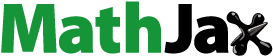
Abstract
Climate change has been a major issue in policy discourse over the past four decades due to its impact on the agriculture sector. This study investigates the effects of climate change variables on food production in Ghana from 1970 to 2019. The results reveal that temperature is inimical to overall food production, maize and roots and tubers production while precipitation is good for cereal and maize production. Furthermore, Carbon dioxide (CO2) emissions impact maize production negatively. The frequency-domain causality test reveals that CO2 Granger causes food production in the long-run. The results suggest that high temperature is harmful to over all food production and some selected crops in Ghana. From a policy perspective, efforts to develop temperature-resilient crops, especially maize, roots, and tubers are necessary to improve food production in Ghana. In addition, there is the need to improve adaptation strategies to reduce the adverse effects of climate change on the food production.
PUBLIC INTEREST STATEMENT
Climate change has been a major issue in policy discourse over the past four decades. This is as a result of its detrimental effect on food security, humanity and the environment. Climate change poses threat to most economies and hence policies to be implemented aimed at reducing its impact are very crucial. In addition, investigating the effect of climate change on food production is a worthwhile task as it is linked to the sustainable development goals (SDGs) such as the zero hunger and no poverty. To this end, this study investigates the effect of climate change on food production in the Ghanaian context. Indeed, the study has shown that high temperature and carbon dioxide emissions are inimical to food production in Ghana, and therefore there is the need to design and implement policies to curb this negative effect of climate change.
1. Introduction
Climate change continues to dominate policy discourse, especially over the past four decades. The impact of climate change on the environment and livelihoods makes it a great deal of concern for policymakers and governments worldwide (see: Ankra et al. Citation2021). The focus on climate change in these recent times is because of the threat that it poses to food insecurity, the environment and humanity especially in developing countries (Intergovernmental Panel on Climate Change [IPCC] 2014; Borras et al., Citation2022; Firdaus et al., Citation2019; Obisesan & Chitakira, Citation2020; Siamabele, Citation2021). Again, there is growing interest in climate change issues due to the continuous increase in greenhouse gas (GHG) concentration in the atmosphere. It is documented that human activities contribute to this growing global conundrum (Asafu-Adjaye, Citation2014; Chin et al., Citation2018; Friedl & Getzner, Citation2003; Rafindadi & Usman, Citation2019), which has affected global temperature and precipitation patterns.
In the context of Ghana, the average temperature increase per decade stands at 0.21°C. This is expected to rise within the interval of 1.7°C to about 2.04°C by 2030. Also, rainfall declined over the past four decades in all the ecological zones in Ghana, and the sea level is expected to rise from 5.8 cm in 2020 to 16.5 cm by 2050 [Ministry of Environment, Science, Technology and Innovation (MESTI), Citation2013]. Since climate change directly influences temperature and precipitation trends as well as extreme events such as drought and flood, the agriculture sector is unequivocally the most vulnerable (Kurukulasuriya & Rosenthal, Citation2003; Wood et al., Citation2021). The impact is more alarming in Africa (Asafu-Adjaye, Citation2014), particularly in Ghana which depends on rainfed agriculture and primitive farming methods (Pinto et al., Citation2012).
The Ghanaian economy is gradually transitioning from an agricultural-led to a service and industry-focused economy, making the contribution of the agricultural sector disproportionally low (Pauw, Citation2018). For example, the sector contributed 41.3% to gross domestic product (GDP) from 1990 to1999, and this declined to 35.4% and 34.0% in 2006 and 2009, respectively (Awunyo-Vitor & Sackey, Citation2018). This further declined to as low as 15.3% in the second quarter of 2019. The decline in the performance of the agricultural sector could partly be attributed to the undesirable effect of climate change. Nonetheless, the agriculture sector continues to play an important role in employment in the Ghanaian economy (Kwawu et al., Citation2021; Ofori-Appiah et al., Citation2021). For example, the sector provides employment to about 44.7% of the labor force. It also provides raw materials for industrial growth and significantly contributes to export earnings (Arndt et al., Citation2015; Enu, Citation2014; Sagoe, Citation2006). In addition, the sector constitutes significantly to food production in the economy. Sagoe (Citation2006) highlights that roots and tuber (cassava, cocoyam, and yam) account for about 58% of per capita food consumption in Ghana, with cassava alone constituting 34% of per annum food crop consumption. This therefore means that the agricultural sector of Ghana is crucial. Hence factors (such as climate change) that could affect its growth and performance must be given the necessary attention.
Most of the crops produced in Ghana, particularly, staple food crops (such as roots and tubers, cereals, and vegetables) are vulnerable to climate change (see: Sagoe, Citation2006). Because these crops are produced annually and are also primarily rainfed. For instance, Popović et al. (Citation2020) point out that global maize yields were 3.5% smaller than the period with moderate climate change. Pinto et al. (Citation2012) argue that temperature and rainfall variation arising from climate change could lead to the loss of arable lands as a result of ecosystem deterioration, reduce yield, and in some cases, trigger the emergence of pests and diseases which are harmful to crop production as documented by MESTI (Citation2013). Ghana is in the warmer climatic zone making its food production emblematic of this climate vulnerability. Climate change could threaten food security, exacerbate rural unemployment, in terms displacement of workers off the agricultural landscape, and eventually could have a restrictive effect on economic growth.
Moreover, in general, food production and agriculture cannot be underestimated as they are tied to the achievement of the 2030 Sustainable Development Goals (SDGs). Specifically, the first goal which aims to end poverty in all forms, and the second goal that is devoted to reducing hunger, and ensuring access to nutritious, safe, and sufficient food throughout the year (MoFA, Citation2020). As a result, studies on the impact of climate change (temperature, rainfall, and carbon dioxide [CO2] emission) on food production are pertinent in aiding the implementation of sustainable policies to address climate change issues in Ghana’s agriculture sector.
In light of the above, this study makes the following contribution to the existing literature on the subject matter. First, the study investigates the long-run effects of disaggregated climate change variables (temperature, precipitation, and carbon dioxide [CO2] emission) on overall food production as well as some selected crops (root and tuber, maize, cereals, rice, and vegetable), in a single study using current data (1970–2020). Amponsah et al. (Citation2015), Owusu and Asumadu-Sarkodie (Citation2017), and Sarkodie and Owusu (Citation2017) used only CO2 as the climate change variable in their analysis. The authors omitted some climate-related variables which are important determinants of Ghana’s food production. Hence, our study fills this void by utilizing three climate-related variables to provide a broader analysis. In addition, Ibn Musah et al. (Citation2018) considered cocoa and cereal production, while Sagoe (Citation2006) and Williams et al. (Citation2017) explored root and tuber and pineapple production in their studies. This study, therefore, provides a broader analysis of the impact of different climate-related variables on four selected staple crops in Ghana. Secondly, unlike previous studies that focused on one region in Ghana (See, Akudugu et al., Citation2012; Amikuzino & Donkoh, Citation2012), this study focuses on a nationwide investigation regarding the effect of climate change variables on food production in Ghana.
Furthermore, this paper employs the novel Maki (Citation2012) cointegration test to examine the long-run relationship among the variables. This model allows one to capture up to five unknown structural breaks when examining cointegration relations, unlike Gregory and Hansen (Citation1996) and Hatemi-j (Citation2008) tests that allow for only one or two a priori number of structural breaks. This model is pertinent to the present study because climate change may or may not be cointegrated with food production in the long-run after allowing for structural breaks. Finally, this study estimates causality among the variables using the frequency domain causality test proposed by Breitung and Candelon (Citation2006). This test has numerous advantages over the time domain causality models; it can establish causality at low, medium and high-frequency levels. It can also remove seasonality-induced variations in small sample sizes. Also, it can capture linear and nonlinear causalities (Breitung & Candelon, Citation2006). The finding of this study would facilitate decision-making on developing effective and efficient policies to combat climate change and boost agricultural productivity and the specific crops to consider when formulating policies.
This paper provides insight and answers to the following questions: Is there a long-run link between climate change and food production in Ghana in the presence of structural breaks? Which of the climatic variables exert long-run pressure on food production in Ghana? At what frequency level(s) does climate change affect food production in Ghana? Which staple food crops (roots and tubers, rice, maize, cereal, and vegetable) is most vulnerable to climate change in Ghana? More importantly, it is worth emphasizing that this paper is the first attempt to apply the Maki (Citation2012) cointegration and the frequency-domain causality models to analyze the impact of temperature, precipitation, and CO2 emission on food production and food crops in Ghana.
The rest of the paper is organized as follows: Section 2 is devoted to literature review whereas section 3 focuses on the data description, measurements, and econometric model specification. The empirical results and discussions are presented in section 4 and section 5 concludes the paper with policy suggestions.
2. Literature review
The effects of climate change are undeniable, and unfortunately, low-income countries that contribute less to climate change issues tend to bear more consequences (Ibn Musah et al., Citation2018). There are four main channels identified in the literature through which climate change may impact agriculture. These channels include precipitation, temperature, CO2 emission and climate variability (Álvarez-Herránz et al., Citation2017; Kurukulasuriya & Rosenthal, Citation2003).
The first channel considers changes in precipitation patterns (World Bank, Citation2007). Precipitation is the primary source of surface water and directly affects soil moisture, which is vital for crop growth. Moreover, precipitation influences crop yield since it is more volatile than crop evapotranspiration which is the main determinant of crop water requirement. Hence, consistent precipitation may induce more yield. However, it may also adversely affect yield if it results in floods and waterlogging (Falloon & Betts, Citation2010; Reilly et al., Citation2003).
Temperature as another channel, on the other hand, determines the duration of growing seasons, crop growth and moisture requirement. The impact of temperature on crop production varies. For instance, in the moderate and high latitudes, high temperature is expected to reduce icy periods and promote cultivation. However, in the tropical and temperate zones, high temperature is expected to increase water requirement, condensing the crop cycle and ultimately reducing crop yield (Parry et al., Citation2007; Rosenzweig & Hillel, Citation1995). Asafu-Adjaye (Citation2014) highlighted that El Niño Southern OscillationFootnote1 (ENSO) had been the main driving force behind temperature variation and precipitation, especially in Southern Africa. For example, there is evidence of ENSO-related temperature variation, which had devastating effects on crops and livestock in countries like Botswana, Zimbabwe, Swaziland, and Zambia around the early 1980s, 1990s, and the late 1990s (Gebreyes et al., Citation2017).
CO2 fertilization has also been considered one of the channels through which climate change impacts food production (Ringius et al., Citation1996). High CO2 concentration in the atmosphere is expected to positively impact crop production by increasing the rate of photosynthesis and greater water use efficiency (Álvarez-Herránz et al., Citation2017). This effect is high among crop categories (including rice, soybean, cotton, peanuts, oats, barley, and spinach). It may also positively impact some crops that follow the C4 photosynthesis pathway (such as sugar cane, maize, millet, and sorghum). However, the impact may be minimal for C4 crops (Betts et al., Citation2007; Gedney et al., Citation2006; Kurukulasuriya & Rosenthal, Citation2003).
The final strand of literature focuses on climate variability (Adams & Hurd, Citation1999). This is particularly important in less developed countries where food production is predominantly rainfed. For example, atmospheric variability which results in frequent floods can deteriorate the ecosystem and reduce arable lands through soil erosion (Kumawat & Yadav, Citation2021) and leaching of agriculture channels (Das, Citation2017). In addition, unpredicted drought may affect the availability of irrigation water and livestock production by disrupting feed supply and surface water for animals to drink (Álvarez-Herránz et al., Citation2017).
The impact of climate change (temperature, precipitation, and CO2 concertation) on agriculture is eminent and has attracted much attention over the past four decades. This is because agriculture, particularly in developing countries, is connected to almost all the sectors of the economy. As a result, recent studies focus on examining both the economic and non-economic impacts of climate change on food production. For example, Ahsan et al. (Citation2020) applied the autoregressive distributed lag (ARDL) model to investigate climate change’s impact on cereal production in Pakistan. The authors revealed a positive long-run relationship between CO2 concentration and cereal crop production. Applying the ARDL for the case of China, Chandio et al. (Citation2020b) reported a negative relationship between temperature, rainfall, and agriculture output in the long-run. However, CO2 was found to exert a positive influence. Their study further revealed a bidirectional causality between these two variables. The finding confirmed the study by Sarkodie and Owusu (Citation2017) who found a positive link between crop and livestock production and CO2 emissions in Ghana by employing the ARDL model for the period 1960 to 2013.
In a related study, Asafu-Adjaye (Citation2014) explored the impact of climate change on agriculture in Africa using the Computable General Equilibrium (CGE) models. The author established that Africa would experience the largest adverse effect of climate change. In northern Ghana, Akudugu et al. (Citation2012) analyzed data from informant interviews and concluded that there is evidence of floods and droughts in some communities, which hitherto never experienced these natural phenomena. The authors highlighted that these occurrences adversely affect food security and households’ livelihood. This finding is confirmed by Arndt et al. (Citation2015). Popović et al. (Citation2020) found that high CO2 concentration may increase global potato yield by 11.12% at an elevated carbon concentration of 550 PPM. However, the authors indicated that a further increase in carbon concentration may reduce potato yield by 13.72% by 2050. In Nigeria, Ayinde et al. (Citation2010) performed an empirical analysis of climate change and agriculture output using descriptive statistics and Granger causality models. Their study revealed that temperature does not statistically affect agriculture output. On the other hand, rainfall was found to be positive and significantly related to agricultural output. Furthermore, Müller et al. (2010a) employed the Lund-Potsdam-Jena managed Land (LPJmL) model to investigate the impact of CO2 fertilization on crop yield on the global front. The outcome of the study revealed that CO2 fertilization promotes yield. Specifically, out of 10 world regions, the average impact indicates rising crop yield in 2046–2055 compared with 1996–2005. However, the authors cautioned that depending on the assumption of the effectiveness of the CO2 fertilization and climate scenario, all regions might experience an increase or a decrease in crop yield. Amponsah et al. (Citation2015) explored the effect of CO2 emission on cereal yield in Ghana by applying the ARDL model to time series data from 1961 to 2010. The study showed that CO2 improves cereal yield in the short-run, but reduces it effects in the long-run. Stige et al. (Citation2006) utilized satellite-derived data on pasture greenness and crop and livestock production data from 1961 to 2003. They reported a strong association between yearly variation in ENSO and maize, millet, sorghum, and groundnut yields. Furthermore, they proclaimed that the effect is strong for Southern African countries, where yield is expected to fall by 20–50% in extreme ENSO years.
Moreover, Thornton (Citation2012) investigated how food production is affected by global warming in developing countries. The study revealed that rice, maize, and wheat are under unfavorable conditions, which could reduce yields. Using crop simulation models, Mereu et al. (Citation2015) examined the impact of climate change on staple crop production in Nigeria. Projections from the model indicated that cereal yield is expected to decline in the long-run, while in the short-run, cassava and millet yields are anticipated to increase by 2020. Also, Zaied and Zouabi (Citation2016) examined the long-run effect of temperature on olive production and concluded that temperature is harmful to olive production. Exploring the link between CO2 and agriculture production in Ghana, Owusu and Asumadu-Sarkodie (Citation2017) found a long-run equilibrium relation running from rice production, copra production, millet production, corn production, coffee production, sorghum production, and palm kernel production to CO2 emission. Using the FMOLS and the cointegration regression as estimation techniques and time series data spanning 1968 to 2014, Chandio et al. (Citation2020a) reported that CO2 emission enhances rice production in Pakistan. On the contrary, Chandio et al. (Citation2021b) revealed that CO2 emissions impede cereal production in Pakistan, using annual data from 1977 to 2014 and applying the ARDL model as the estimation technique. Warsame et al. (Citation2021) employed the ARDL model to investigate the relationship between climate change and crop production in Somalia. Their study showed that temperature reduces crop production in the long and short-run while precipitation is revealed to be good for crop production in the long-run but humpers it in the short-run. Similarly, Chandio et al. (Citation2021b) examined climate change and food security nexus among six Asian countries (Sri Lanka, Pakistan, Nepal, India, Bhutan, and Bangladesh) from 1991 to 2016. The authors revealed that CO2 and temperature harm crop production. Chandio et al. (Citation2021a) found similar outcome for wheat production in Turkey.
Recently, Gul et al. (Citation2022) employed the ARDL model to evaluate climatic change factors (temperature and rainfall patterns) and non-climatic change factors (fertilizer consumption, areas under major crop yield and formal credit) on major food crop yields in Pakistan from 1985 to 2016. The results from the ARDL model revealed that temperature is not suitable for major food crop yield. On the other hand, rainfall and the non-climatic change factors positively impacted major crop yield. Using time-series data from 1961–2016 and the DOLS model to investigate the role of climate change in rice production in Asia, Chandio et al. (Citation2022b) reported that CO2 and temperature significantly reduce rice production in the long-run while precipitation increases it. A similar outcome was reported by Chandio et al. (Citation2022a) with regard to maize production in Nepal. In contrast, Ntiamoah et al. (Citation2022) established that CO2 emissions promote maize production in Ghana.
Aside from climate change variables, other macroeconomic variables such as population growth, economic growth, and inflation has also been considered to influence food production. For example, Byrnes and Bumb (Citation2017) found evidence that rapid population growth may negatively affect global food production. Gambo and Idris (Citation2018) confirmed this result for the case of Nigeria. On the contrary, Okunola et al. (Citation2018) reported that population growth positively impacts food production and further concluded that the Malthusian theory is not evident in Nigeria. Also, Olatunji et al. (Citation2012) reported that inflation negatively impacts food production in Nigeria.
The empirical studies reviewed show that there is limited study on Ghana that has considered the impact of temperature, precipitation, and CO2 emission on food production as well as some selected staple food crops in a single study. Moreover, the cointegration and causality models employed in many of these studies did not account for multiple structural breaks or causality at different frequencies, which could unearth the dynamic impact of climate change on food production in Ghana. This lacuna in the empirical literature reinforces the importance of the present study.
3. Methodology and data
3.1. Data and variable description
The study utilizes annual time series data spanning 1970 to 2019. Based on the empirical studies expounded above, the variables used are agriculture food production index, real gross domestic product, total CO2 emission, temperature, precipitation, population growth, cereal, maize, rice, roots and tubers, vegetable and wheat production. All the variables are converted to natural logarithm to induce covariance stationary and reduce multicollinearity (Chandio et al., Citation2020b).The use of logarithm also helps in interpreting the coefficients as elasticities. The summary of the variables is reported in .
Table 1. Data description and sources
3.2. Theoretical framework
Climate change may distort the agro system to have a diverse impact on agriculture. On the one hand, climate change may have negative effect on agriculture (food production). For instance, high temperature has the tendency of reducing soil moisture, shortening of growth periods (poor vernalization), and this may reduce food production. Furthermore, climate change brings about extreme weather, such as droughts and floods, which in turn reduces food production. On the other hand, there are some positive effects that are associated with climate change. It leads to an increase in food production (through flowering due to high CO2 concentration), and a reduction in heating cost.
3.3. Model specification
The functional and estimable forms of the model are presented in EquationEquations 1(1)
(1) and Equation2
(2)
(2) , respectively.
where is the dependent variable and it is captured in this study by six variables: food production, cereal, maize, rice, roots and tubers and vegetable production.Footnote2 Here, TEM, PRE, CO2, RGDP, POP and INF represent temperature, precipitation (rainfall), carbon dioxide emission, real gross domestic product per capita, population growth and inflation, respectively. Also,
is the natural log operator,
represents the coefficient of the respective variables and
and
are the constant and error terms, respectively.
It is expected that temperature will adversely impact food production. This is probable because Ghana lies in the temperate climatic zone, which experiences high temperatures throughout the year. As a result, further increases in temperature may adversely affect food production by reducing soil moisture and plant growth. Precipitation (rainfall) is expected to affect agriculture food production positively. This is because rain is good for crop growth, especially in Ghana, where agriculture is primarily rainfed due to low irrigation practices and adaptation. However, heavy downpours may destroy farmlands and crops. Similarly, CO2 concentration is expected to positively affect food production due to CO2 fertilization. Regarding inflation, a negative sign is expected because it may increase the prices of food and farm inputs reducing the demand and production of agriculture output. In some cases, domestic agriculture products are substituted with their foreign counterparts, which consumers find relatively cheaper. Also, population and economic growth are expected to affect agriculture food production positively. As the economy expands, households’ purchasing power (income) increases, implying a high demand for food. This then serves as an incentive for farmers to increase production. Moreover, high population growth may increase labour supply to produce more food, especially in the rural areas of Ghana (Gyamfi et al., Citation2018).
3.4. Estimation technique
3.4.1. Unit root test
Time series data mostly exhibit trend or seasonal dynamics and may contain a unit root. We employed the Kwiatkowski et al. (Citation1992) [KPSS] and Dickey and Fuller (Citation1981) [ADF] unit root tests to ascertain the stationarity properties of the data. In the ADF test, we test the null hypothesis of unit root against the alternative hypothesis of no unit root. For the KSPSS test, the null hypothesis assumes that the series is stationary (no unit root) while the alternative hypothesis assumes no stationarity (presence of unit root).
3.4.2. Maki cointegration test with multiple structural breaks
The present study considers cointegration with multiple structural breaks proposed by Maki (Citation2012). This model can determine up to five unknown number of structural breaks when examining cointegration relations among variables, unlike Gregory and Hansen (Citation1996) and Hatemi-j (Citation2008) tests which allow for only one or two a priori number of structural breaks (Maki, Citation2012). Maki (Citation2012) proposes four main regression models, namely, the model with the level shift (EquationEquation [1(1)
(1) ]), the model with the regime shift (EquationEquation [2
(2)
(2) ]), the model with a trend (EquationEquation [3
(3)
(3) ]), and the model that captures structural breaks at level, trends and the explanatory variables (EquationEquation [4]
(4)
(4) ).
where is the time component,
,
is the vector
of observable independent variables with uniform order of integration,
is a vector of dependent variable, and
(
is the error terms.
and
are parameters to be estimated. The value of
is unity and zero if
respectively. Here,
represents the maximum lag order while
is the period of the break. Critical values are presented in Maki (Citation2012) for the maximum number of breaks,
and the number of regressors,
, from
. The GAUSS software version 21 is used for the estimation.
3.4.3. DOLS and FMOLS tests for long-run relationship
To investigate the long-run relationship between food production and its determinants, EquationEquation (2)(2)
(2) is estimated using the dynamic ordinary least squares (DOLS) and fully modified ordinary least squares pioneered by Stock and Mark (Citation1993) and Phillips and Hansen (Citation1990) respectively. The motivation for using these methods is that, for instance, the DOLS allows for the inclusion of lags of the regressors to eliminate the problem of endogeneity (Khan et al., Citation2020). Also, the FMOLS hinges on a nonparametric approach that addresses the issue of serial correlation and produces efficient results even when the series has a unit root. Furthermore, the FMOLS and the DOLS do not require differencing the variables, resulting in the loss of valuable information regarding the long-run characteristics embedded in the level of the data (Arize et al., Citation2015). Monte Carlo estimation by Cappuccio and Lubian (Citation2001) and Khalaf and Urga (Citation2014) supports the FMOLS and the DOLS models. Nonetheless, the precondition of the model is that the variables should be cointegrated.
3.4.4. Frequency domain causality test
The paper also investigates the causal effects of climate change variables on food production at different frequencies, while population growth, inflation, and economic growth are employed as control variables. Consequently, the frequency-domain causality test by Breitung and Candelon (Citation2006) is used, which fulcrums on initial studies by Geweke (Citation1982) and Hosoya (Citation1991). The dichotomy between the “time-domain” causality and “frequency-domain” causality models, according to Gokmenoglu et al. (Citation2019), is that the “time-domain” shows the dynamics that occurred within a historical series while the “frequency domain” measures the extent of the particular variation within the series. The frequency-domain causality test has numerous advantages over its time-domain counterparts. For example, it can establish causality at low, medium and high frequency levels. Furthermore, the test allows one to decouple long-run (permanent) causality from short-run (temporal) causality (Khan et al., Citation2020). Also, it can remove seasonality-induced variations in small sample sizes. Lastly, the test can capture both linear and nonlinear causalities (Breitung & Candelon, Citation2006). The econometric specification of the test is as follows:
Let EquationEquation (7)(7)
(7) represents a bivariate
causality model.
where is a vector of stationary respond variable,
is a vector of regressors,
is the time component,
is the maximum lag order and
is the error term. The traditional Granger causality measures causality based on the ability of
to explain the variations in
. However, this test provides only a single statistic for the whole sample size, submerging the difference between long-run and short-run effects. Furthermore, the sign and direction of causality can also differ between different frequencies. The traditional Granger test fails to recognize these differences (Ozer & Kamisli, Citation2015). As a result, we transform EquationEquation (7)
(7)
(7) based on Breitung and Candelon (Citation2006) to account for the weaknesses outlined. Assuming that
follows a finite-order
representation given by:
where with
. The nuisance vector follows a white noise with
and
, where
is symmetric and a positive definite. For mathematical simplicity, we exclude deterministic time trend, constant or any dummy variable in the model. Since
is symmetric and a positive definite, there exist a Cholesky decomposition such that
and
are the bottom and the top triangles, respectively. In this case,
, where
and
. The moving average representation of the system is as follows:
where ,
)
and
. Using the frontier transformation system, the special density of
is represented in two parts as follows:
The first component of Equation (10) is called the intrinsic term which is driven by the previous values of and the second component captures the predictive power of
. The idea that
does not Granger cause
at a given frequency,
, is ascertained if the predictive factor of
spectrum at a given
is zero. As a result, Geweke (Citation1982) and Hosoya (Citation1991) suggested a causality which is specified in EquationEquation (11)
(11)
(11) .
At frequency
does not Granger cause
if
, making
. The null hypothesis that
does not Granger cause
is expressed as
. Given the fact that the approach by Geweke (Citation1982) and Hosoya (Citation1991) may require the use of numerical differentiation or bootstrap method which may make the test difficult to implement, Breitung and Candelon (Citation2006) proposed a simpler procedure by imposing a linear restriction on the
model in EquationEquation (9)
(9)
(9) as follows:
and the corresponding null hypothesis is , where
The ordinary F-statistics is approximately distributed as for
where
and
represent the number of observations used to estimate the
and restrictions, respectively. Given this, it becomes possible or easy to implement the test within the cointegration framework. In achieving this, Breitung and Candelon (Citation2006) proposed that
in EquationEquation (12)
(12)
(12) should be represented by
while the right hand side of the equation remains unchanged. It is important to mention that “long-run causality” is considered at frequency zero within the cointegration framework. On the other hand, there is no long-run causality between the series in a stationary framework as documented by Ozer and Kamisli (Citation2015).
4. Empirical results
We present the results of the paper in this section. Specifically, the outcome of the unit roots and cointegration tests, the FMOLS and DOLS results, as well as the frequency domain causality tests are discussed.
4.1. Descriptive statistics
The summary statistics of the variables are presented in .
Table 2. Descriptive statistics
The yearly average food production is 3.75. This value is lower than agriculture mechanized countries like South Africa, Morocco, China, and the United States. Unsurprisingly, the average temperature and precipitation in Ghana are 27.4°C () and
respectively. This indicates that the temperature in Ghana is relatively high while precipitation is low. Also, the average CO2 emission is 1.67. Inflation, economic and population growth have average values of 3.05, 6.94 and 16.62, respectively. With regard to cereal production, the average value is 14.15 while maize, rice, vegetable, and roots and tubers production recorded averages of 6.69, 12.04, 13.07, and 15.99, respectively. Roots and tubers recorded the largest average among the crops. This is plausible because it is the primary carbohydrate source in many Ghanaian homes and contributes significantly to export earnings. For instance, Ghana is currently the largest yam exporter in West Africa.
4.2. Stationarity tests
The ADF and the KPSS unit root tests are reported in .
Table 3. ADF and KPSS unit root test results
Generally, the results of the ADF test are consistent with the KPSS test for the model with a constant term. Specifically, the ADF and the KPSS tests with constant term show that temperature, precipitation, and inflation are stationary at level, []. Therefore, the null hypothesis of unit root (no unit root for the KPSS test) is rejected (not rejected for the KPSS test) at a 1% significance level. The results further indicate that food production, economic growth, CO2 concentration, maize, cereal, rice, and roots and tubers are stationary at first-difference, [
]. However, population growth is found to be
in the ADF test but
in the KPSS test. Moreover, Vegetable production is
in the KPSS test but
in the ADF test. On the other hand, the model with a deterministic time trend shows mixed results for both tests. For instance, the KPSS test reveals that all the variables are
, except economic growth, which is
. On the other hand, the ADF results show that temperature, precipitation, CO2 concentration, inflation and cereal production are stationary at level while economic growth, population growth, maize, rice, vegetable, and roots and tubers production are stationary at first-difference. It can therefore be deduced that the series are integrated in a mixture of
and
.
4.3. Maki cointegration test
After confirming the stationarity properties of the data, the Maki (Citation2012) cointegration test is estimated to establish the long-run relationship between food production and its covariates.Footnote3 For the purpose of this study and following the paper by Khan et al. (Citation2020), we allow for three breaks ( and the results are reported in .
Table 4. Cointegration results
The results show the existence of two cointegration among food production and the climate change variables. Specifically, the level shift and the level shift with trend reveal cointegration at 5% and 10% level of significance, respectively. Therefore, both models reject the null hypothesis of no cointegration at 5% and 10%, respectively. This outcome is consistent with the finding by Chandio et al. (Citation2020b).
4.4. FMOLS and DOLS results
The unit root and the cointegration tests presented above lend support for the appropriateness of the FMOLS and the DOLS models. shows the results regarding the effect of the climate change variables in addition to the control variables on food production.Footnote4
Table 5. DOLS and FMOLS results
From , all the variables have the expected signs. Precisely, a 1% increase in temperature corresponds to a 1.707% and 2.132% decline in food production for the DOLS and the FMOLS, respectively, albeit insignificant for the DOLS model. This finding implies that that temperature is inimical to food production. Although precipitation and CO2 positively influence food production, the results are insignificant for both models. Hence, temperature variation is the most important climate-related element that significantly impact food production in Ghana, which should be highly considered when formulating climate change policies. This finding is in line with Warsame et al. (Citation2021) on Somalia, Chandio et al. (Citation2020b), on China and Gul et al. (Citation2022) on Pakistan.
The negative coefficient of temperature is plausible and could be attributed to three reasons. First, Ghana’s climate is tropical and the country is typically warm year-round. Therefore, increase in temperature would exacerbate the already hot climate, which could lead to a reduction in nutrient efficiency. It also increases crop water consumption, reduces soil moisture, and interrupt the crop cycle by prolonging crop growth, thereby reducing crop yield. Second, most farmers depend on rainfall or irrigation for their farming activities. Therefore, given the rising temperature, irrigation dams get dried and this affects food production negatively. More so, rivers in Ghana are shallow and susceptible to temperature variations, which hinders irrigation practices. Due to these dynamics, crops in Ghana may be exposed to the increasing temperature and water variability, ultimately undermining food production. Third, according to Fuhrer (Citation2003) and Warsame et al. (Citation2021), pests and parasites have the most devastating effect on crops during high temperatures, increasing pre- and post-harvest losses. Surprisingly, the coefficient of precipitation was insignificant. This could be possible because of the inconsistent patterns of rainfall in recent years, which has interrupted planting seasons. Also, the insignificant coefficient of CO2 implies that CO2 fertilization is ineffective on food production in Ghana, which could imply that the warmer climate undermines the impact of elevated CO2 on yield.
Moving on to the control variables, the results show that a 1% increase in economic and population growth significantly increases food production by 0.496% and 1.193% (DOLS model) and 0.336% and 1.074% (FMOLS model), respectively. This could be attributed to the fact that, as the economy expands, economic agents get more income for consumption which increases demand for food, which serve as an incentive to producers to increase their production. Also, the population growth in Ghana is driven by the rural areas where farming is the main source of livelihood (Gyamfi et al., Citation2018). As a result, population growth could increase the supply of farm labour which may induce food production. Therefore, the Malthusian theory is not evident in Ghana. On the other hand, inflation is found to reduce food production. Precisely, a 1% increase in inflation leads to a 0.069% and 0.096% decline in food production for the DOLS and the FMOLS models, respectively. As prices rise, food becomes expensive for consumers, resulting in low demand. In some cases, domestic foods are substituted with imported ones. Also, the high inflation could increase the cost of farm inputs such as pesticides, fertilizer, and weedicide, and this may reduce agriculture output. Studies such as Enu (Citation2014), on Ghana and Olatunji et al. (Citation2012), on Nigeria have reported similar findings. However, our results contradict that of Gambo and Idris (Citation2018) who reported an insignificant relationship between Nigeria’s economic growth and agricultural output. The authors also find support for the Malthusian theory in Nigeria, which plausible because Nigeria is the largest populated country in Africa. Therefore, a further increase in population growth may lead to a decline in food production.
Diverse crops respond differently to temperature, precipitation, and CO2 emission. Therefore, we further estimate EquationEquation (2)(2)
(2) using cereal, maize, rice, roots, and tubers production as dependent variables to examine how individual crops may respond to the climate change variable. The results are reported in .
Table 6. DOLS and FMOLS results for cereal, maize, rice, and root and tuber production
It is observed from that temperature reduces cereal, maize, rice, and roots and tubers production in all the models. This outcome further reinforces the notion that temperature is detrimental to food production in Ghana, although the results are significant for only maize and roots and tubers production. Specifically, a 1% increase in temperature leads to a 3.236% and 3.220% decline in maize and roots and tubers production, respectively (see the FMOLS model specifications). This could imply that warming generally reduces grain yields due to accelerated plant development. Also, precipitation positively influences all the selected crops, albeit significant for only cereal and maize production (see the FMOLS model specifications). A 1% increase in precipitation results in a 0.142% and 0.159% increase in cereal and maize production, respectively. This is plausible because most cereal crops, such as rice consumes about 4000–5000 litres of water per kg of grain produced, making precipitation an integral component of its development. Furthermore, the results show that a 1% surge in CO2 concentration significantly reduces maize production by 0.536% and 0.477% for the DOLS and FMOLS models, respectively. This finding may be attributed to the fact that maize is a crop that does not depend much on CO2 fertilization as documented by Kurukulasuriya and Rosenthal (Citation2003), compared with its
counterparts (such as rice, and root and tuber). Also, Fuhrer (Citation2003) claims that the benefits of CO2 may not be realized in warmer climate regions. Studies such as Amponsah et al. (Citation2015), on Ghana, Arndt et al. (Citation2015), on Ghana, and Thornton (Citation2012), from a developing world perspective have also reported similar results.
The results from indicate that a 1% increase in economic growth results in a 0.404% (DOLS model) and 1.379% and 1.182% (DOLS and FMOLS models respectively) increase in cereal and rice production respectively. This is probable because cereal and rice production constitute the major staple foods in Ghana. Also, rice accounts for the largest share of cereal production in Ghana which may explain this result. Population growth is revealed to increase production of all the selected crops—cereal, maize, rice and roots and tubers. This further affirms that the Malthusian theory is not evident in Ghana. Finally, inflation exerts negative effect on all the selected crops except rice production. The result for the rice production may be attributed to the fact that, as prices increase (including that of rice), rice producers find production more profitable and therefore increase output.
4.5. Frequency domain causality
To establish the direction of causality, we estimate the frequency domain causality test. We first perform a lag selection test to determine the optimal lag order for the model. Based on the Akaike Information Criterion (AIC), Schwarz Information Criterion (SIC) and the Hannan-Quinn Criterion, an optimal lag of 4 is selected. It is also important to mention that for brevity, we test causality for only low and high frequencies and the results are reported in .
Table 7. Frequency domain granger causality test results
As shown in panel A of , at low frequencies (long-run), temperature, CO2 concentration, economic growth and population growth are predictive factors of food production in Ghana. Consequently, the null hypothesis of no causality from temperature, CO2 concentration, economic growth, and population growth to food production in the long-run is rejected. Similar outcome is found for economic and population growth.
From Panel B, temperature and population growth Granger cause cereal, maize, vegetable and roots and tubers production at low frequencies (long-run). However, there is evidence that population growth Granger-causes rice production in the short-run (high frequency) as well. CO2 concentration and economic growth, on the other hand, predict only rice production in the short-run (high frequency). Furthermore, we find that inflation predicts vegetable production in both short-and long-run (high and low frequencies), cereal and maize only in the short-run (high frequency) and only rice production in the long-run (low frequency). These results align with the DOLS and FMOLS outcomes presented in .
5. Concluding remarks
This paper has investigated the effect of climate change variables (temperature, precipitation and CO2 concentration) on food production in Ghana from the period 1970 to 2019 using the fully and dynamic ordinary least squares. It further investigated the frequency causality between food production and its covariates. The paper also controlled for non-climate change variables such as economic growth, population growth, and inflation. Individual crops such as cereal, maize, rice, vegetables and roots and tubers were also examined.
The results from the ADF and the KPSS unit root tests show that the variables are integrated of order zero and one [I(0) and I(1)]. The Maki cointegration test also reveals long-run relationship among the variables. The long-run results from the FMOLS model indicate that temperature significantly reduces food production, maize and roots and tubers production. The implication is that food production in Ghana is more sensitive to temperature. CO2 concentration also impacts maize production negatively. On the other hand, precipitation is good for cereal and maize production. In addition, economic growth and population growth variables positively affect food production: cereal, maize, and rice production. Furthermore, inflation impedes food production, cereal, maize, roots and tubers production in Ghana. The frequency-domain causality results revealed that at low frequencies (long-run), temperature, and population growth Granger-cause food production, cereal, maize, vegetable and roots and tubers production. CO2 only predicts agricultural food and vegetable production in the long-run while it predicts rice production in both the long and short-run. The results show that economic growth Granger causes food production in the long-run while it Granger causes rice production in the short-run. Lastly, there is also a long-run causality that runs from inflation to vegetable and rice production and a short-run causality from inflation to maize and cereal production is also established.
The outcome of the study has policy implications for Ghana. First, the government should invest in research to develop and deploy temperature-resilient seeds/varieties of crops in general, particularly cereal, vegetable and root and tuber crops. Also, the government should develop specific programs and policies to assist sectors that are most vulnerable to climate change and food security. This includes creating and supporting safety nets and other programs such as the Ghana food security and adaptation strategy program initiated in Afram Plains, which address food security, livelihood sustainability and poverty reduction in rural areas of Ghana to help vulnerable populations to become food secure. However, based on our findings, adaptation preference should be given to cereal, vegetable and root and tuber crops since they are more susceptible to high temperature. In addition, farmers should be encouraged to practice soil moisture conservation techniques to enhance the quick release of nutrients and other moisture conservation practices to mitigate the adverse effects of temperature on food production. Finally, since individual crops respond differently to climate change variables, crop diversification methods are desirable to increase nitrogen fixation to take advantage of CO2 fertilization. The outcome of the study and the policy recommendations would be valuable to other developing countries with similar climatic characteristics like Ghana.
The paper acknowledges some limitations. For example, it fails to include irrigation, soil fertility or moisture, budget allocation to the agriculture sector, and direct prices of agricultural food products which may affect food production. Nonetheless, these variables are not included due to the availability of reliable data over the study period considered. As a direction for future research, it will be thought-provoking to analyze the effect of climate change variables on cash crops such as palm and cocoa plantations.
Disclosure statement
No potential conflict of interest was reported by the author(s).
Additional information
Funding
Notes on contributors
Bright Tetteh
Bright Tetteh is currently a master’s student in Economics, specializing in Economic Science at the School of Economics, University of Cape Town (UCT), South Africa under the Mastercard Foundation Scholarship Program (MCFSP). He obtained his bachelor’s degree in Economics from the Kwame Nkrumah University of Science and Technology, Kumasi Ghana. His research interests include macroeconomics, energy economics, climate change, and econometric time series modelling.
Samuel Tawiah Baidoo
Samuel Tawiah Baidoo is currently a PhD candidate at the Department of Economics, Kwame Nkrumah University of Science and Technology (KNUST), Kumasi-Ghana and a lecturer at the Department of Accounting and Finance, School of Business, Christian Service University College, Kumasi-Ghana. His research interests include microeconomics, monetary economics, international economics, public economics and economic policy analysis.
Paul Owusu Takyi
Paul Owusu Takyi is currently a lecturer at the Department of Economics, KNUST, Ghana. He obtained his Ph.D. and Master of Arts in Economics from the National Graduate Institute for Policy Studies (GRIPS), Tokyo, Japan. His current teaching and research interests are in the areas of Public Economics, Macroeconomics, Development Finance (Financial Inclusion), and Monetary Economics.
Notes
1. This term was initially used to describe warmer-water currents that occasionally flow along the coast of Ecuador and Peru, affecting the local fishery. However, it is currently synonymous with the basin-wide warming of the global Pacific Ocean east of the dateline. The fluctuation of the global-scale tropical and subtropical surface pressure trends (Southern Oscillation) coupled with the atmosphere-ocean phenomenon over approximately two to seven years is known as El Niño Southern Oscillation. Mostly, it is measured by surface pressure anomaly difference between the sea surface temperatures in the Central and Eastern Equatorial Pacific or Tahiti and Darwin (Sarginson et al., Citation2012).
2. Given the six variables used to capture the dependent variable, EquationEquation (2)(2)
(2) is estimated for each of the dependent variables (cereal, maize, rice, roots and tubers and production).
3. We perform the Maki cointegration test using cereal, maize, rice, roots and tubers, and, vegetable production as the dependent variable in the four models. Except for vegetable production, all the variables record at least one cointegration equation. Maize and rice production record cointegration at level and level shift with trend while cereal and roots and tubers are cointegrated at level shift only. For brevity, the table for these tests is not reported. Also, since no cointegration was established for vegetable production, we exclude it from the DOLS and the FMOLS estimations. However, we include it in the causality model.
4. We do not include the results for the individual crops in this table. They are reported in .
References
- Adams, R. M., & Hurd, B. H. (1999). Graphically speaking: Climate change and agriculture: Some regional implications. Choices, 14(1), 22–24.
- Ahsan, F., Chandio, A. A., & Fang, W. (2020). Climate change Impacts on Cereal Crops production in Pakistan: Evidence from cointegration Analysis. International Journal of Climate Change Strategies and Management, 12(2), 257–269. https://doi.org/10.1108/IJCCSM-04-2019-0020
- Akudugu, M. A., Dittoh, S., & Mahama, E. S. (2012). The implications of climate change on food security and rural livelihoods: Experiences from Northern Ghana. Journal of Environment and Earth Science, 2(3), 21–29. https://d1wqtxts1xzle7.cloudfront.net/13090958/11._21-29_The_Implications_of_Climate_Change_on_Food_Security_and_Rural_Livelihoods-with-cover-page-v2.pdf?Expires=1660233189&Signature=VQAvg7EoAsYoH0P9-Mkd4T~b2cyRb4bz92yVuDLeVQj7o-oZEyQFEpvbldkuheIamwY-6Ye91cv9VMfnVKM7QYAQ79dv1oT6CpG07xwginkUOTydvzQ9YY86VXJ9waaeLhqwbIaNiToBkGqqrHVG0VqULm~6nOqMBsytmxIovxuCoOnCeOQ3kdPYs6S1zGiI4gP03JnPBNlFMqfSsB9JqVCERTTF3emaIaOdUVDHeN8QooNv6i4mk7NZBgo8I6mZd4yp085Cxw2zEZq~Z3xkfHpnC9~~409sH021CmZifGuovL8Tair8Qnb-t7IMqZekZywFHxk~9f3BOyrQDqOQEQ__&Key-Pair-Id=APKAJLOHF5GGSLRBV4ZA
- Álvarez-Herránz, A., Balsalobre, D., Cantos, J. M., & Shahbaz, M. (2017). Energy innovations-GHG emissions Nexus: Fresh empirical evidence from OECD countries. Energy Policy, 101(June 2016), 90–100. https://doi.org/10.1016/j.enpol.2016.11.030
- Amikuzino, J., & Donkoh, S. A. (2012). Climate variability and yields of major staple food crops in Northern Ghana. African Crop Science Journal, 201, 349–360. https://www.ajol.info/index.php/acsj/article/view/81668
- Amponsah, L., Hoggar, K., Yeboah, G., & Asuamah, S. (2015).Climate change and agriculture: Modelling the impact of carbon dioxide emission on cereal yield in Ghana. Agriculture and Food Sciences Research, 2(2), 32–38. https://mpra.ub.uni-muenchen.de/68051/
- Ankrah, D. A., Kwapong, N. A., & Boateng, S. D. (2021). Indigenous knowledge and science-based predictors reliability and its implication for climate adaptation in Ghana. African Journal of Science, Technology, Innovation and Development, 14(4), 1007–1019. https://doi.org/10.1080/20421338.2021.1923394
- Arize, A. C., Malindretos, J., & Ghosh, D. (2015). Purchasing power parity-symmetry and proportionality: Evidence from 116 countries. International Review of Economics and Finance, 37(1), 69–85. https://doi.org/10.1016/j.iref.2014.11.014
- Arndt, C., Asante, F., & Thurlow, J. (2015). Implications of climate change for Ghana’s economy. Sustainability (Switzerland), 7(6), 7214–7231. https://doi.org/10.3390/su7067214
- Asafu-Adjaye, J. (2014). The economic impacts of climate change on agriculture in Africa. Journal of African Economies, 23(suppl 2), ii17–49. https://doi.org/10.1093/jae/eju011
- Awunyo-Vitor, D., & Sackey, R. A. (2018). Agricultural sector foreign direct investment and economic growth in Ghana. Journal of Innovation and Entrepreneurship, 7(1), 1–15. https://doi.org/10.1186/s13731-018-0094-3
- Ayinde, O. E., Ajewole, O. O., Ogunlade, I., & Adewumi, M. O. (2010). Empirical analysis of agricultural production and climate change: A case study of Nigeria. Journal of Sustainable Development in Africa, 12(6), 275–283. https://jsd-africa.com/Jsda/V12No6_Fall2010_B/PDF/Empirical%20Analysis%20of%20Agricultural%20Production%20and%20Climate%20Change.pdf
- Betts, R. A., Boucher, O., Collins, M., Cox, P. M., Falloon, P. D., Gedney, N., Hemming, D. L., Huntingford, C., Jones, C. D., Sexton, D. M. H., & Webb, M. J. (2007). Projected increase in continental runoff due to plant responses to increasing carbon dioxide. Nature, 448(7157), 1037–1041. https://doi.org/10.1038/nature06045
- Borras, S. M., Jr, Scoones, I., Baviskar, A., Edelman, M., Peluso, N. L., & Wolford, W. (2022). Climate change and agrarian struggles: An invitation to contribute to a JPS forum. The Journal of Peasant Studies, 49(1), 1–28. https://doi.org/10.1080/03066150.2021.1956473
- Breitung, J., & Candelon, B. (2006). Testing for short- and Long-Run causality: A frequency-domain approach. Journal of Econometrics, 132(2), 363–378. https://doi.org/10.1016/j.jeconom.2005.02.004
- Byrnes, B. H., & Bumb, B. L. (2017). Population Growth, Food Production and nutrient requirements. In Nutrient use in crop production (pp. 1–27). CRC Press. https://www.taylorfrancis.com/chapters/edit/10.1201/9780203745281-1/population-growth-food-production-nutrient-requirements-bernard-byrnes-balu-bumb
- Cappuccio, N., & Lubian, D. (2001). Estimation and inference on long-run equilibria: A simulation study. Econometric Reviews, 20(1), 61–84. https://doi.org/10.1081/ETC-100104080
- Chandio, A. A., Akram, W., Bashir, U., Ahmad, F., Adeel, S., & Jiang, Y. (2022a). Sustainable maize production and climatic change in Nepal: Robust role of climatic and non-climatic factors in the long-run and short-run. Environment, Development and Sustainability , 1–31. https://link.springer.com/article/10.1007/s10668-022-02111-1
- Chandio, A. A., Gokmenoglu, K. K., & Ahmad, F. (2021a). Addressing the long-and short-run effects of climate change on major food crops production in Turkey. Environmental Science and Pollution Research, 28(37), 51657–51673. https://doi.org/10.1007/s11356-021-14358-8
- Chandio, A. A., Gokmenoglu, K. K., Ahmad, M., & Jiang, Y. (2022b). Towards sustainable rice production in Asia: The role of climatic factors. Earth Systems and Environment, 6(1), 1–14. https://doi.org/10.1007/s41748-021-00210-z
- Chandio, A. A., Jiang, Y., Akram, W., Adeel, S., Irfan, M., & Jan, I. (2021b). Addressing the effect of climate change in the framework of financial and technological development on cereal production in Pakistan. Journal of Cleaner Production, 288(1), 125637. https://doi.org/10.1016/j.jclepro.2020.125637
- Chandio, A. A., Jiang, Y., Rehman, A., & Rauf, A. (2020b). Short and Long-Run Impacts of Climate Change on Agriculture: An Empirical Evidence from China. International Journal of Climate Change Strategies and Management, 12(2), 201–221. https://doi.org/10.1108/IJCCSM-05-2019-0026
- Chandio, A. A., Magsi, H., & Ozturk, I. (2020a). Examining the effects of climate change on rice production: Case study of Pakistan. Environmental Science and Pollution Research, 27(8), 7812–7822. https://doi.org/10.1007/s11356-019-07486-9
- Chin, M. Y., Puah, C. H., Teo, C. L., & Joseph, J. (2018). The determinants of Co2 emissions in Malaysia: A new aspect. International Journal of Energy Economics and Policy, 8(1), 190–194. https://www.researchgate.net/profile/Chin-Hong-Puah/publication/322919362_The_Determinants_of_CO2_Emissions_in_Malaysia_A_New_Aspect/links/608ab305299bf1ad8d67edf2/The-Determinants-of-CO2-Emissions-in-Malaysia-A-New-Aspect.pdf
- Das, M. K. (2017). Impacts of climate change on agricultural yield: Evidence from Odisha. India, 38(1), 58–65. https://d1wqtxts1xzle7.cloudfront.net/54581817/38661-41767-1-PB-with-cover-page-v2.pdf?Expires=1660234854&Signature=f5Yz5Umisnz-EHDjHKeQA77Ij7kg67GEyFoU~J6JU33EQG-n4MhazX4bMaGBxm6jtlDDe~BVRuT7txN3YSKr~DkBRKd6GeOIF2RXe1YOkHUvacYH2WfIT3c34pr3c~4YsddEgkUQimQxOxoLMCa~6dylWevovkMEkURcUCTVQXqiVXzPB5EqnthyZB3EBVs84IcL63Lh~4jtdExHq5rzv3T~zWS-DrSNoxrIBZRxvAVOl6sxjbGWJtLutzNlvK5UQPUqBWARtmykAysbMNJOQum2jJjA3Nf59m4sTzaXXwGDXhShyfQlgSzoTsvibyPSIhRpXrxM41p0eDuw1y5k2w__&Key-Pair-Id=APKAJLOHF5GGSLRBV4ZA
- Dickey, D. A., & Fuller, W. A. (1981). Likelihood ratio statistics for autoregressive time series with a unit root. Econometrica: Journal of the Econometric Society, 49(4), 1057–1072. https://doi.org/10.2307/1912517
- Enu, P. (2014). Analysis of the agricultural sector of Ghana and its economic impact on economic growth. Academic Research International, 5(4), 267–277.
- Falloon, P., & Betts, R. (2010). Climate impacts on European agriculture and water management in the context of adaptation and mitigation-the importance of an integrated approach. Science of the Total Environment, 408(23), 5667–5687. https://doi.org/10.1016/j.scitotenv.2009.05.002
- Firdaus, R. R., Senevi Gunaratne, M., Rahmat, S. R., & Kamsi, N. S. (2019). Does climate change only affect food availability? What else matters? Cogent Food & Agriculture, 5(1), 1707607. https://doi.org/10.1080/23311932.2019.1707607
- Friedl, B., & Getzner, M. (2003). Determinants of CO2 emissions in a small open economy. Ecological Economics, 45(1), 133–148. https://doi.org/10.1016/S0921-8009(03)00008-9
- Fuhrer, J. (2003). Agroecosystem responses to combinations of elevated CO2, ozone, and global climate change. Agriculture, Ecosystems & Environment, 97(1–3), 1–20. https://doi.org/10.1016/S0167-8809(03)00125-7
- Gambo, S. L., & Idris, M. M. (2018). Effect of population growth on food security in Nigeria. UMYUK Journal of Economics and Development, 1(1), 120–135. https://www.researchgate.net/profile/Suleman-Lawal-Gambo/publication/346975744_Effect_of_Population_Growth_on_Food_Security_in_Nigeria/links/5fd72ecda6fdccdcb8c499a4/Effect-of-Population-Growth-on-Food-Security-in-Nigeria.pdf
- Gebreyes, M., Zinyengere, T. F., Theodory, N., & Speranza, C. I. (2017). Beyond agricultural impacts: Multiple perspectives on climate change and agriculture in Africa. Elsevier Inc, 1–308. https://www.researchgate.net/profile/Nkulumo-Zinyengere/publication/319930756_Beyond_agricultural_impacts_Multiple_Perspectives_on_Climate_Change_and_Agriculture_in_Africa/links/5e3daa1b299bf1cdb91715e7/Beyond-agricultural-impacts-Multiple-Perspectives-on-Climate-Change-and-Agriculture-in-Africa.pdf
- Gedney, N., Cox, P. M., Betts, R. A., Boucher, O., Huntingford, C., & Stott, P. A. (2006). Detection of a direct carbon dioxide effect in continental river runoff records. nature, 439(7078), 835–838. https://doi.org/10.1038/nature04504
- Geweke, J. (1982). Measurement of linear dependence and feedback between multiple time series. Journal of the American Statistical Association, 77(378), 304–313. https://doi.org/10.1080/01621459.1982.10477803
- Gokmenoglu, K., Kirikkaleli, D., & Eren, B. M. (2019). Time and frequency domain causality T esting: The causal linkage between FDI and economic risk for the case of Turkey. Journal of International Trade and Economic Development, 28(6), 649–667. https://doi.org/10.1080/09638199.2018.1561745
- Gregory, A. W., & Hansen, B. E. (1996). Residual-based tests for cointegration in models with regime shifts. Journal of Econometrics, 70(1), 99–126. https://doi.org/10.1016/0304-4076(69)41685-7
- Gul, A., Chandio, A. A., Siyal, S. A., Rehman, A., & Xiumin, W. (2022). How climate change is impacting the major yield crops of Pakistan? an exploration from long-and short-run estimation. Environmental Science and Pollution Research, 29(18), 26660–26674. https://doi.org/10.1007/s11356-021-17579-z
- Gyamfi, S., Amankwah, D. F., Nyarko, K. E., Sika, F., & Modjinou, M. (2018). The energy efficiency situation in Ghana. Renewable and Sustainable Energy Reviews, 82(1), 1415–1423. https://doi.org/10.1016/j.rser.2017.05.007
- Hatemi-j, A. (2008). Tests for cointegration with two unknown regime shifts with an application to financial market integration. Empirical Economics, 35(3), 497–505. https://doi.org/10.1007/s00181-007-0175-9
- Hosoya, Y. (1991). The decomposition and measurement of the interdependency between second-order stationary processes. Probability Theory and Related Fields, 88(4), 429–444. https://doi.org/10.1007/BF01192551
- Ibn Musah, A. A., Du, J., Bilaliib Udimal, T., & Sadick, M. A. (2018). The nexus of weather extremes to agriculture production indexes and the future risk in Ghana. Climate, 6(4), 86. https://doi.org/10.3390/cli6040086
- Khalaf, L., & Urga, G. (2014). Identification robust inference in cointegrating regressions. Journal of Econometrics, 182(2), 385–396. https://doi.org/10.1016/j.jeconom.2014.06.001
- Khan, Z., Ali, M., Kirikkaleli, D., Wahab, S., & Jiao, Z. (2020). The impact of technological innovation and public-private partnership investment on sustainable environment in China: Consumption-based carbon emissions analysis. Sustainable Development, 28(5), 1317–1330. https://doi.org/10.1002/sd.2086
- Kumawat, S., & Yadav, B. (2021). Impact of climate change on agriculture. Biomedicine (India), 40(4), 412–413. https://doi.org/10.51248/.v40i4.306
- Kurukulasuriya, P., & Rosenthal, S. (2003). Climate change and agriculture. Climate Change and Agriculture: A Review of Impacts and Adaptations, 6(3), 101. https://doi.org/10.1016/0169-5347(91)90186-2
- Kwawu, J. D., Sarpong, D. B., & Agyire-Tettey, F. (2021). Technology adoption intensity and technical efficiency of maize farmers in the techiman municipality of Ghana. African Journal of Science, Technology, Innovation and Development, 14(2), 532–545. https://journals.co.za/doi/abs/10.1080/20421338.2020.1866145
- Kwiatkowski, D., Phillips, P. C. B., Schmidt, P., & Shin, Y. (1992). Testing the null hypothesis of stationarity against the alternative of a unit root. how sure are we that economic time series have a unit root? Journal of Econometrics, 54(1–3), 159–178. https://doi.org/10.1016/0304-4076(92)90104-Y
- Maki, D. (2012). Tests for cointegration allowing for an unknown number of breaks. Economic Modelling, 29(5), 2011–2015. https://doi.org/10.1016/j.econmod.2012.04.022
- Mereu, V., Carboni, G., Gallo, A., Cervigni, R., & Spano, D. (2015). Impact of climate change on staple food crop production in Nigeria. Climatic Change, 132(2), 321–336. https://doi.org/10.1007/s10584-015-1428-9
- Ministry of Environment, Science, Technology and Innovation. (2013). Ghana national climate change policy ministry.ministry of environment, science, technology and innovation (MESTI). Und Lungenkrankheiten. Atemwegs-.
- MoFA. (2020). Ministry of food and agriculture medium term expenditure framework (MTEF) for 2020-2023 programme based budget estimates for 2020 (pp. 1–87). https://ideas.repec.org/a/rfa/bmsjnl/v6y2020i4p1-13.html
- Ntiamoah, E. B., Li, D., Appiah-Otoo, I., Twumasi, M. A., & Yeboah, E. N. (2022). Towards a sustainable food production: Modelling the impacts of climate change on maize and soybean production in Ghana. Environmental Science and Pollution Research, 1–20. https://doi.org/10.1007/s11356-021-17416-3
- Obisesan, M., & Chitakira, M. (2020). Investigating climate change awareness and adaptation strategies among female farmers in the Lephalale municipal area in South Africa. African Journal of Science, Technology, Innovation and Development, 13(6), 727–734. https://doi.org/10.1080/20421338.2020.1796005
- Ofori-Appiah, Y., Onumah, E. E., & Asem, F. E. (2021). Efficiency and productivity analysis of pineapple farmers in the Akwapim-South District of Ghana: A distance function approach. African Journal of Science, Technology, Innovation and Development, 14(2), 512–522. https://journals.co.za/doi/abs/10.1080/20421338.2020.1857544
- Okunola, A. M., S. P Nathaniel, S. P., & Festus, V. B. (2018). Revisiting population growth and food production nexus in Nigeria: An ARDL approach to cointegration. Agricultural and Resource Economics: International Scientific E-Journal, 3(1), 5–26. https://ageconsearch.umn.edu/record/281759/
- Olatunji, G. B., Omotesho, O. A., Ayinde, O. E., & Adewumi, M. O. (2012). Empirical analysis of agricultural production and inflation rate in Nigeria (1970-2006). Agrosearch, 2(1), 21–30. https://doi.org/10.4314/Agrosh.v.12i1
- Owusu, P. A., & Asumadu-Sarkodie, S. (2017). Is there a causal effect between agricultural production and carbon dioxide emissions in Ghana? Environmental Engineering Research, 22(1), 40–54. https://doi.org/10.4491/eer.2016.092
- Ozer, M., & Kamisli, M. (2015). Frequency domain causality analysis of interactions between financial markets of Turkey. International Business Research, 9(1), 176. https://doi.org/10.5539/ibr.v9n1p176
- Parry, M. L., Canziani, O., Palutikof, J., der Linden, P. V., & Hanson, C.Eds.,(2007).Climate change 2007-impacts, adaptation and vulnerability: Working group II contribution to the fourth assessment report of the IPCC.Vol. 4 Cambridge University Press.Pauw, K. 2018.The state of agriculture in Ghana.” the state of agriculture in Ghana (May) (pp. 1–24). https://d1wqtxts1xzle7.cloudfront.net/4968144/fi-with-cover-page-v2.pdf?Expires=1660238717&Signature=c4ceN2UVktvcfbQ9T0JBdTn0v5wiapxrV~g6HdBeMwQPiOf-sCDuKs~BQIqnsxwpPASf0my1m1Bpi2y~ln5B9i32mQrew5JD2IU7PssPXh6rUCub~EexWQF6NUysoYlcb-98KIo4XQeky8zuPwFPkjAmU~7CuwSNBLVfvlXMVIhs4~amgtsdMvrlVFUj0sbmuw7KCREML7YH7Cef25BHSOBHsy1z83az580wmVnoV8c-C7D~cHztS-BZugA3HXG1aD4bIq9O7oc86psaN3UTeF9eC6eBJoosaa-1pwCFOYX0BFhmx9sbSUukL8Qjtk74nS4Xz~tfJcrAzoNLwdXKvw__&Key-Pair-Id=APKAJLOHF5GGSLRBV4ZA
- Pauw, K. (2018). “The state of agriculture in Ghana.” The State of Agriculture in Ghana, (May), 1–24. http://www.sablog.kpmg.co.za/2013/12/state-agriculture-south-africa/
- Phillips, P. C. B. B., & Hansen, B. E. (1990). Statistical inference in instrumental variables regression with (1) processes. The Review of Economic Studies, 57(1), 99–125. https://doi.org/10.2307/2297545
- Pinto, A. D., Demirag, U., Haruna, A., Koo, J., & Asamoah, M. (2012). Climate change, agriculture, and foodcrop production in Ghana. International Food Policy Research Institute, 3(September), 6. https://www.africaportal.org/publications/climate-change-agriculture-and-foodcrop-production-in-ghana/
- Popović, V., Jovović, Z., Marjanović-Jeromela, A., Sikora, V., Mikić, S., Bojović, R., & Šarčević-Todosijević, L. 2020. “Climatic change and agricultural production.” In Book of proceedings, GEA (geo eco-eco agro) international conference, 28-31 May 2020, Podgorica, Montenegro (pp. 160–166). Podgorica: University of Montenegro, Faculty of Architecture GEA (Geo Eko-Eko Agro).
- Rafindadi, A. A., & Usman, O. (2019). Globalization, energy use, and environmental degradation in South Africa: Startling empirical evidence from the maki-cointegration test. Journal of Environmental Management, 244(January), 265–275. https://doi.org/10.1016/j.jenvman.2019.05.048
- Reilly, J., Tubiello, F., McCarl, B., Abler, D., Darwin, R., Fuglie, K., Hollinger, S., Izaurralde, C., Jagtap, S., Jones, J., Mearns, L., Ojima, D., Paul, E., Paustian, K., Riha, S., Rosenberg, N., & Rosenzweig, C. (2003). U.S. agriculture and climate change: new results. Climatic Change, 57(1–2), 43–69. https://doi.org/10.1023/A:1022103315424
- Ringius, L., Downing, T., Hulme, M., Waughray, D., & Selrod, R. 1996. Climate change in Africa: Issues and challenges in agriculture and water for sustainable development. Cicero report. https://pub.cicero.oslo.no/cicero-xmlui/handle/11250/192102
- Rosenzweig, C., & Hillel, D. (1995). Potential impacts of climate change on agriculture and food supply. Consequences, 1(2), 23–32. https://www2.hcmuaf.edu.vn/data/ndnang/file/KHI%20TUONG%20DAI%20CUONG/Tai%20lieu%20chuyen%20de/Potential%20Impacts%20of%20Climate%20Change%20on%20Agriculture%20and%20Food%20Supply.pdf
- Sagoe, R. (2006). Climate change and root crop production in Ghana a report prepared for environmental protection agency (Epa), accra-Ghana. Environmental Protection, 1–37. https://citeseerx.ist.psu.edu/viewdoc/download?doi=10.1.1.582.1329&rep=rep1&type=pdf
- Sarginson, R. E., Taylor, N., De La Cal, M. A., & Van Saene, H. K. F. (2012). Glossary of terms and definitions. Infection Control in the Intensive Care Unit, 9788847016, 3–16. https://doi.org/10.1007/978-88-470-1601-9_1
- Sarkodie, S. A., & Owusu, P. A. (2017). The relationship between carbon dioxide, crop and food production index in Ghana: By estimating the long-run elasticities and variance decomposition. Environmental Engineering Research, 22(2), 193–202. https://doi.org/10.4491/eer.2016.135
- Siamabele, B. (2021). The significance of soybean production in the face of changing climates in Africa. Cogent Food & Agriculture, 7(1), 1933745. https://doi.org/10.1080/23311932.2021.1933745
- Stige, L. C., Stave, J., Chan, K. S., Ciannelli, L., Pettorelli, N., Glantz, M., Herren, H. R., & Chr Stenseth, N. (2006). The effect of climate variation on agro-pastoral production in Africa. Proceedings of the National Academy of Sciences of the United States of America, 103(9), 3049–3053. https://doi.org/10.1073/pnas.0600057103
- Stock, J. H., & Mark, W. W. (1993). A Simple Estimator of Cointegrating Vectors in Higher Order Integrated Systems. Econometrica, 61(4), 783. https://doi.org/10.2307/2951763
- Thornton, P. (2012). Recalibrating food production in the developing world: Global warming will change more than just the climate. CCAFS Policy Brief No. 6, 1–6, CGIAR Research Program on Climate Change, Agriculture and Food Security (CCAFS). www.ccafs.cgiar.org
- Warsame, A. A., Sheik-Ali, I. A., Ali, A. O., & Sarkodie, S. A. (2021). Climate change and crop production nexus in somalia: An empirical evidence from ARDL technique. Environmental Science and Pollution Research, 28(16), 19838–19850. https://doi.org/10.1007/s11356-020-11739-3
- Williams, P. A., Crespo, O., Atkinson, C. J., & Essegbey, G. O. (2017). Impact of climate variability on pineapple production in Ghana. Agriculture & Food Security, 6(1), 1–14. https://doi.org/10.1186/s40066-017-0104-x
- Wood, A. L., Ansah, P., Rivers, L., III, & Ligmann-Zielinska, A. (2021). Examining climate change and food security in Ghana through an intersectional framework. The Journal of Peasant Studies, 48(2), 329–348. https://doi.org/10.1080/03066150.2019.1655639
- World Bank, 2007. ”World development report 2008: Agriculture for development.” https://doi.org/10.1596/978-0-8213-7235-7
- Zaied, Y. B., & Zouabi, O. (2016). Impacts of climate change on tunisian olive oil output. Climatic Change, 139(3–4), 535–549. https://doi.org/10.1007/s10584-016-1801-3