (Alt & Smith, Citation1988) which was refined by Djauhari (Citation2005a). The properties of this method are studied by Aparisi, Jabaioyes, and Carrion (Citation1999). Aparisi, Jabaloyes, and Carrión (Citation2001) developed the new design of the GV chart |S| with adaptive sample size. Grigoryan and He (Citation2005) introduced the multivariate double sampling (MDS) |S| control chart scheme for controlling shifts in a covariance matrix. Doǧu and Kocakoç (Citation2011) proposed the GV control chart based on maximum likelihood estimation which can be used to monitor the multivariate process dispersion and detect the time of the change in covariance matrix. Hamed (Citation2014) improved the performance of GV chart |S| to monitor the multivariate process. Lee and Khoo (Citation2017) developed the multivariate synthetic generalized sample variance |S| (synthetic |S|) chart. The comparative studies show that the synthetic |S| chart outperforms the conventional |S| chart in detecting shifts in the covariance matrix of a multivariate normally distributed process. The combination of the double sampling, variable sample size and variable sampling interval features (DSVSSI |S| chart) is applied to monitor the shifts in the covariance matrix of a multivariate normally distributed process (Lee & Khoo, Citation2018).
In addition, many other multivariate variability control charts have been developed, namely vector variance chart (Suwanda & Djauhari, Citation2003), successive difference covariance matrix chart (Ahsan et al., Citation2018b; Djauhari, Citation2010; Holmes & Mergen, Citation1993), correlation matrix chart (Sindelar, Citation2007), multivariate coefficient of variation chart (Khatun, Khoo, Lee, & Castagliola, Citation2018; Lim, Khoo, Teoh, & Haq, Citation2017; Wai Chung Yeong, Khoo, Teoh, & Castagliola, Citation2016), VMAX statistic (Costa & Machado, Citation2009; Gadre & Kakade, Citation2018; Machado, Costa, & Rahim, Citation2009), Lasso chart (Maboudou-Tchao & Diawara, Citation2013), Gini mean differences based matrix (Riaz & Does, Citation2008), sample range multivariate (Costa & Machado, Citation2011), and MEWMA (Yeh, Huwang, & Wu, Citation2005). Furthermore, the new chart for monitoring high dimensional variability of individual observation is introduced by Li and Tsung (Citation2019).
3. Charting procedures
3.1. Tr (R2) statistics
Let the p dimension sample random vectors, are taken from the population which follow multivariate normal distribution
The sample correlation matrix R of those random vectors can be written as follows:
where
, for i = 1, 2, …, p and
is the coefficient correlation corresponded to i-th and k-th variable, for i = 1, 2, …, p and j = 1, 2, …, p. Furthermore, the Tr(R2) statistics are defined as follows:
where p denotes the number of variables, and b is a positive number where the value is determined by the correlation among the variables.
For example, three-dimension sample random vectors are taken from the population which follow the multivariate normal distribution. The sample correlation matrix can be written as
Tr(R2) statistics are calculated as follows:
with common form diagonal elements are:
Because R is symmetric, , then
Tr(R2) statistic for the number of quality characteristics p = 3 can be calculated as p + b, where p = 3 and
In general, for p quality characteristics, Tr(R2) can also be written as Tr(R2) = p + b, where p is the number of quality characteristic and b is two times of sum squared coefficient correlation which can be expressed as:
The minimum value of Tr(R2) is p for and its maximum value is p2 for
Figure illustrated the statistics of Tr(R2) for p = 3 with various coefficient correlation. It can be seen that the larger coefficient correlation the larger statistics Tr(R2) produced. Because of the Tr(R2) statistics is obtained from
where b is two times of sum squared coefficient correlation, the distribution of Tr(R2) statistics is not easy to determine. Thus, this research employs the KDE to estimate the empirical distribution of Tr(R2).
3.2. Kernel density estimation
KDE method is a non-parametric method to estimate the probability density function of a random variable. This method was first introduced by Rosenblatt (Citation1956) and Parzen (Citation1962) so that its name is called the Rosenblatt–Parzen kernel density estimator which is the development of the histogram estimator. Chou et al. (Citation2001) proposed KDE to estimate the distribution of T2 statistic. Let T2 is a Hotelling’s statistic which obtained under in-control condition. The distribution of T2 statistic could be calculated with the following kernel function:
where K and h define kernel function and smoothing parameter, respectively. Table presents some kernel functions displayed in (Härdle & Linton, Citation1994), where I is an indicator. The most used Gaussian Kernel is used in the analysis for this paper.
Table 1. List of kernel function (Härdle & Linton, Citation1994)
The control limit in Equationequation (2)(2)
(2) can be calculated using tables of integrals, in closed form distribution. However, the control limit might be not efficient to be calculated if the distribution is not closed form. Thus, the kernel control limit is solved using trapezoidal rule (Burden & Faires, Citation2011), one of the numerical integration methods to approximate the definite value of integral equation.
Furthermore, the control limit of T2 based on KDE could be estimated by taking the percentile of kernel distribution. Hence, the control limit of T2 based on KDE equal to -th percentile of T2 distribution which could be calculated using as follows:
3.3. Tr(R2) control chart based on KDE
The following procedures are used to form the Tr(R2) control chart with the KDE control limit.
Procedures to form the Tr(R2) control chart:
Specify the level of significance
and sample size n.
Input data as a matrix with the dimension of
, where m denotes the number of subgroups.
For each subgroup i from 1 to m:
Calculate the correlation matrix
with a dimension of
from i-th subgroup matrix with a dimension of
.
Calculate
which has the dimension of
.
Calculate the statistics
with a dimension of
For i = 1,2,.,m subgroup the dimension of the vector
is
Create the control chart by plotting the
, where i = 1, 2, … m and Control Limit (CL) calculate using KDE method.
Procedures to calculate the density of using KDE:
1. Determine the type of kernel, in this study Gaussian kernel is used with the following equation:
2. Define so that the Equationequation (3)
(3)
(3) can be written as follows:
where
is the optimum bandwidth.
3. Calculate the density of statistics as follows:
4. Substitute the value of to equation so that the following equation is obtained:
Procedures to calculate the control limit Tr(R2) control chart using KDE:
1. Calculate the cumulative distribution function of using the following equation:
2. By using the trapezoid rule method which is a numerical integration method, the integral can be computed as follows:
3. The control limit of Tr(R2) control chart can be estimated by taking the th percentile of the empirical density of
as the following equation:
4. Simulation studies
In this section, several simulation studies are conducted to investigate the performance of the proposed chart. The quality characteristics are assumed to follow the multivariate normal distribution, First, the performance of the control limit is evaluated by calculating its ARL0. Further, the performance of the proposed chart to detect a shift in the process is also evaluated using ARL1 criteria. The simulation study, ARL0 and ARL1 of the proposed chart is calculated using Algorithm 1.
Algorithm 1. Calculation of ARL0 and ARL1
1. Specify the significance level , number of characteristics p and sample size n.
2. Calculate the control limit (CL) for specified parameters given in step 1 using KDE procedure in section 2.
3. For 1000 repetitions, follow these steps:
a. Generate the data which follow Multivariate Normal distribution with vector and covariance matrix
.
b. Calculate statistics from the generated data.
c. Calculate the run length (RL), number of samples until finding the first statistic which is greater than CL.
4. Calculate ARL0 by taking the average of RL over 1000 replications.
5. Define , where
a shift in process.
6. For 1000 repetitions follow these steps:
a. Generate the data which follow Multivariate Normal distribution with vector and covariance matrix
.
b. Calculate statistics from the generated data in step 6.a.
c. Calculate the RL’, number of samples until finding the first statistic which is greater than CL.
7. Calculate ARL1 by taking the average of RL’ over 1000 replications.
8. Repeat step 1 until 7 for different value of p and n.
4.1. Control limit
In this section, the performance of the KDE control limit is evaluated using ARL0 criteria. Tables and present the KDE control limits of the proposed chart. Since the simulation study is conducted using significance level 0.00273, which corresponds to three-sigma, the ARL0 equals to 370. Three sigma control limit is used in this study because it refers to the processes that run efficiently to create the highest quality of production goods. For several combinations of n and p, the control limits calculated using the KDE method always produce ARL0 at about 370 which indicates that the KDE control limit is reliable for process monitoring. Moreover, it also can be seen that the value of control limits become smaller for the larger value of sample size n and it became larger as the larger value of quality characteristics p.
Table 2. Control limit of the proposed chart with and
for p = 3 to 10 for in-control ARL 370
4.2. Performance of the proposed chart
This section provides the performance evaluation of the proposed control chart using the KDE control limit. The out of control ARL is calculated by adding shift to covariance matrix , where
. The simulation study is conducted for
,
over the various number of characteristics quality p and number of sample n. In addition, the KDE control limit is taken from Tables and for
.
Table 3. Control limit of the proposed chart with and
for p = 11 to 30 for in-control ARL 370
4.2.1. Level of significance sarathkumar
In this subsection, the performance of the proposed chart is evaluated using Table shows the ARLs of the proposed chart with n = 10 for the various number of quality characteristics. It can be known that the larger shift in the process, the faster the proposed chart to detect the out-of-control signal. Furthermore, the proposed chart become more sensitive for the larger number of quality characteristics p. This is confirmed by the value of ARL1 for such condition.
Table 4. ARLs of the proposed chart with ,
and n = 10 for various number of quality characteristics p
Table shows the ARLs of the proposed chart with n = 20 for the various number of quality characteristics p. Similar to the previous case, it can be seen that the larger shift in the process, the faster the proposed chart to detect the out-of-control signal. Furthermore, the proposed chart become more sensitive for the larger number of quality characteristics p. Meanwhile, the performance of the proposed chart to detect a shift in the covariance matrix for n = 30 is presented in Table . The ability of the proposed chart to detect shift is increased as the larger number of sample size n and number of quality characteristics p.
Table 5. ARLs of the proposed chart with ,
and n = 20 for various number of quality characteristics p
Table 6. ARLs of the proposed chart with ,
and n = 30 for various number of quality characteristics p
Table shows the ARLs of the proposed chart with n = 50 for the various number of quality characteristics. For this case, the proposed chart shows great performance in detecting the shift in the process. This is shown by the proposed chart become very sensitive for the larger number of quality characteristics p. Moreover, the performance of the proposed chart to detect a shift in the covariance matrix for n = 100 is presented in Table . The oversensitive performance of the proposed chart to detect the small number of a shift in the covariance matrix is found for this case. It can be seen that the value of ARL1 for this case is 1.00 except p = 3 for only 0.1 shift in the covariance matrix. Figure shows the performance comparison of the proposed chart with a number of quality characteristics p = 3 for various value of sample size n. It can be seen from the figure that the value of ARL1 for n = 100 is very small indicated that the proposed chart is very sensitive to detect the shift for such condition. In general, the proposed chart becomes more sensitive as the number of sample size increase.
Table 7. ARLs of the proposed chart with ,
and n = 50 for various number of quality characteristics p
Table 8. ARLs of the proposed chart with ,
and n = 100 for various number of quality characteristics p
4.2.2. Level of significance sarathkumar
In this subsection, the performance of the proposed chart is evaluated for Simulation studies are conducted using a number of sample n = 10, number of quality characteristic p = 3, 4, 5, 7, and 10, as well as
Table tabulated the performance of the proposed chart for with n = 10 for the various number of quality characteristics. The ARL0 is written as the bold number while the value inside bracket below the ARL0 is the estimated KDE control limit. It can be seen that for
, the ARL0 produced is around 200. These facts prove that estimated ARL0 produce by proposed control limit has a similar value to ARL0 in the theory. The theoretical ARL0 is equal to
or in case is
According to the table, it can be concluded that higher number of quality characteristic p smaller value of ARL1. In other words the larger quality characteristics the more sensitive the proposed chart to detect shift in the covariance matrix.
Table 9. ARLs of the proposed chart with ,
,
and n = 10 for various number of quality characteristics p
Tables and 1 report the performance of the proposed chart for , respectively. Similar to the previous case, the estimated ARL0 is around 100 for
and around 20 for
The pattern is same as the previous case, the proposed chart has a better performance for the larger quality characteristics.
Table 10. ARLs of the proposed chart with ,
,
and n = 10 for various number of quality characteristics p
Table 11. ARLs of the proposed chart with ,
,
and n = 10 for various number of quality characteristics p
4.3. Discussion
The previous sections present the simulation result of the control limit and performance of the proposed chart. From these results, there are several notes that can be used as further discussion. First, the control limit of the proposed chart always shows the consistent ARL0 value at about 370 for . It also has the consistent ARL0 for
by producing value at about
This fact indicated that the proposed KDE method in calculating the control limit has a great ability to obtain the approximation value of the control limit. This happens due to the KDE method employed can capture all changes on the distribution of statistics Tr and estimated the control limit based on the empirical density of the statistics proposed.
Second, the proposed chart has an outstanding ability to detect a shift in the covariance matrix, especially for the larger number of quality characteristics p and sample size n. This may happen due to the ability of the proposed statistics to detect small changes in the covariance matrix. By this result, it can be said that the proposed chart has the great ability to detect the shift in the higher dimensional data.
5. Illustrative example
A numerical example is given in this section in order to illustrate the operation of the Tr(R2) control chart with the KDE control limit. In this section, we use the ZA fertilizer production dataset in carbonation step to illustrate the use of the proposed chart. There are 44 samples with each of them has 12 observations. The quality characteristics measured in this dataset include CO2 (g/L), NH3 (g/L), the ratio of CO2 and NH3, specific weight (kg/L), as well as temperature (°C). It can be seen that the quality characteristics are recorded with different measures.
First, by treating the first 20 samples as the in-control process, the control limit is estimated using the KDE method. Further, the estimated KDE control limit, which equals 14.1633, is used to monitor the full dataset. Figure shows the monitoring process of the ZA fertilizer production dataset. Moreover, the conventional GV in Figure also employed to monitor this dataset as the benchmark of the proposed chart.
According to the figures, it can be seen that the proposed chart can detect the changes in the covariance matrix by declaring three out-of-control samples. Meanwhile, the conventional GV chart did not detect any out of control signal. Furthermore, the conventional method produces very small and similar statistics. This fact can be proved that the proposed chart is more sensitive to detect changes in the covariance matrix than the conventional one. Also, this is confirmed the second drawback of the conventional method that is the multivariate variability becomes small due to the quality characteristic is a linear combination of the other variables.
6. Conclusion
In this paper, the Tr(R2) control chart based on the squared correlation matrix with the trace operator is proposed in order to overcome the drawbacks of the existing multivariate variability chart. Kernel Density Estimation method is used to calculate the better control limit for the proposed chart. The simulation studies show that for the several combinations of a number of quality characteristics p and number of sample size n, the control limit using the KDE method always results in an ARL0 at about 370 for . For
the proposed chart has ARL0 near to
For the shifted process, the proposed chart demonstrated the great performance as shown by the ARL1 value. The performance of the proposed chart becomes better for the larger number of quality characteristics and the number of sample size n in the process. The application of the proposed chart to monitor the ZA fertilizer production dataset shows that the proposed chart has a better performance to monitor the covariance matrix than the GV chart. For future research, the proposed chart will be applied to detect high dimensional big data due to its great ability for such a case. In addition, the use of the bootstrap resampling method is also appropriate for estimating the control limit of the proposed chart.
Disclosure statement
The authors declare that there are no potential conflict of interest
Additional information
Funding
Notes on contributors
Muhammad Mashuri
The corresponding author, Dr. Muhammad Mashuri is an associate professor in the Department of Statistics, Institut Teknologi Sepuluh Nopember, Surabaya Indonesia. His research interest includes statistical quality control and multivariate analysis, especially in industrial field. Haryono, Diaz Fitra Aksioma, and Wibawati are senior lecturers whose research interest includes statistical process control. Hidayatul Khusna and Muhammad Ahsan are PhD students through Master Program of Education Leading to Doctoral Degree for Excellent Graduates, an accelerated program for undergraduate prepared to be candidate lecturers or researchers with doctoral degrees.
References
- Abbasi, S. A., & Miller, A. (2012). On proper choice of variability control chart for normal and non-normal processes. Quality and Reliability Engineering International, 28(3), 279–37. doi:10.1002/qre.1244
- Abu‐Shawiesh, M. O. A., Kibria, G., & George, F. (2014). A robust bivariate control chart alternative to the Hotelling’s T2 Control Chart. Quality and Reliability Engineering International, 30(1), 25–35. doi:10.1002/qre.1474
- Ahsan, M., Mashuri, M., & Khusna, H. (2017). Evaluation of Laney p’ Chart Performance. International Journal of Applied Engineering Research, 12(24), 14208–14217.
- Ahsan, M., Mashuri, M., Kuswanto, H., Prastyo, D. D., & Khusna, H. (2018a). Multivariate control chart based on PCA mix for variable and attribute quality characteristics. Production & Manufacturing Research, 6(1), 364–384. doi:10.1080/21693277.2018.1517055
- Ahsan, M., Mashuri, M., Kuswanto, H., Prastyo, D. D., & Khusna, H. (2018b). T2 control chart based on successive difference covariance matrix for intrusion detection system. In Journal of Physics: Conference Series, Makassar, Indonesia (Vol. 1028, p. 12220). IOP Publishing.
- Alfaro, J. L., & Ortega, J. F. (2009). A comparison of robust alternatives to Hotelling’s T2 control chart. Journal of Applied Statistics, 36(12), 1385–1396. doi:10.1080/02664760902810813
- Ali, H., Syed Yahaya, S. S., & Omar, Z. (2013). Robust hotelling T2 control chart with consistent minimum vector variance. Mathematical Problems in Engineering, 2013, 1–7. doi:10.1155/2013/401350
- Alkindi, M., & Prastyo, D. D. (2016). T2 hotelling fuzzy and W2 control chart with application to wheat flour production process. In AIP Conference Proceedings, Yogyakarta, Indonesia (Vol. 1746). Doi:10.1063/1.4953977
- Alt, F. B., & Smith, N. D. (1988). Multivariate process control. Handbook of Statistics, 7, 333–351.
- Amdouni, A., Castagliola, P., Taleb, H., & Celano, G. (2015). Monitoring the coefficient of variation using a variable sample size control chart in short production runs. The International Journal of Advanced Manufacturing Technology, 81(1), 1–14. doi:10.1007/s00170-015-7084-4
- Amdouni, A., Castagliola, P., Taleb, H., & Celano, G. (2017). A variable sampling interval Shewhart control chart for monitoring the coefficient of variation in short production runs. International Journal of Production Research, 55(19), 5521–5536. doi:10.1080/00207543.2017.1285076
- Aparisi, F., Jabaioyes, J., & Carrion, A. (1999). Statistical properties of the lsi multivariate control chart. Communications in Statistics - Theory and Methods, 28(11), 2671–2686. doi:10.1080/03610929908832445
- Aparisi, F., Jabaloyes, J., & Carrión, A. (2001). GENERALIZED VARIANCE CHART DESIGN WITH ADAPTIVE SAMPLE SIZES. THE BIVARIATE CASE. Communications in Statistics - Simulation and Computation, 30(4), 931–948. doi:10.1081/SAC-100107789
- Arkat, J., Niaki, S. T. A., & Abbasi, B. (2007). Artificial neural networks in applying MCUSUM residuals charts for AR(1) processes. Applied Mathematics and Computation, 189(2), 1889–1901. doi:10.1016/j.amc.2006.12.081
- Burden, R. L., & Faires, J. D. (2011). Numerical analysis. Cengage learning. USA. doi:10.1017/CBO9781107415324.004
- Calzada, M. E., & Scariano, S. M. (2013). A synthetic control chart for the coefficient of variation. Journal of Statistical Computation and Simulation, 83(5), 853–867. doi:10.1080/00949655.2011.639772
- Castagliola, P., Achouri, A., Taleb, H., Celano, G., & Psarakis, S. (2015). Monitoring the coefficient of variation using a variable sample size control chart. The International Journal of Advanced Manufacturing Technology, 80(9), 1561–1576. doi:10.1007/s00170-015-6985-6
- Castagliola, P., Celano, G., & Psarakis, S. (2011). Monitoring the Coefficient of Variation Using EWMA Charts. Journal of Quality Technology, 43(3), 249–265. doi:10.1080/00224065.2011.11917861
- Chatterjee, S., & Hadi, A. S. (2009). Sensitivity analysis in linear regression (Vol. 327). New York, NY: John Wiley & Sons.
- Chen, G., CHENG, S. W., & Xie, H. (2005). A new multivariate control chart for monitoring both location and dispersion. Communications in Statistics—Simulation and Computation®, 34(1), 203–217. doi:10.1081/SAC-200047087
- Chou, Y.-M., Mason, R., & Young, J. (2001). The control chart for individual observations from a multivariate non-normal distribution. Communications in Statistics: Theory & Methods, 30(8/9), 1937. doi:10.1081/STA-100105706
- Costa, A. F. B., & Machado, M. A. G. (2009). A new chart based on sample variances for monitoring the covariance matrix of multivariate processes. The International Journal of Advanced Manufacturing Technology, 41(7), 770–779. doi:10.1007/s00170-008-1502-9
- Costa, A. F. B., & Machado, M. A. G. (2011). A control chart based on sample ranges for monitoring the covariance matrix of the multivariate processes. Journal of Applied Statistics, 38(2), 233–245. doi:10.1080/02664760903406413
- Croux, C., & Haesbroeck, G. (1999). Influence function and efficiency of the minimum covariance determinant scatter matrix estimator. Journal of Multivariate Analysis, 71(2), 161–190. doi:10.1006/jmva.1999.1839
- Djauhari, A. M. (2005a). Improved monitoring of multivariate process variability. Journal of Quality Technology, 37(1), 32–39. Retrieved from http://proquest.umi.com/pqdweb?did=773835061&Fmt=7&clientId=3740&RQT=309&VName=PQD
- Djauhari, M. A. (2005b). Outlier detection: Some challenging problem for future research. Proceedings of the Eighth Islamic Countries Conference on Statistical Sciences, National University of Computer and Emerging Sciences, Lahore.
- Djauhari, M. A. (2010). A multivariate process variability monitoring based on individual observations. Modern Applied Science, 4(10), 91. doi:10.5539/mas.v4n10p91
- Doǧu, E., & Kocakoç, İ. D. (2011). Estimation of change point in generalized variance control chart. Communications in Statistics - Simulation and Computation, 40(3), 345–363. doi:10.1080/03610918.2010.542844
- Gadre, M. P., & Kakade, V. C. (2018). Two group inspection-based control charts for dispersion matrix. Communications in Statistics - Simulation and Computation, 47(6), 1652–1669. doi:10.1080/03610918.2017.1321120
- Grambow, S. C., & Stromberg, A. J. (1998). Combining the EID and FSA for computing the minimum volume ellipsoid. Deptartment of Stats., University of Kentucky.
- Grigoryan, A., & He, D. (2005). Multivariate double sampling |S| Charts for controlling process variability. International Journal of Production Research, 43(4), 715–730. doi:10.1080/00207540410001716525
- Hamed, M. S. (2014). Generalized variance chart for multivariate quality control process procedure with application. Applied Mathematical Sciences, 8(163), 8137–8151. doi:10.12988/ams.2014.49734
- Härdle, W., & Linton, O. (1994). Applied nonparametric methods. Handbook of Econometrics, 4(26), 2295–2339. doi:10.1016/S1573-4412(05)80007-8
- Holmes, D. S., & Mergen, A. E. (1993). Improving the performance of the T2 control chart. Quality Engineering, 5(4), 619–625. doi:10.1080/08982119308919004
- Huwang, L., Yeh, A. B., & Wu, C.-W. (2007). Monitoring multivariate process variability for individual observations. Journal of Quality Technology, 39(3), 258–278. doi:10.1080/00224065.2007.11917692
- Issam, B. K., & Mohamed, L. (2008). Support vector regression based residual MCUSUM control chart for autocorrelated process. Applied Mathematics and Computation, 201(1–2), 565–574. doi:10.1016/j.amc.2007.12.059
- Kang, C. W., Lee, M. S., Seong, Y. J., & Hawkins, D. M. (2007). A control chart for the coefficient of variation. Journal of Quality Technology, 39(2), 151–158. doi:10.1080/00224065.2007.11917682
- Khatun, M., Khoo, M. B. C., Lee, M. H., & Castagliola, P. (2018). One-sided control charts for monitoring the multivariate coefficient of variation in short production runs. Transactions of the Institute of Measurement and Control, 0142331218789481.
- Khaw, K. W., Khoo, M. B. C., Yeong, W. C., & Wu, Z. (2017). Monitoring the coefficient of variation using a variable sample size and sampling interval control chart. Communications in Statistics - Simulation and Computation, 46(7), 5772–5794. doi:10.1080/03610918.2016.1177074
- Khusna, H., Mashuri, M., Ahsan, M., Suhartono, S., & Prastyo, D. D. (2018). Bootstrap based maximum multivariate CUSUM control chart. Quality Technology & Quantitative Management, 1–23. doi:10.1080/16843703.2018.1535765
- Khusna, H., Mashuri, M., Suhartono, P. D., & Ahsan, M. (2018). Multioutput least square SVR based multivariate EWMA control chart. Journal of Physics: Conference Series, 1028(1), 12221. Retrieved from http://stacks.iop.org/1742-6596/1028/i=1/a=012221
- Lee, M. H., & Khoo, M. B. C. (2017). Optimal designs of multivariate synthetic |S| Control chart based on median run length. Communications in Statistics - Theory and Methods, 46(6), 3034–3053. doi:10.1080/03610926.2015.1048884
- Lee, M. H., & Khoo, M. B. C. (2018). Double sampling |S| Control chart with variable sample size and variable sampling interval. Communications in Statistics - Simulation and Computation, 47(2), 615–628. doi:10.1080/03610918.2017.1288246
- Li, Z., & Tsung, F. (2019). A control scheme for monitoring process covariance matrices with more variables than observations. Quality and Reliability Engineering International, 35(1), 351–367. doi:10.1002/qre.2403
- Lim, A. J. X., Khoo, M. B. C., Teoh, W. L., & Haq, A. (2017). Run sum chart for monitoring multivariate coefficient of variation. Computers & Industrial Engineering, 109, 84–95. doi:10.1016/j.cie.2017.04.023
- Maboudou-Tchao, E. M., & Diawara, N. (2013). A LASSO chart for monitoring the covariance matrix. Quality Technology & Quantitative Management, 10(1), 95–114. doi:10.1080/16843703.2013.11673310
- Machado, M. A. G., Costa, A. F. B., & Rahim, M. A. (2009). The synthetic control chart based on two sample variances for monitoring the covariance matrix. Quality and Reliability Engineering International, 25(5), 595–606. doi:10.1002/qre.992
- Mashuri, M., Haryono, P., Wibawati, A. D. F., Khusna, H., & Ahsan, M. (2016). Trace R2 control chart for monitoring variability. In International Conference on Theoretical and Applied Statistics-Conference Proceedings, Surabaya, Indonesia.
- Montgomery, D. (2009). Introduction to statistical quality control. New York: John Wiley & Sons Inc. doi:10.1002/1521-3773(20010316)40:6<9823::AID-ANIE9823>3.3.CO;2-C
- Noorossana, R., & Vaghefi, S. J. M. (2006). Effect of autocorrelation on performance of the MCUSUM control chart. Quality and Reliability Engineering International, 22(2), 191–197. doi:10.1002/qre.695
- Page, E. S. (1961). Cumulative sum charts. Technometrics, 3(1), 1–9. doi:10.1080/00401706.1961.10489922
- Parzen, E. (1962). On estimation of a probability density function and mode. The Annals of Mathematical Statistics, 33(3), 1065–1076. doi:10.1214/aoms/1177704472
- Peña, D., & Rodrı́guez, J. (2003). Descriptive measures of multivariate scatter and linear dependence. Journal of Multivariate Analysis, 85(2), 361–374. doi:10.1016/S0047-259X(02)00061-1
- Phaladiganon, P., Kim, S. B., Chen, V. C. P., Baek, J.-G., & Park, S.-K. (2011). Bootstrap-Based T 2 multivariate control charts. Communications in Statistics - Simulation and Computation, 40(5), 645–662. doi:10.1080/03610918.2010.549989
- Phaladiganon, P., Kim, S. B., Chen, V. C. P., & Jiang, W. (2013). Principal component analysis-based control charts for multivariate nonnormal distributions. Expert Systems with Applications, 40(8), 3044–3054. doi:10.1016/j.eswa.2012.12.020
- Pirhooshyaran, M., & Niaki, S. T. A. (2015). A double-max MEWMA scheme for simultaneous monitoring and fault isolation of multivariate multistage auto-correlated processes based on novel reduced-dimension statistics. Journal of Process Control, 29, 11–22. doi:10.1016/j.jprocont.2015.03.008
- Riaz, M. (2008). A dispersion control chart. Communications in Statistics - Simulation and Computation, 37(6), 1239–1261. doi:10.1080/03610910802049623
- Riaz, M., & Does, R. J. M. M. (2008). An alternative to the bivariate control chart for process dispersion. Quality Engineering, 21(1), 63–71. doi:10.1080/08982110802445579
- Roberts, S. W. (1959). Control chart tests based on geometric moving averages. Technometrics, 1(3), 239–250. doi:10.1080/00401706.1959.10489860
- Rosenblatt, M. (1956). Remarks on some nonparametric estimates of a density function. The Annals of Mathematical Statistics, Volume, 27, 832–837. doi:10.1214/aoms/1177728190
- Shewhart, W. A. (1924). Some applications of statistical methods to the analysis of physical and engineering data. Bell Labs Technical Journal, 3(1), 43–87. doi:10.1002/j.1538-7305.1924.tb01347.x
- Sindelar, M. F. (2007). Multivariate statistical process control for correlation matrices. University of Pittsburgh.
- Suwanda, & Djauhari, A. M. (2003). A new concept in monitoring multivariate process variability. Data Analysis Research Group, 2(2), 1–2.
- Tang, P. F., & Barnett, N. S. (1996). Dispersion control for multivariate processes. Australian Journal of Statistics, 38(3), 235–251. doi:10.1111/anzs.1996.38.issue-3
- Wibawati, M., Purhadi, M., & Ahsan, M. (2018). Perfomance fuzzy multinomial control chart. Journal of Physics: Conference Series, 1028(1), 12120. Retrieved from http://stacks.iop.org/1742-6596/1028/i=1/a=012120
- Wibawati, M., Purhadi, M., & Irhamah. (2016). Fuzzy multinomial control chart and its application. In AIP Conference Proceedings, Surabaya, Indonesia (Vol. 1718). doi:10.1063/1.4943351
- Yeh, A. B., Huwang, L., & Wu, C.-W. (2005). A multivariate EWMA control chart for monitoring process variability with individual observations. IIE Transactions, 37(11), 1023–1035. doi:10.1080/07408170500232263
- Yeong, W. C., Khoo, M. B. C., Teoh, W. L., & Castagliola, P. (2016). A control chart for the multivariate coefficient of variation. Quality and Reliability Engineering International, 32(3), 1213–1225. doi:10.1002/qre.1828
- Yeong, W. C., Khoo, M. B. C., Tham, L. K., Teoh, W. L., & Rahim, M. A. (2017). Monitoring the coefficient of variation using a variable sampling interval EWMA chart. Journal of Quality Technology, 49(4), 380–401. doi:10.1080/00224065.2017.11918004