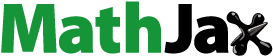
Abstract
In the Ilorin metropolis, there are power challenges. Energy supplied by Power Holding Company of Nigeria is insufficient for the social, technological, and industrial requirements of the metropolis. Moreover, the huge municipal solid waste (MSW) produced daily that supposed to be converted to energy is only constituting a nuisance. In waste to energy (WTE) procedures, the heating value (HV) of the MSW generated is pertinent in the selection or design of an appropriate waste to energy (WTE) technology required for waste conversion. The HV determination using ultimate analysis is tedious, expensive, and requires specialized equipment. A proximate analysis method that is less tedious and cheaper was adopted to obtain the dependent variables for the modeling of the HV. The high heating value (HHV) of MSW components was determined using a bomb calorimeter, and proximate analysis was used to determine the typical values for fixed carbon (FC), volatile matter (VM), Ash, and moisture (MC) to be 32%, 37%, 13%, and 5% correspondingly. The typical HV was estimated to be 24 MJ/kg. The heating value obtained from the bomb calorimeter was modeled against the dependent variables from proximate analysis. The conventional ordinary least squares (OLS) estimator is popularly used to estimate the model parameters. However, the performance of the estimator suffers a setback when the predictor variables are correlated. Alternatively, the ridge estimator (RE) and the principal component regression estimator (PCE) can be adopted. In this study, we combined PCE and RE to form the r-k class estimator for effective modeling. The estimators’ performances are assessed using the mean squares error (MSE) criterion. The estimator with the smallest MSE is generally preferred. The result, the MSE of OLSE, ridge, PCE, and r-k are 581.84, 2.56, 523.69, and 0.239, respectively. The r-k class estimator outperforms other estimators considered in this study and is employed for the modeling. With a unit increase in the volatile matter and fixed carbon, heating values increased by about 21% and 36%, respectively. Also, the heating values decrease by about 0.2% and 40%, respectively, with a unit increase in Ash and Moisture.
PUBLIC INTEREST STATEMENT
Enormous waste is generated daily in the Ilorin metropolis. The poor management system of waste results in the environmental pollution in the Ilorin metropolis. Moreover, power supplied to the city could not meet the social and economic demand; hence, there is a need for a renewable source of energy project to complement the hydropower source. The waste-to-energy management system will serve a dual purpose of efficient waste management and sustainable and renewable source of energy. Waste generated must be characterized physically, chemically, and thermally to predict the sustainability and the waste potential power. To select the appropriate technology for waste conversion, the heating value of the waste is very pertinent. This study, therefore, has developed a proximate-based model for modeling the heating value of combustible waste fractions using regression analysis. This model can be a tool to select the technology to be adopted for a waste conversion process.
1. Introduction
Municipal Solid Waste (MSW), otherwise called garbage or trash, consists of household wastes, institutional wastes, street wastes, market waste, and waste from business centres. It excludes hazardous waste from hospitals and industries. According to Ibikunle et al. (Citation2018), MSW includes food residue, glass/ceramic wastes, plastics, textiles, metals/tins, paper, wood, leather, nappies, slug, ash, etc. The extent of waste generation in different urban centres depends on population growth, change in fashion, consumption pattern, and technological development in such nations (Titiladunayo et al., Citation2018; Tozlu et al., Citation2016; Omari et al., Citation2014). Most municipals in the developing nations find it difficult to establish an efficient and sufficient waste management system, because of the financial implications on equipment and the expertise required, lack of reliable data that to be used in taking management decisions, unconsciousness, and inability to venture into WTE system (Ibikunle et al., Citation2020b, Citation2020a). Akdag et al. (Citation2016) and Khuriati et al. (Citation2017) also reported that efficient MSW management has become a principal environmental challenge in the metropolitan areas.
Increase in population, economic, and urbanization growth, and rise in standard of living have evidently contributed to the aggregate of waste generated in a metropolis. The disposal of MSW generated poses a significant problem, because of its enormous magnitude that may surpass the capacity of the environment (Khuriati et al., Citation2017). Burning and energy recovery has become one of the common methods to reduce the capacity of the waste (Khuriati et al., Citation2017). The thermal decomposition procedures obtainable for the treatment of solid MSW include combustion, pyrolysis, and gasification. The principal parameters required in the thermal procedures of MSW involve its composition and higher heating value (Zhou et al., Citation2014). HHV is the quantity of chemical energy contained in a fuel that is liberated during combustion (Erol et al., Citation2010). According to Ghugare et al. (Citation2014) and Parikh et al. (Citation2005), HHV, that is also known as the gross calorific value (GCV), is the heat energy produced during combustion of solid fuel, assuming the water content present in the fuel before and during combustion process as condensed liquid.
In the developed nations, waste components are valuables for the production sector and energy generation sector (SWM, Citation2015). Recovery of energy from waste is made possible, when non-recyclable waste components are converted into fuel, heat, and electrical energy through different processes that include gasification, combustion, pyrolysis, anaerobic digestion, and recovery of gas from the landfill (Reza et al., Citation2021). These processes are called WTE procedures. MSW can be a source of economic valuables if the processes or procedures involved in the conversion are understood and undertaken. It could be utilized as fuel for waste-to-energy (WTE) technology or as a material to produce other chemical products (Ibikunle et al., Citation2020). The conversion of MSW fractions to valuables could be through biochemical, thermochemical, or incineration processes. Psomopoulos et al. (Citation2009) and Shi et al. (Citation2016) submitted that incineration is still considered as the broadly used process in the conversion of MSW to energy. Laith et al. (Citation2017) and Ayodele et al. (Citation2017) reported that majority of the growing countries, do not have adequate technology to process the waste generated, or to recover its energy, to meet the demand for effective waste management and the pressure for energy.
Deficient waste management system has grossly increased environmental pollution, degradation of natural resources and also contributed to energy inadequacy. Furthermore, the waste generated are indiscriminately dumped and burnt in the open by the road or in an unscientific dump site, resulting in environmental consequential effects. They are hazardous to human health through unsightly scenes, surface, and underground water pollution. Also, as a source of greenhouse gases (GHGs) emission, it contributes to the depletion of Ozone layer. Therefore, sufficient and efficient management, exploitation, and conversion of MSW to useful energy are the optimal process to ameliorate carbon emission and ensuring a sustainable and ecological-friendly solution to bridging the gap between energy and the environment.
In 2014, Sweden was able to convert about 5.7 million tons of combustible waste fractions into 16,600 kWh of energy with 33 WTE plants, installed in the country. Electrical energy takes about 2000 kWh of the total energy, and the remaining 14,600 kWh was for heating (Akhator et al., Citation2016; SWM, Citation2015). In 2018, America combusted about 34.6 million tons of MSW fractions for energy recovery. Food waste took about 22%. Textiles, leather, and rubber took over 16%, while plastics and others were about 16%, paper and paperboard made about 12%. Other fractions were less than 10% (US EPA, Citation2018). In 2016, World Bank reported that the global waste generation was about 2010 million tons. And the generation projection for year 2055 is about 3400 million tons (Kaza et al., Citation2018). Abhishek et al., (Citation2021), reported that MSW generated in India in the year 2016 was about 52 million tons, with generation rate of about 0.14 million tons per day.
In Nigeria, notwithstanding the availability of renewable and non-renewable resources for energy generation, that include biomass, natural gas, coal, solar, hydro, and fossil fuels; Nigeria is still faced with the electricity crisis. This crisis has impeded both the nation’s socio-economic and technological development, which resulted in the shutdown of some companies, and relocation of others to the nearby countries. The forecast for national power demand for 2016 was put at 17,520 MW, and the capacity of the available power plant is just about 11,165.4 MW (Nnodim, Citation2016). The maximum power generation recorded thus far in Nigeria is about 5074.70 MW and the highest daily energy available is 109,372 MWh (Akhator et al., Citation2016). Nigeria generates almost 14 million tons of combustible MSW annually (Uchendu, Citation2008). These wastes are disposed to dumpsite because of insufficient knowledge and lack of interest in modern WTE technology. This waste could be conveniently converted to electrical energy of about 4400 GWh per year via combustion processes and would have helped ensure efficient waste management that engenders a clean environment (Akhator et al., Citation2016). In 2016, Ibadan Electricity Distribution Company (IBEDC) predicted that the availability of a 270 MW stable power supply will successfully cater for the power demand in the whole Kwara State (Ibadan Electricity Distribution Company (IBEDC), Citation2016). The quantity of MSW generated in Ilorin in 2016 was predicted to be about 320,000 tons in a year, with a generating rate of 0.78 kg/capita/day. It was also forecasted that 584 tons/day of combustible MSW in Ilorin with 21 MJ/kg heating value has the potential of 3200 MWh heat energy, 41 MW electrical energy, and 27 MW of power to the grid, which would have catered for 15% of the power demand for Kwara State if utilized (Ibikunle et al., Citation2019).
The demand for energy globally is on increase due to growth in the world population, and a considerable increase in the world economy (Smallbone et al. (Citation2020) and Haller et al. (Citation2020)). This demand is a great concern and challenge for the generation, distribution, economic-development, and sustainability of energy worldwide. Sustainable energy technologies contributed immensely to the provision of an alternative source of energy to reduce the burden of energy demand (Alkasrawi (Citation2004) and Salameh et al. (Citation2020)). Pyrolysis of MSW is a reliable technology for transforming MSW into chemicals and biofuels. MSW incineration can be replaced by Pyrolysis, to provide a clean energy (Song et al., Citation2020). One of the thermochemical processes that can be used to transform carbon-based organic waste into syngas by incomplete oxidation of waste in a reactor is called gasification (Arif et al., Citation2019). Prior to the conversion of MSW to gas, the waste is first pelletized to ensure a steady size and moisture content (Bosmans et al., Citation2013). Biochemical conversion of MSW into methane, ethanol, and other valuable products requires digestion of biomass with microorganisms and their enzymes. Biochemical process of energy generation is cost-effective and ecological-friendly. Anaerobic digestion and fermentation are the common biochemical conversion processes for waste to energy (Beyene et al., Citation2018; Chhotu et al., Citation2021). Zhang et al. (Citation2018) also reported that anaerobic digestion (AD) is an economical biotechnology for the treatment of biodegradable waste and recovery of bioenergy. It can be used to convert organic matter into biogas of about 50–70% CH4 and about 20–75 40% CO2. Fertilizer can be produced from the organic residue of AD. AD is not suitable for refractory organic wastes such as woods, because it does not degrade easily by AD directly due to high amount of cellulose, lignin, or hemicellulose components. It has to be pretreated to break the refractory compounds to biodegradable matters before they could be digested.
To quantify and characterize MSW for energy production, the source of waste, the type of materials, and the inherent physical compositions are important. Nevertheless, to design WTE facility, it is very important to consider factors, like the moisture content, the volatile matter content, the fixed carbon content, the proportion of combustible and non-combustible waste fractions, and the percentage of sulfur content. These properties are essential in choosing technology, capacity determination for power plant equipment, and the auxiliary facilities’ design, like the equipment to clean the flue gas (Shi et al., Citation2016). The heating or calorific value is one of the most important characteristics of MSW in determining the fuel’s energy content. It is almost inversely proportional to the capacity of a WTE furnace/boiler (Reddy, Citation2011) and is either measured by a bomb calorimeter or calculated using an empirical model. Fuel characteristics such as heating value (HV), proximate properties, and elemental composition should be considered to design an efficient system for the combustion of biomass for energy production (Sheng & Azevedo, Citation2015; Yin, Citation2011). The HV (or CV) refers to the heat released during the complete combustion of fuel (Ghugare et al., Citation2014), and it defines the energy content of fuel which is essential in the design analysis, simulations of thermal conversion systems and energy balance (Nhuchhen & Afzal, Citation2017).
The HHV of MSW can be determined experimentally by combusting the fuel in a high-pressure oxygen ambience in a bomb calorimeter; this measures the enthalpy change between the reactants and the products (Cordero et al., Citation2001; Parikh et al., Citation2005). The process involving an analogue bomb calorimeter is tedious, and using digital calorimeter is costly. Nevertheless, mathematical models based on the proximate characteristics of MSW can also be used to determine the HV. The weight percentage of volatile matter and fixed carbon must be considered to develop the model. Amin and Yang (Citation2012) reported that using characteristic values obtained from proximate analysis to develop models for predicting heating value is advantageous because, it is based on sample sizes and it gives an accurate estimation of the calorific values (Lawal et al., Citation2014). Various models that include Steuer’s and Dulong’s models have been used to predict MSW components’ HV (Ibikunle et al., Citation2019). These models utilized the percentage of elemental components obtained from the ultimate analysis, some models used the values from proximate analysis, and others used physical composition. Yin (Citation2011) reported that models based on the ultimate (chemical) analysis exhibit more appropriate accuracy.
WTE is a feasible alternative method for MSW management. The heating value of MSW has a major impact on the application of WTE techniques. MSW consists of heterogeneous material, thereby making the measurement of direct heating value not readily feasible (Khuriati et al., Citation2015). Many prefer the models based on proximate analysis; because, the experimental procedures involved in the ultimate analysis are tedious and costly (Ibikunle et al., Citation2020b; Küçükbayrak et al., Citation1991). In this paper, the heating value of MSW was described by the development of empirical model based on the values of proximate analysis conducted on the waste components because the proximate analysis is cheaper and faster. Engineer or scientist that is familiar with laboratory equipment such as digital top-loading balance, digital oven (of about 200°C) and digital furnace (of about 1200° C) based on standard test methods like American Society for Testing and Materials (ASTM) can conduct the experiment (Demirbas, Citation2003; Ibikunle et al., Citation2019; Küçükbayrak et al., Citation1991). The proximate analysis determines the moisture content (MC), volatile matter (VM), fixed carbon (FC) and ash contents (Ash) contained in MSW components (Ibikunle et al., Citation2019; Yin, Citation2011).
Few studies have shown us that the predictor variables in the modeling of HHV are correlated. Thus, the performance of the conventional ordinary least squares estimator will suffer a breakdown. The ridge estimator (RE) and the principal component regression estimator (PCE) are generally preferred to mitigate this problem. The two estimators were combined as a single estimator called the r-k class estimator. The estimator combines the advantage of both the ridge estimator and the principal component regression estimator.
This study aims at developing a proximate-based model that can be used to model the HHV of the combustible waste fractions by adopting regression analysis. Also, to adopt a more robust technique to estimate the coefficient in the model for effective prediction. The proposed models are based on physicochemical characteristics of MSW fractions (FC, VM, MC, and Ash) obtained from the proximate analysis, to ensure availability of reliable model that can predict the HV of MSW, combustible biowaste or biomass that prevents the rigours and cost involved in ultimate analysis procedures. The following estimators were considered in this study: the ordinary least squared estimator (OLSE), principal component estimator (PCE), ridge estimator (RE), and PCE-Ridge Estimator (r-k class estimator).
2. Study area
A case of Ilorin, Kwara State Nigeria, was surveyed for this study because of its population growth and, consequently, the massive MSW generated daily. Ilorin is the capital town of Kwara State comprising three municipals: Ilorin East, West, and South Local Government Areas. Ibikunle et al. (Citation2019) estimated the quantity of MSW generated in Ilorin in 2016 to be 302,000 tons per year at 0.78 kg per capita per day. The people responsible for the waste generation was predicted to be 1,055515, with Ilorin West having the highest population of 493,001 people, followed by Ilorin South with 282,465 people and Ilorin East with 280,049 people. The city is located on latitude 80 30ʹN and longitude 40 35ʹE, on land of about 100 km2 area. It is situated between Southwestern and middle belt parts of Nigeria.
Ilorin is divided into zones of traditional settlement and modern area. The center of the city comprises the palace zone, the king’s market, and the central mosque. The traditional zone encompasses the ancient settlement with old buildings. Another zone is a settlement for middle-class people, businessmen, and the professionals. The other zone comprises the neighboring villages. During this study, the Lasoju dumpsite of about 20 acres perimeter area was the only functional dumpsite; it is about 25 km away along Lagos—Ilorin expressway. is the Ilorin map showing the spatial study of MSW generation in Ilorin by Adedibu in 1985. shows some row-row bins (dumpsters) locations for collecting MSW in Ilorin metropolis (Ajadi & Tunde, Citation2010; Ibikunle et al., Citation2019). This study will encourage the prediction of MSW heating value for the procedures required to design WTE technology for energy generation.
The MSW generated in Ilorin is too enormous to be handled by the existing system of collection and disposal to the dumpsite. The MSW generation rate in Ilorin is very alarming and constituting a great challenge for the government to manage; because of the increasing daily population of the metropolis (Ajadi & Tunde, Citation2010). The insufficient waste management system in Ilorin is quite evident by some piles of uncollected waste bags along the streets and some illegal acts of indiscriminate disposal of wastes by the public to underdeveloped sites and waterways (Ibikunle et al., Citation2019, Citation2020). The MSW components produced in Ilorin, according to Ibikunle et al. (Citation2019), are huge comprising food residue, nylon, plastic bottle, bones, glass/ceramics, packaging box (carton), polypropylene sack, wood, cow-dung, excrement, sand/ash, others, tins/metals, grass/trimmings, paper, leather, rubber, textiles (rag), and toiletries (pampers, tissues and pads). Despite the day-to-day development in the WTE technological sector, Ilorin’s massive MSW is yet to witness conversion for other purposes. Rather, it still constitutes a nuisance to the public and various challenges to the management system.
3. Materials and method
In this paper, a survey on the municipal solid waste (MSW) fractions generated in Ilorin municipalities, Nigeria, was conducted. The MSW produced in Ilorin is an enormous one. Ibikunle et al. (Citation2019) and (Ibikunle et al., Citation2020) reported the composition of waste generated in Ilorin from households, institutions, event centres, market centres, parks, business centres, and yards, include paper, carton, sand/ash, grass/trimmings, nylon, plastic bottle, food residue, textiles (rag), cow-dung, leather, toiletries (sanitary pads), glass/ceramics, polypropylene sack, excrement, rubber, bones, leather metal/tins, and other biogenic waste. Eleven combustible fractions subjected to Laboratory analysis, out of the 19 fractions identified, were randomly selected at Lasoju dumpsite (about 20 km away from Ilorin) along Lagos-Ilorin expressway. Each sample presented for experimental tests was prepared in triplicates. The high heating or calorific value (HHV/HCV) of the selected components was determined using a digital bomb calorimeter. The Proximate analysis of each component was also performed using the digital oven furnace of models DHG 9053 and TWD, respectively. The HHV of the waste fractions was later modeled with the physicochemical properties obtained from proximate analysis to ascertain the thermal and chemical relationships between the HHV and the proximate characteristics.
3.1. Sampling of the MSW
In this study, a random sampling procedure adopted by Abdellah and Balla (Citation2013) was used to collect samples from Lasoju dumpsite of Ilorin metropolis. The aggregate MSW dumped on the site was very huge; therefore, few heaps of waste were selected randomly from which samples were drawn for physical characterization after pooling as suggested by NT ENVIR 001, (2005) and Issam et al. (Citation2010). Random subsets of waste sample were collected and poured on a large mat made of polypropylene sac. The subsets were mixed properly with shovel, later heaped to a shape inform of a cone, and were divided into four slices as suggested by Slavko et al. (Citation2012) and Sudhir et al. (Citation2010). Two of the slices that are diagonally opposite were discarded and the others were mixed again to obtain a sample representing the parent population of the MSW. Each sample is 240 l of a bin waste volume (EC SWA-Tool, Citation2004). Thirty (30) samples was suggested as being sufficient for waste characterization by Sharma and McBean (Citation2007), nevertheless 62 samples were considered in this study within the period of eight months of characterization to avoid error that may occur due to insufficient samples. Each of the 240 l of waste components were hand-sorted differently into components, and each component identified is kept in designated receptacle, and weighed.
3.2. Tests samples preparation
The eleven waste samples prepared for Laboratory analysis were sun-dried in the open air for five days shredded into particles of less than 30 mm (Omari, Citation2015). They were later milled into smaller particles to permit a larger surface area for easy digestion with reactants’ aid (Ibikunle et al., Citation2020a).
3.3. Laboratory analysis of MSW samples
3.3.1. Determination of moisture content
Percent moisture content of the waste samples was determined, by measuring three test samples of about 5-g each into crucibles and was dried for 1 h at 110°C in a digital oven (DHG 9053 model), based on ASTM D1348. It was later withdrawn into a desiccator to cool. The percentage moisture content (MC) of each test sample was calculated as a loss in weight fraction of sample before and after drying as presented in EquationEquation (1(1)
(1) ; Ibikunle et al., Citation2019; Vairam & Ramesh, Citation2013).
where is the mass (g) of empty crucible,
is the mass(g) of crucible + test-sample,
is the mass (g) of empty crucible + test-sample after heating,
is the moisture content.
3.3.2. Determination of volatile content
The percentage volatile matter of the waste components was carried out by heating the test samples whose moisture test have been performed at a temperature of 950° C for about 7 min in a muffle furnace based on ASTM D3175-11. After that the crucibles were cooled inside the desiccator and weighed. The percentage mass loss in the samples except that of percentage moisture gives the percentage volatile matter as presented in EquationEquation (2)(2)
(2) according to Adekunle et al. (Citation2015).
where is the mass (g) of empty crucible, is the mass(g) of crucible + test-sample before heating,
is the mass (g) of empty crucible + test-sample after heating and MC, is the percentage moisture content.
Determination of Ash content
Determination of the percentage Ash content that is in a waste fraction is to establish how much non-combustible residue is left in such component after combustion process. Percentage Ash content was determined by combusting the test-samples left in the crucible after having determined the percentage volatile matter. The crucible containing the test sample was not covered with lid, and the sample was combusted in the furnace at 750° C for 30 min based on ASTM D5468-02. The test samples were withdrawn and cooled. The residue left is the ash content presented in EquationEquation (3)(3)
(3) according to Kuleape et al. (Citation2014).
where is the mass (g) of empty crucible,
is the mass(g) of crucible + test-sample before heating,
is the mass (g) of empty crucible + residue and
, is the ash content.
3.3.3. Determination of Fixed carbon
The percentage composition of the fixed carbon (FC)of each test sample was determined by subtracting the sum of the percentage contents of ash, moisture, and volatile matter from the total percentage as given in EquationEquation (4)(4)
(4) following Ibikunle et al. (Citation2021).
3.3.4. Determination of the HHV or HCV
The HHV or HCV of the MSW fractions was determined, using a digital bomb calorimeter according to Titiladunayo et al. (Citation2018), Ibikunle et al. (Citation2019), and Ibikunle et al., Citation2020aa) based on ASTM D5865M-19 standard (Mushtaq et al., Citation2020). About 0.5 g of the test sample was introduced to the cylinder of the bomb calorimeter and the value of the mass was inputted to the system through a data logger connected to the calorimeter; at high-pressure oxygen ambience, the test sample was combusted. After the combustion is complete, the result was presented on the screen. The typical value (result) of the experiments on the replicated samples was considered as the heating value (MJ/kg) for every sample.
3.4. Statistical methodology
The Linear regression model has been adopted in many studies to model the HHV of municipal solid waste, biomass, and others (García et al., Citation2014; Khuriati et al., Citation2017; Akhtar et al., Citation2017; Özyuguran & Yaman, Citation2017; Nhuchhen & Afzal, Citation2017; Qian et al., Citation2018; Baghban & Shamshirband, Citation2019; Ayinde et al. Citation2020 and Lukman et al. Citation2020b,; Baghban & Ebadi, Citation2018; Ibikunle et al., Citation2020a, Citation2020b and others). The model is defined as follows:
where y is an vector of response variable,
is a known
full rank matrix of predictor or explanatory variables,
is an
vector of unknown regression parameters,
is an
vector of errors such that
and
,
is an
identity matrix.
The parameter in model (1) is commonly estimated using the ordinary least squares (OLS) estimator which is defined in Equationequation (6)
(6)
(6) .
Most work on the modeling of the HHV of MSW earlier mentioned adopted the ordinary least squares estimator. Literature has shown that the predictor variables might be correlated, which gives rise to multicollinearity. The OLS estimator suffers a setback when there is multicollinearity (Lukman & Ayinde, Citation2017; Lukman et al., Citation2019a, Citation2019b). The consequences of multicollinearity to the OLS estimator include large variance, insignificant t-test, wider confidence interval, wrong regression coefficients, and others (Ayinde et al., Citation2018; Dawoud & Kibria, Citation2020; Lukman & Ayinde, Citation2017; Qasim et al., Citation2019). Multicollinearity can be tested using the following methods: variance inflation factor, condition number, condition index, eigenvalues, and others (Aslam & Ahmad, Citation2020; Ibikunle et al., Citation2020b, Citation2020a). Different estimators have been proposed to estimate the parameter when there is multicollinearity. These include the principal component regression estimator (PCRE) by Massy (Citation1965), the ridge estimator by Hoerl and Kennard (Citation1970), the Liu estimator by Liu (Citation1993), the modified Liu estimator by Lukman et al. (Citation2020a), and the K-L estimator by Kibria and Lukman (Citation2020).
The principal components of some of the estimators mentioned above have been combined in some studies. For instance, the r − k class estimator was proposed by combining the ridge estimator (RE) and the PCRE to form a single estimator (Baye & Parker, Citation1984). This new estimator is better than PCRE and RE using the mean-squared error (MSE) criterion. The r-d class estimator was developed by combining the PCRE and the Liu estimator (Kaciranlar & Sakallioglu, Citation2001).
Limited studies on the modeling of MSW have adopted some of these alternative estimators to modeling the high heating values of MSW. It is important to diagnose the model to be free of multicollinearity. Few studies have revealed the possibility of multicollinearity in the regression modeling of HHV values (Ibikunle et al., Citation2020b, Citation2020a; Sun & Chungpaibulpatana, Citation2017). Noori et al. (Citation2009) and Sun and Chungpaibulpatana (Citation2017) employed the principal component regression estimator to forecast MSW in Tehran and Bangkok. Ibikunle et al. (Citation2020a) adopted the ridge regression estimator to model both the ultimate and proximate MSW HHV analysis. Ibikunle et al. (Citation2020b) employed the robust version of the ridge estimator, the Liu and K-L estimator to model the MSW HHV based on the ultimate analysis. There is presently no study where they have combined the principal components with the ridge estimator.
In this study, we will consider the r-k class estimator. The estimator is discussed in the next session.
3.4.1. R-k class estimator
Model (1) is transformed as follows:
where ,
and
is a p
p orthogonal matrix with
where and
are the ordered eigenvalues of
By definition,
is the n
p matrix of the principal components (PCs), where
is the ith PC. Let
contains PCs corresponding to near zero eigenvalues, Z can be portioned as
and
such that
will be eliminated. Model (2.2) can further be written as
The PCR estimator of is
Baye and Parker (Citation1984) defined the r—k class estimator of as
where is the ridge estimator.
For more detail on the principal component procedure employed in this study (see, Saito et al., Citation2001; Sun et al., Citation2015a). The criterion for evaluating the best estimator is the mean-squared error (MSE). The estimator with the lowest MSE is generally preferred.
4. Result and discussion
4.1. Physicochemical analysis of MSW components
The physical characterization of MSW in Ilorin presented in was generated from the seasonal (wet and dry) description of MSW components conducted by Ibikunle et al. (Citation2019) and (Ibikunle et al., Citation2020a). Physicochemical analysis of the eleven (11) combustible fractions, selected out of the 19 MSW components identified in is presented in .
Table 1. Characterization of wet and dry seasons MSW generated in Ilorin (Ibikunle et al., Citation2019)
Table 2. Physicochemical and thermal analyses of the selected MSW combustible fractions
The physicochemical properties of the waste components are obtained from the proximate analysis. shows that the proximate analysis’s highest typical value is possessed by a volatile matter of 37.35%, followed by a fixed carbon of 32.72%, followed by ash content of 12.55%, and moisture content 4.82%. The HHV presented in is considered as an approximate inverse proportion to a WTE power plant (Reddy, Citation2011) and was determined via a bomb calorimeter. The typical heating value of the components was determined to be 24 MJ/kg; nylon fraction has the highest HHV of 46 MJ/kg, followed by poly-sac with about 39 MJ/kg also a plastic bottle with 37 MJ/kg and the least is bones with about 15 MJ/kg. The nylon fraction having the highest HHV could be due to its lowest moisture content of 0.01%, which implies that not much heat energy would be lost to the component’s combustion’s vaporization effect. The bone fraction with the lowest percentage of HHV could be due to its highest percentage of ash (64.7%), which has non-combustible characteristics (Sun et al., Citation2015b).
Wood fraction and trimmings have high moisture contents of about 13% and 10%, respectively, and consequential low heating value of 6.8 and 6.6 MJ/kg correspondingly, compared to 13.8 and 17.15 MJ/kg for plastic bottle and nylon fractions sequentially. This could be because the evaporation period of MSW as fuel was extended, thus, weakening the combustion process; the increase of moisture content would reduce the potential of the heating (Saito et al., Citation2001; Sun et al., Citation2015a).
4.2. Regression modeling
The correlation matrix in revealed that the variables are correlated. The variance inflation factor shows that there is multicollinearity. According to Kibria and Lukman (Citation2020), there is multicollinearity if the VIF is greater than 10. shows that PC1 explains about 67.181% of the total variance while PC2 and PC3 explained about 90.53% and 99.292%, respectively. The percentage variation explained by PC1 is low. Therefore, we decided to select PC2. The value of k is obtained to be 0.0629 with sample size n = 44.
Table 3. Result of correlation coefficient
Table 4. Total variance explained
shows that the performance of the OLS estimator drops when there is multicollinearity. The mean square error of the estimator is very high as compared to the other estimator. PCE and r-k both have a smaller mean-squared error, and the intercept term for both estimators is positive. According to Lukman and Ayinde (Citation2017), one of the consequences of multicollinearity on the OLS estimator is that the coefficient occasionally exhibit a wrong sign. For instance, the intercept term of OLS estimator in this study is negative while ridge and r-k estimators show a positive coefficient. The result for the principal component is not too far from the OLS estimate. It is evident that combining the principal component with ridge provides a great improvement to the result. The positive intercept terms show that some other estimators can positively influence high heating values that are not considered in this study while the OLS estimator says something contrary. However, we recommended the r-k class estimator for efficiently modeling because it possesses the smallest mean-squared error. The regression model is as follows:
Table 5. Regression estimates using different estimators
Every one-unit increase in volatile matter and fixed carbon, heating values increased by about 21% and 36%, respectively. For every one-unit increase in ash and moisture, heating values decrease by about 0.2% and 40%, respectively. The result agrees with the findings of the following study (Saito et al., Citation2001; Sun et al., Citation2015a; Adekunle et al., Citation2015; Motghare et al., Citation2016). The result in revealed that r-k class estimator helps to efficiently model the HHV. are the graph of the actual value of HHV against the respective predicted value produced by each estimator. In terms of prediction, shows that the r-k estimator gave a better prediction in terms of the coefficient of determination (R2) followed by the ridge estimator.
5. Conclusion
The ordinary least squares estimator (OLSE) is famous for modeling municipal solid waste’s high heating values. A recent study has shown that the variables that act as predictors for the HHV can be correlated a condition called multicollinearity. When this happens, the efficiency of the OLSE breakdown by possessing high variance. Some alternative estimators are available as alternatives to the OLSE. These include the principal component regression estimator, the ridge estimator, and the r-k class estimator. In this study, we build a linear regression model based on the proximate analysis of municipal solid waste to predict the HHV. The combustible waste components’ heating value is the dependent variable, while the following variables are the predictors: Volatile matter, ash, moisture, and fixed carbon. We diagnosed the model and observed that the predictor variables are related (multicollinearity exists). Thus, the conventional OLSE will not provide a reliable estimate. Hence, we estimate using a few alternative robust estimators and judge their performances using the mean-squared error. The estimator with the minimum mean-squared error is generally preferred. The MSE of OLSE, ridge, PCE, and r-k are as follows: 581.84, 2.56, 523.69, and 0.239, respectively. The result shows that the r-k class estimator outperforms other estimators because it possesses the minimum mean-squared error. We provide a more efficient method of estimating the regression coefficients of the ultimate-based model of the HHV of Ilorin MSW. In a future study, we will combine the principal components with machine-learning tools such as the artificial neural network, support vector regression, and random forest regression.
Credit authorship contribution statement
1. R. A. Ibikunle: Conceptualization, Investigation, Methodology, Writing - original draft.
2. A. F. Lukman: Methodology, modeling and Writing - original draft.
3 I. F. Titiladunayo: Supervision of the Experimentation and editing of the original draft.
4. H. Abdul-Rahaman: Reviewing and Editing.
Acknowledgements
The author is very grateful to the technologists in the central Laboratory of Landmark University Omu Aran, for the help rendered during the experimentation processes.
Disclosure statement
No potential conflict of interest was reported by the author(s).
Additional information
Funding
Notes on contributors
Rotimi Adedayo Ibikunle
Some of the authors are members of SDG 7, the research cluster of Landmark University and teamwork with some foreign faculty. This multidisciplinary research synergy is to tackle challenges militating against the welfare and development of our surrounding environment. The authors have been researching energy recovery from waste for 7 years now. They have predicted the energy and power potentials of the waste generated in their cities and developed a model to predict the heating value, using ultimate analysis. The authors have developed software to predict the energy and power potential of MSW when the quantity of waste to be converted and the corresponding heating value is known. The software can also predict the capacity of the steam power plant required for WTE. As part of the contribution to enable Nigeria’s Renewable Energy Master Plan to achieve the goals set for 2025, a research topic titled “Modeling energy content of municipal solid waste based on proximate analysis: r-k class estimator approach” was developed.
References
- Abdellah, A. M., & Balla, Q. I. (2013). Domestic solid waste management and its impacts on human health and the environment in Sharg El Neel locality, Khartoum State, Sudan. The Journal of Biological Sciences, 16(22), 1538–20. https://doi.org/10.3923/pjbs.2013.1538.1544
- Abhishek, S., Anil, K. G., Brajesh, D., & Makrand, M. G. (2021). Seasonal characterization of municipal solid waste for selecting feasible waste treatment technology for Guwahati city, India. Journal of the Air and Waste Management Association 72 (2) 147–160 . https://doi.org/10.1080/10962247.2021.1980450
- Adekunle, J. O., Ibrahim, J. S., & Kucha, E. I. (2015). Proximate and ultimate analyses of biocoal briquettes of nigerian’s ogboyaga and okaba sub-bituminous coal. British Journal of Applied Science & Technology, 7(1), 114–123. https://doi.org/10.9734/BJAST/2015/15154
- Ajadi, B. S., & Tunde, A. M. (2010). Spatial variation in solid waste composition and management in Ilorin Metropolis, Nigeria. International Journal of Human Ecology, 32(2), 101–106. https://doi.org/10.1080/09709274.2010.11906327
- Akdag, A. S., Atimtay, A., & Sanin, F. D. (2016). Comparison of fuel value and combustion characteristics of two different rdf samples. Waste Management, 47, 217–224. https://doi.org/10.1016/j.wasman.2015.08.037
- Akhator, E. P., Obanor, A. I., & Igbinomwanhia, D. I. (2016). Thermal analysis of small-scale solid waste-fired steam boiler for power generation in benin city, nigeria. Nigerian Journal of Technology, 3(3), 555–561. https://doi.org/10.4314/njt.v35i3.13
- Akhtar, J., Sheikh, N., & Munir, S. (2017). Linear regression-based correlations for estimation of high heating values of Pakistani lignite coals. Energy Sources, Part A: Recovery, Utilization, and Environmental Effects, 39(10), 1063–1070. https://doi.org/10.1080/15567036.2017.1289283
- Alkasrawi, M. 2004. Development of simultaneous saccharification and fermentation for production of ethanol from softwood. Lund University.
- Amin, K., & Yang, G. S. (2012). Identification of the municipal solid waste characteristics and potential of plastic recovery 5 (7) . https://doi.org/10.5539/jsd.v5n7p11
- Arif, R. S., Bambang, S., Hamzah, F., & Oki, M. (2019). Syngas production from municipal solid waste with a reduced tar yield by three-stages of air inlet to a downdraft gasifier doi:10.1016/j.fuel.2019.116509.
- Aslam, M., & Ahmad, S. (2020). The modified Liu-ridge-type estimator: A new class of biased estimators to address multicollinearity. Communications in Statistics - Simulation and Computation, 1–20. https://doi.org/10.1080/03610918.2020.1806324
- Ayinde, K., Lukman, A. F., Alabi, O. O., & Bello, H. A. (2020). A new approach of principal component regression estimator with applications to collinear data. International Journal of Engineering Research and Technology, 13(7), 1616–1622. https://doi.org/10.37624/IJERT/13.7.2020.1616-1622
- Ayinde, K., Lukman, A. F., Samuel, O. O., & Ajiboye, S. A. (2018). Some new adjusted ridge estimators of linear regression model. International Journal of Civil Engineering and Technology, 9(11), 2838–2852.
- Ayodele, T. R., Ogunjuyigbe, A. S. O., & Alao, M. A. (2017). Life cycle assessment of waste-to-energy (WTE) technologies for electricity generation using municipal solid waste in Nigeria. Applied Energy, 201, 200–218. http://dx.doi.org/10.1016/j.apenergy.2017.05.097
- Baghban, A., & Ebadi, T. (2018). GA-ANFIS modeling of higher heating value of wastes: Application to fuel upgrading. Energy Sources, Part A: Recovery, Utilization, and Environmental Effects, 41(1), 1–7. https://doi.org/10.1080/15567036.2017.1344746
- Baghban, A., & Shamshirband, S. (2019). On the estimation of higher heating value of municipal wastes using soft computing approaches. Energy Sources, Part A: Recovery, Utilization, and Environmental Effects, 1–9. https://doi.org/10.1080/15567036.2019.1645764
- Baye, M. R., & Parker, D. F. (1984). Combining ridge and principal component regression: A money demand illustration. Communications in Statistics: Theory and Methods, 13(2), 197–205. https://doi.org/10.1080/03610928408828675
- Beyene, H. D., Werkneh, A. A., & Ambaye, T. G. (2018). Current updates on waste to energy (WTE) technologies: A review. Renewable Energy Focus, 24, 1–11. https://doi.org/10.1016/j.ref.2017.11.001
- Bosmans, A., Vanderreydt, I., Geysen, D., & Helsen, L. (2013). The crucial role of waste-to-energy technologies in enhanced landfill mining: A technology review. Journal of Cleaner Production, 55, 10–23. https://doi.org/10.1016/j.jclepro.2012.05.032
- Chhotu, R., Amit, K., & Pushpa, R. (2021). Municipal solid waste management: a review of waste to energy (wte) approaches. BioResources, 16(2 4275–4320).
- Cordero, T., Marquez, F., Rodriguez-Mirasol, J., & Rodriguez, J. J. Predicting heating values of lignocellulosic and carbonaceous materials from proximate analysis. (2001). Fuel, 11(11), 1567–1571. https://doi.org/10.1016/S0016-2361(01)00034-5
- Dawoud, I., & Kibria, B. M. G. (2020). A new biased estimator to combat the multicollinearity of the Gaussian linear regression model. Stats, 3(4), 526–541. https://doi.org/10.3390/stats3040033
- Demirbas, A. (2003). Relationships between heating value and lignin, fixed carbon, and volatile material contents of shells from biomass products. Energy Source, 25(7), 629–635. https://doi.org/10.1080/00908310390212336
- EC SWA-Tool.2004. European Commission solid waste tool: Development of a methodology tool to enhance the precision and comparability of solid waste analysis data. http://www.wastesolutions.org. Accessed 12 Dec 2016
- Erol, M., Haykiri-Acma, H., & Kucukbayrak, S. (2010). calorific value estimation of biomass from their proximate analyses data. Renewable Energy, 35(1), 170–173. https://doi.org/10.1016/j.renene.2009.05.008
- García, R., Pizarro, C., Lavín, A. G., & Bueno, J. L. (2014). Spanish biofuels heating value estimation. Part II: Proximate analysis data. Fuel, 117, 1139–1147. https://doi.org/10.1016/j.fuel.2013.08.049
- Ghugare, S. B., Tiwary, S., Elangovan, V., & Tambe, S. S. (2014). Prediction of higher heating value of solid biomass fuels using artificial intelligence formalisms. Bioenergy Research, 7(2), 681–692. https://doi.org/10.1007/s12155-013-9393-5
- Haller, M. Y., Carbonell, D., Dudita, M., Zenhäusern, D., & Häberle, A. (2020). Seasonal energy storage in aluminium for 100 percent solar heat and electricity supply. Energy Conversion and Management, 5, 100017 doi:10.1016/j.ecmx.2019.100017.
- Hoerl, A. E., & Kennard, R. W. (1970). Ridge regression: Biased estimation for nonorthogonal problems. Technometrics, 12(1), 55–67. https://doi.org/10.1080/00401706.1970.10488634
- Ibadan Electricity Distribution Company (IBEDC), 2016. Kwara needs 270 MW for 24-hour electricity. Available from: https://www.ilorin.info/fullnews.php?id=18082 03 October 2018)
- Ibikunle, R. A., Lukman, A. F., Titiladunayo, I. F., Akeju, E. A., & Dahunsi, S. O. (2020b). Modeling and robust prediction of high heating values of municipal solid waste based on ultimate analysis. Energy Sources, Part A: Recovery, Utilization, and Environmental Effects, 1–18. https://doi.org/10.1080/15567036.2020.1841343
- Ibikunle, R. A., Titiladunayo, I. F., Akinnuli, B. O., Dahunsi, S. O., & Olayanju, T. M. A. (2019). Estimation of power generation from municipal solid wastes: A case study of Ilorin metropolis, Nigeria. Energy Reports, 5, 126–135. https://doi.org/10.1016/j.egyr.2019.01.005
- Ibikunle, R. A., Titiladunayo, I. F., Akinnuli, B. O., Lukman, A. F., Ikubanni, P. P., & Agboola, O. O. (2018). Modelling the energy content of municipal solid waste and determination of its physiochemical correlation using multiple regression analysis. International Journal of Mechanical Engineering Technology, 9(11), 220–232.
- Ibikunle, R. A., Titiladunayo, I. F., Dahunsi, S. O., Akeju, E. A., & Osueke, C. O. (2021). Characterization and projection of dry season municipal solid waste for energy production in Ilorin metropolis, Nigeria. Waste Management & Research, 1–10. https://doi.org/10.1177/0734242X2098
- Ibikunle, R. A., Titiladunayo, I. F., Lukman, A. F., Dahunsi, S. O., & Akeju, E. A. (2020). Municipal solid waste sampling, quantification and seasonal characterization for power evaluation: Energy potential and statistical modelling. Fuel, 277, 1. https://doi.org/10.1016/j.fuel.2020.118122
- Ibikunle, R. A., Titiladunayo, I. F., Lukman, A. F., Dahunsi, S. O., & Akeju, E. A. (2020a). Municipal solid waste sampling, quantification and seasonal characterization for power evaluation: Energy potential and statistical modelling. Journal of Fuels, 277(1), 118122. https://doi.org/10.1016/j.fuel.2020.118122
- Issam, A., Maria, M., Abdul, S. F., Abu, Z., Hafez, Q. S., & Despo, K. (2010). Solid waste characterization, quantification, and management practices in developing countries. Journal of Environmental Management, 91(5), 1131–1138. https://doi.org/10.1016/j.jenvman.2010.01.003
- Kaciranlar, S., & Sakallioglu, S. (2001). Combining the Liu estimator and the principal component regression estimator. Communications in Statistics - Theory and Methods, 30(12), 2699–2705. https://doi.org/10.1081/STA-100108454
- Kaza, S., Yao, L. C., Bhada-Tata, P., & Van Woerden, F. (2018). What a waste 2.0: a global snapshot of solid waste management to 2050. World Bank.
- Khuriati, A., Setia, B. W., Nur, M., Istadi, I., & Suwoto, G. (2017). Modeling of heating value of municipal solid waste Based on ultimate analysis using multiple stepwise regression linear in Semarang. ARPN Journal of Engineering and Applied Sciences, 12(9), 2870–2876.
- Khuriati, A., Setiabudi, W., Nur, M., & Istadi, I. (2015). Heating value prediction for combustible fraction of municipal solid waste in Semarang using backpropagation neural network. AIP Conference Proceedings, 1699, 030028. https://doi.org/10.1063/1.4938313
- Kibria, G. B. M., & Lukman, A. F. (2020). A new ridge-type estimator for the linear regression model: simulations and applications. Scientifica, 2020, 1–16. https://doi.org/10.1155/2020/9758378
- Küçükbayrak, S., Dürüs, B., Meríçboyu, A. E., & Kadioglu, E. (1991). Estimation of calorific values of Turkish lignites. Fuel, 70(8), 979–981. https://doi.org/10.1016/0016-2361/2891/2990054-E
- Kuleape, R., Cobinna, S. J., Danpare, S. B., Duwiejuah, A. B., Amoako, E. E., & Asare, W. (2014). Assessment of the energy recovery potentials of solid waste generated in Akosombo, Ghana. Africa Journal Environmental Science and Technology, 8(5), 297–305. https://doi.org/10.5897/AJEST2014.1663
- Laith, A., Hadidi, L., & Omer, M. M. (2017). A financial feasibility model of gasification and anaerobic digestion waste-to-energy (WTE) plants in Saudi Arabia. Waste Manage, 59, 90–101. https://doi.org/10.1016/j.wasman.2016.09.030
- Lawal, A. D., Joseph, E., & Rufai, A. I. (2014). Characteristics and composition analysis of municipal solid waste in Kano, Nigeria. International Journal of Scientific and Engineering Research, 9, 972–975.
- Liu, K. (1993). A new class of biased estimate in linear regression. Communications in Statistics, 22(2), 393–402. https://doi.org/10.1080/03610929308831027
- Lukman, A. F., & Ayinde, K. (2017). Review and Classifications of the ridge parameter estimation techniques. Hacettepe Journal of Mathematics and Statistics, 46(5), 953–967. https://doi.org/10.15672/HJMS.201815671
- Lukman, A. F., Ayinde, K., Binuomote, S., & Onate, A. C. (2019a). Modified ridge-typeestimator to combat multicollinearity: Application to chemical data. Journal of Chemometrics, 33(5), e3125. https://doi.org/10.1002/cem.3125
- Lukman, A. F., Ayinde, K., Oludoun, O., & Onate, C. (2020b). Combining modified ridge type and principal component regression estimators. Scientific African, 9, e00536. https://doi.org/10.1016/j.sciaf.2020.e00536
- Lukman, A. F., Ayinde, K., Sek, S. K., & Adewuyi, E. A. (2019b). Modified new two-parameter estimator in a linear regression model. Modelling and Simulation in Engineering, 2019, 1–10. https://doi.org/10.1155/2019/6342702
- Lukman, A. F., Kibria, G. B. M., Ayinde, K., & Jegede, S. L. (2020a). Modified one-parameter liu estimator for the linear regression model. Modelling and Simulation in Engineering, 9574304. https://doi.org/10.1155/2020/9574304
- Massy, F. (1965). Principal components regression in exploratory statistical research. Journal of the American Statistical Association, 60(309), 234–266. https://doi.org/10.1080/01621459.1965.10480787
- Motghare, K. A., Rathod, A. P., Wasewar, K. L., & Labhsetwar, N. K. (2016). Comparative study of different waste biomass for energy application (Waste Management). https://doi.org/10.1016/j.wasman.2015.07.032
- Mushtaq, J., Dar, A. Q., & Ahsan, N. (2020). Spatial–temporal variations and forecasting analysis of municipal solid waste in the mountainous city of north-western Himalayas. Springer Nature. https://doi.org/10.1007/s42452-020-2975-x
- Nhuchhen, D. R., & Afzal, M. T. (2017). HHV predicting correlations for torrefied biomass using proximate and ultimate analyses. Bioengineering, 4(1), 7. https://doi.org/10.3390/bioengineering4010007
- Nnodim, O. 2016: ‘Power generation crashes to 1,400MW’, punch newspaper, 18 May. http://www.punchng.com/powergenerationcrashes1400mwtcn/. (Accessed 05 January 2020)
- Noori, R., Abdoli, M. A., Jalili Ghazizade, M., & Samieifard, R. (2009). Comparison of neural network and principal component-regression analysis to predict the solid waste generation in tehran. Iranian Journal of Public Health, 38(1), 74–84.
- NT ENVR-01 (Nordtest Method, 2005) Solid waste municipal sampling and characterization. (2005) Published by nordtest tekniikantie 12, FIN—02150 ESPOO FINLAND http://www.nordtest.info/index.php/methods/item/solid-waste-municipal-samplingand-characterisation-nt-envir-001.html Accessed 07 July 2015
- Omari, A. M. 2015. Characterization of Municipal solid waste for energy recovery. A case study of Arusha, Tanzania. https://www.researchgate.net/publication/281620004_Characterization_of_Municipal_solidwaste_for_energy_recovery. (Accessed 05 January 2020)
- Omari, A., Said, M., Njau, K., John, G., & Mtul, P. (2014). Energy recovery routes from municipal solid waste, A case study of Arusha-Tanzania. International Journal of Energy Technology and Policy.
- Özyuguran, A., & Yaman, S. (2017). Prediction of calorific value of biomass from proximate analysis. Energy Procedia, 107, 130–136. https://doi.org/10.1016/j.egypro.2016.12.149
- Parikh, J., Channiwala, S. A., & Ghosal, G. K. (2005). A correlation for calculating HHV from proximate analysis of solid fuels. Fuel, 84(5), 487–494. https://doi.org/10.1016/j.fuel.2004.10.010
- Psomopoulos, C. S., Bourka, A., & Themelis, N. J. J. (2009). Waste-to-energy: A review of the status and benefits in USA. Waste Management, 5(5), 1718–1724. https://doi.org/10.1016/j.wasman.2008.11.020
- Qasim, M., Amin, M., & Omer, T. (2019). Performance of some new Liu parameters for the linear regression model. Communications in Statistics - Theory and Methods 49 17 4178–4196 . https://doi.org/10.1080/03610926.2019.1595654
- Qian, X., Lee, S., Soto, A., & Chen, G. (2018). Regression model to predict the higher heating value of poultry waste from proximate analysis. Resources, 7(3), 39. https://doi.org/10.3390/resources7030039
- Reddy, P. J. (2011). Municipal solid waste management: processing energy recovery global examples. BS Publications.
- Reza, E., Mehdi, B., & Behrooz, G. (2021). Technoeconomic feasibility review of hybrid waste to energy system in the campus: A case study for the University of Victoria. Renewable and Sustainable Energy Reviews, 146, 111190. https://doi.org/10.1016/j.rser.2021.111190
- Saito, M., Amagai, K., Ogiwara, G., & Arai, M. (2001). Combustion characteristics of waste material containing high moisture. Fuel, 80(9), 1201–1209. https://doi.org/10.1016/S0016-2361(00)00208-8
- Salameh, T., Tawalbeh, M., Al-Shannag, M., Saidan, M., Melhem, K. B., & Alkasrawi, M. (2020). Energy saving in the process of bioethanol production from renewable paper mill sludge. Energy, 196, 117085. https://doi.org/10.1016/j.energy.2020.117085
- Sharma, M., & McBean, E. A. (2007). A methodology for solid waste characterization based on diminishing marginal returns. Journal of Waste Management, 27(3), 337–344. https://doi.org/10.1016/j.wasman.2006.02.007
- Sheng, C., & Azevedo, J. L. T. (2015). Estimating the higher heating value of biomass fuels from basic analysis data. Biomass & Bioenergy, 28(5), 499–507. https://doi.org/10.1016/j.biombioe.2004.11.008
- Shi, H., Maphinpey, N., Aqsha, A., & Silbermann, R. (2016). Characterization, thermochemical conversion studies and heating value modeling of municipal solid waste. Journal of Waste Management, 48, 34–47.
- Slavko, D., Jozsef, V., Stavra, K., Maria, L., Vassilis, I., Christos, V., Lulian, M., Nona, A., & Lucia, I. 2012. Sampling and analysis of solid municipal waste in Balkan region: The frst results and their significance. Natural Technology University of Anthens, School of Chemical Engineering, UEST 9, Heroon Polytechniou Street, Zographou Campus. https://www.iswa.org/uploads/tx_iswaknowledgebase/Moustakas.pdf. Accessed 15 Sep 2019
- Smallbone, A., Jia, B., Atkins, P., & Roskilly, A. P. (2020). The impact of disruptive powertrain technologies on energy consumption and carbon dioxide emissions from heavy-duty vehicles. Energy Conversion and Management, 6, 100030 doi:10.1016/j.ecmx.2020.100030.
- song, Q., Zhao, H., Jia, J., Yang, L., Ly, W., Bao, J., Shu, X., Gu, Q., & Zhang, P. (2020). Pyrolysis of municipal solid waste with iron-based additives: A study on the kinetic, product distribution and catalytic mechanisms. Journal of Cleaner Production, 258, 120682. https://doi.org/10.1016/j.jclepro.2020.120682
- Sudhir, K., Venkata, S., & Prasada, R. (2010) Waste – To – energy: a case study of Eluru City, Andra Pradesh. International Journal of Environmental Sciences, 1 151–162 2
- Sun, N., & Chungpaibulpatana, S. (2017). Development of an appropriate model for forecasting Municipal Solid waste generation in Bangkok. Energy Procedia, 138, 907–912. https://doi.org/10.1016/j.egypro.2017.10.134
- Sun, R., Ismail, T. M., Ren, X., & Abd El-Salam, M. (2015a). Numerical and experimental studies on effects of moisture content on combustion characteristics of simulated municipal solid wastes in a fixed bed. Waste Management, 39, 166–178. https://doi.org/10.1016/j.wasman.2015.02.018
- Sun, R., Ismail, T. M., Ren, X., & Abd El-Salam, M. (2015b). Effect of ash content on the combustion process of simulated MSW in the fixed bed. Waste Management, 48 236–249 . https://doi.org/10.1016/j.wasman.2015.10.007
- SWA-Tool, E. 2004. European commission solid waste tool: Development of a methodology tool to enhance the precision and comparability of solid waste analysis data: www.wastesolutions.org. [Accessed 12 December 2016]
- SWM (2015). Swedish waste management. www.avfallsverige.se/fileadmin/uploads/Rapporter/SWM_2015.pdf. (Accessed 04 January 2020)
- Titiladunayo, I. F., Akinnuli, B. O., Ibikunle, R. A., Agboola, O. O., & Ogunsemi, B. T. (2018). Analysis of combustible municipal solid waste fractions as fuel for energy production: Exploring its physicochemical and thermal characteristics. International Journal of Civil Engineering and Technology, 9, 1557–1575.
- Tozlu, A., Ozahi, E., & Abusoglu, A. (2016). Waste to energy technologies for municipal solid waste management in Gaziantep. Renewable and Sustainable Energy Reviews, 54, 809–815. https://doi.org/10.1016/j.rser.2015.10.097
- Uchendu, C. (2008). Municipal solid waste treatment and recycling technologies for developing countries: A typical Nigeria case study. Journal of Solid Waste Treatment and Management, 34, 127–135.
- US EPA, 2018: National overview: facts and figures on materials, wastes and recycling (combustion with energy recovery). https://www.epa.gov/facts-and-figures-about-materials-waste-and-recycling/national-overview-facts-and-figuresmaterials#:~:text=In%202018%2C%2034.6%20million%20tons (Accessed 05 January 2020)
- Vairam, S., & Ramesh, S. (2013). Engineering Chemistry. John Wiley and sons Ltd.
- Yin, C. Y. (2011). Prediction of higher heating values of biomass from proximate and ultimate analyses. Fuel, 90(3), 1128–1132. https://doi.org/10.1016/j.fuel.2010.11.031
- Zhang, J., Kan, X., Shen, Y., Loh, K. C., Wang, C. H., Dai, Y., & Tong, Y. W. (2018). A hybrid biological and thermal waste-to-energy system with heat energy recovery and utilization for solid organic waste treatment. Energy, 152, 214–222. https://doi.org/10.1016/j.energy.2018.03.143
- Zhou, H., Meng, A. H., Long, Y. Q., Li, Q. H., & Zhang, Y. G. (2014). An overview of characteristics of municipal solid waste fuel in china. physical. chemical composition and heating value. Renewable And Sustainable Energy Reviews, 36, 107–122. https://doi.org/10.1016/j.rser.2014.04.024