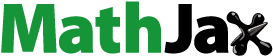
Abstract
Contract farming is an innovative institutional arrangement with potential benefits to the livelihoods of value chain actors. However, there are mixed results in the literature on the extent to which participation in contracts contributes to farm efficiency and optimal use of available technology. This study estimated and compared technical efficiency (TE) and technology gap ratios (TGRs) between contracted and non-contracted farmers of chili pepper and spider plants in rural areas of Kenya. The study used both qualitative and quantitative data from a multistage sample of 300 vegetable farmers. The stochastic frontier and two-limit Tobit models were applied to analyze TE and its determinants, respectively. Further, a metafrontier method was used to estimate TGRs. Results showed that, for both spider plant and chili, contract participants had higher mean TE with respect to the metafrontier (0.66 and 0.24) compared to non-participants (0.12 and 0.15), respectively. Based on the positive effect of contract farming on TE, this study emphasizes the need for targeted interventions that reduce the bottlenecks that hinder effective participation in contracts.
Public interest statement
Smallholder vegetable farmers face many market challenges including delayed payments, no payments, faulty weighing scales and rejection of their produce by different market channels due to unstandardized quality criteria. Contract farming is considered as a potential solution to the numerous marketing challenges. However, effective uptake of contracts by farmers requires transparency and full disclosure of the contractual terms to farmers. Clarification of roles and responsibilities of contracting parties is also essential to ensure win-win situation and minimize potential violation of the contractual terms. Ultimately, well-functioning contracts are envisaged to incentivize rural smallholder farmers to increase their output and transition to profitable commercial farming.
1. Introduction
Smallholder farmers in many rural areas of developing countries in Africa depend on vegetable production as a key enterprise for livelihood sustenance. Schreinemachers et al. (Citation2018) noted that vegetable production forms an integral part of farm diversification and offers an economic opportunity for reducing unemployment and poverty in less developed countries. Fischer et al. (Citation2020) also found that traditional vegetable value chains contribute substantially to food and income requirements of women and poor farmers in less privileged areas of sub-Saharan Africa. In Kenya, indigenous vegetables such as chili pepper (Capsicum species) and spider plants (Cleome gynandra) are widely grown by smallholder farmers due to their nutritional and economic importance. The nutritional relevance of chili derives from its high amounts of vitamin B6, vitamin K, vitamin A and minerals such as magnesium, calcium, potassium, iron, thiamin, copper and folate.
Vegetables make a significant contribution to the economy of Kenya where 57% of spider plant is produced for home consumption while the rest is for income generation. Nationally, the area under chili is about 1,322 hectares (ha), producing a total of 11,133 metric tons (MT) with a monetary value of Kenyan shillings (Kshs) 444,778,506.Footnote1 The area under indigenous vegetables is 45,099 ha with a total volume of 224,751 MT valued at Kshs 5,621, 514, 888 (Republic of Kenya, Citation2019). In the recent years, there has been an increasing awareness of the medicinal and nutritional value of chili and African indigenous vegetables including spider plant among consumers. There has thus, been an increased demand of the same in both rural and urban areas motivating smallholder farmers to venture into production of these vegetables in the process of meeting the prevailing demand and making economic profits from sales of crops. Although farmers expect to gain profits from the sale of these vegetables, their production capacity is limited due to a number of constraints and as a result the supply does not match the demand.
Vegetable production by smallholder farmers has been evolving overtime. For instance, in the early years (1980s) smallholder farmers used to produce these vegetables for subsistence only implying that the vegetables were being cultivated in small quantities. As time went by (2000s) farmers started producing the vegetables for both consumption and marketing purposes (Alulu et al., Citation2020). As from late 2010, smallholder farmers had started commercializing vegetable production and one of the drivers of this trend was increased market demand due to increased awareness of health benefits by consumers in both rural and urban areas. Initially, the point of sale of vegetables was local spot markets but with time, high-value markets such as supermarkets and formal contracts emerged. There have been fundamental constraints faced by farmers over time for instance, inadequate access to production inputs including seeds and credit, poor market linkages and low farm efficiencies. Currently, despite the notable multiple roles of these vegetables, their production is characterized by inefficiency arising from smallholder farmers’ limited access to and optimization of requisite inputs, skills and markets (Mburu et al., Citation2014). Most of the smallholder farmers are semi-commercially oriented and resource-poor, hence lacking a structured organization to enable them meet the dynamic market needs. The vegetable farmers are thus constrained from accessing high value markets due to low production levels triggered by low technical efficiencies across farms among other factors. To respond to the changing consumption patterns, market opportunities occasioned by the rising demand for these vegetables and to address most of the smallholder farmers’ production constraints, contract farming has been viewed as the solution. Overtime, there has been a debate of whether to focus on technological change (introduction of new technologies such as seeds) or technical change (enhancing the productivity of existing technology) in terms of farm-based policies. It appears that most smallholder farmers lack the capacity to optimize on the available resources leading to low farm efficiencies and slow growth of productivity, which is a significant problem among smallholder farmers. This provides a useful basis for comparing the technical efficiency (TE) and technology gap ratios (TGRs) as suggested by Danso-Abbeam and Baiyegunhi (Citation2020).The present study aimed at validating the perceived role of contract farming by comparing TE and TGRs in order to enhance smallholder farmers’ welfare. Literature suggests that contract farming could help to eliminate the resource access challenges that hinder agricultural productivity and efficiency in developing countries (Bellemare & Novak, Citation2017). Some studies also hypothesize that contract farming could improve smallholder farmers’ efficiency and productivity by enhancing coordination among farmers and other actors in the value chain in terms of production, processing and marketing (Nguyen et al., Citation2015). However, there is limited empirical insight on the effect of contract farming on TE and technology use among smallholder vegetable farmers. While some studies (Barret et al., Citation2012); Bellemare & Novak, Citation2017; Dube & Mugwagwa, Citation2017) indicate positive effect of contract farming on welfare, others (Miyata et al., Citation2009) find negative or no significant effect. Moreover, there is inadequate analytical insight on motivations for contract violations among smallholder farmers. This study contributes to the body of knowledge by computing TE and TGRs for contract participants and non-participants, and analyzing the determinants of TE for chili pepper and spider plant, the rarely investigated value chains. The rationale for comparing TG and TGRs is based on two arguments. First TE and TGRs are inseparable despite some of the previous studies separating the two. Secondly, there have been inconclusive findings from previous literature as far as the effect of contract farming on TE and TGRs is concerned as some studies find a positive while others a negative relationship. The present study therefore aims at establishing whether indeed, contract farming has a positive contribution to TE and TGRs on vegetables that have hitherto been ignored in previous empirical research of this nature. The comparison between contracted and non-contracted farmers is envisaged to illuminate development policy towards targeted interventions that enhance the contribution of contracts on farm efficiency and smallholder livelihoods.
2. A review of knowledge gaps
2.1. Technical efficiency, technology gaps and contract farming
A considerable amount of literature has focused on the effect of contract farming on the welfare of farmers using food security as the main indicator, while relatively little has been done on its effects on efficiency. Studies like Bellemare and Novak (Citation2017) and Narayanan (Citation2014) used aggregate on-farm income, which could lead to misappropriation of the benefits of contract farming since it is difficult to attribute whether the income increase is actually from contract farming or other factors. In order to overcome this challenge, the present study fills this gap by using income from the target crop under contract farming. There are major gaps in the study of contract farming. For instance, Henningsen et al. (Citation2015) raises a concern that contract farming improves potential yield levels but leads to a decline in TE. Dube and Mugwagwa (Citation2017) found that contract farming had a significant positive effect on efficiency of smallholder farmers in Zimbabwe. The study revealed that, farmers who do not participate in contracts are about 10% more inefficient than contract farmerse. In addition, Chang et al. (Citation2006) noted that a contract farmer on average is 20% more efficient than a farmer not in contract. Other studies such as Miyata et al. (Citation2009) found no significant difference in TE of farmers in contract farming and non-participants.
Mensah and Brummer (Citation2016) in their study of drivers of TE and technology gaps in Ghana’s mango production sector used the metafrontier estimation technique to derive performance estimates. Their findings revealed that, other than technical inefficiency, technology gaps play an important role in explaining production deficits. This supports the comparison of TE and TGRs in the present studies. Their study suggested that it would be economically more rational to design programmes that enhance farmers’ managerial capabilities to enable such farmers to make better use of existing technologies to increase their output. However, the present study sought to improve on this suggestion by investigating an institutional innovation (contract farming) that entails a wider scope and incorporates sector-wide policies.
Jiang and Sharp (Citation2015) assessed TE and technological gap of New Zealand dairy farms using a stochastic meta-frontier model and found that farm size had a smaller and insignificant positive impact on individual farm efficiency performance. This is against the expectation and hence, the need for validation in the present study. Kolawole (Citation2006) applied the stochastic frontier model to measure efficiency. The study found that the coefficients for farming experience and the age of the farmer were negative. This implied that the aged and most experienced farmers are more technically efficient compared to young farmers. This previous study however, found that the level of education had a positive coefficient meaning that the cost inefficiency of farmers increases with the years of education. This contradicts the ideal assumption that education empowers farmers with knowledge and skills to improve their overall farm efficiency.
Haryanto et al. (Citation2016) estimated TE and technology gap in Indonesian rice farming using Data Envelopment Analysis (DEA), metafrontier and Tobit regression. However, DEA has its own shortcomings for instance, results are sensitive to the selection of inputs and outputs, high efficiency values can be obtained by being truly efficient or having a niche combination of inputs/outputs and the number of efficient firms on the frontier increases with the number of inputs and output variables. The present study aims at overcoming these shortcomings by applying metafrontier approach. Additionally, Berre et al. (Citation2017) implemented a non-parametric frontier efficiency method, DEA, whose merit is that it allows for the assessment of TE with respect to a production frontier. The frontier efficiency is based on best-performing farms in terms of input minimization and output maximization. However, as pointed out earlier, coefficient estimates of DEA may not be robust. Gatti et al. (Citation2015) points out that estimation of the technological gap between stochastic frontier approach and metafrontier can be useful to design public policies and programs that promote innovation, investment and technological change because they measure the potential improvement in performance resulting from changes in the production environment by investing in physical, financial and human capital. It is against this background that the present study embraces metafrontier analysis.
The present study builds on Danso-Abbeam and Baiyegunhi (Citation2020) who used stochastic frontier and metafrontier models in estimating TE and TGRs of cocoa farmers in GhanaMetafrontier analysis accounts for heterogeneity in farm technologies across groups or regions. While Danso-Abbeam and Baiyegunhi (Citation2020) compared regions, the present study compared TE and TGRs of contracted and non-contracted vegetable farmers. Similarly, Alem et al. (Citation2019) estimated TGR using a single output framework.
Tavva et al. (Citation2017) assessed TE of wheat farmers and options for minimizing yield gaps in Afghanistan using stochastic frontier method. There are documented methodological limitations in using stochastic frontier production function independently for instance, maximum likelihood does not allow assessing the reliability of inferences in small samples, the absolute level of TE is quite sensitive to distributional assumptions, but rankings are less sensitive and an assumption regarding to a specific functional form of stochastic frontier. Moreover, Khanal et al. (Citation2018) assessed technical efficiencies and technology gaps of Nepalese farmers in different agro-ecological regions using metafrontier approach. Tsiboe et al. (Citation2019) who estimated vegetable production technical efficiency and technology gaps in Ghana, highlights the need to improve farmers’ access to agricultural markets and strengthening social capital such as farmers’ participations in relevant agricultural organizations and institutions. The present study bridges this gap by investigating contract farming which is an institutional innovation in relation to TE and TGRs. Likewise, Bahta and Hikuepi. (Citation2015) estimated TE and technological gaps of beef farmers in three regions of Botswana and recommended metafrontier analysis as access to technologies may differ within regions due to different farm characteristics.
Most of the previous studies have used deterministic production functions to estimate the effect of contract farming on efficiency, using such approaches has however brought in inherent limitations in statistical inferences (Mabe et al., Citation2019). The present study therefore uses the parametric stochastic frontier estimation of efficiency using input variables; fertilizer quantity, seed quantity, paid labor and land size and metafrontier approach to estimate TGRs. In measurement of labor, unlike previous studies, the present study uses labor directly involved in the production of the target crops to overcome bias.
2.2. Theoretical framework
This study is based on two key theories; the principal-agent theory and the theory of production. Agency theory explains relationships among actors in a given context. It describes the relationship between principals or agents and delegation of control. It gives strategies to best structure relationships where one party determines what is to be done and the other performs decisions on behalf of the principal (Belot & Schröder, Citation2013). This theory forms the basis for showing relationships between contracted farmers and firms. The agency theory points out that it is difficult to account for uncertainties in contracts; hence, this increases transaction costs as a result. Increased transaction costs have a possible effect on uncertainty and information asymmetry result into two main types of agency problems, which are moral hazard and adverse selection.
The theory of production posits that rational individuals will combine inputs in an efficient manner in order to obtain optimal outputs from available technology given input costs (Coelli et al., Citation2005). The theory of production is operationalized through the Agricultural household model, where it is considered that a household produces a variety of outputs to consume and/or market. A household is thus faced with utility maximization problem. Rationally, a household maximizes utility by going for goods at a level where they produce (Qi); using inputs (Xi), consume (Ci), buy (Ni) and sell (Si). The household is thus required to maximize utility subject to several constraints for instance, production technology, income and resources. Following the assumption that markets are perfect (with zero transaction costs), the household will have the following constrained optimization problem (Azam et al., Citation2012).
Subject to
where:
represents the market price,
denotes household endowment in a good, B is the exogenous income,
denotes household attributes and
represents technology attributes.
The income constraint (EquationEquation 2(2)
(2) ) states that total transfers and revenue should be greater or equal to expenditures. The resource constraint (EquationEquation 3
(3)
(3) ) shows that the quantities of goods used as inputs, consumed, and sold should not be more than the total amount of output produced. The production constraint (EquationEquation 4
(4)
(4) ) shows the kind of technology used in production, which is the interaction of inputs and outputs.
Cognizant of the resource constraints described above and the underlying decisions that a rational farmer must make in order to optimize their returns, this study analyzes TE and TGRs for contracted and non-contracted farmers.
3. Methodology
3.1. Sampling procedure and data collection
Data was collected from a survey of chili and spider plant farmers in Bungoma and Busia counties in Western Kenya. Bungoma and Busia counties were selected because of suitable climatic conditions for production of indigenous vegetables that require less capital inputs and thus potential for poverty reduction among rural households and; their strategic geographic location at the Kenya-Uganda border, which provides an opportunity for marketing. Other high potential agricultural zones of Kenya such as the central highlands where contract farming for exported agricultural produce is dominant were omitted due to the need to understand how rural smallholder farmers in traditionally subsistence systems are likely to adopt contract farming, which is a relatively new institutional phenomenon to them.
This study employed Cochran (Citation1963:75) formula to compute the sample size as follows:
where,
n0 = sample size
Z = Abscissa of normal curve that cuts off an area α at the tail (1- α is the desired confidence level for this case, 95%)
e = desired level of precision
p = estimated proportion of an attribute present in the population (0.5 for this case)
q = (1-p)
The eventual sample size was 300 (78% response rate) due to incomplete questionnaires that were missing crucial data for key variables such as input use and income.
A multistage sampling procedure (Bakshi et al., Citation2019) was applied in the selection of the respondents. First, two sub-counties, Bumula and Matayos were purposively selected in Bungoma and Busia counties, respectively due to the level of concentration of chili and spider plant farmers in the two areas compared to the rest. In the second stage, two wards were selected from each sub-county using simple random sampling. The third stage had two villages selected from each ward using simple random sampling method. In the fourth stage, contracted farmers were selected from lists provided by farmers’ field school (FFS) officers from each sub-county using systematic random sampling method; where every second responded was selected. The list for Bumula sub-county had 225 contracted farmers while that for Matayos had 90. A total of 110 and 39 contracted farmers were selected from Bumula and Matayos sub-counties, respectively. Non-contracted farmers were selected from a sampling frame provided using systematic random sampling method where every second and fifth respondent was picked for interview from Bumula and Matayos, respectively based on the differences in number of contracted farmers on lists for both sites. We acknowledge that while our selection of contract participants and non-participants from the FFS lists was meant to provide a neutral sampling frame and thereby overcome outright bias that would have occurred if the lists had been obtained directly from the contracting companies, our sample may not be fully unbiased. As such, we recommend that future studies of this nature should develop ways of eliminating the sampling bias by use of a combination of self-reported lists from contracting firms, the contracted farmers, FFS and other neutral agencies such as public and private extension workers.
It is important to note that, there were several contracting firms in the study area but they all had similar contractual terms of delivering inputs upfront, offering support services and buying the crop at relatively close prices. This implies that there was no heterogeneity in contracting firms to affect the smallholder farmer’s decision to participate in contract farming. In addition, FFS members were farmers producing vegetables, chili and spider plant included and poultry though not all members of FFSs were contracted.
The study also employed a combination of participatory approaches; key informant interviews and a focus group discussion (FGD) for preliminary data collection prior to the main survey. Key informant interview involved consultations with 4 input suppliers, 2 agricultural extension officers, 2 value addition experts and 2 local administrators summing up to 10 participants. This was useful in obtaining insights on evolution of contracts and other production techniques over the years. The stakeholders involved in the FGD included; 2 input suppliers, 2 producers of vegetables, 1 private and 1 government extension officer, 1 broker, 1 farm laborer, 2 vegetables assemblers, 1 distributor of vegetables, 1 value addition expert, 1 local administrator, 1 vegetables traders and 1 vegetable consumer making a total of 15 participants. Focus group discussion enhances a broader perspective of the research issues and eliminates individual bias in data collection (Boateng, Citation2012). The aim of the FGD was to get insights concerning the determinants of participating in contract farming, its effects on farm efficiency, and income.
Semi-structured questionnaires were used for collecting primary data. The questionnaire had five major sections. First, questions on household identification, then the second section had questions on land ownership and vegetable production including input use. The third section had questions concerning vegetable marketing, the fourth section dealt with institutional support with questions on social capital and extension services. Finally the last section had questions on livelihoods and socio-demographic.
3.2. Estimation of technical efficiency of vegetable farmers
This analysis is relevant in the sense that it will provide information needed to improve technical performance of farmers by enabling them to adopt better farming practices. It could be misleading to compare performance of various value chains based on yield per acre or hectare alone. Bringing in contract in the efficiency analysis will help in appreciating value chain-based innovations and their role in enhancing efficiency and improved welfare in general.
Previous studies focused on measurement of TE using deterministic production functions. Due to inherent limitations on the statistical inferences from such approaches, this study adopted the parametric stochastic frontier advanced by Meeusen and van Den Broeck (Citation1977). This is empirically specified as follows:
where Yi is output, Xi represents the input vector, β denotes the vector of production parameters and ε represents the error term that consists of two components, shown below:
The first term Vi is the random error. The second component Ui represents the inefficiency component.
According to Jondrow et al. (Citation1982), the TE estimation is given by the mean of the conditional distribution of inefficiency term, Ui, ε as follows:
where,
F represents the cumulative distribution function and ƒ the standard normal density which are determined at, ελ/σ.
Using the readily available technology that is derived from results of EquationEquation (11)(11)
(11) , the farm-specific TE is defined in terms of the observed output, which is given by Yi to the corresponding frontier output given by Y* as shown below.
From the stochastic frontier, the TE of farmer can be calculated as:
Following Jondrow et al. (Citation1982), the conditional mean of U is given as
where,
;
;
.
The TE takes values within the interval (0, 1), whereby 1 represents a fully efficient farm.
3.3. Metafrontier estimation of technology gap ratios
This approach is applicable in estimating TE among different groups with varying levels of technology. The groups used in this study were contract participants and non-participants for chili and spider plant independently. This method involved estimation of separate stochastic frontiers for the groups. It was assumed that vegetable farmers had different levels of technology in operation. A likelihood ratio (LR) test was first conducted to determine whether differences in technology between contract participants and non-participants for each vegetable were statistically significant to form a basis for constructing the metafrontier.
Assuming there are z locations, the stochastic frontiers of contract participants and non-participants are specified as:
represents vegetable output of
location from the
farm for the
farmer
represents a vector for the
variable input used in
location by the
farmer the
farm,
is a vector of coefficients associated with the independent variables for the stochastic frontier for the
farmer involved in
location,
denote an error time that is decomposed to statistical noise
and inefficiency term
.
According to Battese and Corra (Citation1977), output variation from the frontier due to can be defined as:
where
The LR test was conducted to establish the most appropriate functional form between conventional Cobb-Douglas and alternative translog specifications; the Cobb-Douglas form fitted the data better. The Cobb-Douglas production frontier for contract participants and non-participants was specified as shown in EquationEquation (16)(16)
(16) :
where represents vegetable output (kg),
denotes vectors for variable inputs used on farms such as vegetable seeds (kg), land size (acres), labor (man-days) and fertilizer (kg),
is the constant term,
denote coefficients of the inputs used which were estimated,
represents statistical noise and
is the technical inefficiency.
The TE of the farm in the
region with respect to the stochastic frontier is defined as the ratio of the observed output
to
given that there are no inefficiencies in the production (Battese et al., Citation2004). This is given as:
The most appropriate predictor of TE is derived by specifying EquationEquation (22)(22)
(22) as;
The LR test was used to test the existence of technology gaps between contract participants and non-participants among vegetable farmers. The test has been used by Junaedi et al. (Citation2016) to assess existence of technology gaps between different groups. The test involves estimation of specific stochastic frontiers for the two groups separately followed by a pooled sample from the two groups and assumes a null hypothesis that the stochastic frontiers (technologies) for the participants and non-participants are equal. The LR test is given by;
where denotes log likelihood function value for stochastic frontier of the pooled sample and
are the summed functions for the stochastic frontiers estimated separately for the contract participants and non-participants. The null hypothesis is rejected () implying that there are differences in production technologies across farms thus a justification for the estimation of the metafrontier (Battese et al., Citation2004).
Technology differences between contract participants and non-participants were addressed by the metafrontier, which is assumed to be a smooth function that envelopes the specific participants’ and non-participants’ stochastic frontiers (Battese & Rao, Citation2002). The metafrontier of the pooled vegetable farmers is given by:
where;
represents the metafrontier output from
regions
is the vector of variable inputs used in the farms such as vegetable seeds (kg), land size (acres), labor (man days) and fertilizer (kg),
is the constant,
are the parameters to be estimated,
Asterisk (*) represents the metafrontier
is the error term.
In this model, only the output and input variables were fitted. The metafrontier approach accounts for deviation between an observed level of output and the highest output that is realized in the group frontiers given a specific input level as well as accounting for the differences in technology (Battese et al., Citation2004). The parameters of the metafrontier were estimated through solving a linear minimization problem, expressed as:
where denotes the metafrontier and
are the farmers’ frontiers (Battese et al., Citation2004).
In reference to the metafrontier, the observed vegetable output in region of the
farm in the
farmer measured using the stochastic frontier shown earlier is illustrated as:
In Equationequation 17(17)
(17) ,
refers to the TGR and it is a measure that lies between 0 and 1, hence:
Therefore, can be derived through multiplying the TE in relation to the stochastic frontier of the individual group and the TGR such that:
3.4. Assessment of the determinants of technical efficiency among vegetable farmers
biased results, because the model estimated at the first step is misspecified. The solution
to this bias problem is a one-step procedure based on the correctly specified model for the
distribution of ygiven xand z. In the one-step procedure the assumed relationship between
zand technical efficiency is imposed in estimating the technology and the firms’efficiency
levels, not just at the last stage of the exerc
A two-limit Tobit model was applied in assessing determinants of TE among vegetable farmers since TE scores are normally distributed and bounded between 0 and 1 (Mirza et al., Citation2015).
The two-limit Tobit model that was estimated can be expressed as:
where denotes a continuous latent value of the TE score:
denotes the observed value of the metafrontier TE score;
denotes a matrix various socio-economic characteristics of vegetable farmers and other independent variables that may affect TE
s represents vectors to be estimated;
denotes the random term.
4. Results and discussion
4.1. Stochastic frontier estimates
below shows stochastic frontier TE estimates for spider plant farmers.
Table 1. Stochastic frontier TE results for spider plant farmers
From the results in , among spider plant farmers, contract participants had higher TE scores (0.80) compared to non-participants (0.45). These results are consistent with the findings of Mishra et al. (Citation2018), Tavva et al., Citation2017) and Haryanto et al. (Citation2016) who found a positive relationship between contract farming and TE. Mishra et al. (Citation2018) in their study found that contract farming increased TE of high value crop producers from 87% to 94% and low value crops from 89% to 97%. This is attributed to the fact that contract participants have a better access to inputs such as fertilizer and seeds compared to non-participants. Land and labor had positive coefficients among spider plant contract participants implying that increased used of the inputs increased output. These results are in accordance with those of Alem et al. (Citation2019), Khanal et al. (Citation2018), Bellemare and Novak (Citation2017), El-Ghoraba et al. (Citation2013) and Barrett et al. (Citation2012).
In the pooled sample results, fertilizer had a negative coefficient showing an inverse relationship with output. This could be due to application of the wrong fertilizers on the soil. This happens when soil characteristics conflict with the fertilizer applied. Too much fertilizer could also cause scorching effect. While previous studies like Miyata et al. (Citation2009) showed contradictory results that question the benefits of contract farming for instance, the balance of power between the producers and input suppliers, the present study validates the positive effect of contract farming on TE. Our finding is attributed to the fact that most contracts provide technical assistance to their farmers as well as production inputs upfront, thus enhancing efficient combination of inputs leading to higher TE.
shows stochastic frontier TE coefficient estimates for chili farmers.
Table 2. Stochastic frontier TE results for chili farmers
Results in indicate that contract participants among chilli farmers recorded higher TE scores (0.675) than contract non-participants (0.338).These results are synonymous to those of Bellemare and Novak (Citation2017) thus validating the previous findings that contract farming has a positive effect on TE. The lower TE of non-participants is attributed to imbalanced use of inputs. Land and labor had positive coefficients showing a direct relationship with output among chili contract participants. For non-participants, fertilizer and seeds had a positive relationship with output. The mean TE remains low because farmers were not able to optimally apply the inputs due to inaccessibility of the inputs as implied by Mounirou (Citation2020).
4.1.1. Regularity of production function parameters
In the theory of production, fulfilment of concavity test is a very crucial regularity condition. This test requires that the second order derivatives of all the subject parameters should be negative. This is to imply that the slope of the marginal physical product (MPP) should be negative. The MPP of each production factor must be diminishing at the sample average. The present study fulfils the concavity requirement for all the inputs and for both vegetables as shown in .
Table 3. Second—order derivatives for production parameters of chili
Table 4. Second—order derivatives for production parameters for spider plant
The fulfilment of concavity requirement and the significance of the parameters imply that both chili and spider plant farmers are rational in the utilization of their farm inputs.
where, Q is output, Xi denotes the ith production factor and β the corresponding elasticity (Coelli et al., Citation2005).
shows the second order derivatives for production parameters of spider plant farmers. All the production parameters are significant except for land and labor among contract non-participants. This shows that majority of the farmers are rational in input allocation.
shows hypotheses tests on the production structure.
Table 5. Hypothesis tests on the production structure
The critical values for the distribution were obtained from the statistical table of Kodde and Palm (Citation1986). For the two groups (contract participants and non-participants) for both spider plant and chili, the null hypothesis that the stochastic frontiers (technologies) for the participants and non-participants are equal was rejected meaning that there were differences in technologies among the farmers (groups) thus a justification for the use of metafrontier estimation.
4.2. Technical efficiency and technology gap ratios for vegetable contract participants and non-participants
below shows metafrontier results for vegetable farmers.
Table 6. Metafrontier-based TE and TGRs
From the results above, contract participants among chili farmers had higher TE scores (0.236) with respect metafrontier compared to contract non-participants (0.147). This suggests that contract participants are more efficient in utilization of inputs. Chili contract participants also had higher standard deviation (SD), 0.219 compared to non-participants (0.104). Higher SD implies use of varied technologies such as irrigation and improved varieties among contract participants compared to non-participants (Chang et al., Citation2015).
Results showed that, for spider plant farmers, contract participants had a higher mean TE (0.79) compared to their non-participating counterparts (0.45). Chili contract participants also registered a higher mean TE of 0.68 compared to non-participants who had 0.34. This is because farmers in contracts have better access to production inputs and technical advice hence translating to higher TE (Barrett et al., Citation2012). For both spider plant and chili, contract participants had higher TE with respect to the metafrontier (0.655), (0.236) compared to non-participants (0.123), (0.147). Chili contract participants recorded a slightly higher mean TGR (0.349) compared to non-participants (0.329) while the TGRs for spider plant, were 0.821 for participants and 0.270 for non-participants.
below shows the distribution of TGRs for spider plant farmers in Bungoma and Busia counties. For spider plant contracted farmers, the maximum TGR is 1. This implies that their frontiers are tangent to the metafrontier (Battese et al., Citation2004). Given that the group frontier is tangent to the metafrontier, it means that in order to further increase production of spider plant, a better technology should be introduced for those farmers who have fully exhausted the existing technology.
The highest number of contracted spider plant farmers had their TGRs ranging from 0.65 to 0.85 while a majority of their uncontracted counterparts had their TGRs ranging from 0 to 0.25.
shows the TGRs for chili farmers in Bungoma and Busia counties. For both contract participants and non-participants chili farmers, majority of the farmers had their TGRs ranging from 0 to 0.25. The least number of both contracted and non-contracted chili farmers had their TGRs between 0.45 and 0.65. However, for both contracted and non-contracted farmers, their maximum TGR was 1 implying tangency of their farm frontiers to the metafrontier.
shows comparative distribution of TE with respect to stochastic frontier among spider plant farmers.
There were no contracted spider plant farmers who had TE scores ranging from 0 to 0.25, 0.25 to 0.45 and 0.45 to 0.65. There were also no contract non-participating spider plant farmers who had TE scores ranging from 0.85 to 1. The majority of contract participants among spider plant farmers had their TE scores ranging from 0.65 to 0.85.
shows distribution of TE with respect to stochastic frontier for chili farmers. There were no chili contracted farmers whose TE scores ranged from 0 to 0.25 and 0.85 to 1 as well. However, the majority of chili contract participating farmers had their TE scores ranging from 0.65 to 0.85.
These results therefore lead to the rejection of the null hypothesis that there are no significant differences in TE between contracted and non-contracted vegetable farmers.
4.3. Determinants of technical efficiency among vegetable farmers
shows Two-Limit Tobit results for determinants of TE for spider plant farmers.
Table 7. Two-Limit Tobit results for determinants of technical efficiency for spider plant farmers
Understanding the factors that influence efficiency and technology use helps in identification of sources of inefficiencies for appropriate policy interventions. From , results show that, household size was found to have a positive and significant effect on TE of farmers in Busia. This is explained by the fact that larger households are in a position to supply more labour on the farm that is useful in vegetable production leading to higher TE compared to the smaller households. The results are in line with the observations of Abate et al. (Citation2019). As expected, the household size was hypothesized to positively affect TE due to the capacity of the household to counter the constraints of labor, thus improving TE.
The distance from home to the nearest local market had a negative effect on TE of farmers in Busia County, implying that the further the farmer is located from the market the lower the TE is. This could be due to the difficulty in accessing inputs, essential services and high transaction costs involved. These results coincide with the findings of Mensah and Brummer (Citation2016).
Fertilizer had a positive effect on TE for spider plant farmers in Bungoma County. Increments in units of fertilizer applied increases TE up to a certain level due to the law of diminishing marginal returns. These findings validates the results from Mamary et al. (Citation2018) who found a positive coefficient for organic fertilizer. Seeds had a negative effect on TE of farmers in both Bungoma and Busia counties. These findings coincide with Trujillo and Iglesias (Citation2013). The quantity of seeds was hypothesized to be negative due to the law of diminishing marginal returns.
Participation in contract farming was found to have a positive and significant effect on TE for Bungoma and Busia counties and pooled sample. This is attributed to the fact that contracting firms in most cases issue farmers with inputs and training upfront hence there is efficient combination of inputs and good agricultural practices leading to higher TE. These results contradict the findings by Miyata et al. (Citation2009) who found insignificant differences in efficiencies between contracted and non-contracted farmers. On-farm income had a positive effect on TE among farmers in Busia county. As on-farm income increases, farmers obtain capital to re-invest in production activities through purchase of farm inputs as noteded by Gramzow et al. (Citation2018).
Similarly, shows results for two-Limit Tobit results for determinants of TE for chili farmers
Table 8. Two-Limit Tobit results for determinants of technical efficiency for chili farmers
Just like the case of the spider plant farmers, results in show that, household size had a positive effect on TE for pooled chilli farmers. This is similarly explained by the fact that the larger the size of the household the more labor is provided for production activities thus improving TE. These results support the findings by Tabe-Ojong and Molua (Citation2017) who found a positive relationship between TE and household size.
Consistent with the findings of Akamin et al. (Citation2017), our results show that, years of farming experience had a positive and significant effect on TE for chilli farmers in Bungoma county and for pooled chili farmers. Farmers with more years of experience have gained knowledge on appropriate combination of inputs on the farm. Years of formal education was found to have a positive and significant effect on TE for chilli farmers in Busia and Bungoma counties. The level of education plays a major role in in the improvement of the quality of decisions made by the farmers as far as input use is concerned hence a key tool of improvement as stated by Abdallah (Citation2016). The more years of formal education a farmer has, the more technically efficient the farmer will be. These results support the findings by Danso-Abbeam and Baiyegunhi (Citation2020) who found that education increases understanding of the communication from the extension and hence increases managerial practices and TE.
For chilli farmers in Bungoma county, hired labor had a negative effect on TE. This is against expectations as stated by Huy and Nguyen (Citation2019) that labour increases agricultural production and technical efficiency as it facilitates the utilization of other farm inputs. The negative effect of hired labor could be explained by one or a combination of these factors; low technical skills of the hired laborers, inadequate supervision of hired labor and low motivation by casual laborers due to low wages as argued by Kloss and Petrick (Citation2018).
Results reveal that fertilizer had a positive effect on TE for chilli farmers in Bungoma county. Fertilizer nourishes the soils and increases nutrients supply to the vegetables increasing productivity as well as TE as illustrated by Mburu et al. (Citation2014). Similarly, access to agricultural extension services had a positive effect on TE for chilli farmers in Bungoma county and for the pooled sample as it enhances the capacity of producers and increases the productivity and TE. The findings are similar to those of (Danso-Abbeam & Baiyegunhi, Citation2020). Access to agricultural extension services enables farmers to build their capacity to use new innovations and appropriately respond to climatic and natural challenges like pests and diseases.
Finally, results show that contract farming had a positive effect on TE of chilli farmers in both counties due to the increased access to production inputs and technical support from most of the contractors. The results validate studies by Bidzakin et al. (Citation2018), Bellemare and Novak (Citation2017), and Azumah et al. (Citation2016) who found a positive relationship between contract farming and TE.
5. Conclusion
This study compared the technical efficiencies and technology gap ratios between contracted and non-contracted vegetable farmers in western Kenya and analyzed the determinants of TE. Results indicated that contract farming has a positive effect on TE and TGRs where contract participants had higher mean TE and TGR scores compared to non-participants for both vegetables. Further, it was noted that, household size, seeds, fertilizer and hired labor positively affected TE while distance from home to the nearest local market had a negative effect on TE. The findings thus, offer insights that address the inconclusiveness of previous research regarding the effect of contract farming on TE and TGRs. The findings from this study are applicably generalized in that geographically, smallholder farmers in the study area face similar constraints and policy challenges as farmers from other parts of SSA and possibly other geographic regions. The implication of the findings thus apply to a wider population with no limits of timeframe except for technological advancement that goes a long way in bridging timing differences.
5.1. Policy implications
Following the perceived benefits of contract farming, the government and private stakeholder should put in place concerted efforts that encourage investments in institutional innovations like contract farming. This could be achieved though leveraging on new technologies such as better seeds and fertilizers, and make them more accessible to smallholder farmers who will benefit from enhanced farm efficiency, market linkages and improved incomes. This can be made possible through distribution of roles and resources in devolved governments to optimize on the existing agricultural potential for improved farmers’ income, societal welfare and economic expansion as well. The government and regulatory bodies should consider the incentives and disincentives of contracting firms when designing programmes and policies of promoting contract farming to ensure that there is a balance in benefits between the contracting and contracted parties. Targeted interventions for instance, producer-driven policies should be encouraged to increase participation in contract farming by creating conducive operation atmosphere for all the stakeholders thus encouraging multi-dimensional approach in the quest to improve TE and TGRs among smallholder farmers in SSA. Agricultural extension services had a positive effect on TE. The county government, non-governmental agricultural organizations and contracting firms should therefore increase the frequency and intensity of extension services given to smallholder producers through use of innovative information communication technologies to enhance their capabilities and boost TE at farm level for better output and livelihoods. This study contributes to the body of literature by providing insights on mechanisms to strengthen contract farming that has been found to have positive effect on TE and TGRs of smallholder farmers. Future research should explore the effect of contract farming on various types of efficiencies alongside the evaluation of governance structures to establish the effect of value chain governance on smallholder farmers’ productivity. Better knowledge on the effect of contract farming on livelihoods is necessary; therefore, further research should assess other indicators of livelihoods for instance, food and nutrition security apart from income.
5.2. Limitations of the study
This study provides useful insights on relevance of contract farming in improving efficiency. However, the selection of respondents from lists provided by FFSs could potentially introduce bias in the sampling. A more diversified sampling approach that incorporates insights from FFS, extension agents, contracting firms, farmers and other stakeholders should be explored in future studies in order to overcome sampling limitation. Moreover, a disaggregated analysis of contracts at firm-level would offer more conclusive insights to inform contract participation in different institutional contexts and farmer profiles. Ideally, more representative sampling should entail disaggregation of contracting firms by their terms of service provision and farmers' resource categories and enterprises. This would adequately address the diverse public interests in contract farming.
Competing interests
The authors declare no competing interests.
Acknowledgements
The authors are grateful to African Economic Research Consortium (AERC) for funding data collection.
Data availability statement
We hereby confirm availability of data analyzed in this study upon request.
Additional information
Funding
Notes on contributors
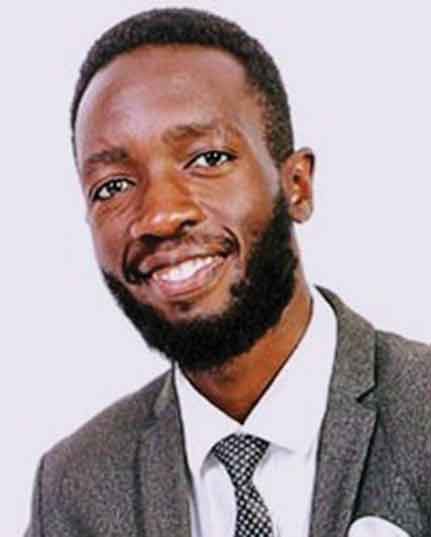
Joseph Alulu
Joseph Alulu is a young researcher in the field of Agricultural Economics with a professional training in Collaborative Masters in Agricultural and Applied Economics from the University of Nairobi, Kenya and University of Pretoria, South Africa. He has relevant professional experience working with various international research organizations in various agricultural value-chains as a trainer, agricultural project coordinator and as a research assistant. Among the key areas of experience are; farmer education, development of work-plans and action plans, agricultural production, incorporation of technology in production activities, value-addition, food fortification, market linkages, data cleaning, analysis and report writing. He is passionate about development and sustainability studies encompassing Agricultural and Rural development, food and nutrition security, agricultural marketing and price analysis aimed at transforming rural lives.
Notes
1. United States Dollar (USD)=Kshs. 101 during the period of the survey.
References
- Abate, T., Dessie, A., & Mekie, T. (2019). Technical efficiency of smallholder farmers in red pepper production in North Gondar zone Amhara regional state, Ethiopia. Journal of Economic Structures, 8(1), 18. https://doi.org/https://doi.org/10.1186/s40008-019-0150-6
- Abdallah, A. (2016). Agricultural credit and technical efficiency in Ghana: Is there a nexus? Agricultural Finance Review, 76(2), 309–25. https://doi.org/https://doi.org/10.1108/AFR-01-2016-0002
- Akamin, A., Bidogeza, J., & Afari-Sefa, V. (2017). Efficiency and productivity analysis of vegetable farming within root and tuber-based systems in the humid tropics of Cameroon. Journal of Integrative Agriculture, 16(8), 1865–1873. https://doi.org/https://doi.org/10.1016/S2095-3119(17)61662-9
- Alem, H., Lien, G., Hardaker, J., & Guttormsen, A. (2019). Regional differences in technical efficiency and technological gap of Norwegian dairy farms: A stochastic meta-frontier model. Applied Economics, 51(4), 409–421. https://doi.org/https://doi.org/10.1080/00036846.2018.1502867
- Alulu, J., Otieno, D., Oluoch-Kosura, W., & Justus, O. (2020). Drivers of transformations in smallholder indigenous vegetable value chains in Western Kenya: Evolution of contract farming. Journal of Applied Business and Economics, 22(6), 1–10. https://doi.org/https://doi.org/10.33423/jabe.v22i6.3082
- Azam, M., Gaiha, R., & Imai, K. (2012). Agricultural supply response and smallholders market participation: The case of Cambodia. School of Social Sciences, University of Manchester.
- Azumah, S., Donkoh, S., & Ethiakpor, D. (2016). Examining the determinants and effects of contract farming on fasrm income in Northern Region of Ghana. Ghana Journal of Science, Technology and Development, 4(12), 1–10.
- Bahta, S., & Hikuepi., K. (2015). Measuring technical efficiency and technological gaps of beef farmers in three regions of Botswana: An application of meta-frontier approach. International Farm Management Congress Proceedings, 1(1), 44–60.
- Bakshi, S., Choudhary, H., & Badal, P. (2019). A multistage sampling case study in india of food packaging on buyers’ behavior. Asian Journal of Agricultural Extension, Economics and Sociology, 1(1), 1–7. https://doi.org/https://doi.org/10.9734/ajaees/2019/v31i130124
- Barrett, C., Bachke, M., Bellemare, M., Michelson, H., Narayanan, S., & Walker, T. (2012). Smallholder participation in contract farming: Comparative evidence from five countries. World Development, 40(4), 715–730. https://doi.org/https://doi.org/10.1016/j.worlddev.2011.09.006
- Battese, G., & Corra, S. (1977). Estimation of a production frontier model: With application to the pastoral zone of Eastern Australia. Australian Journal of Agricultural Economics, 21(3), 169–179. https://doi.org/https://doi.org/10.1111/j.1467-8489.1977.tb00204.x
- Battese, G., Rao, D., & O’donnell, C. (2004). A metafrontier production function for estimation of technical efficiencies and technology gaps for firms operating under different technologies. Journal of Productivity Analysis, 21(1), 91–103. https://doi.org/https://doi.org/10.1023/B:PROD.0000012454.06094.29
- Battese, G., & Rao, P. (2002). Technology gap, efficiency, and a stochastic metafrontier function. International Journal of Business and Economics, 1(2), 87.
- Bellemare, M., & Novak, L. (2017). Contract farming and food security. American Journal of Agricultural Economics, 99(2), 357–378. https://doi.org/https://doi.org/10.1093/ajae/aaw053
- Belot, M., & Schröder, M. (2013). Sloppy work, lies and theft: A novel experimental design to study counterproductive behavior. Journal of Economic Behavior and Organization, 1(93), 233–238. https://doi.org/https://doi.org/10.1016/j.jebo.2013.03.019
- Berre, D., Corbeels, M., Rusinamhodzi, L., Mutenje, M., Thierfelder, C., & Lopez-Ridaura, S. (2017). Thinking beyond agronomic yield gap: Smallholder farm efficiency under contrasted livelihood strategies in Malawi. Field Crops Research, 214(2017), 113–122. https://doi.org/https://doi.org/10.1016/j.fcr.2017.08.026
- Bidzakin, J., Fialor, S., & Yahaya, I. (2018). Production efficiency of smallholder rice farms under contract farming scheme in Ghana. Asian Journal of Agricultural Extension, Economics and Sociology, 25(1), 1–12. https://doi.org/https://doi.org/10.9734/AJAEES/2018/41057
- Boateng, W. (2012). Evaluating the efficacy of focus group discussion (FGD) in qualitative social research. International Journal of Business and Social Science, 3(7), 1–6.
- Chang, C., Chen, C., Chin, M., & Tseng, W. (2006). Is contract farming more profitable and efficient than non-contract farming-a survey study of rice farms in Taiwan. ( No. 379-2016-21901).
- Chang, C., Chen, C., Tseng, W., & Hu, W. (2015). Estimating the profit efficiency of contract and non-contract rice farms in Taiwan. A Meta-Frontier and a Cross-Frontier Approach. Applications (No. 330-2016-13462).
- Cochran, W. (1963). Sampling Techniques (2nd ed.). John Wiley and Sons, Inc.
- Coelli, J., Rao, D., O’Donnell, C., & Battese, G. (2005). An introduction to efficiency and productivity analysis. Springer Science & Business Media.
- Danso-Abbeam, G., & Baiyegunhi, L. (2020). Technical efficiency and technology gap in Ghana’s cocoa industry: Accounting for farm heterogeneity. Applied Economics, 52(1), 100–112. https://doi.org/https://doi.org/10.1080/00036846.2019.1644439
- Dube, L., & Mugwagwa, K. (2017). Technical efficiency of smallholder tobacco farmers under contract farming in Makoni district of Manicaland province, Zimbabwe: A Stochastic Frontier Analysis. Journal Agricultural and Veterinary Sciences, 4(2), 68–78. DOI: https://doi.org/10.21276/sjavs.2017.4.2.5
- El-Ghoraba, A., Javedb, Q., Anjumb, F., Hamedc, S., & Shaabana, H. (2013). Pakistani Bell Pepper (Capsicum annum L.): Chemical compositions and its antioxidant activity. International Journal of Food Properties, 16(1), 18–32. https://doi.org/https://doi.org/10.1080/10942912.2010.513616
- Fischer, G., Patt, N., Ochieng’, J., & Mvungi, H. (2020). Participation in and gains from traditional vegetable value chains: A gendered analysis of perceptions of labour, income and expenditure in producers’ and traders’ households. The European Journal of Development Research, 32(4), 1080–1104. https://doi.org/https://doi.org/10.1057/s41287-020-00257-0
- Gatti, N., Lema, D., & Brescia, V. (2015). A meta-frontier approach to measuring technical efficiency and technology gaps in beef cattle production in Argentina. ( No. 1008-2016-80271).
- Gramzow, A., Seguya, H., Afari-Sefa, V., Bekunda, M., & Lukumay, P. (2018). Taking agricultural technologies to scale: Experiences from a vegetable technology dissemination initiative in Tanzania. International Journal of Agricultural Sustainability, 1(1), 20–40. https://doi.org/https://doi.org/10.1080/14735903.2018.1473103
- Haryanto, T., Talib, B., & Salleh, N. (2016). Technical efficiency and technology gap in Indonesian rice farming. Agris On-line Papers in Economics and Informatics, 8(665–2016–45102), 29–38. https://doi.org/https://doi.org/10.7160/aol.2016.080303
- Henningsen, A., Mpeta, D., Adem, A., Kuzilwa, J., & Czekaj, T. (2015). The effects of contract farming on efficiency and productivity of small-scare sunflower farmers in Tanzania. ( No. 1008-2016-80377).
- Huy, H., & Nguyen, T. (2019). Cropland rental market and farm technical efficiency in rural Vietnam. Land Use Policy, 1(81), 408–423. https://doi.org/https://doi.org/10.1016/j.landusepol.2018.11.007
- Jiang, N., & Sharp, B. (2015). Technical efficiency and technological gap of New Zealand dairy farms: A stochastic meta-frontier model. Journal of Productivity Analysis, 44(1), 39–49. https://doi.org/https://doi.org/10.1007/s11123-015-0429-z
- Jondrow, J., Lovell, C., Materov, I., & Schmidt, P. (1982). On the estimation of technical inefficiency in the stochastic frontier production function model. Journal of Econometrics, 19(2–3), 233–238. https://doi.org/https://doi.org/10.1016/0304-4076(82)90004-5
- Junaedi, M., Daryanto, H., Sinaga, B., & Hartoyo, S. (2016). Technical efficiency and the technology gap in wetland rice farming in Indonesia: A metafrontier analysis. International Journal of Food and Agricultural Economics (IJFAEC), 4(1128), 39–50.
- Khanal, U., Wilson, C., Shankar, S., Hoang, V., & Lee, B. (2018). Farm performance analysis: Technical efficiencies and technology gaps of Nepalese farmers in different agro-ecological regions. Land Use Policy, 76(1), 645–653. https://doi.org/https://doi.org/10.1016/j.landusepol.2018.02.045
- Kloss, M., & Petrick, M. (2018). The productivity of family and hired labour in European Union arable farming (No. 174). Leibniz Institute of Agricultural Development in Transition Economies Halle (Saale) Econstor.
- Kodde, D., & Palm, F. (1986). Wald criteria for jointly testing equality and inequality restrictions. Journal of the Econometric Society, 1(1), 1243–1248. https://doi.org/https://doi.org/10.2307/1912331
- Kolawole, O. (2006). Determinants of profit efficiency among small scale rice farmers in Nigeria: A profit function approach. ( No. 1004-2016-78920).
- Mabe, F., Danso-Abbeam, G., & Sienso, G. (2019). Retracted article: Does contract farming improve rice yield in Ghana? Cogent Food and Agriculture, 5(1), 1643518. https://doi.org/https://doi.org/10.1080/23311932.2019.1643518
- Mamary, K., Lagat, J., Langat, J., & Teme, B. (2018). Determinants of technical efficiency of small scale vegetables production under different irrigation systems in Koulikoro and Mopti Regions, Mali. American Journal of Agriculture and Forestry, 6(4), 71–77. https://doi.org/https://doi.org/10.11648/j.ajaf.20180604.12
- Mburu, S., Ackello-Ogutu, C., & Mulwa, R. (2014). Analysis of economic efficiency and farm size: A case study of wheat farmers in Nakuru District, Kenya. Economics Research International, 1(4), 40–50.
- Meeusen, W., & van Den Broeck, J. (1977). Efficiency estimation from Cobb-Douglas production functions with composed error. International Economic Review, 18(2), 435–444. https://doi.org/https://doi.org/10.2307/2525757
- Mensah, A., & Brummer, B. (2016). Drivers of technical efficiency and technology gaps in Ghana’s mango production sector: A stochastic metafrontier approach Amos. African Journal of Agricultural and Resource Economics, 11(311–2016–5651), 101–117.
- Mirza, F., Najam, N., Mehdi, M., & Ahmad, B. (2015). Determinants of technical efficiency of wheat farms in Pakistan. Pakistan Journal of Agricultural Science, 52(2), 565–570.
- Mishra, A., Shaik, S., Khanal, A., & Bairagi, S. (2018). Contract farming and technical efficiency: Evidence from low‐value and high‐value crops in Nepal. Agribusiness, 34(2), 426–440. https://doi.org/https://doi.org/10.1002/agr.21533
- Miyata, S., Minot, N., & Hu, D. (2009). Impact of contract farming on income: Linking small farmers, packers, and supermarkets in China. World Development, 37(11), 1781–1790. https://doi.org/https://doi.org/10.1016/j.worlddev.2008.08.025
- Mounirou, I. (2020). Does participation in contracts affect agricultural income? An empirical evidence from parboiled rice farmers in central Benin. Cogent Food and Agriculture, 6(1), 1800237. https://doi.org/https://doi.org/10.1080/23311932.2020.1800237
- Narayanan, S. (2014). Profits from participation in high-value agriculture: Evidence of heterogeneous benefits in contract farming schemes in Southern India. Food Policy, 44(1), 142–157. https://doi.org/https://doi.org/10.1016/j.foodpol.2013.10.010
- Nguyen, A., Dzator, J., & Nadolny, A. (2015). Does contract farming improve productivity and income of farmers?: A review of theory and evidence. The Journal of Developing Areas, 49(6), 531–538. https://doi.org/https://doi.org/10.1353/jda.2015.0094
- Republic of Kenya. (2019). Validated report, agriculture and food authority horticultural crops directorate. Kenya National Bureau of Statistics.
- Schreinemachers, P., Simmons, E., & Wopereis, M. (2018). Tapping economic and nutritional power of vegetables. Global Food Security, 16(2018), 36–45. https://doi.org/https://doi.org/10.1016/j.gfs.2017.09.005
- Tabe-Ojong, J., & Molua, E. (2017). Technical efficiency of smallholder tomato production in semi-urban farms in Cameroon: A stochastic frontier production approach. Journal of Management and Sustainability, 7(1), 22–27. https://doi.org/https://doi.org/10.5539/jms.v7n4p27
- Tavva, S., Aw-Hassan, A., Rizvi, J., & Saharawat, Y. (2017). Technical efficiency of wheat farmers and options for minimizing yield gaps in Afghanistan. Outlook on Agriculture, 46(1), 13–19. https://doi.org/https://doi.org/10.1177/0030727016689632
- Trujillo, J., & Iglesias, W. (2013). Measurement of the technical efficiency of small pineapple farmers in Santander, Colombia: A stochastic frontier approach. Revista De Economia E Sociologia Rural, 51(suppl 1), 049–062. https://doi.org/https://doi.org/10.1590/S0103-20032013000600003
- Tsiboe, F., Aravor, J., & Osei, E. (2019). Vegetable production technical efficiency and technology gaps in Ghana. African Journal of Agricultural and Resource Economics, 14(311–2020–259), 255–278.