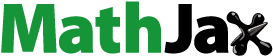
Abstract
Alpha-Beta Brass alloys has an excellent hot working, high strength and malleability properties. This makes us to understand the plastic deformation performance of a material for finite element simulations. This research works gives, numeric analysis flow responses for α-β brass at higher temperatures by utilizing uniaxial tensile experiments. Isothermal tension experiments were been carried on α-β Brass alloy from 773 K temperature to 973 K at an span of 100 K with quasi-static rate of change in velocity (0.1, 0.001, and 0.01 s−1). The outcomes disclosed flow stress was more notably effect on temperature variation compared to strain rate. The material properties, like strength at elongation percentage, strength at ultimate level and yield point were noted at different temperatures. Microstructure features of fractured test samples have been investigated using scanning electron microscope. The fractography study revealed that at higher temperatures fractured specimens exhibits both ductile and brittle behaviour. In addition, constitutive based J-C model have been evolved by using experimental hot flow stress values. The acceptability of this J-C model is verified with statistical parameter. Various parameters like deviation standard (S%), correlation coefficient (R) and average percentage error (Δ%) were utilized to validate the significance of Johnson Cook method for α-β brass. The obtained predicted data are in favourable consensus with experimental data.
1. Introduction
Every structures and machine components have interaction with environmental conditions. Developing the best material that can with stand all climatic condition and maintain the aesthetic is the today’s major challenge. Few metals helps to improve performance in major applications (Sajun Prasad et al., Citation2017). Brass based alloys fills the gap for this applications, and α-β brass is one among these alloys that can be stressed. In this regard α-β brass alloy, with its good strength, corrosion resistance, hot working conditions, excellent electrical and mechanical properties at higher temperatures are seeking attention towards them (Lin et al., Citation2018). This alloys has the major applications in Architecture, marine hardware, tanks and radiator cores, electrical terminals, lamp fittings and plugs, cylinder liners for pumps (Lin et al., Citation2014). However, brass alloys have high deformation resistance, various forming temperatures and complicated microstructures (Alves, Citation2000; Johnson & Cook, Citation1985; Lin & Chen, Citation2011; Zhang et al., Citation2015). Thus, an investigation on flow behavior of α-β brass at higher temperatures is significant.
Understanding flow stress deformation behavior is very important to enhance parameters inorder, to have better performance and material processing conditions throughout machining and forming. A precise constitutive model is necessary for analyzing material deformation behaviour and obtain good numerical simulation results in sheet metal forming. Lin and Chen gave a clear view in their review paper about the classification on constitutive models and discussed briefly about the phenomenological model. This model gives the information about viscoplasticity theory where various variables are stimulating process parameters through thermal softening, strain rate softening and work hardening. Johnson and Cook obtain a relation in JC (Johnson cook) model by correlating temperature and rate of change of strain, strain and flow stress. In this model we can understand effect on individual process parameters clearly (Challa & Rao, Citation2021; Chen et al., Citation2017).
Inorder to get material constant values, stress–strain experimentally obtained data from hot isothermal tensile experiments at various strain rates, strain and temperatures are used. For appropriate prediction most of the constitutive models involve few material constants (Verleysen et al., Citation2011). Most preferably, constitutive equations involves material constants to validate with less experimental data and meanwhile, predict flow behaviour data with good reliability and accuracy for various temperatures and strain rates (Khan et al., Citation2004; Rule & Jones, Citation1998). These mathematical equations that are obtained by using material constants represents the material flow behaviour can also be used as finite element code inputs for simulation. Under particular loading conditions these simulating results gives the material’s response (He et al., Citation2008; Lin & Liu, Citation2010).
The main intention of work is concentrated on hot flow behavior operation at temperatures span of (723–923 K) and strain rate (0.1, 0.01 and 0.001 s−1) of α-β brass alloy. By using the obtained flow stress experimental data J-C model was evolved. Furthermore, microstructure characteristics study has been performed for fractured specimens. The constitutive model results were justified with experimental hot flow stress behavior data. Thus, study aims to examine the behavioral flow stress of α-β brass at wide temperature range and rate of strains. Further, behavioral hot flow stress experimental results were verified with the J-C model.
2. Material and experimental details
α-β Brass is a copper zinc alloy with other small quantities. The elemental composition for alpha-beta Brass is shown in the .
Table 1. The elemental composition for Alpha-Beta Brass alloy.
The specimens are prepared in different orientation like rolling direction (0°), Normal direction (45°) and transverse direction (90°) for tensile test. Isothermal tensile experiment sample of 1 mm thickness were designed as per the ASTM E08/E8M-11 gauge and are represented in . The tensile test samples as represented in were furnished by utilizing wire EDM method inorder, to obtain less distortion, desirable surface finish and good accuracy. The hot tensile experiments are done on Universal testing machine with computer restraint (UTM) with a two-zone split furnace as displayed in , with 100 kN maximum load capacity. The tensile experiments are done at temperature from 773 to 973 K at an intervals of 100 K at quasi static rate of strain of 0.1, 0.01 and 0.001 s−1. For every value, three distinct specimens are tested and reported the average readings. Thus, for all the test samples true strain versus true stress graphs are obtained.
For fractography, the fractured tensile specimens are examined under scanning electron microscope (SEM). These tensile fractured specimens are cut alongside the fractured area are as displayed in . The location of the sample is taken at deformation zone as shown in . Under various magnification fractured samples are observed to know macroscopic mode of fracture and characteristic features during uniaxial deformation.
3. Development of constitutive models
3.1. Johnson–Cook (JC) model
In Johnson-cook model flow stress is expressed as EquationEquation (1)(1)
(1) :
(1)
(1)
Here σ represents flow stress of the material, A represents yield stress at reference temperature and strain rate, B represents strain hardening coefficient, n represents strain hardening exponent, ε represents plastic strain, C represents strain rate hardening coefficient, where
represents strain rate,
represents reference strain rate.
represents homologous temperature and m represents thermal softening exponent. Homologous temperature is expressed as EquationEquation (2)
(2)
(2) :
(2)
(2)
where T is absolute temperature, Tm is melting temperature, Tref is reference temperature, always T ≥ Tref. Normally, room temperature or minimum temperature that is used in the experiment is considered as reference temperature. Three independent variables such as strain rate hardening, thermal softening and isotropic hardening are considered in JC model. But, under various conditions these variables are isolated each other. Thus inorder to obtain total effect all the three terms are multiplied.
3.1.1. Statement 1: Reference temperature and reference strain rate
Under this statement, second and third terms are equal to unity. Therefore, only first term in the equation remains that is:
(3)
(3)
The yield stress gives the value of A at room temperature and 0.1s − 1 strain rate. A graph is plotted in between ln (σ − A) and ln (ε) at the reference temperature and reference strain rate gives n and B in EquationEquation (3)(3)
(3) .
3.1.2. Statement 2: Reference temperature and fixed strain
In this statement third term in Equationequation (1)(1)
(1) equal to unity. Therefore, first and second terms remains that is:
(4)
(4)
Consequently, for each ε value, a graphical line is drawn between and
from this plot slope C value is obtained. Therefore, for each value of ε, C values is attained.
3.1.3. Statement 3: Reference strain rate and fixed strain
This statement gives us, at reference strain rate, = 1 and
= 0. Hence EquationEquation (1)
(1)
(1) is rewritten as EquationEquation (5)
(5)
(5)
(5)
(5)
Under specific flow stress data, for each ε-value, a graphical line is plotted between and
and attain m values. Therefore, for each value of ε, m values is attained.
3.1.4. Constrained optimization
From the above equations, multiple C and m values are attained, but by using the least square method, the ultimate values for material constants C and m are obtained. Least square method involves constrained optimization values by decreasing the average absolute errors (Δ) between experimental flow stress (σexp) and predicted flow stress (σp). The average absolute error is given as Equationequation (6)(6)
(6)
(6)
(6)
Here, N represents total number of data point to be considered. The constitutive models are predicted and quantified by considering standard statistical parameters like average absolute error (D) and correlation coefficient (R). This correlation coefficient provides linear strength relationship between the predicted values and experimental values. Mathematically, it can be expressed as Equationequation (7)(7)
(7)
(7)
(7)
where σp and σexp are average values of σp and σexp respectively. Though the R values are high, it doesn’t represent that the performance of model can be considered as higher. This model have the tendency to change from higher to lower values (Phaniraj & Lahiri, Citation2003). Thus, the values that are obtained for D by compare term by term of relative error is impartial statistics inorder to calculate the predictability of model (Srinivasulu & Jain, Citation2006). Python was used for calculations.
4. Results and discussion
4.1 Behavioural hot-flow stress
The true stress versus true strain flow stress curves are represented in with distinct temperatures at 0.1s−1 rate of strain and other one with different strain rates at 873 K. From the graphs it is observed that as temperature rises, significantly flow stress decline. Similarly, with rise in rate of strain, hot flow stress also rises. A full factorial experiments were performed to evaluate the process and a sample graphs for tensile test at 0.1 s−1 strain versus true stress is presented in . L27 level of parameters are represented in .
Figure 5. True stress versus true strain graphs of α-β brass alloy (a) with distinct temperatures at 0.1 s−1 rate of strain in RD (b) with different strain rates at 873 k. (a) With different temperatures at 0.1 s-1 strain rate. (b) With different strain rates at 873 K.
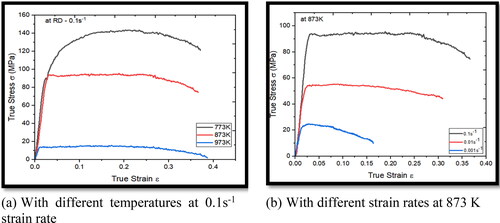
Table 2. Level of parameters.
At distinct temperatures and rate of strains, 27 test runs are performed and material properties are represented in the . The properties of brass material like yield stress (YS), strength at ultimate level (UTS) decreases as temperature rises. Yet, percentage elongation (%E) increases with increase in temperature. It was observed that in 0° orientation all the material properties are shown better results compared to 45° and 90° orientation. This is due to grains elongation in 0° orientation. As ultimate tensile strength and yield stress decreases with increase in temperature in rolling direction indicates flow ability of material. So, alpha-beta Brass alloys are suitable for intricate shapes in forming processes.
Figure 6. True stress versus true strain graphs of α-β brass alloy at different orientation. (a) 673 K, (b) 773 K and (c) 873 K.
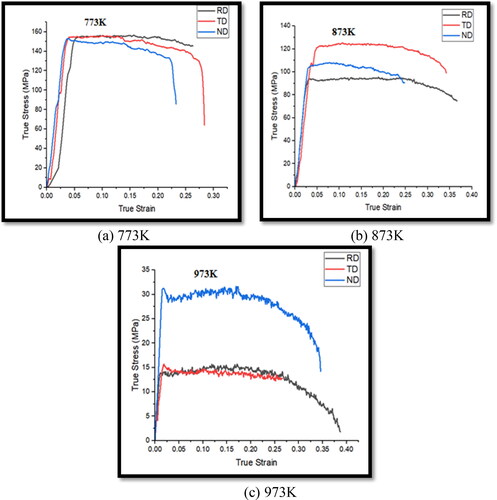
It is observed that serrations are seen at temperatures 773–973 K for all strain rates.
Serrated yielding behavior is explained by the effect of Portevin–Le Chatelier or DSA – dynamic strain ageing. Generally serration in metals are of three types: Type A, B and C (Lin et al., Citation2018; Rodriguez, Citation1984). The serrations noted in α-β Brass alloys are type A and B. The change of hot flow stress behaviour is due to rapid dynamic recrystallization, the former process terminates before the latter one begins (Challa et al., Citation2022; Zhao et al., Citation2014).
Strain rate sensitivity is another way used to define DSA region (Hussaini et al., Citation2014). This parameter is calculated by comparing experimental strains and stress values at identical strain of tensile test at various temperatures and strain rates. Modified Hollomon equation (Prasad et al., Citation2015) is used to calculate parameter m. At various temperatures m values are represented in . From the tabular column and negative value of m indicates DSA effect for α-β Brass from 773 to 973 K at all rate of strains. Therefore, rate of strain sensitivity indicates dynamic recrystallization effect is more generally noticed at elevated temperatures.
Table 3. Values for strain rate sensitivity (m).
4.2. Microstructural analysis
The fractured uniaxial tensile test sample was investigated by utilizing a scanning electron microscope (SEM). These specimens were observed at divergent magnifications to characterize inherent features of deformed surface. Fractography of α-β brass alloy at distinct temperatures are shown in . It was observed that at higher magnification dimples of different shapes and size are seen. With increase in strain, voids growth forms continuous fractured surface. Also, the carbides present near the grain boundaries improves rupture at higher temperatures. The curved shaped impressions in the images are known as dimples and fractured surfaces are referred as dimple rupture. Mixture of voids, dimples, tearing edges and shear region are identified in fractography images. Thus, due to the presence of shear regions and dimplesspecifies mostly ductile failure.
4.3. Constitutive model
4.3.1. J-C model
The graphical comparison of predicted J-C model flow stress with experimental flow stress results are shown in . The represent flow stress at specific strain rates and at different temperatures. The Coefficient of Correlation (R) is statistical term that provides relation between predicted and experimental values. With the help of the correlation coefficient alone, cannot decide the working condition of model. So, prediction of the constitutive model were evaluated by using standard deviation and average absolute error (Challa & RaoSeeram, Citation2023; Challa & Srinivasa Rao, Citation2022; Kotkunde et al., Citation2018). Therefore, for evaluating the prediction capability of constitutive model deviation standard (S), average absolute error (Δ) and coefficient of correlation (R) were used.
Figure 9. Comparison of the JC model projected flow stress for various strain rates and experimentally measured flow stress (a) at 773 K and (b) at 973 K.
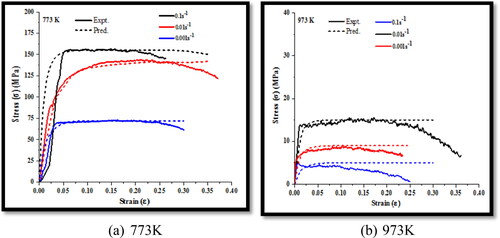
represents the statistical parameters like average absolute error (Δ), correlation coefficient (R) and deviation standard (S) for evaluating the JC model.
Table 4. Statistical parameters obtained for JC model.
5. Conclusion
Present work involves flow stress behaviour, fractography and forecast of flow stress by Johnson Cook constitutive model for α-β brass alloy at various temperatures and rate of strains. The subsequent interpretations are drawn:
The tensile flow stress behavior of α-β brass alloy is mainly subjected on the temperature and rate of strains. The Dynamic strain ageing phenomenon is studied from 773 to 973 K at all strain rates. It was observed that A type and B type serrations at various strain rates and temperatures.
The fractured tensile specimens of α-β Brass alloy show mostly ductile fracture. shear region, dimples and voids were important for fracture phenomena that were observed in this alloy.
Johnson Cook constitutive model is utilized to predict behavioral hot flow stress of α-β Brass. Based upon comparison of various statistical parameters, Johnson Cook model shows superior prediction capability for flow stress.
Future work involves development of finite element analysis to JC model for various applications of metal forming processes.
Author contributions
Bandhavi Challa: Conception and Design of work. A. Anitha Lakshmi: Material Testing. G. Revathi: Analysis of the data. A.V.S Ram Prasad: Drafting the introduction of the paper. S Shilpa: Drafting the experimental part of data. Evgeny Vladimirovich Kotov: Paper Revision with conclusion. Nitin Duklan: Paper Revision and Composite preparation.
Disclosure statement
No potential conflict of interest was reported by the authors.
Data availability statement
The authors confirm that the data supporting the findings of this study are available within the article any other data required will be available from the corresponding author, Bandhavi Challa, upon reasonable request.
Additional information
Notes on contributors
Bandhavi Challa
Bandhavi Challa - Dr. Ch. Bandhavi, Assistant Professor of Mechanical Engineering, has a Ph.D. from KL University. She completed her M.Tech from JNTUK, with specialization CAD/CAM and B.Tech from JNTU Hyderabad in Mechanical Engineering. Her research interest is Metal Forming
A. Anitha Lakshmi
A. Anitha Lakshmi - Dr. A. Anitha Lakshmi, Associate Professor, has a total of 16 Years of Teaching and Industrial Experience. She has completed her Ph.D from Andhra University. She has pursued her M.Tech from Andhra University. Her research area is Metal Forming.
G. Revathi
G. Revathi - Dr. Gadamsetty Revathi is Assistant Professor of Mathematics in the Department of Humanities and Basic Sciences, GRIET, Hyderabad. She completed her M.Sc Mathematics from Acharya Nagarjuna University, Guntur in 2007. She also completed B.Ed from Acharya Nagarjuna University in 2005. She has completed her Ph.D from K L University, Andhra Pradesh. Her research area is Optimization Techniques.
A. V. S. Ram Prasad
A V S Ram Prasad - A V S Ram Prasad, Associate Professor of Mechanical Engineering, in Koneru Lakshmaiah Education Foundation. His research area of interest is Materials and Manufacturing.
S. Shilpa
S. Shilpa - Dr. S. Shilpa, Assistant Professor in Department of BS … H, B V Raju Institute of Technology, Narsapur. She completed her M.Sc. from Osmania University-2006, Ph.D. Applied Mathematics, from GITAM university, Hyderabad,2023. Her teaching Experience is13 years. Area of Interest is Fluid Dynamics.
Evgeny Vladimirovich Kotov
Evgeny Vladimirovich Kotov - Evgeny Vladimirovich Kotov is Peter the Great St.Petersburg Polytechnic University, Doctor of Engineering. His area of interest is numerical simulation and modelling.
Nitin Duklan
Nitin Duklan - Nitin Duklan is from Uttaranchal University. He completed his Computer science MCA,M-Tech(CSE) and his area of interest is Machine learning, pattern recognition.
References
- Alves, M. (2000). Material constitutive law for large strains and strainrates. Journal of Engineering Mechanics, 126(2), 215–218. https://doi.org/10.1061/(ASCE)0733-9399(2000)126:2(215)
- Challa, B., & Rao, S. S. (2021). Study of mechanical properties and microstructural behaviour of alpha-beta brass at elevated temperature. E3S Web of Conferences, 309, 01146. https://doi.org/10.1051/e3sconf/202130901146
- Challa, B., & RaoSeeram, S. (2023). Hot deformation behavior and strain rate sensitivity of α+ β brass sheet by uniaxial material constitutive equations. Indian Journal of Engineering and Materials Sciences (IJEMS), 29(6), 826–831.
- Challa, B., & Srinivasa Rao, S. (2022). High temperatures deformation and formability evaluation of α+ β brass sheet by experimental and theoretical approaches. Advances in Materials and Processing Technologies, 8(sup4), 2173–2190. https://doi.org/10.1080/2374068X.2022.2036044
- Challa, B., Srinivasa Rao, S., Manne, P., Buddi, T., & Satyanarayana, K. (2022). Experimental investigation and optimisation of α–β brass at elevated temperatures using Taguchi approach. Advances in Materials and Processing Technologies, 8(sup2), 537–548. https://doi.org/10.1080/2374068X.2021.1945310
- Chen, G., Chen, L., Zhao, G., Zhang, C., & Cui, W. (2017). Microstructure analysis of an Al-Zn-Mg alloy during porthole die extrusion based on modeling of constitutive equation and dynamic recrystallization. Journal of Alloys and Compounds, 710, 80–91. https://doi.org/10.1016/j.jallcom.2017.03.240
- He, X., Yu, Z., & Lai, X. (2008). A method to predict flow stress considering dynamic recrystallization during hot deformation. Computational Materials Science, 44(2), 760–764. https://doi.org/10.1016/j.commatsci.2008.05.021
- Hussaini, S. M., Singh, S. K., & Gupta, A. K. (2014). Formability of austenitic stainless steel 316 sheet in dynamic strain aging regime. Acta Metallurgica Slovaca, 20(1), 71–81. https://doi.org/10.12776/ams.v20i1.187
- Johnson, G. R., & Cook, W. H. (1985). Fracture characteristics of three metals subjected to various strains, strain rates, temperatures and pressures. Engineering Fracture Mechanics, 21(1), 31–48. https://doi.org/10.1016/0013-7944(85)90052-9
- Khan, A. S., Suh, Y. S., & Kazmi, R. (2004). Quasi-static and dynamic loading responses and constitutive modeling of titanium alloys. International Journal of Plasticity, 20(12), 2233–2248. https://doi.org/10.1016/j.ijplas.2003.06.005
- Kotkunde, N., Krishnamurthy, H. N., Singh, S. K., & Jella, G. (2018). Experimental and numerical investigations on hot deformation behavior and processing maps for ASS 304 and ASS 316. High Temperature Materials and Processes, 37(9-10), 873–888. https://doi.org/10.1515/htmp-2017-0047
- Lin, Y. C., & Chen, X. M. (2011). A critical review of experimental results and constitutive descriptions for metals and alloys in hot working. Materials & Design, 32(4), 1733–1759. https://doi.org/10.1016/j.matdes.2010.11.048
- Lin, Y. C., & Liu, G. (2010). A new mathematical model for predicting flow stress of typical high-strength alloy steel at elevated high temperature. Computational Materials Science, 48(1), 54–58. https://doi.org/10.1016/j.commatsci.2009.06.026
- Lin, Y. C., Wen, D. X., Deng, J., Liu, G., & Chen, J. (2014). Constitutive models for high-temperature flow behaviors of a Ni-based superalloy. Materials & Design, 59, 115–123. https://doi.org/10.1016/j.matdes.2014.02.041
- Lin, Y. C., Yang, H., Xin, Y., & Li, C. Z. (2018). Effects of initial microstructures on serrated flow features and fracture mechanisms of a nickel-based superalloy. Materials Characterization, 144, 9–21. https://doi.org/10.1016/j.matchar.2018.06.029
- Phaniraj, M. P., & Lahiri, A. K. (2003). The applicability of neural network model to predict flow stress for carbon steels. Journal of Materials Processing Technology, 141(2), 219–227. https://doi.org/10.1016/S0924-0136(02)01123-8
- Prasad, Y. V. R. K., Rao, K. P., & Sasidhar, S. (Eds.) (2015). Hot working guide: a compendium of processing maps. ASM international.
- Rodriguez, P. (1984). Serrated plastic flow. Bulletin of Materials Science, 6(4), 653–663. https://doi.org/10.1007/BF02743993
- Rule, W. K., & Jones, S. E. (1998). A revised form for the Johnson–Cook strength model. International Journal of Impact Engineering, 21(8), 609–624. https://doi.org/10.1016/S0734-743X(97)00081-X
- Sajun Prasad, K., Panda, S. K., Kar, S. K., Sen, M., Murty, S. N., & Sharma, S. C. (2017). Microstructures, forming limit and failure analyses of inconel 718 sheets for fabrication of aerospace components. Journal of Materials Engineering and Performance, 26(4), 1513–1530. https://doi.org/10.1007/s11665-017-2547-4
- Srinivasulu, S., & Jain, A. (2006). A comparative analysis of training methods for artificial neural network rainfall–runoff models. Applied Soft Computing, 6(3), 295–306. https://doi.org/10.1016/j.asoc.2005.02.002
- Verleysen, P., Peirs, J., Van Slycken, J., Faes, K., & Duchene, L. (2011). Effect of strain rate on the forming behaviour of sheet metals. Journal of Materials Processing Technology, 211(8), 1457–1464. https://doi.org/10.1016/j.jmatprotec.2011.03.018
- Zhang, P., Hu, C., Ding, C. G., Zhu, Q., & Qin, H. Y. (2015). Plastic deformation behavior and processing maps of a Ni-based superalloy. Materials & Design (1980-2015), 65, 575–584. https://doi.org/10.1016/j.matdes.2014.09.062
- Zhao, B., Zhao, T., Li, G., & Lu, Q. (2014). The kinetics of dynamic recrystallization of a low carbon vanadium-nitride microalloyed steel. Materials Science and Engineering: A, 604, 117–121. https://doi.org/10.1016/j.msea.2014.03.019