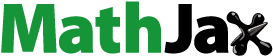
Abstract
Studies reviewed in this paper show anomaly for temperature pertaining to streamflow and rainfall showing different trends, especially in Ethiopia to support the research findings and interpretation. There are many hydrological models, including 54 physically distributed, lumped, and conceptual hydrological models, of which 28 have been used in Ethiopian river basins. The models include the most adaptable and commonly used SWAT model applicable from small areas up to large basins. It is indeed a challenge to use a single hydrological model as the data rely on consistency, limitation-free, and exactly fitted output. The overall performance of individual physically-based, conceptual, and machine learning (ML) models varied at different watersheds. Reasonably, ML performs very well, up to 0.99 for R2 and NSE and up to 0.001 for PBIAS. Inopportunely, using a single hydrological model has its limitations; ensemble multi-individual models, coupling or hybridization of physical or conceptual models with machine learning, combining evolutionary optimization algorithms with ML, and also comparisons of multi-single hydrological models, and selecting the best one are recommended options. No single hydrological model is indispensable and can be termed as better than the other for any watershed. Somewhat, ML outperforms SWAT but cannot be considered an absolute substitute. The size of the watershed, the number of data used, and the ratio between calibrations year to validation year do not have a clear correlation with the performance, particularly for the SWAT model accounted for in this review. Optimization algorithms explore multiple options and choosing the right one is a tedious task before a final decision is taken.
REVIEWING EDITOR:
1. Introduction
Water, defined as an essential natural resources, is considered to be finite and subject to change under various circumstances. Droughts and floods also pose hazards to society under unpredictable scenarios (Van Loon, Citation2015; Cenobio-Cruz et al., Citation2023; Nivesh et al., Citation2022). Planning, policymakers, and managers need to know the amount of streamflow to effectively allocate water resources in the watersheds they are responsible for (Alamdari et al., Citation2017). In most developing and impoverished countries, direct streamflow monitoring is quite challenging due to lack of funds and inadequate equipment. As a result, on the condition where stream flow has not been recorded, the hydrological model is obligatory to estimate the water availability at the relevant location and real time (Sanjay Shekar & Vinay, Citation2021; Tibangayuka et al., Citation2022).
The hydrological model can partially or fully represent the water cycle and can interpolate and extrapolate the hydrological cycle components of catchments both spatially and temporally (Fniguire et al., Citation2022). Events and patterns related to stream flow are hard to predict. This is because, stream flow is incredibly complicated, dynamic, non-stationary, and non-linear (Yaseen et al., Citation2015). Many water professionals worldwide are meticulous in reducing the uncertainty of hydrological models used for determining stream flow discharge to enhance the sustainable management and development of water resources (Sempewo et al., Citation2022).
The estimated stream flow or runoff from a catchment is useful for many purposes, such as determining flood peaks, determining the availability of water for municipal needs, designing storage facilities for a range of uses, organizing irrigation operations for agricultural or other industrial uses, protecting wildlife, and securing future supplies of potable water (Ranjan & Singh, Citation2022). The proficiency and accuracy of flow prediction models can have a direct impact on management decisions about water resources (Noori & Kalin, Citation2016). Although, there are many hydrological models available to forecast current and future stream flow, choosing a specific model is a challenge because of the uncertainty in model prediction caused by input factors (climate and land use), model parameters, consideration of scale in watershed modeling, and methods of calibration (Veettil et al., Citation2021).
The ideal model uses the fewest parameters and the least amount of model complexity while producing results that are very similar to reality (Suliman et al., Citation2015). To avoid needless costs resulting from poorly informed predicted planning, decision-makers must rely on reliable hydrological modeling results (Mubialiwo et al., Citation2021).
2. Effect of climate on the catchments hydrology response
Climate change, which has laid down attention recently, is one of the major global challenges of the 21st century by altering the catchment hydrological process (Shahid et al., Citation2018; Merga et al., Citation2022; Kamilia et al., Citation2024). Based on scientific pieces of evidence, an increase in greenhouse gas emissions might cause an average temperature rise of 1.4–5.8 °C by the end of the century. There will be noticeable repercussions from climate change, particularly in poorer countries where agriculture is a significant economic factor (Worqlul et al., Citation2018). Currently, there are many climate effects on catchments across both developing countries, including Ethiopia and developed countries.
Using the Liuxihe (LXH) model, Sheng et al. (Citation2023) evaluated how the hydrology of the Jinshan River (455,000 km2) in China’s upper Yangtze River basin has been affected by climate change. His findings showed that, for various global climate models, rainfall and temperature will rise by 0.7% to 11% and 1.0 to 2.1, respectively, over the next 30 years (up to 2050) in comparison to the base period. Runoff variations, on the other hand, show greater uncertainty and range from −5.6% to 11.0% without any discernible pattern. Not every increase in precipitation causes runoff to alter in the same way.
In the Huaihe River Basin (123,900 km2) in China, Bian et al. (Citation2023) forecasted future changes in the flood regime using the Coupled model inter-comparison project (CMIP6) and the Xinanjiang hydrological model. The results demonstrated that there will likely be an increase in the average intensity, quantity, and extreme precipitation annually, as well as significant future increases in the average, maximum, and lowest temperature values. In the distant future (2071–2100), the annual peak flood is similarly predicted to rise by 46.4% (43.1%) and 45.4% (51.1%), respectively, at Wangjiaba station and Wujiadu station, based on the shared socioeconomic pathways (SSP5-8.5) scenario. Therefore, the findings of this study suggest that a technical body in the country should take necessary measures to minimize the disasters caused at the time of increased peak floods.
N. M. Reddy et al. (Citation2023) used a conceptual lumped model called the Sacramento model with CMIP6 to study the effects of climate change on streamflow in the Godavari basin (5380 km2), India. According to the findings, there will be an increase in annual precipitation ranging from 9% to 55.0% in all SSP126, SSP245, SSP370, and SSP585 scenarios, as well as in all near, mid, and far future years. The SSP585 scenario is probably going to analyze the biggest future increase in precipitation. In the near future, the stream flow will also increase minimally by 47.12% and up to 127.48% in the distant future.
Using HEC-HMS, Lone (2022) determined the effects of hydro-climatic changes in the Sindh River in the Kashmir basin (1560.32 km2) and concluded that, while the maximum temperature in the spring will continue to decline in the near and far future, it will also decline in the near future during the winter. In contrast, the other three seasons will see an increase in the near and far future in both RCP4.5 and RCP8.5. The lowest temperature will decrease in the winter and spring and increase in the summer and autumn for both RCP4.5 and RCP8.5, as well as for both the near and distant future. In both the near and distant future, at RCP4.5 and RCP8.5, the amount of rainfall during the spring will decline while that of the other three seasons will rise. The yearly streamflow will rise in both the near future and long term in both RCP4.5 and RCP8.5, with a range of 2.8% to 18.3% in all three gauge sites. Nevertheless, because this study only used one general circulation model (GCM), the conclusions cannot be fully authenticated.
Using the SWAT model, Nilawar and Waikar (Citation2018) determined how the Purna River basin (15,579 km2) of India is impacted by the response to climate change. The outcome showed that the maximum temperature will rise by 0.05 to 1.46 °C and that in the near and distant future, RCP8.5 will have a value that is comparatively larger than RCP4.5. Under RCP4.5 and RCP8.5, respectively, the anticipated average annual precipitation (PCP) increases by 1.22–5.59% (10.81 mm to 49.47 mm) and 2.79–12.29% (24.67 mm to 108.63 mm). In RCP4.5 and RCP8.5, as well as in all subsequent periods, the rainy season will rise and the dry season will fall. In one of the Congo River Basin’s big watersheds, under RCP4.5, the average percent change in annual discharge for the near, mid, and far futures are −1.8%, −6.1%, and −6.0%, respectively. Under RCP8.5, there will be a corresponding decline of −3.1%, −3.9%, and 0.25% for the near, mid, and far futures, respectively (Karam et al., Citation2023).
The research evaluated in this paper indicates that the hydrological response varies with percentage change as well as trend change (increasing or decreasing) on the impact of climate change on future hydro-climatological reaction, which includes temperature, streamflow, and rainfall. The temperature will rise in almost every basin, with a few notable exceptions and seasonal variations.
Ethiopia has been considered as an example in this review paper to highlight how the climate may affect future hydrological responses. Research has been conducted across the nation’s river basins to analyze the effects of climate change on the hydrological response in the future (). The works of literature considered in this review were; Dibaba et al. (Citation2020) on the Fincha Abay basin, Merga et al. (Citation2022) on the Dhidessa/Upper Blue Nile basin, Gebre and Ludwig (Citation2017) on the Gilgel Abay/Upper Blue Nile, Eshete et al. (Citation2022) on the Megech/Upper Blue Nile, Gizaw et al. (Citation2021) on the Upper Awash river basin, Genale Dawa, Baro river basin, Bekele et al. (Citation2019) on the Keleta/Awash River basin, Gebrechorkos et al. (Citation2020) on the Awash (61,032 km2), Fita and Abate (Citation2022) on the Melka Wakana, Fentaw et al. (Citation2018) on the Tekeze river basin, Orkodjo et al. (Citation2022) on the Omo river basin, Mekonnen et al. (Citation2023) and Akirso et al. (Citation2022) on the Gidabo river/Rift valley lake basin, Edamo et al. (Citation2022) and Kuma et al. (Citation2021) on the Bilate River/Rift valley lake basin, Abdule et al. (Citation2023) on the Yado/Genale Dawa river basin.
Figure 1. (a) Annual maximum temperature (); (b) Annual rainfall; (c) Annual streamflow change in Ethiopian river basins respectively.
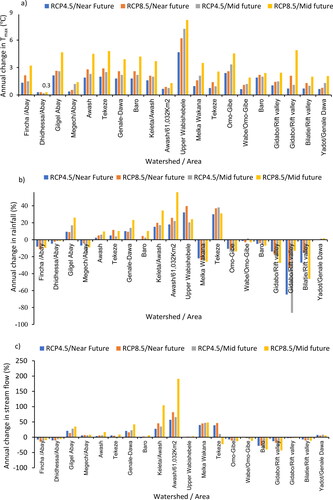
Based on the review articles taken into consideration for this review, the temperature will rise in all scenarios in both the mid and long-term future with a maximum change of up to 8.2 The yearly rainfall and outflow, however, indicate an inconsistent pattern moving forward. Rainfall and streamflow will increase in certain areas of the watershed, such as the Awash River basin, while they will drop in other areas, such as the Gidabo and Bilate rivers of the Rift Valley Lake Basin.
Furthermore, different researchers have come to differing results about how hydrology will be impacted by climate change; using different hydrological models could be one reason for this. For example, Mengistu et al. and Gizaw et al. (Citation2021) have used SWAT and HSPF models, respectively, and assessed the influence of climate on the hydrological response of the Baro River basin. According to Mengistu et al. (2023), there will be a decline in the annual streamflow in the far and near future. Conversely, Gizaw et al. (Citation2021) predicted an increase in streamflow in the near and far future. Akirso et al. (Citation2022) and Mekonnen et al. (Citation2023) also used HEC-HMS and SWAT to evaluate the impact of climate change on the hydrology of the Gidabo watershed (Rift Valley Lakes Basin), respectively. According to them, there will likely be a future decrease in annual stream flow and precipitation; however, under RCP 8.5 and in the distant future, the magnitude of change remains extremely high (−5.2% and −46.84%, respectively). The results of Kuma et al. (Citation2021) and Edamo et al. (Citation2022) studied in the Billate/Rift Valley using SWAT and HEC-HMS in RCP8.5 and RCP4.5 showed that future annual stream flow and rainfall would decrease; on the other hand, future annual stream flow and rainfall would increase and decrease, respectively (Edamo et al., Citation2022).
In summary, the anticipated hydrological reaction to climate change varies from one watershed to another, among models, and simultaneously among authors. It is difficult to get reliable interpretations and information because of the variance in predictions between models and researchers. According to the Ethiopian basin’s climatic impact on future hydrology findings, the upper Blue Nile River basin’s Dhidessa watershed will see nearly zero temperature change, while the Wabishebele River basin will have an 8.2 °C temperature shift. Gidabo may have a loss of up to 86% in yearly precipitation, whereas, Upper Awash may see an increase of up to 56% in precipitation. As a result, the Gidabo watershed’s future hydrology may be seriously threatened. The Awash River basin may see a 191% rise in streamflow and will cause flooding problems, while, the Gidabo River may result in a 43% decrease and will cause a water shortage.
The variation in the estimation could be caused by various factors, including different hydrological model types, different amounts of input data, different global climate models, and different calibration approaches used by different authors. Therefore, before any planning, policy-making, or development of water resources for any given watershed, a climate impact assessment for future hydrology should be conducted. Furthermore, a single hydrological model’s output cannot produce a trustworthy result for the comprehensive planning of water resources; rather, the output’s accuracy can be raised by utilizing additional, more sophisticated techniques and methods.
3. Performance evaluation of the hydrological model
Assessing the accuracy of generated data to the measured data and constituent values requires more than just simple professional guidance is unacceptable. Scientifically, the accuracy of the streamflow simulation generated by the hydrological model is evaluated using a goodness-of-fit statistical index (Lamontagne et al., Citation2020). For this reason, many goodness-of-fit criteria have been developed (Althoff & Neiva, Citation2021). It is possible to assess the correctness of a hydrological model using a variety of measures, which will also boost the predictability of the results (Jackson et al., Citation2019). The assessment of model performance is critical for calibration, and evaluation of hydrological models. One goodness-of-fit criterion, which is either maximized or minimized by modifying the model’s parameters, is frequently used as the basis for calibrating hydrological models. A minimum of one dimensionless criterion (maximization) and one error measure (minimization) is required among the numerous studies for assessing the performance of a hydrological model (Gelete et al., Citation2023).
Three categories are commonly used to evaluate streamflow: hydrological signatures, performance evaluation index, and error measure. While the ideal value of a performance index (dimensionless criterion) is one and should be maximized as an objective function, the ideal value of an error measure is zero and should be minimized (Althoff & Neiva, Citation2021). There are numerous performance evaluation statistics for the hydrological simulation; nevertheless, different researchers employed different evaluations. It’s unclear why they chose one out of the many and why others were overlooked.
Januário et al. (Citation2022) employed NSE and KGE to assess the simulation’s correctness. KGE was used for the calibration and validation. KGE is preferred over NSE because it overcomes several of NSE's stated faults. Nevertheless, error metrics like PBIAS and RSR were overlooked. Akiso et al. used NSE, R2, and RVE for climate impact assessment on streamflow. However, Mekonnen et al. (Citation2023) have used NSE, PBIAS, and R2 for the same watershed as Akirso et al. (Citation2022). Evaluating the accuracy of the model with multi-evaluation statistics is better than using minimum-number evaluation statistics. Ang and Oeurng (Citation2019) evaluated the simulation with a performance index (NSE) and an error index (PBIAS and RSR). The simulation’s PBIAS evaluation (1.14) indicates very good performance; on the other hand, the RSR evaluation (0.63) shows satisfactory performance. This result suggested that assessing the model’s accuracy solely using PBIAS or RSR might not offer sufficient and reliable conclusions. KGE is an enhanced NSE evaluation index as well, but the majority of the research has still optimized the parameter values during calibration and validation using the traditional NSE.
Oversensitivity to high flows, overestimation of low flows, and underestimation of high flows are some of the drawbacks of the traditional performance evaluation method, which primarily uses performance indexes like R2, NSE, and error measurements like PBIAS, RMSE, and RSR (Althoff & Neiva, Citation2021; Boisvert et al., Citation2016). In recent decades, various hydrological simulations have employed hydrologic signature evaluation to address the shortcomings and enhance classical performance evaluation (Topalović et al., Citation2020; Chilkoti et al., Citation2018). However, the current studies still use conventional evaluations (NSE, R2, PBIAS, and RSR) without considering hydrologic signatures, including Sheng et al. (Citation2023) and Abdule et al. (Citation2023).
Hydrological signatures (indices characterizing hydrologic behavior) are now commonly used to understand space-time variability in hydrological processes and to diagnose weaknesses in hydrological models (Addor et al., Citation2018). A comparison of signatures obtained from simulated and observed flows can indicate poorly simulated runoff components (peak flow, mean flow, median flow, low flow) that cannot be detected by statistical performance measures alone (Topalović et al., Citation2020).
Furthermore, the uncertainty analysis method or optimization algorithms employed for the model’s calibration and validation have an impact on its performance. Because of nature’s randomness and our limited knowledge of the hydrological system, uncertainty is an inherent part of all hydrologic models. The use of hydrological modeling uncertainty in climate impact assessments has received far less attention (Bastola et al., Citation2011). Adapted uncertainty evaluation techniques include Bayesian statics (BS), particle swarm optimization (PSO), parameter solution (PS), sequential uncertainty fitting version-2 (SUFI-2), Monte Carlo (MC), Markov chain Monte Carlo (MCMC), Generalised likelihood uncertainty estimation (GLUE), and Bayesian statics (BS) (L. Zhang et al., Citation2019).
In the Xinxue and Xiaoqing rivers, L. Zhang et al. (Citation2019) compared the SWAT performance using the SUFI-2 and GLUE uncertainty evaluation methodologies. The Xinxue River basin showed a higher applicability for the SUFI-2 method because of its more logical and accurate analysis results, whereas the Xiaoqing River basin was proven to have a higher application for the GLUE approach. Tang et al. (Citation2021) used SUFI-2, GLUE, PS, and PSO to analyze the uncertainty of SWAT modeling for the Lancang River Basin. NSE and R2 of SUFI-2 (0.89, 0.89), GLUE (0.87, 0.89), PS (0.90, 0.92), and PSO (0.86, 0.84) respectively for the whole time simulation. The findings demonstrated that PS outperformed the others and also SUFI-2 remains well performing. As a result, the two authors’ findings demonstrated that a single uncertainty analysis strategy may also reduce the model’s performance; as a result, it is preferable to compare several approaches and select the best one.
Performance evaluation statistics that are employed as objective functions in calibration and validation, including NSE, R2, PBIAS, and RSR, also have an impact on overall performance (). The objective function used in peak flow and low flow simulation also varied (Fowler et al., Citation2018). According to Fowler et al. (Citation2018), the low flow is better fitted by using the split KGE and index of agreement than using NSE and KGE. Using various objective functions also results in various values for the optimal parameter values, which influences the estimation of water resources (Kouchi et al., Citation2017). Based on Paul and Negahban-Azar (Citation2018) the performance index for R2, NSE, PBIAS, and KGE was 0.91, 0.82, −53.36, and 0.56 for R2, 0.91, 0.84, −40.45, and 0.62 for NSE, 0.80, 0.77, −14.93, and 0.70 for PBIAS, and 0.89, 0.85, −32.93, and 0.74 for KGE was used as objective function. KGE objective function has performed best out of the four objective functions. For improved performance, NSE, R2, and PBIAS were ranked second, third, and fourth respectively. According to Lee and Choi (Citation2021) for calibration and validation, the RMSE of the extremely high flow and extremely low flow estimated using NSE, KGE, and adjusted KGE as objective function showed that, respectively, they were (0.86, 0.79), (0.74,0.74), and (0.74,0.72) and (0.04, 0.04), (0.03, 0.01), and (0.06, 0.04). For high flow adjusted KGE is better performance whereas for low flow KGE is better performance than the other objective function. Thus, various objective functions and optimization procedures must also be taken into consideration to increase the models’ simulation accuracy.
Table 1. Performance evaluation types and description.
4. Types of hydrological models
Hydrological systems are complicated and full contentment in simulation is challenging, researchers are searching for more advanced ways to anticipate (Haznedar & Kilinc, Citation2022) better approach. The simulation of catchments’ hydrological processes by hydrological models is considered a significant concern nowadays. Consequently, a large number of hydrological models have been developed (Tran et al., Citation2018). Many investigations are being conducted on questions such as which model will yield better results that are consistent with observed discharges. They also conclude that most investigations probably just need a simple to moderately complicated model. It was said that even highly advanced modeling does not yield better results (Devi et al., Citation2015).
Many hydrological models from various countries at different eras have been developed to model the hydrology of the watersheds. Accurately determining the most practical and cost-effective hydrological models for a given watershed, however, becomes difficult (Doliso & Hatiye, Citation2021). The models’ results vary greatly depending on the site, and no single model is better under all hydrologic conditions (Aliye et al., Citation2020; Banda et al., Citation2022). The following criteria can be used to classify hydrological models; spatial (lumped, distributed) (Elsanabary & Gan, Citation2015); temporal (static and dynamic) (Tanksali & Soraganvi, Citation2021; Nesru, Citation2023); the dynamic portion is further divided into event-based and continuous (Ayalew et al., Citation2022; De Luca et al., Citation2022); an aspect of randomness (deterministic and stochastic) (Fanta & Sime, Citation2022; Gebremichael et al., Citation2023); process-based (physical, conceptual, and empirical) (Devi et al., Citation2015; Jehanzaib et al., Citation2022); distributed model (partially distributed, fully distributed) (Onyutha et al., Citation2021; Usman et al., Citation2021). From all, parts of 54 physically-based, distributed, conceptual, and lumped models reviewed in this paper (), 28 hydrological models were also utilized in Ethiopia mostly in the upper Blue Nile river basin ().
Table 2. Types of some physically distributed, conceptual, and lumped hydrological models.
Table 3. Some streamflow simulation hydrological models used in Ethiopia.
4.1. Physically-based, distributed, lumped, and conceptual model
Despite the hydrologic model’s classification as distributed and physically based, based on process and space, respectively, it should be noted that the physically based model is also a distributed hydrological model (). In augmenting , the Liuxihe model, which Osman et al. (Citation2021) refer to as a physically based, distributed hydrological model (PBDHM), is one instance to show that physically based models can also be distributed models. Additionally, as indicated in , the model classified as lumped is also classified as a conceptual model. There are two types of models in the distributed model; full-distributed and partially-distributed. The partial-distributed model can be both a conceptual model and a physically based model, like SWAT and HEC-HMS. SWAT is semi-distributed (Singh et al., Citation2022); physically-based (Thapa et al., Citation2017); and conceptual (Noori & Kalin, Citation2016). HEC-HMS is physically based; semi-distributed (Gebre, Citation2015; and conceptual hydrological model (Gebre, Citation2015).
Concerning model parameters, they varied from 4 parameters in the GR4J model to high parameters greater than 25, like SWAT and DHSWM. However, a few researchers only put the sensitive parameters when listing the model’s parameters, which prevents this review paper from inserting the precise number of parameters in a few models. As a result, it is advised that one must be aware that the model’s parameters have their significance (), which may not be consistent and precise for a few models. The parameter count presentation in this review paper aims to highlight the models with comparatively high and low counts. It is also possible to easily check whether the input data and the parameters are easily accessible from the study area before starting the model’s analysis, to show that future researchers will find it easy to identify which model requires a large number of parameters, as well as to check the list of parameters using the references (). This review study focuses on compiling a few alternative journals as a backup plan and then selecting the most appropriate one out of all of them.
For instance, Khattati et al. (Citation2016) utilized eight parameters for their stream flow simulation using the ATHYS hydrological model; Maref and Seddini used six parameters; and Bouadila et al. (Citation2023) used five parameters. This review used the maximum number of parameters (8) () from the 8, 6, and 5 parameters of the ATHYS model was utilize.
The physically based distributed hydrological model, including MIKE-SHE, MISBA, SWBM, CEQUEAU, QSWAT, SWAT, DHSVM, APEX, LARSIM, TOPKAPI, and DWSM, has a relatively large number of parameters, which is greater than 23, whereas, the conceptual model, including GR4J, RCC-WBM, DBM, IHACRES, WASMOD, AWBM, GRSD, HYMOD, HIMS, MIKE NAM, PED-WM, SIMHYD, WEAP, HMSV, MOHYSE, and SMARG, has a relatively low number of parameters. From the distributed models (), CEQUEAU, DHSVM, GSSHA, MIK-SHE, MISBA, TOPKAPI, and WATFLOOD are fully distributed models, whereas, the remaining distributed models are semi-distributed.
The physically based distributed model is generally flexible and can be used for most of the types of hydrological responses. For example, one of the versatile SWAT models is used for long-term continuous simulations of flow (Haleem et al., Citation2022); soil erosion (Mosbahi & Benabdallah, Citation2020); sediment simulation (Ricci et al., Citation2018); movement of nutrients in watersheds (Zeiger & Hubbart, Citation2016); event-based flood simulation (Jodar-Abellan et al., Citation2019); streamflow drought forecasts (Sohoulande Djebou, Citation2019); streamflow regionalization to ungauged sites (Kanishka & Eldho, Citation2020); urban water stormwater simulation (Glick et al., Citation2023); and water quality evaluation (Olaoye et al., Citation2021) with different sizes and hydrologic, geological, and climatic variables; and evaluating the effects of climate change, land use and cover, and watershed management (Nilawar & Waikar, Citation2018). However, specifically, some models have been developed for particular purposes including the APEX model for small watersheds, fields, and plots at a higher spatial resolution, VIC for macro-scale watershed, and SWMM for urban watershed flow simulation. The lumped conceptual hydrological model including the simplest GR4J model can used for streamflow regionalization (Arsenault et al., Citation2019); operational flood forecasting (Buddika & Coulibaly, Citation2020); land use land cover change effect on streamflow (Mouche et al., Citation2014; Troy et al., Citation2007; Leila et al., Citation2024); climate and human impact on streamflow (Y. Liu et al., Citation2021).
4.2. Machine learning-based streamflow modeling
Streamflow modeling is currently receiving greater attention with machine learning-based hydrological models () than from other data-driven hydrological models (Hu et al., Citation2018). The primary driving force behind the shift from physically and conceptually based hydrological modeling to machine learning modeling is the lack of observational data, and relatively high performance which makes it more difficult for these models to be applied in locations with poor gauges (He et al., Citation2021) as summerised in . Multilayer perceptron (MLP), support vector regression (SVR) (Hussain & Khan, Citation2020); long-short-term memory (LSTM) (Hu et al., Citation2018); Gaussian linear regression model (GLM), Gaussian generalized additive model (GAM), multivariate adaptive regression splines (MARSs); artificial neural network (ANN), random forest (RF), and 1D convolutional neural network (1DCNN) (D. Singh et al.,Citation2023); recurrent neural network (RNN), and adaptive fuzzy neural inference system (ANFIS) (M. Khan et al., Citation2023) are examples of machine learning streamflow modeling.
Table 4. Physically based-distributed and lumped-conceptual hydrologic model summary.
Table 5. Summary of the physical and conceptual model with a machine learning model.
To assess the effectiveness of machine learning-based streamflow modeling with the impact of climate change on future hydrology, the following studies have been conducted: Lin et al. (Citation2019) studied the response of surface runoff to climate change in the Upper Minjiang River (22,900 km2), Southwest China; M. Khan et al. (Citation2023) evaluated the effects of climate change on stream flow dynamics in the Hunza watershed (13,567 km2), which is shared by Pakistan, China, and Afghanistan; D. Singh et al. (Citation2023) studied machine learning and deep learning-based streamflow prediction in a hilly catchment for future scenarios using CMIP6 GCM data in Satluj basin (2457 km2).
Furthermore, for continuous long-term streamflow simulation, Tibangayuka et al. (Citation2022) simulated streamflow for a high-humid tropical catchment of the Upper Ruvu (7663 km2) in Tanzania; S. Khan et al. (Citation2023) investigated the inter-comparisons of ANN and SWAT in the Astore watershed, upper Indus basin (45, 400 km2), India; Jimeno-Sáez et al. (Citation2018) compared SWAT and ANN Models for Daily Runoff Simulation in Different Climatic Zones of Ladra river (843 km2), Peninsular Spain; Khazaee Poul et al. (Citation2019) compared MLR, KNN, ANN, and ANFIS Models with Wavelet Transform in Monthly StreamFlow Prediction in the Clair river (579,000 km2), Canada; Haznedar et al. (Citation2023) forecast streamflow using a hybrid LSTM PSO approach in the Seyhan river Basin (22.04 km2), Eastern Mediterranean Region of Turkey.
In summary, the model has been used to streamflow modeling based on machine learning, ranging in size from small watersheds (22.04 km2) to macro watersheds (579,000 km2). As seen in , there isn’t a single model that performs flawlessly in every watershed or during calibration and validation. As an example of an ANN, the model fits very well in some watersheds and satisfactorily or unsatisfactorily in others. Thus, it is not advised to choose a single machine learning model at random for streamflow analysis; rather, comparing multiple models and choosing the best one could be a better way to increase performance and ensure that the model’s output is accepted for future applications.
4.3. Event-based hydrological model
Hydrologists frequently utilize incident-grounded models to simulate hypothetical and actual flood disasters. The timescale required by event hydrologic models is sub-daily (Xie et al., Citation2019). Event-based models consider a single rainfall event separately, as opposed to continuous models that simulate flow over a longer period (Stephens et al., Citation2018). Regarding the input rainfall for the event-based model, it is a design storm, but the extended synthetic rainfall scenario pertains to the continuous model (Ayalew et al., Citation2022). Soil Conservation Service Lag-and-Route (SCS-LR) (Nguyen & Bouvier, Citation2019), Watershed Boundary Network Model (WBNM) (Stephens et al., Citation2018), Event-Based Approach for Small and Ungauged Basins (EBA4SUB) (Ayalew et al., Citation2022), Sacramento Soil Moisture Accounting (SAC-SMA) (Bournas & Baltas, Citation2021), Hydrological Simulation Program–Fortran (HSPF) (Xie et al., Citation2019), HEC-HMS (Nourali et al., Citation2016), SWAT (Yaduvanshi et al., Citation2018), TOPKAPI-X (Janabi et al., Citation2021), TOPMODEL (Januário et al., Citation2022), GSSHA (Al-Areeq et al., Citation2021) are some of the event based hydrologic model.
4.4. Ensemble of hydrological model
Due to inadequate input data, model structure, and model output uncertainty in large-scale exercises, using a single hydrologic model typically results in simulation uncertainties. When modeling hydrological processes, the ensemble model technique may be a more effective tool for making decisions (Himanshu et al., Citation2023). Chathuranika et al. (Citation2022) have compared the streamflow simulation using SWAT and HEC-HMS, and the simulation result showed that the HEC-HMS model caught medium flows better than the SWAT model did for high flows. Additionally, the SWAT model accurately predicted both dry and wet seasonal flows within seasonal time bases, with modest under predictions of 2.12% and 13.52% relative to the observed values. In comparison to observed flows, the HEC-HMS model underpredicted the dry and wet seasonal flows by 10.76% and 18.54%, respectively. The results showed that the model ensemble had a great capacity to fill in the gaps created by individual models. Hydrology research using ensemble forecasting is presently a well-established field that covers a wide range of operating scenarios (Troin et al., Citation2021). By using ensemble approaches to improve the existing model’s capability, predictive performance can be enhanced. Consequently, it is thought that by leveraging the advantages of each model, an ensemble approach that integrates the output of several models could increase the accuracy of the individual models (Gelete et al., Citation2023).
In the Cauvery catchment of the Indian Peninsula, Horan et al. (Citation2018) compared the performance of a single hydrological model with a multi-model ensemble. The results show that in catchments containing reservoirs, the ensemble mean performs better in terms of prediction than the individual models. Teklesadik et al. (Citation2017) assessed the inter-model comparison of the hydrological impacts of climate change on the Upper Blue Nile basin using global climate models and an ensemble of hydrological models. They concluded that future studies on the effects of climate change ought to take into account the use of an ensemble of hydrological models instead of basing assessments on a single hydrological model, which could produce biased results.
The simulation performance of three physically-based hydrological models (SWAT, VIC, and BTOPMC) was assessed by Z. Li et al. (Citation2018). The individual models SWAT (0.77, 0.66), VIC (0.75, 0.65), and BTOPMC (0.82, 0.75), evaluated with NSE for calibration and validation, respectively, are less fitted than the ensemble of the models (0.95, 0.90). Tian et al. (Citation2017) evaluated the impact of climate change on low flows in the Xiangjiang river basin using the Bayesian averaging method with the GR4J, HBV, and Xinanjiang hydrological model. The Bayesian averaging strategy improved the ensemble’s NSE value, and all simulated streamflows fell inside the 95% confidential interval.
The performance is impacted by the ensemble technique employed when employing the ensemble model. Neural network-based averaging, weighted averaging, and simple averaging are among the various ensemble averaging techniques. The neural network performs the best out of the three ensembles when using the two-ensemble technique. Accurate model simulation results can also be achieved by comparing multiple ensemble techniques and choosing the best ensemble technique (Gelete et al., Citation2023). The ensemble hydrological model performs better than the individual model simulation, to put it briefly. The ensemble can be carried out using a data-driven model or a distributed hydrological model, or it can be lumped and distributed, lumped and lumped, or distributed. The accuracy of the model simulation output can also be influenced by the ensemble technique used.
The ensemble model is superior to the individual model simulation, as demonstrated by the numerical value comparison of the goodness of fit values conducted by Gelete et al. (Citation2023) and Himanshu et al. (Citation2023) in the Katar watershed, Ethiopia, using neural network ensemble (NNE) and the Moral watershed, India, respectively, as a sample shown in . According to the results of both authors, the ensemble model performs well across all NSE, R2, and PBIAS metrics.
4.5. Hybridization of hydrological model
It has been established that machine learning (ML) is a promising method for correctly predicting streamflow. Unfortunately, there is a lack of transparency because artificial intelligence hydrological modeling is a black box and its parameters hardly ever explain the physical significance of catchment characteristics (Poonia & Tiwari, Citation2020). Hydrologists have been using hybrid modeling more and more in recent years (Haznedar & Kilinc, Citation2022).
When multiple hydrological models are integrated, coupled, embedded, and combined for hydrological component simulations, the term ‘hybrid hydrological model’ is used (Kilinc, Citation2022; Okkan et al., Citation2021). Hydrological framework in comparison to competitors, hybridized models can combine the advantages of both the physical hydrological model and the data-driven model, or the strengths of each data-driven model individually, resulting in more accurate streamflow predictions and realistic uncertainty intervals (Ren et al., Citation2018). The hybrid technique is a multi-model strategy that uses the time series produced by the conceptual or physically based hydrological model as inputs of a machine learning system, known as a nested hybrid, to boost the accuracy of flow predictions (Farfán et al., Citation2020; Okkan et al., Citation2021; Haznedar et al., Citation2023).
Examples include the following: merging HBV with a Bayesian neural network (Ren et al., Citation2018); hybridizing lumped GR4J with a deep learning machine (Kapoor et al., Citation2023; Kumar et al., Citation2023); combining the physically based SWAT model with ANN and the HEC-HMS model with ANN for flood forecasting (Kurian et al., Citation2020; Gebremichael et al., Citation2023). The hybrid model offers a significant improvement in predictive performance over the component models. According to all of the study findings of the hybridized physically and conceptually hydrological model with ML reviewed in this paper.
Moreover, the hybrid approach combines machine learning and evolutionary algorithms to predict streamflow. This includes integrating an adaptive neuro-fuzzy inference system (ANFIS) with a genetic algorithm (GA) (ANFI-GA) (Kurian et al., Citation2020); combining particle swarm optimization (PSO) and long short-term memory networks (LSTM) (Haznedar et al., Citation2023); combining feedforward neural networks (FFNNs) with genetic algorithm (GA) (Zakhrouf et al., Citation2020); hybrid ANFIS models with GA, particle swarm optimization (PSO), and ant colony optimization for continuous domain (ACOR) optimization algorithms (Azad et al., Citation2018).
To bolster the hybrid model’s capabilities, this review paper employed numerical comparisons of individual models with the hybrid. These comparisons were carried out by taking Kapoor et al. (Citation2023) in Australia for 222 unregulated catchments taken from the CAMELS-AUS data set by hybridizing GR4J with LSTM and CNN; Kassem et al. (Citation2020) in the northern Iraqi Khazir basin using Asmawa and Khanis stations using SWAT and ANN; Kilinc and Haznedar (Citation2022) in the Euphrates River at Yazikvy and Kilay gauge stations using LSTM and genetic algorithms (GA). The NSE value in the Kapoor et al. (Citation2023) study represents the 95th percentile of the entire catchment.
To support numerical comparisons of individual models with the hybrid: a study conducted by Kapoor et al. (Citation2023) in Australia for 222 unregulated catchments taken from CAMELS-AUS data set by hybridize of GR4J with LSTM and CNN; Kassem et al. (Citation2020) in Khazir basin which located in the north of Iraq using Asmawa and Khanis stations using SWAT and ANN; Kilinc and Haznedar (Citation2022) in the longest river in Western Asia is the Euphrates at Yazikvy and Kilay gauge station using LSTM and genetic algorisms (GA) have used as shown in . The NSE value of Kapoor et al. (Citation2023) study is not the single watershed instead the 95 percentile of the whole catchment. 95 percentile is the statistical measure that indicates the value is equal to or greater than 95 percent of the total population. There were differences in the 5 percentile performance across CNN (−0.001), GR4J (0.17), and LSTM (0.23) concerning NSE values. This finding suggests that not all watersheds have uniformly high performance from machine learning. The hybrid hydrological model thus performs better than the single model.
5. Performance of some individual physically based-distributed hydrological models
shows that SWAT can estimate streamflow in all sizes of watersheds, ranging from small scales (Maybar, 1.12 km³) and Megech River (170 km³) in Ethiopia’s upper Blue Nile basin to enormous scales (860,000 km2 in China’s upper Yangtze and 3.7 million km2 in the Congo River). The SWAT models able to simulate streamflow are very good, satisfactory, and, to a small extent, not satisfactory when measured using R2, NSE, and PBIAS at various watersheds, as shown in . As a result, the SWAT model cannot function well in every watershed. The performance rating status of the other models, such as HEC-HMS, QSWAT, MIKE-SHE, and VIC, is identical to that of the SWAT model. The proportion of very good and good performance ratings is comparatively higher than the percentage of satisfactory and not satisfactory performance ratings, even though the percentage of performance ratings is not consistent as the number of review papers varied. While MIKE-SHE performs very well in the Shaya watershed in Ethiopia, the performance in the Chauhe watershed at the Dage stream flow station in China is both inadequate and satisfactory. As a result, it is uncertain if MIKE-SHE, SWAT, or any other model will be chosen based on performance. Therefore, there isn’t a single model that works superior to the others every time and everywhere. It could be more advantageous to compare multiple hydrological models rather than applying a single model to each watershed.
Table 6. The various watershed performance index values of some models.
Using the SWAT model as an example, the review’s findings also attempted to show whether or not the watershed area, the number of streamflow data, and the ratio of calibration to validation years have an impact on the goodness of fit value. As seen in , respectively, the results showed that there isn’t a clear relationship. The size of watershed, numbers of data used, and the numbers of data used in calibration and validation have not indicated an influence on the performance of the SWAT model. Nonetheless, the choice of time step as daily or monthly directly affects the R2 and NSE values (). The monthly time step exhibits higher NSE and R2 values when compared with daily time bases. However, it maynot be always true. Generally, less than 25 years of data are used, the lowest and maximum numbers of recorded streamflow data points are 5 and 30, respectively. This implies a scarcity of long-term historical data for most rivers.
Not only does the model’s performance differ from one watershed to the other, but it also varies amongst authors who utilized the same model in the same watershed. For instance, SWAT has been utilized for streamflow modeling for various objectives in the Katar watershed, Rift Valley Lake Basin, Ethiopia by Aliye et al. (Citation2020), Sime et al. (Citation2020), Gelete et al. (Citation2023), Balcha et al. (Citation2023). Gelete et al. (Citation2023) simulated very good or comparatively better suited in daily time bases compared from the results of the four author’s performance index. The three authors did a good simulation performance. Based on the findings of the four authors’ performance index, Gelete et al. (Citation2023) simulated with very good or somewhat better matched on a daily time basis ().
Neither Sime et al. (Citation2020) nor Aliye et al. (Citation2020) used error measure performance evaluation. Rather, Gelete et al. (Citation2023) and Balcha et al. (Citation2023) employed PBIAS as an error measuring index. The PBIAS index error measure demonstrated satisfactory performance on both a daily and monthly basis, with approximately equal magnitudes. About eighteen years’ worth of streamflow data were utilized by all writers in a comparable quantity. A primary distinction is that Gelete et al. (Citation2023) employed the validation data (1st January 2000 to 31st December 2005) from portions of older observed data and the calibration (1st January 2006 to 31st December 2017) from the relatively current data. While most researchers conclude that the monthly output simulation of streamflow performs better than the daily time bases, a study carried out in Katar revealed that the daily time bases had a comparatively higher performance index (). This summary demonstrated that streamflow simulation is a challenging task that necessitates careful examination to produce consistent results that do not vary with time and watersheds. Some studies in this review paper have not used the warming year, as indicated in , even if it might be that the authors did not write the warming year in the journal as they used it in their analyses. The warming year that authors utilized varied as well (from 2 years up to 4 years). Further research may be necessary, particularly for single watersheds, to determine whether or not the warming year, the ratio of calibration to validation data, the size of the watershed, and the sequence of validation and calibration data affect the model’s performance.
5.1. Comparison of daily and monthly time step simulation performance
The SWAT model produces precise simulation results for the daily and monthly time steps (), which are beneficial for the basin’s water resource management (Vilaysane et al., Citation2015). The outcomes showed that the daily time step streamflow simulation is not as accurate as the monthly flow. The error statistics like PBIAS, RMSE, and others haven’t been examined, though, and it’s unclear whether the time step has the greatest or lowest bias among the simulated and actual data. Himanshu et al. (Citation2023) have compared the daily and monthly simulation performance of the individual model with the ensemble model. The monthly time-based strong performances are based on the same findings as Vilaysane et al. (Citation2015) for the index measures of NSE in all models (SWAT, VIC, and ensemble of VIC and SWAT). On the other hand, in all models for PBIAS, the daily time base performs better than the monthly time base. The results of Chathuranika et al. (Citation2022) also supported the monthly time bases’ superior fit in terms of NSE and R2, with the PBIAS for the HEC-HMS model being the same for both monthly and daily time bases.
Tibangayuka et al. (Citation2022) evaluated the HBV, HEC-HMS, and ANN models’ daily and monthly streamflow simulation performance for a high-humidity tropical watershed in Tanzania. The study’s conclusions showed that in every R2, NSE, and PBIAS of the three models taken into consideration, the monthly time bases performed better than the daily time bases. HBV (0.74, 0.64), HEC-HMS (0.76, 0.76), ANN (0.67, 0.52); HBV (0.73, 0.64), HEC-HMS (0.76, 0.68), ANN (0.66, 0.55); HBV (0.39, −3.7), HEC-HMS (0.07, 20.61), ANN (−12.73, −16.93) are the daily time bases for R2, NSE, and PBIAS, respectively. supports the comparisons between the daily and monthly streamflow simulation output performance evaluation interpretation as an example. It shows that, in terms of performance index (NSE and R2), the monthly time bases outperformed the daily time bases; however, the error measure (PBIAS) does not indicate better performance.
5.2. Performance of some lumped conceptual hydrologic model
The lumped-conceptual hydrological model (MIKE-NAM) performance findings also revealed that different watersheds have different performance ratings, ranging from very good performance to not satisfactory, with the same performance rating status as the physically based-distributed model, as shown in . Even if the percentage of performance ratings fluctuated when the quantity of review papers changed, the proportion of very good and good performance ratings is still significantly greater than the percentage of satisfactory and not satisfactory performance ratings ().
Table 7. MIKE-NAM performance index value.
Using the GR4J model, Li et al. (Citation2014) have looked into how runoff in the Yarlung Tsangpo River basin on the Tibetan Plateau is being impacted by climate change. Eight streamflow gauge station watersheds in the basin were the subject of independent investigations, with watershed areas ranging from 3761 km2 to 49,739 km2. When the model’s performance was assessed using the NSE, it was found to be independent of the watershed’s area, the number of data points per year, the number of streamflow data points (years) used for both calibration and validation, and the ratio of the number of calibration points to the number of validation points (). Of the eight distinct watersheds in the basin, five exhibit very good performance; the other three exhibit satisfactory, poor, and good performance, respectively, and are represented by the Shigatse Station Watershed, Lhatse Station Watershed, and Gyantse Station Watershed as shown in . Tian et al. (Citation2013) also evaluated the streamflow’s climate impact using GR4J. Based on the NSE performance evaluation, it performed exceptionally well (0.93 and 0.91 for validation and calibration, respectively).
6. Performance comparisons of the SWAT model with some model
As seen in the summary of and in and , the overall single hydrological model performance comparisons showed that neither model is unquestionably superior to the other comparative model. It is interesting to note that hydrologic models were created in certain environments and then applied to watersheds with different climatic and watershed characteristics (Chathuranika et al., Citation2022). The watershed’s hydrological features determine whether a model’s structure is appropriate. It’s possible that a model that faithfully captures the main hydrological processes in one watershed won’t work as well in another with distinct features. Watersheds differ in terms of soil types, land cover, and topography hetrogeniety, which can affect the ideal parameter values. There’s a chance that a model that’s been calibrated for one watershed using the right parameter values won’t translate well to another (Ávila et al., Citation2022). Due to variations in hydrological features, data accessibility and quality, model complexity and structure, parameterization, human activity, climate variability and change, and model uncertainty, the performance of hydrological models can differ between watersheds. To get dependable and accurate results, hydrological modelling techniques must be specifically tailored to each watershed’s unique characteristics (Sempewo et al., Citation2022).
Table 8. Comparisons of the SWAT model with HBV, HEC-HMS, GR4J, and ML.
Table 9. Comparisons of some lumped conceptual model.
As a result, choosing models for a particular watershed based only on the work of one author or one watershed’s results may introduce uncertainty and reduce the acceptability of the model’s output. A well-fitting model will result in worse performance in a different watershed when used as a comparative model. The most flexible model, SWAT, was reviewed in this study along with conceptual lumped models (GR4J), spatially distributed physically-base models (HEC-HMS, MIKE-SHE, HBV, and VIC), and machine learning models ().
A comparison of the effectiveness of the SWAT and MIKE-SHE models was conducted by J. Liu et al. (Citation2016) and Golmohammadi et al. (Citation2014). The two authors’ results diverge. J. Liu et al. (Citation2016) state that using the NSE, R2, and PBIAS assessment indexes, respectively, SWAT (0.76, 0.78, and 0.22%) performs better than MIKE-SHE (0.71, 0.70, and 8. 12%). In contrast, Golmohammadi et al. (Citation2014) found that using NSE, R2, and PBIAS assessment indices for calibration and validation, respectively, MIKE-SHE (0.84, 0.64; 0.79, 0.71; and 6.67, 3.57) performs better than SWAT (0.74, 0.64; 0.73, 0.7; and −3.14, −12.5).
Himanshu et al. (Citation2023) and Z. Li et al. (Citation2018) have compared the SWAT model with the macro watershed model VIC. All three authors’ results indicated that the SWAT model has better performance than the VIC model. However, Wu et al. (Citation2021) results indicated VIC is outperformed than SWAT. Overall, the HEC-HMS outperforms the SWAT model in various watersheds, according to comparisons between the two models. Nevertheless, the percentage also fluctuated when the number of evaluating papers changed, and the percentage of SWAT might have improved or decreased. This review’s conclusions unmistakably showed that SWAT performs better than HEC-HMS in some watersheds and that HEC-HMS performs better than SWAT in others. Furthermore, at different study times, Gelete et al. (Citation2023) and Aliye et al. (Citation2020) compared SWAT and HEC-HMS in the Katar watershed, Ethiopia. Gelete’s (2023) results suggest that SWAT performs better, but Aliye et al. (Citation2020) found that HEC-HMS performs better. This finding demonstrates the complexity of the hydrological system and the difficulty of simulating it.
The results of comparing machine learning (ML) and SWAT showed that ML performed significantly better than SWAT. Planning and managing sustainable water resources depends on accurate hydrological process simulation. The better performance of machine learning-based streamflow simulation than the SWAT model is due to various reasons. The reasons given by Rahman et al. (Citation2022) for the high performance of machine learning than SWAT model are choosing sensitive parameters in SWAT modeling, possible drawbacks of the SCS-CN method for simulating streamflow, and SWAT's inability to record the hydro peaking. The low accuracy of the snowmelt runoff simulations in the SWAT model may be attributed in part to the assumption regarding the snowmelt factor not being well supported by the actual processes (Zhao et al., Citation2022). In mountainous areas, SWAT frequently performed poorly and needed extra procedures to be effective (K. I. Islam et al., Citation2023). Numerous types of input data are needed for the SWAT model, including elevation, data on land use and cover, drainage, geology, and climate. Unfortunately, due to unavoidable issues like uneven observed station distribution, social and political unrest, and limited data sharing amongst nations in transboundary basins, some of these data are not entirely accessible in many regions. Since this method doesn’t require a lot of data, machine learning models are helpful in this situation Rahman et al. (Citation2022). Therefore, due to the limited input data especially in poor countries, the processed-based model (SWAT) had limitations to topography like mountainous areas, peak flow, and snow areas making the SWAT model low-performance.
Comparing the two lumped models (GR4J with AWBM and GR4J with IHACRES) also showed that while GR4J performs better than both AWBM and IHACRES in some watersheds, its performance is inferior in other watersheds as shown in and . The models’ performance comparisons also reveal that GR4J performs worse during validation and better during calibration with R2 or vice versa. Furthermore, GR4J performs worse in the NSE or PBIAS and is superior with R2. This review concludes that there is a lack of consistency in the performance of the hydrological models.
7. Conclusion
Climate change is currently the biggest issue affecting water resources. The interpretation of this review paper is bolstered by the use of a relatively water-resource-rich country in Africa (Ethiopia). The majority of the studies in this paper indicate that future temperatures would rise in most basins of the country, with the Wabishebele River basin experiencing an 8.2 °C change in annual maximum temperature. Its future streamflow and rainfall prediction vary with trend (increase or decrease) and also the rate of change between watershed, season, models, and researchers. For instance, Gidabo may suffer an 86% yearly decrease in precipitation, but Upper Awash might receive a 56% annual increase in precipitation. The Gidabo River may witness a 43% decline in stream flow, which may result in water scarcity, while the Awash River basin may see a 191% increase in stream flow, which may lead to flooding issues. Because of this stark disparity in the trend of hydrological change, one needs to conduct a study on climate impact effect evaluation for each watershed or basin to minimize the havoc of water and maximize the benefits associated with it. Therefore, through a simplified and continually upgraded hydrologic model, the streamflow simulation accuracy is evaluated with the model performance evaluation index.
The model’s performance in the simulation is the primary criterion after taking into account all other factors including cost, time, simplicity, and study aim when choosing a model. At least one performance index and one error measure are required for performance evaluation. However, the classical performance evaluation has limitations for oversensitivity to high flows, overestimation of low flows, and underestimation of high flows. Therefore, currently, the NSE-based shortcomings improved with KGE, and Log NSE; the over sensitivity to high flow, underneath valuation of peak flow, and excess valuation of low flow problem can be improved by using hydrological signature. The strength of the hydrologic signature is to diagnose weaknesses in hydrological models.
Additionally, the performance of the model is also affected by the uncertainty analysis technique used in the calibration and validation. The comparisons of the uncertainty evaluation techniques include GLUE, MC, MCMC, SUFI-2, BS, PSO, and PS, and selecting the one to provide high-performance simulations will help to enhance the model’s functionality. Even though most studies underestimate this process evaluating the streamflow simulation using uncertainty measure indices like the relative width of a 95% probability band (R factor) and 95% prediction uncertainty (95PPU) (P factor) shows the strength of the simulation accuracy.
Numerous hydrological models have been developed worldwide, ranging from the basic empirical rational model to the lumped conceptual model and then upgraded to the physically based and distributed model. Choosing one hydrological model for investigation from a variety of models is a difficult issue for a researcher because both knowledge-based hydrological models and machine learning model components are numerous.
Machine learning can approximate NSE and R2 quite well, up to 0.99, though this isn’t always the case for all watersheds. For instance, the results of performance evaluations for lumped conceptual models (MIKE-NAM and GR4J) and physically and distributed models (SWAT, HEC-HMS, MIKE-SHE, VIC, and QSWAT) in this review showed that all models can perform well (not satisfactory up to very good).
The most flexible and versatile SWAT model has been compared with MIKE-SHE, VIC, HEC-HMS, HBV, GR4J, and ML to determine which model can perform better than the others. The review’s results showed that ML performed well with SWAT, with notable variation. Nevertheless, neither SWAT nor any other model outperformed the other model. There are study areas where SWAT performed very well and also areas where SWAT performed poorly. A single hydrological model has limits, such as certain models that overestimate low flow and perform well for mean flow; other models may perform well for peak flow, but because of this, the model’s total performance may be reduced. Thus, the shortcomings of the individual model can be improved by the ensemble multi-single hydrological model. The ensemble strategy used, which includes the weighted average, simple averaging, and Bayesian averaging method based on the output of the ensemble of single hydrological models, also affects the ensemble model’s performance. It is therefore necessary to compare the results of the various ensemble techniques to ascertain which is superior.
Relatively, ML modeling has high performance, however its hydrological significance is low. Instead, especially the physically based-distributed has relatively higher hydrological significance and considering the heterogeneity of the watershed though has relatively low performance even if not applicable to all watersheds. Therefore, from this review, it can be depicted that hybridizing the conceptual models based on physical aspects along with the machine learning model is important to maximize the model’s performance. Using this approach, the water resources potential in the river basins and watersheds for a long period of time with out the existence of extreme events can be managed. Based on reviewing the overall summary of future research directions, this review recommends that single-hydrological model cannot provide satisfactory information. Therefore, selecting the best ensemble of conceptual & physical based hydrological models coupled with machine learning and evolutionary algorithms to compare objective functions like NSE and KGE as hydrological signatures and choosing the best uncertainty evaluation techniques, especially for automatic calibration can be a healthy approach.
Authors contributions
Yilak Taye Bihon and Tarun Kumar Lohani conceived of the presented idea, drawn the graphs and figures, and drafted the manuscript. Bogale Gebremariam Neka and Abdella Kemal Mohammed developed the theory, prepared the tables, and performed the computations. Elias Gebeyehu Ayele and Abebe Temesgen Ayalew verified the analytical methods and supervised the findings of the entire work. Getachew Bereta Geremew revised it critically for intellectual content. All authors discussed the results contributed to the final manuscript and agreed to be accountable for all aspects of the work.
Disclosure statement
The authors declare that they have no known competing financial interests or personal relationships that could have appeared to influence the work reported in this paper.
Data availability statement
The data that support the findings of this study are available from the corresponding author upon reasonable request.
Additional information
Notes on contributors
Yilak Taye Bihon
Yilak Taye Bihon is a PhD scholar in Hydraulic Engineering, Faculty of Hydraulic & Water Resources Engineering, Water Technology Institute, Arba Minch University, Arba Minch, Ethiopia.
Tarun Kumar Lohani
Tarun Kumar Lohani is a Professor, Faculty of Hydraulic & Water Resources Engineering, Water Technology Institute, Arba Minch University, Arba Minch, Ethiopia.
Abebe Temesgen Ayalew
Abebe Temesgen Ayalew is an Assistant Professor cum Deanin Faculty of Hydraulic & Water Resources Engineering, Water Technology Institute, Arba Minch University, Arba Minch, Ethiopia.
Bogale Gebremariam Neka
Bogale Gebremariam Neka is an Associate Professor in Faculty of Hydraulic & Water Resources Engineering cum Scientific Director,Water Technology Institute, Arba Minch University, Arba Minch, Ethiopia.
Abdella Kemal Mohammed
Abdella Kemal Mohammedis an Associate Professor in Faculty of Hydraulic & Water Resources Engineering, Water Technology Institute, Arba Minch University, Arba Minch, Ethiopia.
Getachew Bereta Geremew
Getachew Bereta Geremewis an Assistant Professor in Faculty of Hydraulic & Water Resources Engineering, Water Technology Institute, Arba Minch University, Arba Minch, Ethiopia.
Elias Gebeyehu Ayele
Elias Gebeyehu Ayeleis an Assistant Professor in Faculty of Hydraulic & Water Resources Engineering, Water Technology Institute, Arba Minch University, Arba Minch, Ethiopia.
References
- Abdi, D. A. (2021). Evaluation of the WEAP model in simulating subbasin hydrology in the Central Rift Valley basin, Ethiopia. Ecological Processes, 10, 41.
- Abdule, A. M., Muluneh, A., & Woldemichael, A. (2023). Impact of climate and land use/cover changes on streamflow in Yadot Watershed, Genale Dawa Basin, Ethiopia. Air, Soil and Water Research, 16. https://doi.org/10.1177/11786221231200106
- Abera Abdi, D., & Ayenew, T. (2021). Evaluation of the WEAP model in simulating subbasin hydrology in the Central Rift Valley basin, Ethiopia. Ecological Processes, 10(1), 41. https://doi.org/10.1186/s13717-021-00305-5
- Addor, N., Nearing, G., Prieto, C., Newman, A. J., Vine, N. L., & Clark, M. P. (2018). A ranking of hydrological signatures based on their predictability in space. https://doi.org/10.1029/2018WR022606
- Ahmadi, M., Moeini, A., Ahmadi, H., Motamedvaziri, B., & Zehtabiyan, G. R. (2019). Comparison of the Performance of SWAT, IHACRES and Artificial Neural Networks models in rainfall-runoff simulation (Case study: Kan watershed, Iran). Physics and Chemistry of the Earth, 111, 65–77. https://doi.org/10.1016/j.pce.2019.05.002
- Akhter, M., Malik, M. I., Mehraj, T., Shah, A. F., Ahmad, S., Bhat, W. A., & Mujeeb, S. (2022). Runoff modelling of Aripal watershed using SWAT model. Arabian Journal of Geosciences, 15(16), 1419. https://doi.org/10.1007/s12517-022-10708-z
- Akirso, B., Haile, A., Desta, B., & Itana, B. (2022). Assessment of climate change impact on hydro ‑ climatic variables and its trends over Gidabo Watershed. Modeling Earth Systems and Environment, 8(3), 3769–3791. https://doi.org/10.1007/s40808-021-01327-w
- Al-Areeq, A. M., Al-Zahrani, M. A., & Sharif, H. O. (2021). The performance of physically based and conceptual hydrologic models: A case study for Makkah watershed, Saudi Arabia. Water, 13(8), 1098. https://doi.org/10.3390/w13081098
- Al-Mukhtar, M., & Al-Yaseen, F. (2019). Modeling water quality parameters using. Hydrology, 6(1), 24. https://doi.org/10.3390/hydrology6010024
- Alamdari, N., Sample, D. J., Steinberg, P., Ross, A. C., & Easton, Z. M. (2017). Assessing the effects of climate change on water quantity and quality in an urban watershed using a calibrated stormwater model. Water, 9(7), 464. https://doi.org/10.3390/w9070464
- Aliye, M. A., Aga, A. O., Tadesse, T., & Yohannes, P. (2020). Evaluating the performance of HEC-HMS and SWAT hydrological models in simulating the rainfall-runoff process for data scarce region of Ethiopian Rift Valley Lake Basin. Open Journal of Modern Hydrology, 10(04), 105–122. https://doi.org/10.4236/ojmh.2020.104007
- Althoff, D., & Neiva, L. (2021). Goodness-of-fit criteria for hydrological models: Model calibration and performance assessment. Journal of Hydrology, 600(July), 126674. https://doi.org/10.1016/j.jhydrol.2021.126674
- Ang, R., & Oeurng, C. (2019). Simulating streamflow in an ungauged catchment of Tonlesap Lake Basin in Cambodia using Soil and Water Assessment Tool (SWAT) model Simulating streamflow in an ungauged catchment of Tonlesap Lake Basin in Cambodia using Soil and Water Assessment Tool. Water Science, 32(1), 89–101. https://doi.org/10.1016/j.wsj.2017.12.002
- Anshuman, A., Kunnath, A., & Eldho, P. T. I. (2019). Towards the use of conceptual models for water resource assessment in Indian tropical watersheds under monsoon ‑ driven climatic conditions. Environmental Earth Sciences, 78(9), 1–15. https://doi.org/10.1007/s12665-019-8281-5
- Aqnouy, M., Ahmed, M., Ayele, G. T., Bouizrou, I., Bouadila, A., & Stitou El Messari, J. E. (2023). Comparison of hydrological platforms in assessing rainfall-runoff behavior in a Mediterranean watershed of. Water, 15(3), 447. –https://doi.org/10.3390/w15030447
- Aqnouy, M., Eddine, J., El, S., Ismail, H., Bouadila, A., Gabriel, J., Navarro, M., Loubna, B., Reda, M., & Mansour, A. (2019). Assessment of the SWAT model and the parameters affecting the flow simulation in the watershed of Oued Laou (Northern Morocco). Journal of Ecological Engineering, 20(4), 104–113. https://doi.org/10.12911/22998993/102794
- Aredo, M. R., Hatiye, S. D., & Pingale, S. M. (2021a). Impact of land use/land cover change on stream flow in the Shaya catchment of Ethiopia using the MIKE SHE model. Arabian Journal of Geosciences, 14(2), 114. https://doi.org/10.1007/s12517-021-06447-2
- Aredo, M. R., Hatiye, S. D., & Pingale, S. M. (2021b). Modeling the rainfall-runoff using MIKE 11 NAM model in Shaya catchment, Ethiopia. Modeling Earth Systems and Environment, 7(4), 2545–2551. https://doi.org/10.1007/s40808-020-01054-8
- Arsenault, R., Breton-Dufour, M., Poulin, A., Dallaire, G., & Romero-Lopez, R. (2019). Streamflow prediction in ungauged basins: analysis of regionalization methods in a hydrologically heterogeneous region of Mexico. Hydrological Sciences Journal, 64(11), 1297–1311. https://doi.org/10.1080/02626667.2019.1639716
- Arsenault, R., Gatien, P., Renaud, B., Brissette, F., & Martel, J. (2015). A comparative analysis of 9 multi-model averaging approaches in hydrological continuous streamflow simulation. Journal of Hydrology, 529, 754–767. https://doi.org/10.1016/j.jhydrol.2015.09.001
- Atanaw, S. B., Zimale, F. A., Ayenew, T., & Ayele, G. T. (2023). Modeling future hydrological responses through parameter optimization and climate change scenarios in Dirima Watershed, Ethiopia. Modeling Earth Systems and Environment, 10(1), 1117–1135. https://doi.org/10.1007/s40808-023-01817-z
- Atif, I., Iqbal, J., & Su, L. J. (2019). Modeling hydrological response to climate change in a data-scarce glacierized high mountain Astore basin using a fully distributed TOPKAPI model. Climate, 7(11), 127. https://doi.org/10.3390/cli7110127
- Ávila, L., Silveira, R., Campos, A., Rogiski, N., Gonçalves, J., Scortegagna, A., Freita, C., Aver, C., & Fan, F. (2022). Comparative evaluation of five hydrological models in a large-scale and tropical river basin. Water, 14(19), 3013. https://doi.org/10.3390/w14193013
- Ayalew, D. W., Petroselli, A., De Luca, D. L., & Grimaldi, S. (2022). An evidence for enhancing the design hydrograph estimation for small and ungauged basins in Ethiopia. Journal of Hydrology: Regional Studies, 42(May), 101123. https://doi.org/10.1016/j.ejrh.2022.101123
- Azad, A., Farzin, S., Kashi, H., Sanikhani, H., Karami, H., & Kisi, O. (2018). Prediction of river flow using hybrid neuro-fuzzy models. Arabian Journal of Geosciences, 11(22), 718. https://doi.org/10.1007/s12517-018-4079-0
- Balcha, S. K., Awass, A. A., Hulluka, T. A., Bantider, A., & Ayele, G. T. (2023). Assessment of future climate change impact on water balance components in Central Rift Valley Lakes Basin, Ethiopia. Journal of Water and Climate Change, 14(1), 175–199. https://doi.org/10.2166/wcc.2022.249
- Banda, V. D., Dzwairo, R. B., Singh, S. K., & Kanyerere, T. (2022). Hydrological modelling and climate adaptation under changing climate: A review with a focus in Sub-Saharan Africa. Water, 14(24), 4031. https://doi.org/10.3390/w14244031
- Barrientos, G., Herrero, A., Iroumé, A., Mardones, O., & Batalla, R. J. (2020). Modelling the effects of changes in forest cover and climate on hydrology of headwater catchments in. Water, 12(6), 1828. https://doi.org/10.3390/w12061828
- Bastola, S., Murphy, C., & Sweeney, J. (2011). The role of hydrological modelling uncertainties in climate change impact assessments of Irish river catchments. Advances in Water Resources, 34(5), 562–576. https://doi.org/10.1016/j.advwatres.2011.01.008
- Beg, N. A., Leandro, J., Bhola, P., Konnerth, I., Willems, W., Carvalho, R. F., & Disse, M. (2019). Discharge Interval method for uncertain flood forecasts using a flood model chain: city of Kulmbach. Journal of Hydroinformatics, 21(5), 925–944. |, https://doi.org/10.2166/hydro.2019.131
- Bekele, D., Alamirew, T., Kebede, A., Zeleke, G., & M. Melesse, A. (2019). Modeling climate change impact on the hydrology of Keleta watershed in the Awash River Basin, Ethiopia. Environmental Modeling & Assessment, 24(1), 95–107. https://doi.org/10.1007/s10666-018-9619-1
- Bian, G., Zhang, J., Song, M., Qian, X., Guan, T., & Wang, G. (2023). Projections of flood regime changes over the upper-middle Huaihe River Basin in China based on CMIP6 models. Frontiers in Environmental Science, 11, 1–16. https://doi.org/10.3389/fenvs.2023.1247753
- Bibi, T. S. (2022). Modeling urban stormwater management in the town of Dodola based on landuse and climate change using SWMM 5. Journal of Hydrology: Regional Studies, 44(November), 101267. https://doi.org/10.1016/j.ejrh.2022.101267
- Bingeman, A. K., Kouwen, N., & Soulis, E. D. (2006). Validation of the hydrological processes in a hydrological model. Journal of Hydrologic Engineering, 11(5), 451–463.) https://doi.org/10.1061/(ASCE)1084-0699(2006)11:5(451)
- Birocchi, P., Augusto, D., Dalton, S., Sasaki, K., & Dottori, M. (2023). Estimating river discharge from rainfall satellite data through simple statistical models. Theoretical and Applied Climatology, 153(1–2), 241–261. https://doi.org/10.1007/s00704-023-04459-4
- Bizuneh, B. B., Moges, M. A., Sinshaw, B. G., & Kerebih, M. S. (2021). SWAT and HBV models’ response to streamflow estimation in the upper Blue Nile Basin, Ethiopia. Water-Energy Nexus, 4, 41–53. https://doi.org/10.1016/j.wen.2021.03.001
- Boisvert, J., El-Jabi, N., St-Hilaire, A., & Adlouni, S. E. (2016). Parameter estimation of a distributed hydrological model using a genetic algorithm. Open Journal of Modern Hydrology, 06(03), 151–167. https://doi.org/10.4236/ojmh.2016.63013
- Bouadila, A., Bouizrou, I., Aqnouy, M., En-Nagre, K., El-Yousf, Y., Khafouri, A., Hilal, I., Kamal, A., Lahcen, B., Tamer, A.-A., Eddine, J., Messari, E., & Abioui, M. (2023). Streamflow simulation in semiarid data-scarce regions. Water, 15(8), 1602. https://doi.org/10.3390/w15081602
- Bournas, A., & Baltas, E. (2021). Increasing the efficiency of the Sacramento model on event basis in a mountainous River Basin. Environmental Processes, 8(2), 943–958. https://doi.org/10.1007/s40710-021-00504-4
- Buddika, D., & Coulibaly, P. (2020). Identification of hydrological models for operational flood forecasting in St. John’s, Newfoundland, Canada. Journal of Hydrology: Regional Studies, 27(March 2019), 100646. https://doi.org/10.1016/j.ejrh.2019.100646
- Bugaets, A., Gartsman, B., Gubareva, T., Lupakov, S., Kalugin, A., Shamov, V., & Gonchukov, L. (2023). Comparing the runoff decompositions of small experimental catchments: End-member mixing analysis (EMMA) vs. Hydrological modelling. Water, 15(4), 752. https://doi.org/10.3390/w15040752
- Cenobio-Cruz, O., Quintana-Seguí, P., Barella-Ortiz, A., Zabaleta, A., Garrote, L., Clavera-Gispert, R., Habets, F., & Beguería, S. (2023). Improvement of low flows simulation in the SASER hydrological modeling chain. Journal of Hydrology, 18(September 2022), 100147. https://doi.org/10.1016/j.hydroa.2022.100147
- Chathuranika, I. M., Gunathilake, M. B., Baddewela, P. K., Sachinthanie, E., Babel, M. S., Shrestha, S., Jha, M. K., & Rathnayake, U. S. (2022). Comparison of two hydrological models, HEC-HMS and SWAT in runoff estimation: Application to Huai Bang Sai Tropical Watershed, Thailand. Fluids, 7(8), 267. https://doi.org/10.3390/fluids7080267
- Chilkoti, V., Bolisetti, T., & Balachandar, R. (2018). Multi-objective autocalibration of SWAT model for improved low flow performance for a small snowfed catchment. Hydrological Sciences Journal, 63(10), 1482–1501. https://doi.org/10.1080/02626667.2018.1505047
- Costa, D., Avelino, R., Sára, S., Thomazini, L., Aurélio, M., & Caiado, C. (2015). Application of the SWAT hydrologic model to a tropical watershed at Brazil. CATENA, 125, 206–213. https://doi.org/10.1016/j.catena.2014.10.032
- Cui, T., Yang, T., Xu, C.-Y., Shao, Q., Wang, X., & Li, Z. (2018). Assessment of the impact of climate change on flow regime at multiple temporal scales and potential ecological implications in an alpine river. Stochastic Environmental Research and Risk Assessment, 32(6), 1849–1866. https://doi.org/10.1007/s00477-017-1475-z
- Darbandsari, P., & Coulibaly, P. (2020). Inter-comparison of lumped hydrological models in data-scarce watersheds using different precipitation forcing data sets: Case study of Northern Ontario, Canada. Journal of Hydrology: Regional Studies, 31(August), 100730. https://doi.org/10.1016/j.ejrh.2020.100730
- De Luca, D. L., Apollonio, C., & Petroselli, A. (2022). The benefit of continuous hydrological modelling for drought hazard assessment in small and coastal ungauged basins: A case study in Southern Italy. Climate, 10(3), 34. https://doi.org/10.3390/cli10030034
- Dehua, Z., Samiran, D., & Qiwei, R. (2017). Hydrological appraisal of climate change impacts on the water resources of the Xijiang Basin. Water, 9(10), 793. https://doi.org/10.3390/w9100793
- Dessalegn, T. A., Moges, M. A., Dagnew, D. C., & Gashaw, A. (2017). Applicability of galway river flow forecasting and modeling system (GFFMS) for Lake Tana Basin, Ethiopia. Journal of Water Resource and Protection, 09(12), 1319–1334. https://doi.org/10.4236/jwarp.2017.912084
- Devi, G. K., Ganasri, B. P., & Dwarakish, G. S. (2015). A review on hydrological models. ScienceDirect, 4(Icwrcoe), 1001–1007. https://doi.org/10.1016/j.aqpro.2015.02.126
- Dibaba, W. T., Demissie, T. A., & Miegel, K. (2020). Watershed hydrological response to combined land use/land cover and climate change in highland. Water, 12(6), 1801. https://doi.org/10.3390/w12061801
- Didovets, I., Lobanova, A., Bronstert, A., Snizhko, S., Maule, C. F., & Krysanova, V. (2017). Assessment of climate change impacts on water catchments using eco-hydrological modelling. Water, 9(3), 204. https://doi.org/10.3390/w9030204
- Diriba, B. T. (2021). Surface runoff modeling using SWAT analysis in Dabus watershed, Ethiopia. Sustainable Water Resources Management, 7(6), 1–11. https://doi.org/10.1007/s40899-021-00573-1
- Doliso, B. D., & Hatiye, S. D. (2021). Comparative performance evaluation of HEC-HMS and SWAT models in stream flow simulation: The case of Bilate and Gidabo Watersheds, Ethiopia. Ethiopian Journal of Water Science and Technology, 4, 90–122. https://doi.org/10.59122/xc8543210
- Edamo, M. L., Bushira, K. M., Ukumo, T. Y., Ayele, M. A., Alaro, M. A., & Borko, H. B. (2022). Effect of climate change on water availability in Bilate catchment, Southern Ethiopia. Water Cycle, 3(December 2021), 86–99. https://doi.org/10.1016/j.watcyc.2022.06.001
- Elsanabary, M. H., & Gan, T. Y. (2015). Evaluation of climate anomalies impacts on the Upper Blue Nile Basin in Ethiopia using a distributed and a lumped hydrologic model. Journal of Hydrology, 530, 225–240. https://doi.org/10.1016/j.jhydrol.2015.09.052
- Eshete, D. G., Rigler, G., Shinshaw, B. G., Belete, A. M., & Bayeh, B. A. (2022). Evaluation of streamflow response to climate change in the data-scarce region, Ethiopia. Sustainable Water Resources Management, 8(6), 1–16. https://doi.org/10.1007/s40899-022-00770-6
- Faiz, N. M., Cortes, A. L., Guy, J. S., Reddy, S. M., & Gimeno, I. M. (2018). Differential attenuation of Marek’s disease virus-induced tumours and late-Marek’s disease virus-induced immunosuppression. The Journal of General Virology, 99(7), 927–936. https://doi.org/10.1099/jgv.0.001076
- Fanta, S. S., & Sime, C. H. (2022). Performance assessment of SWAT and HEC-HMS model for runoff simulation of Toba watershed, Ethiopia. Sustainable Water Resources Management, 8(1), 1–16. https://doi.org/10.1007/s40899-021-00596-8
- Farfán, J. F., Palacios, K., Ulloa, J., & Avilés, A. (2020). A hybrid neural network-based technique to improve the flow forecasting of physical and data-driven models : Methodology and case studies in Andean watersheds. Journal of Hydrology: Regional Studies, 27(December 2019), 100652. https://doi.org/10.1016/j.ejrh.2019.100652
- Fentaw, F., Hailu, D., Nigussie, A., & Melesse, A. M. (2018). Climate change impact on the hydrology of Tekeze Basin, Ethiopia: Projection of rainfall-runoff for future water resources planning. Water Conservation Science and Engineering, 3(4), 267–278. https://doi.org/10.1007/s41101-018-0057-3
- Ferreira, R. G., da Silva, D. D., Elesbon, A. A. A., Fernandes-Filho, E. I., Veloso, G. V., de Souza Fraga, M., & Ferreira, L. B. (2021). Machine learning models for streamflow regionalization in a tropical watershed. Journal of Environmental Management, 280, 111713. https://doi.org/10.1016/j.jenvman.2020.111713
- Fita, T., & Abate, B. (2022). Impact of climate change on stream flow of Melka Wakena catchment, Upper Wabi Shebelle sub-basin, south-eastern Ethiopia. Journal of Water and Climate Change, 13(5), 1995–2010. https://doi.org/10.2166/wcc.2022.191
- Fniguire, F., Laftouhi, N. E., Al-Mahfadi, A. S., El Himer, H., Khalil, N., & Saidi, M. E. (2022). Hydrological modelling using the distributed hydrological model CEQUEAU in a semi-arid mountainous area: a case study of Ourika watershed, Marrakech Atlas, Morocco. Euro-Mediterranean Journal for Environmental Integration, 7(1), 89–102. https://doi.org/10.1007/s41207-021-00287-y
- Fouchal, A., & Souag, D. (2019). Long ‑ term monthly streamflow forecasting in humid and semiarid regions. Acta Geophysica, 67(4), 1223–1240. https://doi.org/10.1007/s11600-019-00312-3
- Fowler, K., Peel, M., Western, A., & Zhang, L. (2018). Improved rainfall-runoff calibration for drying climate: Choice of objective function. Water Resources Research, 54(5), 3392–3408. https://doi.org/10.1029/2017WR022466
- Gao, C., Yao, M. T., Wang, Y. J., Zhai, J. Q., Buda, S., Fischer, T., Zeng, X. F., Yao, M. T., Wang, Y. J., Zhai, J. Q., Buda, S., Fischer, T., & Zeng, X. F. (2016). Hydrological model comparison and assessment: Criteria from catchment scales and temporal resolution. Hydrological Sciences Journal, 61(10), 1–11. https://doi.org/10.1080/02626667.2015.1057141
- Gao, J., Holden, J., & Kirkby, M. (2017). Modelling impacts of agricultural practice on flood peaks in upland catchments: An application of the distributed TOPMODEL. Hydrological Processes, 31(23), 4206–4216. https://doi.org/10.1002/hyp.11355
- Gebre, S. L. (2015). Application of the HEC-HMS model for runoff simulation of Upper Blue Nile Hydrology: Current research application of the HEC-HMS model for runoff simulation of Upper Blue Nile River Basin. March. https://doi.org/10.4172/2157-7587.1000199
- Gebre, S. L., & Ludwig, F. (2017). Hydrological response to climate change of the Upper Blue Nile River Basin: Climatology & weather forecasting hydrological response to climate change of the Upper Blue Nile River Basin: Based on IPCC Fifth Assessment Report (AR5). Climatology and Weather Forecasting, 3(1), 1–15. https://doi.org/10.4172/2332-2594.1000121
- Gebrechorkos, S. H., Bernhofer, C., & Hülsmann, S. (2020). Climate change impact assessment on the hydrology of a large river basin in Ethiopia using a local-scale climate modelling approach. The Science of the Total Environment, 742, 140504. https://doi.org/10.1016/j.scitotenv.2020.140504
- Gebremeskel, G., & Kebede, A. (2018). Estimating the effect of climate change on water resources: Integrated use of climate and hydrological models in the Werii watershed of the Tekeze river basin, Northern Ethiopia. Agriculture and Natural Resources, 52(2), 195–207. https://doi.org/10.1016/j.anres.2018.06.010
- Gebremichael, H. B., Raba, G. A., Beketie, K. T., Feyisa, G. L., & Anose, F. A. (2023). Projection of hydrological responses to changing future climate of Upper Awash Basin using QSWAT model. Environmental Systems Research, 12(1), 25. https://doi.org/10.1186/s40068-023-00305-8
- Gelete, G., Nourani, V., Gokcekus, H., & Gichamo, T. (2023). Ensemble physically based semi-distributed models for the rainfall-runoff process modeling in the data-scarce Katar catchment, Ethiopia. Journal of Hydroinformatics, 25(2), 567–592. https://doi.org/10.2166/hydro.2023.197
- Ghosh, A., Roy, M. B., & Roy, P. K. (2022). Evaluating the performance of MIKE NAM model on rainfall–runoff in lower Gangetic floodplain, West Bengal, India. Modeling Earth Systems and Environment, 8(3), 4001–4017. https://doi.org/10.1007/s40808-021-01347-6
- Gizaw, M. S., Biftu, G. F., Gan, T. Y., Moges, S. A. & Koivusalo, H. (2021). Potential impact of climate change on streamflow of major Ethiopian rivers. Climate Change, 143, 371–383. https://doi.org/10.1007/s10584-017-2021-1
- Glick, R., Jeong, J., Srinivasan, R., Arnold, J. G., & Her, Y. (2023). Adaptation of SWAT watershed model for stormwater management in urban catchments: Case study in Austin, Texas. Water, 15(9), 1770. https://doi.org/10.3390/w15091770
- Golmohammadi, G., Prasher, S., Madani, A., & Rudra, R. (2014). Evaluating three hydrological distributed watershed models: MIKE-SHE, APEX, SWAT. Hydrology, 1(1), 20–39. https://doi.org/10.3390/hydrology1010020
- Gumindoga, W., Rientjes, T. H. M., Haile, A. T., & Dube, T. (2014). Predicting streamflow for land cover changes in the Upper Gilgel Abay River Basin, Ethiopia: A TOPMODEL based approach. Journal of Physics and Chemistry of the Earth, 76–78, 3–15. https://doi.org/10.1016/j.pce.2014.11.012
- Haleem, K., Khan, A. U., Ahmad, S., Khan, M., Khan, F. A., Khan, W., & Khan, J. (2022). Hydrological impacts of climate and land-use change on flow regime variations in upper Indus basin. Journal of Water and Climate Change, 13(2), 758–770. https://doi.org/10.2166/wcc.2021.238
- Hasan, M. M., Strong, C., Brooks, P. D., Burian, S. J., & Barber, M. E. (2023). Quantifying climate change impacts on low flows of small high mountain watersheds: A nonstationary approach. Journal of Hydrology: Regional Studies, 48(January 2022), 101463. https://doi.org/10.1016/j.ejrh.2023.101463
- Haznedar, B., Cagan, H., Furkan, K., & Adem, O. (2023). Streamflow forecasting using a hybrid LSTM ‑ PSO approach: The case of Seyhan Basin. Natural Hazards, 117(1), 681–701. https://doi.org/10.1007/s11069-023-05877-3
- Haznedar, B., & Kilinc, H. C. (2022). A hybrid ANFIS-GA approach for estimation of hydrological time series. Water Resources Management, 36(12), 4819–4842. https://doi.org/10.1007/s11269-022-03280-4
- He, S., Gu, L., Tian, J., Deng, L., Yin, J., Liao, Z., Zeng, Z., & Shen, Y. (2021). Machine learning improvement of streamflow simulation by utilizing remote sensing data and potential application in guiding reservoir operation. Sustainability, 13(7), 3645. https://doi.org/10.3390/su13073645
- Himanshu, S. K., Pandey, A., Madolli, M. J., Palmate, S. S., Kumar, A., Patidar, N., & Yadav, B. (2023). An ensemble hydrologic modeling system for runoff and evapotranspiration evaluation over an agricultural watershed. Journal of the Indian Society of Remote Sensing, 51(1), 177–196. https://doi.org/10.1007/s12524-022-01634-4
- Horan, R., Gowri, R., Wable, P. S., Baron, H., Keller, V. D. J., Mujumdar, P. P., Gwyn, R., Houghton-Carr, H., & Rees, G. (2018). Multi-model approaches for improving seasonal ensemble streamflow prediction scheme with various statistical post-processing techniques in the Canadian Prairie Region. Water, 10(11), 1604. https://doi.org/10.3390/w10111604
- Hu, C., Wu, Q., Li, H., Jian, S., Li, N., & Lou, Z. (2018). Deep learning with a long short-term memory networks approach for rainfall-runoff simulation. Water, 10(11), 1543. https://doi.org/10.3390/w10111543
- Huang, T., Yu, D., Cao, Q., & Qiao, J. (2019). Impacts of meteorological factors and land use pattern on hydrological elements in a semi-arid basin. The Science of the Total Environment, 690(19), 932–943. https://doi.org/10.1016/j.scitotenv.2019.07.068
- Hussain, D., & Khan, A. A. (2020). Machine learning techniques for monthly river flow forecasting of Hunza River, Pakistan. Earth Science Informatics, 13(3), 939–949. https://doi.org/10.1007/s12145-020-00450-z
- Ibrahim, U. A., Dan’azumi, S., Bdliya, H. H., Bunu, Z., & Chiroma, M. J. (2022). Comparison of WEAP and SWAT models for streamflow prediction in the Hadejia-Nguru Wetlands, Nigeria. Modeling Earth Systems and Environment, 8(4), 4997–5010. https://doi.org/10.1007/s40808-022-01435-1
- Islam, K. I., Elias, E., Carroll, K. C., & Brown, C. (2023). Exploring random forest machine learning and remote sensing data for streamflow prediction: An alternative approach to a process-based hydrologic modeling in a snowmelt-driven watershed. Remote Sensing, 15(16), 3999. https://doi.org/10.3390/rs15163999
- Islam, Z., & Gan, T. Y. (2015). Potential combined hydrologic impacts of climate change and El Nino southern oscillation to South Saskatchewan River Basin. Journal of Hydrology, 523, 34–48. https://doi.org/10.1016/j.jhydrol.2015.01.043
- Ismail Dhaqane, A., Murshed, M. F., Mourad, K. A., & Abd Manan, T. S. B. (2023). Assessment of the streamflow and evapotranspiration at Wabiga Juba Basin using a water evaluation and planning (WEAP) model. Water, 15(14), 2594. https://doi.org/10.3390/w15142594
- Jackson, E. K., Roberts, W., Nelsen, B., Williams, G. P., Nelson, E. J., & Ames, D. P. (2019). Introductory overview: Error metrics for hydrologic modelling – A review of common practices and an open source library to facilitate use and adoption. Environmental Modelling & Software, 119, 32–48. https://doi.org/10.1016/j.envsoft.2019.05.001
- Janabi, F. A., Ongdas, N., Bernhofer, C., Reyes Silva, J. D., Benisch, J., & Krebs, P. (2021). Assessment of topkapi-x applicability for flood events simulation in two small catchments in saxony. Hydrology, 8(3), 109. https://doi.org/10.3390/hydrology8030109
- Januário, T. E., Pereira Filho, A. J., & Salviano, M. F. (2022). Hydrometeorological modeling of Limpopo River Basin in Mozambique with TOPMODEL and remote sensing. Open Journal of Modern Hydrology, 12(02), 55–73. https://doi.org/10.4236/ojmh.2022.122004
- Jehanzaib, M., Ajmal, M., Achite, M., & Kim, T. W. (2022). Comprehensive review: Advancements in rainfall-runoff modelling for flood mitigation. Climate, 10(10), 147. https://doi.org/10.3390/cli10100147
- Jiang, Y., Liu, C., Li, X., Liu, L., & Wang, H. (2015). Rainfall-runoff modeling, parameter estimation and sensitivity analysis in a semiarid catchment. Environmental Modelling & Software, 67, 72–88. https://doi.org/10.1016/j.envsoft.2015.01.008
- Jimeno-Sáez, P., Senent-Aparicio, J., Pérez-Sánchez, J., & Pulido-Velazquez, D. (2018). A comparison of SWAT and ANN models for daily runoff simulation in different climatic zones of peninsular Spain. Water, 10(2), 192. https://doi.org/10.3390/w10020192
- Jodar-Abellan, A., Valdes-Abellan, J., Pla, C., & Gomariz-Castillo, F. (2019). Impact of land use changes on flash flood prediction using a sub-daily SWAT model in five Mediterranean ungauged watersheds (SE Spain). The Science of the Total Environment, 657, 1578–1591. https://doi.org/10.1016/j.scitotenv.2018.12.034
- Kahsay, K. D., Pingale, S. M., & Hatiye, S. D. (2017). Impact of climate change on groundwater recharge and base flow in the Sub_Catchment of Tekeze Basin, Ethiopia. Groundwater for Sustainable Development, 6, 121–133. https://doi.org/10.1016/j.gsd.2017.12.002
- Kaleris, V., & Langousis, A. (2017). Comparison of two rainfall – runoff models: Effects of conceptualization on water budget components. Hydrological Sciences Journal, 62(5), 729–748. https://doi.org/10.1080/02626667.2016.1250899
- Kamilia, M., Tarik, C., Lmahfoud, A., & Mohamed, T. (2024). Assessing hydrological response to future climate change in the Bouregreg watershed, Morocco. Scientific African, 23, e02046. https://doi.org/10.1016/j.sciaf.2023.e02046
- Kanishka, G., & Eldho, T. I. (2020). Streamflow estimation in ungauged basins using watershed classification and regionalization techniques. Journal of Earth System Science, 129(1), 186. https://doi.org/10.1007/s12040-020-01451-8
- Kapoor, A., Pathiraja, S., Marshall, L., & Chandra, R. (2023). DeepGR4J: A deep learning hybridization approach for conceptual rainfall-runoff modelling. Environmental Modelling & Software, 169(September), 105831. https://doi.org/10.1016/j.envsoft.2023.105831
- Karam, S., Zango, B., Seidou, O., Perera, D., Nagabhatla, N., & Tshimanga, R. M. (2023). Impacts of climate change on hydrological regimes in the Congo River Basin. Sustainability, 15(7), 6066. –https://doi.org/10.3390/su15076066
- Kassem, A. A., Raheem, A. M., Khidir, K. M., & Alkattan, M. (2020). Predicting of daily Khazir basin flow using SWAT and hybrid SWAT-ANN models. Ain Shams Engineering Journal, 11(2), 435–443. https://doi.org/10.1016/j.asej.2019.10.011
- Kavian, A., Javidan, N., Bahrehmand, A., Gyasi, Y., Hazbavi, Z., & Rodrigo-Comino, J. (2020). Assessing the hydrological effects of land-use changes on a catchment using the Markov chain and WetSpa models. Hydrological Sciences Journal, 65(15), 2604–2615. https://doi.org/10.1080/02626667.2020.1797046
- Ketema, A., & Dwarakish, G. S. (2021). Hydro-meteorological impact assessment of climate change on Tikur Wuha watershed in Ethiopia. Sustainable Water Resources Management, 7(4), 1–20. https://doi.org/10.1007/s40899-021-00547-3
- Khan, M., Khan, A. U., Khan, S., & Khan, F. A. (2023). Assessing the impacts of climate change on stream flow dynamics : A machine learning perspective. Water Science & Technology, 88(9), 2309–2331. https://doi.org/10.2166/wst.2023.340
- Khan, S., Khan, A. U., Khan, M., Khan, F. A., Khan, S., & Khan, J. (2023). Intercomparison of SWAT and ANN techniques in simulating stream flows in the Astore Basin of the Upper Indus Sunaid Khan. Water Science & Technology, 88(7), 1847–1862. https://doi.org/10.2166/wst.2023.299
- Khattati, M., Serroukh, M., Rafik, I., & Mesmoudi, H. (2016). Hydrological Modelling of Sidi Jabeur watershed (Morocco) using spatially distributed model ATHYS. Journal of Geoscience and Environment Protection, 4(1), 77–83.
- Khazaee Poul, A., Shourian, M., & Ebrahimi, H. (2019). A comparative study of MLR, KNN, ANN and ANFIS models with wavelet transform in monthly stream flow prediction. Water Resources Management, 33(8), 2907–2923. https://doi.org/10.1007/s11269-019-02273-0
- Khodakhah, H., Aghelpour, P., & Hamedi, Z. (2022). Comparing linear and non ‑ linear data ‑ driven approaches in monthly river flow prediction, based on the models SARIMA, LSSVM, ANFIS, and GMDH. Environmental Science and Pollution Research, 29(15), 21935–21954. https://doi.org/10.1007/s11356-021-17443-0
- Khoi, N. (2016). Comparision of the Hec-Hms and Swat hydrological models in simulating the streamflow. Journal of Science and Technology, 53(5A), 189–195.
- Kilinc, H. C. (2022). Daily streamflow forecasting based on the hybrid particle swarm optimization and long short-term memory model in the Orontes Basin. Water, 14(3), 490. https://doi.org/10.3390/w14030490
- Kilinc, H. C., & Haznedar, B. (2022). A hybrid model for streamflow forecasting in the Basin of Euphrates. Water, 14(1), 80. https://doi.org/10.3390/w14010080
- Koch, H., Lígia, A., Silva, C., Liersch, S., Roberto, J., Azevedo, G. D., & Hattermann, F. F. (2020). Effects of model calibration on hydrological and water resources management simulations under climate change in a semi-arid watershed. Climatic Change, 163, 1247–1266.
- Kouchi, D. H., Esmaili, K., Faridhosseini, A., Sanaeinejad, S. H., Khalili, D., & Abbaspour, K. C. (2017). Sensitivity of calibrated parameters and water resource estimates on different objective functions and optimization algorithms. Water, 9(6), 384. https://doi.org/10.3390/w9060384
- Krysanova, V., & Wechsung, F. (2022). The soil and water integrated model (SWIM) user manual. https://www.pik-potsdam.de/∼wortmann/swim/swim_manual.pdf
- Kuma, H. G., Feyessa, F. F., & Demissie, T. A. (2021). Hydrologic responses to climate and land-use/land-cover changes in the Bilate. Journal of Water and Climate Change, 12(8), 3750–3769. https://doi.org/10.2166/wcc.2021.281
- Kumar, D., & Bhattacharjya, R. K. (2020). Review of different methods and techniques used for flood vulnerability analysis. Natural Hazards and Earth System Sciences Discussions, 1–30. https://doi.org/10.5194/nhess-2020-297
- Kumar, K. S., Galkate, R. V., & Tiwari, H. L. (2021). River basin modelling for Shipra River using MIKE BASIN. ISH Journal of Hydraulic Engineering, 27(2), 188–199. https://doi.org/10.1080/09715010.2018.1534219
- Kumar, R., Huda, M. B., Maryam, M., & Lone, M. A. (2022). Rainfall runoff modeling using MIKE 11 NAM of the Jhelum river in Kashmir Valley, India. MAUSAM, 73(2), 365–372. https://doi.org/10.54302/mausam.v73i2.804
- Kumar, V., Azamathulla, H. M., Sharma, K. V., Mehta, D. J., & Maharaj, K. T. (2023). The state of the art in deep learning applications, challenges, and future prospects: A comprehensive review of flood forecasting and management. Sustainability, 15(13), 10543. https://doi.org/10.3390/su151310543
- Kunnath-Poovakka, A., & Eldho, T. I. (2019). A comparative study of conceptual rainfall-runoff models GR4J, AWBM and Sacramento at catchments in the upper Godavari river basin, India. Journal of Earth System Science, 128(2), 1–15. https://doi.org/10.1007/s12040-018-1055-8
- Kurian, C., Sudheer, K. P., Krishna, V., & Sahoo, D. (2020). Effective flood forecasting at higher lead times through hybrid modelling framework. Journal of Hydrology, 587(April), 124945. https://doi.org/10.1016/j.jhydrol.2020.124945
- Lafdani, E. K., Nia, A. M., Ahmadi, A., Jajarmizadeh, M., Ghafari, M., & Neural, A. (2013). Short communication stream flow simulation using SVM, ANFIS and NAM models (a case study). Caspian Journal of Applied Sciences Research, 2(4), 86–93.
- Lakew, H. B. (2020). Investigating the effectiveness of bias correction and merging MSWEP with gauged rainfall for the hydrological simulation of the upper Blue Nile basin. Journal of Hydrology: Regional Studies, 32(October), 100741. https://doi.org/10.1016/j.ejrh.2020.100741
- Lamontagne, J. R., Barber, C. A., & Vogel, R. M. (2020). Improved estimators of model performance efficiency for skewed hydrologic data. Water Resources Research, 56(9), 1–25. https://doi.org/10.1029/2020WR027101
- Laura, R., Lay, L., Federico, G., Moine, L., Frédéric, H., Céline, M., & Pierre, R. (2017). Impact of mesoscale spatial variability of climatic inputs and parameters on the hydrological response. Journal of Hydrology, 553, 13–25. https://doi.org/10.1016/j.jhydrol.2017.07.037
- Lavenne, A. D., Thirel, G., Andréassian, V., Perrin, C., Ramos, M., & Cedex, A. (2016). Spatial variability of the parameters of a semi-distributed hydrological model. Proceedings of the International Association of Hydrological Sciences, 373, 87–94. https://doi.org/10.5194/piahs-373-87-2016
- Lee, J. S., & Choi, H. I. (2021). Improved streamflow calibration of a land surface model by the choice of objective functions-a case study of the Nakdong river watershed in the Korean peninsula. Water (Switzerland), 13(12), 1709. https://doi.org/10.3390/w13121709
- Leila, N., Mahtabi, G., Seyyed, H. H., & Venkata, S. R. (2024). Hydrological responses to future climate change in semi-arid region of Iran (Golabar and Taham Basins, Zanjan Province). Results in Engineering, 21, 101871. https://doi.org/10.1016/j.rineng.2024.101871
- Li, C., Cai, Y., Li, Z., Zhang, Q., Sun, L., Li, X., & Zhou, P. (2022). Hydrological response to climate and land use changes in the dry–warm valley of the Upper Yangtze River. Engineering, 19, 24–39. https://doi.org/10.1016/j.eng.2021.04.029
- Li, F., Xu, Z., Liu, W., & Zhang, Y. (2014). The impact of climate change on runoff in the Yarlung Tsangpo River basin in the Tibetan Plateau. Stochastic Environmental Research and Risk Assessment, 28(3), 517–526. https://doi.org/10.1007/s00477-013-0769-z
- Li, Z., Yu, J., Xu, X., Sun, W., Pang, B., & Yue, J. (2018). Multi-model ensemble hydrological simulation using a BP Neural Network for the upper Yalongjiang River Basin, China. Proceedings of the International Association of Hydrological Sciences, 379, 335–341. https://doi.org/10.5194/piahs-379-335-2018
- Lin, Y., Wen, H., & Liu, S. (2019). Surface runoff response to climate change based on artificial neural network (ANN) models: A case study with Zagunao catchment in Upper Minjiang River, Southwest China. Journal of Water and Climate Change, 10(1), 158–166. https://doi.org/10.2166/wcc.2018.130
- Liu, J., Liu, T., Bao, A., De Maeyer, P., Feng, X., Miller, S. N., & Chen, X. (2016). Assessment of different modelling studies on the spatial hydrological processes in an Arid Alpine catchment. Water Resources Management, 30(5), 1757–1770. https://doi.org/10.1007/s11269-016-1249-2
- Liu, Y., Zhang, J., Yang, Q., Zhou, X., & Wang, G. (2021). Changes in and modelling of hydrological process for a semi-arid catchment in the context of human disturbance. Frontiers in Earth Science, 9(September 2021), 1–11. https://doi.org/10.3389/feart.2021.759534
- Ludwig, P., Ehmele, F., Franca, M. J., Mohr, S., Caldas-Alvarez, A., Daniell, J. E., Ehret, U., Feldmann, H., Hundhausen, M., Knippertz, P., Küpfer, K., Kunz, M., Mühr, B., Pinto, J. G., Quinting, J., Schäfer, A. M., Seidel, F., & Wisotzky, C. (2023).). A multi-disciplinary analysis of the exceptional flood event of July 2021 in central Europe – Part 2: Historical context and relation to climate change. Natural Harzards and Earth System Sciences, 23(2), 1287–1311.
- Ma, J., Sun, W., Yang, G., & Zhang, D. (2018). Hydrological analysis using satellite remote sensing big data and CREST model. IEEE Access, 6, 9006–9016. https://doi.org/10.1109/ACCESS.2018.2810252
- Ma, L., He, C., Bian, H., & Sheng, L. (2022). MIKE SHE modeling of ecohydrological processes: Merits, applications, and challenges. Ecological Engineering, 96, 137–149. https://doi.org/10.1016/j.ecoleng.2016.01.008
- Mai, J., Arsenault, R., Tolson, B. A., Latraverse, M., & Demeester, K. (2020). Application of parameter screening to derive optimal initial state adjustments for streamflow forecasting. Water Resources Research, 56(9), 1–22. https://doi.org/10.1029/2020WR027960
- Malik, M. A., Dar, A. Q., & Jain, M. K. (2022). Modelling streamflow using the SWAT model and multi-site calibration utilizing SUFI-2 of SWAT-CUP model for high altitude catchments, NW Himalaya’s. Modeling Earth Systems and Environment, 8(1), 1203–1213. https://doi.org/10.1007/s40808-021-01145-0
- Martel, J., Brissette, F., & Arsenault, R. (2017). HMETS—A simple and efficient hydrology model for teaching hydrological modelling, flow forecasting and climate change impacts. International Journal of Engineering Education, 33(4), 1307–1316.
- Mekonnen, H., Surendra, A., Mishra, K., & Kumar, M. (2023). Assessing the impact of climate change on the hydrology of Gidabo River sub ‑ basin, Ethiopian Rift Valley Lakes Basin. Sustainable Water Resources Management, 9(3), 1–18. https://doi.org/10.1007/s40899-023-00858-7
- Mekonnen, S., Dessie, M., Tadesse, A., Nega, H., & Zewdu, A. (2023). Predicting the daily flow in ungauged catchments of the eastern part of the upper Blue Nile basin, Ethiopia. Sustainable Water Resources Management, 9(3), 71. https://doi.org/10.1007/s40899-023-00856-9
- Meresa, H. K., & Gatachew, M. T. (2019). Climate change impact on river flow extremes in the Upper Blue Nile River basin. Journal of Water and Climate Change, 10(4), 759–781. https://doi.org/10.2166/wcc.2018.154
- Merga, D. D., Adeba, D., Regasa, M. S., & Leta, M. K. (2022). Evaluation of surface water resource availability under the impact of climate change in the Dhidhessa Sub-Basin, Ethiopia. Atmosphere, 13(8), 1296. https://doi.org/10.3390/atmos13081296
- Moges, M. A., Schmitter, P., Tilahun, S. A., Langan, S., Dagnew, D. C., Akale, A. T., & Steenhuis, T. S. (2017). Suitability of watershed models to predict distributed hydrologic response in the Awramba Watershed in Lake Tana Basin. Land Degradation & Development, 28(4), 1386–1397. https://doi.org/10.1002/ldr.2608
- Mohammadi, B., Safari, M. J. S., & Vazifehkhah, S. (2022). OPEN IHACRES, GR4J and MISD ‑ based multi conceptual ‑ machine learning approach for rainfall ‑ runoff modeling. Scientific Reports, 12(1), 12096. https://doi.org/10.1038/s41598-022-16215-1
- Mohammady, M., Moradi, H. R., Zeinivand, H., Temme, A. J. A. M., Yazdani, M. R., & Pourghasemi, H. R. (2018). Modeling and assessing the effects of land use changes on runoff generation with the CLUE-s and WetSpa models. Theoretical and Applied Climatology, 133(1–2), 459–471. https://doi.org/10.1007/s00704-017-2190-x
- Mosbahi, M., & Benabdallah, S. (2020). Assessment of land management practices on soil erosion using SWAT model in a Tunisian semi-arid catchment. Journal of Soils and Sediments, 20(2), 1129–1139. https://doi.org/10.1007/s11368-019-02443-y
- Mouche, E., Moussu, F., Mügler, C., Ribolzi, O., Valentin, C., Sengtahevanghoung, O., & Lacombe, G. (2014). Impact of land-use change on the hydrology of North Lao PDR watersheds. IAHS-AISH Proceedings and Reports, 363, 84–89.
- Mubialiwo, A., Abebe, A., & Onyutha, C. (2021). Performance of rainfall–runoff models in reproducing hydrological extremes: a case of the River Malaba sub-catchment. SN Applied Sciences, 3(4), 515. https://doi.org/10.1007/s42452-021-04514-7
- Muhury, N., Ayele, G. T., Balcha, S. K., Jemberie, M. A., & Teferi, E. (2023). Basin runoff responses to climate change using a rainfall-runoff hydrological model in Southeast Australia. Atmosphere, 14(2), 306. https://doi.org/10.3390/atmos14020306
- Munoth, P., & Goyal, R. (2020). Hydromorphological analysis of Upper Tapi River Sub-basin, India, using QSWAT model. Modeling Earth Systems and Environment, 6(4), 2111–2127. https://doi.org/10.1007/s40808-020-00821-x
- Naha, S., Rico-Ramirez, M. A., & Rosolem, R. (2021). Quantifying the impacts of land cover change on hydrological responses in the Mahanadi river basin in India. Hydrology and Earth System Sciences, 25(12), 6339–6357. https://doi.org/10.5194/hess-25-6339-2021
- Nannawo, A. S., Lohani, T. K., Eshete, A. A., & Ayana, M. T. (2022). Evaluating the dynamics of hydroclimate and streamflow for data-scarce areas using MIKE11-NAM model in Bilate river basin, Ethiopia. Modeling Earth Systems and Environment, 8(4), 4563–4578. https://doi.org/10.1007/s40808-022-01455-x
- Narsimlu, B., Gosain, A. K., Chahar, B. R., Singh, S. K., & Srivastava, P. K. (2015). SWAT model calibration and uncertainty analysis for streamflow prediction in the Kunwari River Basin, India, using sequential uncertainty fitting. Environmental Processes, 2(1), 79–95. https://doi.org/10.1007/s40710-015-0064-8
- Nayeb, M., Ketabchy, M., Sample, D. J., Scott, D., & Liao, H. (2019). An evaluation of HSPF and SWMM for simulating streamflow regimes in an urban watershed. Environmental Modelling & Software, 118(March), 211–225. https://doi.org/10.1016/j.envsoft.2019.05.008
- Ndhlovu, G. Z., & Woyessa, Y. E. (2021). Evaluation of streamflow under climate change in the Zambezi river basin of Southern Africa. Water, 13(21), 3114. https://doi.org/10.3390/w13213114
- Nesru, M. (2023). A review of model selection for hydrological studies. Arabian Journal of Geosciences, 16(2), 102. https://doi.org/10.1007/s12517-023-11194-7
- Nguyen, S., & Bouvier, C. (2019). Flood modelling using the distributed event-based SCS-LR model in the Mediterranean Real Collobrier catchment. Hydrological Sciences Journal, 64(11), 1351–1369. https://doi.org/10.1080/02626667.2019.1639715
- Nilawar, A. P., & Waikar, M. L. (2018). Use of SWAT to determine the effects of climate and land use changes on streamflow and sediment concentration in the Purna River basin, India. Environmental Earth Sciences, 77(23), 1–13. https://doi.org/10.1007/s12665-018-7975-4
- Ning, Z., Zhang, J., Yuan, S., & Wang, G. (2022). Impact of climate change on water resources in the western route areas of the South-to-North water diversion project. Atmosphere, 13(5), 799.
- Nivesh, S., Patil, J. P., Goyal, V. C., Saran, B., Singh, A. K., Raizada, A., Malik, A., & Kuriqi, A. (2022). Assessment of future water demand and supply using WEAP model in Dhasan River Basin, Madhya Pradesh, India. Environmental Science and Pollution Research, 30(10), 27289–27302. https://doi.org/10.1007/s11356-022-24050-0
- Noori, N., & Kalin, L. (2016). Coupling SWAT and ANN models for enhanced daily streamflow prediction. Journal of Hydrology, 533, 141–151. https://doi.org/10.1016/j.jhydrol.2015.11.050
- Nourali, M., Ghahraman, B., Pourreza-Bilondi, M., & Davary, K. (2016). Effect of formal and informal likelihood functions on uncertainty assessment in a single event rainfall-runoff model. Journal of Hydrology, 540, 549–564. https://doi.org/10.1016/j.jhydrol.2016.06.022
- Nuri, M., & Abdüsselam, B. (2020). The impacts of climate change on the runoff volume of Melen and Munzur Rivers in Turkey based on calibration of WASMOD model with multiobjective genetic algorithm. Meteorology and Atmospheric Physics, 132(1), 85–98. https://doi.org/10.1007/s00703-019-00676-7
- Ocio, D., Beskeen, T., & Smart, K. (2020). Fully distributed hydrological modelling for catchment-wide hydrological data verification. Hydrology Research, 50(6), 1520–1534. https://doi.org/10.2166/nh.2019.006
- Okiria, E., Okazawa, H., Noda, K., Kobayashi, Y., Suzuki, S., & Yamazaki, Y. (2022). A comparative evaluation of lumped and semi-distributed conceptual hydrological models: Does model complexity enhance hydrograph prediction? Hydrology, 9(5), 89. https://doi.org/10.3390/hydrology9050089
- Okkan, U., Beril, Z., Ali, A., & Fistikoglu, O. (2021). Embedding machine learning techniques into a conceptual model to improve monthly runoff simulation: A nested hybrid rainfall-runoff modeling. Journal of Hydrology, 598(May), 126433. https://doi.org/10.1016/j.jhydrol.2021.126433
- Olaoye, I. A., Confesor, R. B., & Ortiz, J. D. (2021). Impact of seasonal variation in climate on water quality of old woman creek watershed Ohio using swat. Climate, 9(3), 50. https://doi.org/10.3390/cli9030050
- Onyutha, C. (2019). Hydrological model supported by a step-wise calibration against sub-flows and validation of extreme flow events. Water, 11(2), 244. https://doi.org/10.3390/w11020244
- Onyutha, C., Amollo, C. J., Nyende, J., & Nakagiri, A. (2021). Suitability of averaged outputs from multiple rainfall-runoff models for hydrological extremes: a case of River Kafu catchment in East Africa. International Journal of Energy and Water Resources, 5(1), 43–56. https://doi.org/10.1007/s42108-020-00075-4
- Orkodjo, T. P., Kranjac-Berisavijevic, G., & Abagale, F. K. (2022). Impact of climate change on future precipitation amounts, seasonal distribution, and streamflow in the Omo-Gibe basin, Ethiopia. Heliyon, 8(6), e09711. https://doi.org/10.1016/j.heliyon.2022.e09711
- Osman, S., Chen, L., Hafiz, A., Xing, L., & Chen, Y. (2021). Flood modeling of Sungai Pinang Watershed under the impact of urbanization. Tropical Cyclone Research and Review, 10(2), 96–105. https://doi.org/10.1016/j.tcrr.2021.06.001
- Paul, M., & Negahban-Azar, M. (2018). Sensitivity and uncertainty analysis for streamflow prediction using multiple optimization algorithms and objective functions: San Joaquin Watershed, California. Modeling Earth Systems and Environment, 4(4), 1509–1525. https://doi.org/10.1007/s40808-018-0483-4
- Lucas‐Picher, P., Arsenault, R., Poulin, A., Ricard, S., Lachance‐Cloutier, S., & Turcotte, R. (2020). Application of a high ‐ resolution distributed hydrological model on a U. S. ‐ Canada Transboundary Basin: Simulation of the multiyear mean annual hydrograph and 2011 flood of the Richelieu river basin journal of advances in modeling earth systems. Journal of Advances in Modeling Earth Systems, 12(4), 1–20. https://doi.org/10.1029/2019MS001709
- Poonia, V., & Tiwari, H. L. (2020). Rainfall-runoff modeling for the Hoshangabad Basin of Narmada River using artificial neural network. Arabian Journal of Geosciences, 13(18), 944. https://doi.org/10.1007/s12517-020-05930-6
- Rabba, Z. A. (2023). Flood frequency analysis with PyTOPKAPI model ‑ simulated stream flows from Aweitu river in Jimma town, Ethiopia. Sustainable Water Resources Management, 9(2), 1–14. https://doi.org/10.1007/s40899-023-00825-2
- Rahman, K. U., Pham, Q. B., Jadoon, K. Z., Shahid, M., Kushwaha, D. P., Duan, Z., Mohammadi, B., Khedher, K. M., & Anh, D. T. (2022). Comparison of machine learning and process-based SWAT model in simulating streamflow in the Upper Indus Basin. Applied Water Science, 12(8), 1–19. https://doi.org/10.1007/s13201-022-01692-6
- Ranjan, S., & Singh, V. (2022). HEC-HMS based rainfall-runoff model for Punpun river basin. Water Practice and Technology, 17(5), 986–1001. https://doi.org/10.2166/wpt.2022.033
- Reddy, N. M., Saravanan, S., Almohamad, H., Al Dughairi, A. A., & Abdo, H. G. (2023). Effects of climate change on streamflow in the godavari basin simulated using a conceptual model including CMIP6 dataset. Water, 15(9), 1701. https://doi.org/10.3390/w15091701
- Reddy, N. N., Reddy, K. V., Vani, J. S. L. S., Daggupati, P., & Srinivasan, R. (2018). Climate change impact analysis on watershed using QSWAT. Spatial Information Research, 26(3), 253–259. https://doi.org/10.1007/s41324-017-0159-6
- Ren, W. W., Yang, T., Sheng, C., Chong, H., Xu, Y., & Xi, Q. (2018). Improving monthly streamflow prediction in alpine regions: Integrating HBV model with Bayesian neural network. Stochastic Environmental Research and Risk Assessment, 32(12), 3381–3396. https://doi.org/10.1007/s00477-018-1553-x
- Ricci, G. F., De Girolamo, A. M., Abdelwahab, O. M. M., & Gentile, F. (2018). Identifying sediment source areas in a Mediterranean watershed using the SWAT model. Land Degradation & Development, 29(4), 1233–1248. https://doi.org/10.1002/ldr.2889
- Ruiz-García, V. H., Asensio-Grima, C., Ramírez-García, A. G., & Monterroso-Rivas, A. I. (2023). The hydrological balance in micro-watersheds is affected by climate change and land use changes. Applied Sciences, 13(4), 2503. https://doi.org/10.3390/app13042503
- Sahraei, S., Asadzadeh, M., & Unduche, F. (2020). Calibration of hydrologic models: Application in flood. Journal of Hydrology, 588, 125095. https://doi.org/10.1016/j.jhydrol.2020.125095
- Sajadi Bami, Y., Porhemmat, J., Sedghi, H., & Jalalkamali, N. (2020). Performance evaluation of Mike Nam Rainfall-Runoff (R-R) model in daily flow simulation (case study: Gonbad Catchment in Hamedan). Journal of Applied Engineering Sciences, 10(1), 1–6. https://doi.org/10.2478/jaes-2020-0001
- Sanjay Shekar, N. C., & Vinay, D. C. (2021). Performance of HEC-HMS and swat to simulate streamflow in the sub-humid tropical Hemavathi Catchment. Journal of Water and Climate Change, 12(7), 3005–3017. https://doi.org/10.2166/wcc.2021.072
- Santos, L., Andersson, J. C. M., & Arheimer, B. (2022). Evaluation of parameter sensitivity of a rainfall- runoff model over a global catchment set. Hydrological Sciences Journal, 67(3), 342–357. https://doi.org/10.1080/02626667.2022.2035388
- Saran, S., Sterk, G., Aggarwal, S. P., & Dadhwal, V. K. (2021). Coupling remote sensing and GIS with KINEROS2 model for spatially distributed runoff modeling in a Himalayan watershed. Journal of the Indian Society of Remote Sensing, 49(5), 1121–1139. https://doi.org/10.1007/s12524-020-01295-1
- Sempewo, J. I., Twite, D., Nyenje, P., & Mugume, S. N. (2022). Comparison of SWAT and HEC-HMS model performance in simulating catchment runoff. Journal of Applied Water Engineering and Research, 11(4), 481–495. https://doi.org/10.1080/23249676.2022.2156401
- Shahid, M., Cong, Z., & Zhang, D. (2018). Understanding the impacts of climate change and human activities on streamflow: a case study of the Soan River basin, Pakistan. Theoretical and Applied Climatology, 134(1-2), 205–219. https://doi.org/10.1007/s00704-017-2269-4
- Shen, M., Chen, J., Zhuan, M., Chen, H., Xu, C., & Xiong, L. (2018). Estimating uncertainty and its temporal variation related to global climate models in quantifying climate change impacts on hydrology. Journal of Hydrology, 556, 10–24. https://doi.org/10.1016/j.jhydrol.2017.11.004
- Sheng, S., Chen, Q., Li, J., & Chen, H. (2023). The improved reservoir module of SWAT model with a dispatch function and its application on assessing the impact of climate change and human activities on runoff change. Water, 15(14), 2620. https://doi.org/10.3390/w15142620
- Shrestha, N. K., Seglenieks, F., Temgoua, A. G. T., & Dehghan, A. (2022). The impacts of climate change on land hydroclimatology of the Laurentian Great Lakes Basin. Frontiers in Water, 4(July), 1–22. https://doi.org/10.3389/frwa.2022.801134
- Sime, C. H., Demissie, T. A., & Tufa, F. G. (2020). Surface runoff modeling in Ketar watershed, Ethiopia. Journal of Sedimentary Environments, 5(1), 151–162. https://doi.org/10.1007/s43217-020-00009-4
- Singh, D., Vardhan, M., Sahu, R., Chatterjee, D., Chauhan, P., & Liu, S. (2023). Machine-learning- and deep-learning-based streamflow prediction in a hilly catchment for future scenarios using CMIP6 GCM data. Hydrology and Earth System Sciences, 27(5), 1047–1075. https://doi.org/10.5194/hess-27-1047-2023
- Singh, K., Mohit, R., Mohd, K., Sofi, S., Chandra, J., Sami, K., & Bhat, U. (2022). Modelling of streamflow and water balance in the Kuttiyadi River Basin using SWAT and remote sensing/GIS tools. International Journal of Environmental Research, 16(4), 1–14. https://doi.org/10.1007/s41742-022-00416-7
- Singh, S. K., & Marcy, N. (2017). Comparison of simple and complex hydrological models for predicting catchment discharge under climate change. AIMS Geosciences, 3(3), 467–497. https://doi.org/10.3934/geosci.2017.3.467
- Sirigu, S., & Montaldo, N. (2022). Climate change impacts on the water resources and vegetation dynamics of a forested Sardinian basin through a distributed ecohydrological model. Water, 14(19), 3078. https://doi.org/10.3390/w14193078
- Sitotaw, R., Lulekal, E., & Abate, D. (2020). Ethnomycological study of edible and medicinal mushrooms in Menge District, Asossa zone, Benshangul Gumuz region, Ethiopia. Journal of Ethnobiology and Ethnomedicine, 16(1), 11. https://doi.org/10.1186/s13002-020-00361-9
- Sohoulande Djebou, D. C. (2019). Streamflow drought interpreted using SWAT model simulations of past and future hydrologic scenarios: Application to Neches and Trinity River Basins, Texas. Journal of Hydrologic Engineering, 24(9), 1–12. https://doi.org/10.1061/(ASCE)HE.1943-5584.0001827
- Stephens, C. M., Johnson, F. M., & Marshall, L. A. (2018). Implications of future climate change for event-based hydrologic models. Advances in Water Resources, 119(March), 95–110. https://doi.org/10.1016/j.advwatres.2018.07.004
- Su, B., Huang, J., Zeng, X., Gao, C., & Jiang, T. (2017). Impacts of climate change on streamflow in the upper Yangtze River basin. Climatic Change, 141(3), 533–546. https://doi.org/10.1007/s10584-016-1852-5
- Sulamo, M. A., Kassa, A. K., & Roba, N. T. (2021). Evaluation of the impacts of land use/cover changes on water balance of Bilate watershed, Rift valley basin, Ethiopia. Water Practice and Technology, 16(4), 1108–1127. https://doi.org/10.2166/wpt.2021.063
- Suliman, A. H. A., Jajarmizadeh, M., Harun, S., & Mat Darus, I. Z. (2015). Comparison of semi-distributed, GIS-based hydrological models for the prediction of streamflow in a large catchment. Water Resources Management, 29(9), 3095–3110. https://doi.org/10.1007/s11269-015-0984-0
- Tang, X., Zhang, J., Wang, G., Jin, J., Liu, C., Liu, Y., He, R., & Bao, Z. (2021). Uncertainty analysis of swat modeling in the Lancang River Basin using four different algorithms. Water,)13(3), 341. https://doi.org/10.3390/w13030341
- Tanksali, A., & Soraganvi, V. S. (2021). Assessment of impacts of land use/land cover changes upstream of a dam in a semi-arid watershed using QSWAT. Modeling Earth Systems and Environment, 7(4), 2391–2406. https://doi.org/10.1007/s40808-020-00978-5
- Tegegne, G., Park, D. K., & Kim, Y. (2017). Comparison of hydrological models for the assessment of water resources in a data-scarce region, the Upper Blue Nile River Basin. Journal of Hydrology: Regional Studies, 14(November), 49–66. https://doi.org/10.1016/j.ejrh.2017.10.002
- Teklesadik, A. D., Alemayehu, T., van Griensven, A., Kumar, R., Liersch, S., Eisner, S., Tecklenburg, J., Ewunte, S., & Wang, X. (2017). Inter-model comparison of hydrological impacts of climate change on the Upper Blue Nile Basin using ensemble of hydrological models and global climate models. Climatic Change, 141(3), 517–532. https://doi.org/10.1007/s10584-017-1913-4
- Temesgen, A. (2019). Rainfall - runoff modeling: A comparative analyses: Semi distributed HBV light and SWAT models in Geba catchment, Upper Tekeze Basin, Ethiopia. American Journal of Science Engineering and Technolog, 4(2), 34.
- Teshome, F. T., Bayabil, H. K., Thakural, L. N., & Welidehanna, F. G. (2020). Modeling stream flow using SWAT model in the Bina River Basin, India. Journal of Water Resource and Protection, 12(03), 203–222. https://doi.org/10.4236/jwarp.2020.123013
- Thapa, B. R., Ishidaira, H., Pandey, V. P., & Shakya, N. M. (2017). A multi-model approach for analyzing water balance dynamics in Kathmandu Valley, Nepal. Journal of Hydrology: Regional Studies, 9, 149–162. https://doi.org/10.1016/j.ejrh.2016.12.080
- Thirel, G., Perrin, C., Drogue, G., Gerlinger, K., Krumm, J., & . Wagner, J. (2015). Future evolution of river discharge for the French Rhine Basin in a context of climate change - An updated evaluation based on the AR5 IPCC climate. climatique - Une évaluation actualisée s ’ appuyant sur les simulations climatiques issues du 5ème rappor. https://hal.inrae.fr/hal-02601883
- Tian, Y., Xu, Y.-P., Ma, C., & Wang, G. (2017). Modeling the impact of climate change on low flows in Xiangjiang River Basin with Bayesian averaging method. Journal of Hydrologic Engineering, 22(9), 04017035. https://doi.org/10.1061/(ASCE)HE.1943-5584.0001557
- Tian, Y., Xu, Y., & Zhang, X. (2013). Assessment of climate change impacts on river high flows through comparative use of GR4J, HBV and xinanjiang models. Water Resources Management, 27(8), 2871–2888. (2013), https://doi.org/10.1007/s11269-013-0321-4
- Tibangayuka, N., Mulungu, D. M. M., & Izdori, F. (2022). Evaluating the performance of HBV, HEC-HMS and ANN models in simulating streamflow for a data scarce high-humid tropical catchment in Tanzania. Hydrological Sciences Journal, 67(14), 2191–2204. https://doi.org/10.1080/02626667.2022.2137417
- Topalović, Ž., Todorović, A., & Plavšić, J. (2020). Evaluating the transferability of monthly water balance models under changing climate conditions. Hydrological Sciences Journal, 65(6), 928–950. https://doi.org/10.1080/02626667.2020.1725238
- Touseef, M., Chen, L., Masud, T., Khan, A., Yang, K., Shahzad, A., Ijaz, M. W., & Wang, Y. (2020). Assessment of the future climate change projections on Streamflow Hydrology and water availability over upper Xijiang River Basin, China. Applied Sciences, 10(11), 3671. https://doi.org/10.3390/app10113671
- Tran, Q. Q., De Niel, J., & Willems, P. (2018). Spatially distributed conceptual hydrological model building: A generic top-down approach starting from lumped models. Water Resources Research, 54(10), 8064–8085. https://doi.org/10.1029/2018WR023566
- Troin, M., Arsenault, R., Wood, A. W., Brissette, F., & Martel, J. (2021). Generating ensemble streamflow forecasts: A review of methods and approaches over the past 40 years water resources research. Water Resources Research, 57(7), 1–48. https://doi.org/10.1029/2020WR028392
- Troy, B., Sarron, C., Fritsch, J. M., & Rollin, D. (2007). Assessment of the impacts of land use changes on the hydrological regime of a small rural catchment in South Africa. Physics and Chemistry of the Earth, 32(15–18), 984–994. https://doi.org/10.1016/j.pce.2007.07.049
- Usman, M., Ndehedehe, C. E., Manzanas, R., Ahmad, B., & Adeyeri, O. E. (2021). Impacts of climate change on the hydrometeorological characteristics of the Soan River Basin, Pakistan. Atmosphere, 12(6), 792. https://doi.org/10.3390/atmos12060792
- Uysal, E., Alavi, S., & Bezençon, V. (2023). Anthropomorphism in artificial Intelligence: A review of empirical work across domains and insights for future research. In Artificial intelligence in marketing (pp. 273–308). https://doi.org/10.1108/S1548-643520230000020015
- Van Loon, A. F. (2015). Hydrological drought explained. WIREs Water, 2(4), 359–392. https://doi.org/10.1002/wat2.1085
- Veettil, A. V., Green, T. R., Kipka, H., Arabi, M., Lighthart, N., Mankin, K., & Clary, J. (2021). Fully distributed versus semi-distributed process simulation of a highly managed watershed with mixed land use and irrigation return flow. Environmental Modelling & Software, 140(March), 105000. https://doi.org/10.1016/j.envsoft.2021.105000
- Vilaysane, B., Takara, K., Luo, P., Akkharath, I., & Duan, W. (2015). Hydrological stream flow modelling for calibration and uncertainty analysis using SWAT model in the Xedone River Basin, Lao PDR. Procedia Environmental Sciences, 28, 380–390. https://doi.org/10.1016/j.proenv.2015.07.047
- Wakigari, S. A. (2017). Evaluation of conceptual hydrological models in data scarce region of the upper Blue Nile Basin: Case of the Upper Guder Catchment. Hydrology, 4(4), 59. https://doi.org/10.3390/hydrology4040059
- Wang, G., Zhang, J., He, R., Liu, C., Ma, T., Bao, Z., & Liu, Y. (2017). Runoff sensitivity to climate change for hydro-climatically different catchments in China. Stochastic Environmental Research and Risk Assessment, 31(4), 1011–1021. https://doi.org/10.1007/s00477-016-1218-6
- Wang, J., Shi, P., Jiang, P., Hu, J., Qu, S., Chen, X., Chen, Y., Dai, Y., & Xiao, Z. (2017). Application of BP neural network algorithm in traditional hydrological model for flood forecasting. Water, 9(1), 48. https://doi.org/10.3390/w9010048
- Wang, S., Zhang, Z., Sun, G., Strauss, P., Guo, J., Tang, Y., Yao, A., Optimization, E. S., Conservation, W., Combating, D., Conservation, W., Forest, E., & Threat, E. (2012). Multi-site calibration, validation, and sensitivity analysis of the MIKE SHE Model for a large watershed in northern China. Hydrology and Earth System Sciences, 16(12), 4621–4632. https://doi.org/10.5194/hess-16-4621-2012
- Wang, X., & Jeong, J. (2019). APEX-CUTE 4 user manual. Texas A/M Agricultural Research.
- White, M. J., Gambone, M., Haney, E., Arnold, J., & Gao, J. (2017). Development of a station based climate database for SWAT and APEX assessments in the US. Water, 9(6), 437. https://doi.org/10.3390/w9060437
- Worqlul, A., Dile, Y. T., Ayana, E., Jeong, J., Adem, A., & Gerik, T. (2018). Impact of climate change on streamflow hydrology in headwater catchments of the Upper Blue Nile. Water, 10(2), 120. https://doi.org/10.3390/w10020120
- Worqlul, A. W., Dile, Y. T., Schmitter, P., Jeong, J., Meki, M. N., Gerik, T. J., Srinivasan, R., Lefore, N., & Clarke, N. (2019). Water resource assessment, gaps, and constraints of vegetable production in Robit and Dangishta watersheds, Upper Blue Nile Basin, Ethiopia. Agricultural Water Management, 226(September), 105767. https://doi.org/10.1016/j.agwat.2019.105767
- Wu, Y., Xu, Y., Yin, G., Zhang, X., Li, C., Wu, L., Hao, F., Wang, X., & Hu, Q. (2021). A collaborated framework to improve hydrologic ecosystem services management with sparse data in a semi-arid basin. Hydrology Research, 52(5), 1159–1172. https://doi.org/10.2166/nh.2021.146
- Xie, H., Shen, Z., Chen, L., Lai, X., Qiu, J., Wei, G., Dong, J., Peng, Y., & Chen, X. (2019). Parameter estimation and uncertainty analysis: A comparison between continuous and event-based modeling of streamflow based on the hydrological. Water, 11(1), 171. https://doi.org/10.3390/w11010171
- Xu, S., Chen, Y., Xing, L., & Li, C. (2021). Baipenzhu reservoir inflow flood forecasting based on a distributed hydrological model. Water, 13(3), 272. https://doi.org/10.3390/w13030272
- Yaduvanshi, A., Sharma, R. K., Kar, S. C., & Sinha, A. K. (2018). Rainfall – runoff simulations of extreme monsoon rainfall events in a tropical river basin of India. Natural Hazards, 90(2), 843–861. https://doi.org/10.1007/s11069-017-3075-0
- Yaseen, Z. M., El-Shafie, A., Jaafar, O., Afan, H. A., & Sayl, K. N. (2015). Artificial intelligence based models for stream-flow forecasting: 2000-2015. Journal of Hydrology, 530, 829–844. https://doi.org/10.1016/j.jhydrol.2015.10.038
- Yu, B., & Zhu, Z. (2015). Comparaison des modèles AWBM et SimHyd pour des bassins versants boisés. Hydrological Sciences Journal, 60(7-8), 1200–1212. https://doi.org/10.1080/02626667.2014.961924
- Zakhrouf, M., Bouchelkia, H., Stamboul, M., & Kim, S. (2020). Novel hybrid approaches based on evolutionary strategy for streamflow forecasting in the Chellif River, Algeria. Acta Geophysica, 68(1), 167–180. https://doi.org/10.1007/s11600-019-00380-5
- Zeiger, S. J., & Hubbart, J. A. (2016). A SWAT model validation of nested-scale contemporaneous stream flow, suspended sediment and nutrients from a multiple-land-use watershed of the central USA. The Science of the Total Environment, 572, 232–243. https://doi.org/10.1016/j.scitotenv.2016.07.178
- Zhang, L., Xue, B., Yan, Y., Wang, G., Sun, W., Li, Z., Yu, J., Xie, G., & Shi, H. (2019). Model uncertainty analysis methods for semi-arid watersheds with different characteristics: A comparative. Water, 11(6), 1177. https://doi.org/10.3390/w11061177
- Zhang, X., Zhang, X., Hu, S., Liu, T., & Li, G. (2013). Runoff and sediment modeling in a peri-urban artificial landscape: Case study of Olympic Forest Park in Beijing. Journal of Hydrology, 485, 126–138. https://doi.org/10.1016/j.jhydrol.2012.01.038
- Zhao, H., Li, H., Xuan, Y., Li, C., & Ni, H. (2022). Improvement of the SWAT model for snowmelt runoff simulation in seasonal snowmelt area using remote sensing data. Remote Sensing, 14(22), 5823. https://doi.org/10.3390/rs14225823