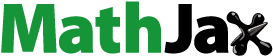
Abstract
The implacable list of diversification indices allows a wide range of selection opportunities for the researchers. The absence of consensus on the selection of suitable technology diversification index, however, may lead to a lack of objectivity with ample grounds. In this study, we focus on the case of technology diversification using patent to derive empirical implication for selecting suitable diversification index. To obtain the content validity of diversification index, three cases were tested: cross section, single and multiple time periods. As a result, diversification indices are separated into two groups: HHI, Gini-Simpson, 1/HHI, and Entropy for PC1 and Variety and Rao-Stirling for PC2. In this context, technology diversification can be explained by two perspectives of diversification: balance-centered and hetero-centered diversification. The balance-centered diversification implies the proportion of elements are the target of interest while hetero-centered diversification refers to variety and disparity of diversification, which focuses on the degree of differentiation among elements. In applicant-level technology diversification studies, these two diversification perspectives are recommended to be used. Subject classification codes: include these here if the journal requires them
PUBLIC INTEREST STATEMENT
This paper discusses the selection problem of technology diversification index using patent data. In order to address the content validity of selecting proper diversification indices, the lists of diversification indices with diversity properties and three different time-domain cases were examined. From the result, technology diversification is divided into two perspectives of diversification: balance-centered and hetero-centered diversification. The balance-centered diversification implies the proportion of elements are the target of interest while hetero-centered diversification refers to variety and disparity of diversification, which focuses on the degree of differentiation among elements. Referring to this, a consideration of two diversification perspectives and using representative indices for each are recommended.
1. Introduction
In management and economics, diversification has been discussed in the context of research, business (Geringer, Tallman, & Olsen, Citation2000; Singh, Gaur, & Schmid, Citation2010; Stephan, Citation2002; Woerheide & Persson, Citation1992), geography (Geringer et al., Citation2000; Stephan, Citation2002), and technology (Chiu, Lai, Lee, & Liaw, Citation2008; Kim, Lee, & Cho, Citation2016; Kook, Kim, & Lee, Citation2017; Lin & Chang, Citation2015; Wang, Ning, & Prevezer, Citation2015). Especially, technology diversification has been regarded as one of the important issues in technology management, technology portfolio analysis, and etc. with relation to the contribution of technology on economic performance and growth. As the advent of technology convergence phenomena among different industries and technologies highlights the value of technology competitiveness, technology diversification study can contribute for understanding the diversification activities and establishing a diversification strategy.
Along with the importance of technology diversification, various diversification measurements were introduced and used in previous studies. The variety of available diversification indices provides a wide range of selection opportunities to researchers. At the same time, it implies the lack of a rigorous consensus for choosing an appropriate diversification index either in general or specific situations. In the case of ecology, Morris et al. (Citation2014) pointed out the difficulty of quantifying biodiversity due to the multitude of proposed indices. The absence of consensus on the selection of a technology diversification index (TDI) may lead to justified allegations of a lack of objectivity. Since both interpretation and consequences can differ depending on the characteristics of the index used, a diversification index should be selected with the consideration of the validity of TDI.
There were few attempts to address this problem, but they were limited to certain situations and not of general application. To prove the content validity of TDI, the analytical perspective should not be restricted to limited cases. In order to obtain the content validity, the evaluation should be considered more rigorously in the context of various cases. For instance, the result of diversification can differ according to the measurement, the analytic sample, and the target of interest. A more generalized understanding of diversification indices, therefore, can contribute to the various research areas where diversification is a point of interest.
In this study, the empirical implications of selecting a suitable TDI are derived using patent data. To prove the content validity of the selection of a TDI, the three cases include cross-section, single time period, and multiple time periods, with comparisons among three country groups of 18 countries. Due to the reliability and usability of patents, various cases can be studied and comparisons among them can be made to obtain a more generalized understanding. In addition, the list of diversification indices that are frequently used in previous studies are examined to determine which are better suited for measuring technology diversification. For the evaluation of diversification indices, Stirling (Citation2007)’s general framework for diversity property is used as a reference for understanding the difference among indices. As a methodology, PCA is conducted to determine distinctive relations among the variables and derive the dominant variable of each principal component. Since the relationships between diversification indices do not always satisfy the mathematically predicted patterns (Nagendra, Citation2002; Stirling & Wilsey, Citation2001), performing PCA on real data can ensure that the conclusions are valid (Andy Stirling, Citation2007).
The remainder of this chapter is laid out as follows. Section 2 reviews the content validity of TDI and its related indices in detail. Section 3 describes the data and methods used in this study. Lastly, the results and conclusions are set out in Sections 4 and 5.
2. Literature review
2.1. The content validity of diversification index
The validity of an index can be studied from the perspective of either face or content validity. Face validity observes whether a test measures what it is supposed to measure while content validity observes whether a measure represents what it superficially appears to measure. Simply put, monthly income indicates face validity of one’s financial ability per month. Compared to face validity, however, content validity requires a rigorous evaluation of the measure. In general, content validity requires either recognition by an expert or statistical testing as it deals with superficial measurements. Robins and Wiersema (Citation2003) pointed out the importance of using an appropriate diversification index by emphasizing the content validity of a diversification measure.
The main purpose for conducting content validity of a diversification index is that the lack of a prior assessment of content validity may cause problems in obtaining consistent results due to the sensitivity of using each index. Although the result can differ depending on the diversity measurement (Morris et al., Citation2014), there is no sufficient consensus on the selection of a diversification measure (Robins & Wiersema, Citation1995, Citation2003); the sensitivity of diversification measurements may cause contradictory results and unexpected confusion depending on the choice of a diversification index. Robins and Wiersema (Citation2003) examined the content validity of an entropy index and a concentric index to see whether these indices are suitable for use as indicators of portfolio relatedness. Their results showed that the concentric index positively influenced dominant business focus (the relative size of dominant businesses) and negatively influenced pure diversification (the number of businesses); the entropy index exhibited the opposite characteristics. From these findings, they concluded that these sorts of sensitivities can create ambiguities in strategy research. Woerheide and Persson (Citation1992) evaluated five diversification indices for measuring unevenly distributed stock portfolios. Among the five indices—the complement of the Herfindhal index, Rosenbluth’s index, the exponential of entropy index (Marfels, Citation1971), the comprehensive concentration index (Marfels, Citation1971), and the entropy index (Hart, Citation1971)—the first was found to be the most adequate for general use.
Therefore, rather than simply adopting one, the prior assessment of selecting a proper index is needed.
2.2. Technology diversification
Technology diversification refers to the degree of a firm’s technology diversity. Although there is consensus on the definition and the sources of technology diversification, there has not been an in-depth discussion on the TDI. In case of technology diversification, Variety, the Herfindahl-Hirschman index (HHI), the modified HHI, and Entropy are some of the generally used indices (Table ). These indices are widely used in various areas of research and detailed explanations of them will be provided in the following section.
Table 1. Technology diversification targets and indices
As described in Table , patent data are used as analytic material for measuring technology diversification. As an effective and valid indicator for firm-level technological activities, patents allow the grasping of a firm’s technological activities rooted in a formal R&D organization (Pavitt, Citation1988). In relevant studies, it is regarded as a form of technological output that explains a firm’s innovative capabilities (Kim, Lim, & Park, Citation2009; Kim, Jung, & Hwang, Citation2018; Kim, Jung, Hwang, & Hong, Citation2018; Lee & Kang, Citation2015). Since patent data span 80 patent offices worldwide from the 1970s, comparisons across countries and over a wide range of time periods are available.
In general, the IPC is used as a target of technology diversification. Argyres (Citation1996) suggested that patents assigned to different areas of technology can be observed as different technological applications. In this sense, the technology classifications assigned to a patent can be used to distinguish a firm’s technological applications. Jaffe (Citation1989) viewed technology as consisting of a number of distinct “technological areas” and used this approach to characterize a firm’s technological position. Technology classifications provided by patents offer detailed information on the area of technology, which is relevant for assessing a firm’s technological activities (Stephan, Citation2002). In this sense, a patent is acceptable material as it includes the IPC, which is a type of technology classification that distinguishes different technologies in a hierarchical order. Even though the IPC is selected as a diversification target, its measurement level has to be determined. As described in Table , technology diversification is measured either with reference to a sub-class or technology field. The sub-class refers to the hierarchical level of the IPC and technology field refers to a bundle of related industry classifications from the IPCs. Accordingly, the criteria for the technology field may differ depending on either references or countries. Since the sub-class level of the IPC can be used without restrictions across different countries, it is adopted as a diversification target instead of the technology field.
2.3. Diversity properties
The main reason that the values measured by each diversification index differ is due to differences in their points of interest. In this vein, Stirling (Citation2007) proposed a general framework for diversity that can be applied in the field of science, technology, and society. He classified three basic properties of diversity: the number of elements (Variety), the distribution of elements (Balance), and the difference between the elements (Disparity). All these properties are necessary but individually insufficient as each property constitutes other two (Stirling, Citation1998; Citation2007). Thus, it is more likely to assume that the selection of a diversification index should consider multiple aspects rather than only one particular index. In this study, a total of six diversity indices that are widely used in technology diversification studies are selected: Variety, HHI, 1-HHI, 1/HHI, Entropy, and Rao-Stirling (Table ). Although it was not mentioned in the previous section, Rao-Stirling is included to cover the disparity of diversification.
Table 2. Formulas of diversification indices
Variety, which is the simplest and the most intuitive diversification index, counts the number of entities (Macarthur, Citation1965). As already described in its title, it only considers the variety of diversification. In economics, it is often used as a simple enumeration of firms or products (Cohendet, Llerena, & Sorge, Citation1992; Kauffman, Citation1992; Saviotti & Mani, Citation1995). For example, the kinds of IPC sub-classes owned by a firm represents the richness of its technology diversification.
Instead of counting a total number, using the proportion of each target of diversification is a more general approach used by most indices. The HHI is one of the most widely adopted indices for the measurement of technological concentration or diversification (Berry, Citation1971). Stirling’s framework (Citation2007) covers the variety and balance properties of diversification. This index was initially adopted in management literature to observe firms’ diversification (Geringer et al., Citation2000; Sambharya, Citation1995), and also used in the technology diversification (Gambardella & Torrisi, Citation1998). The results of the HHI are quite intuitive as its formula is designed to treat proportions equally using a square root. For example, an applicant with an equally distributed proportion of IPC sub-classes has a lower HHI compared to one with concentrated technologies.
Rather than simply adopting the HHI, modified HHIs are introduced (Bas & Patel, Citation2005; Garcia-Vega, Citation2006; Lin & Chang, Citation2015; Quintana-García & Benavides-Velasco, Citation2008). It is obvious that a concentrated firm’s value is low while diversified firms require higher values of diversification. A firm with a higher diversification value is assumed to be more diversified. Another modification of the HHI is measuring diversification by taking its inverse (Leten, Belderbos, & Van Looy, Citation2007). The implications of inversed HHI results are similar to those of the 1-HHI, but it emphasizes the apparent differences in diversification. For instance, a comparison between HHI values of 1/4 and 5/8 becomes 6/8 and 3/8 when transformed into the 1-HHI. When they are converted into the inverse HHI, they become 4 and 5/8; the difference between them is greater than in the previous indices.
Similar to the HHI, Entropy also covers the variety and balance of diversification. It was first introduced in the second law of thermodynamics. In thermodynamics, entropy represents the amount of energy that can no longer be reused. An increase in entropy represents chaos at the molecular level where the possibility of transforming energy into work is low. The concept of entropy used in diversification is somewhat similar, but more related to information theory. In information theory, entropy, also known as the Shannon index, deals with the uncertainty (or imbalance) of information (Shannon, Citation1948). An increased value of entropy indicates an increase in information uncertainty. For instance, if a firm obtains new information, it means that the understanding of its own overall information decreases. Here, the degree of information uncertainty depends on the proportion of new information to overall information. In the case of diversification, an increased value of entropy implies an increase in diversification in the sense of information uncertainty. Unlike the HHI, however, entropy gives more weight to lower values so that it can highlight the imbalance among components.
Lastly, the Rao-Stirling index not only measures the proportion of each entity, but also the Euclidean distance between the entities (Stirling, Citation2007). Unlike previous indices, it considers disparity in addition to variety and balance. In order to determine the disparity among elements, it conceives of the distance between them in a so-called disparity space (Solow, Polasky, & Broadus, Citation1993). Disparity space is a unique n-dimensional space where n refers to the number of attributes of elements. Here, the attributes of elements can either be cardinal, interval, or binary terms. In this case, the attributes of elements are obtained by the cardinal terms of an applicant’s IPC classes. After normalization and weighting, the Euclidean distance between elements can be scaled to reflect distances in disparity space (Kruskal, Citation1964).
3. Data
For the empirical analysis, Worldwide Patent Statistical Database of the European Patent Office (EPO) is used. The EPO patent data have the advantage of minimizing home-country bias as firms are headquartered in different countries (Schmoch, Citation1999). From previous studies, technology diversification has been discussed in three different cases: cross-section, single period, and multiple period (Table ). The cross-sectional case observes technology diversification at a certain time. The single-period case observes technology diversification within a specified time period. The multiple-period case separates the time periods. For comparison, three data sets are constructed for the three cases including the three groups of countries (high-, mid-, and low-level) classified by their level of patent applications. For the high-level patent application group, the top-six patent application countries—the United States (US), Germany (DE), China (CN), Spain (EP), Japan (JP), and Korea (KR)—are included. For the mid-level patent application group, Sweden (SE), the Russian Federation (RU), Italy (IT), Brazil (BR), and the Netherlands (NL) are selected. For the low-level patent application group, Bulgaria (BG), Malaysia (MY), Serbia and Montenegro (YU), the Eurasian Patent Organization (EA), Slovenia (SI), and the Philippines (PH) are included. Here, YU is intentionally included to see how diversification indices are observed where the number of patent applications dramatically decreases. Case 1 uses cross-sectional data in 2015 and 10,000 applicants are randomly selected from each country. Case 2 covers a single time period of ten years. Case 3 covers multiple five-year time periods. Here, the empirical cases of the US, the NL, and the PH are chosen. For the latter two cases, applicants who have patents in the beginning and end years are selected. Overall descriptions of the sample data for each case are set out in Table .
Table 3. Summary of the empirical studies on technology diversification
Table 4. Description of sample data for the three different cases
4. Methodology
4.1. Technology diversification calculation
Prior to the calculation of TDIs, the data is organized by each applicant’s IPC sub-class assigned to the patent. In order to remain consistency among countries, technology diversification is measured with IPC sub-classes for all cases. The sub-class level of the IPC is considered as units of technology for the selection of new technologies (Kim, Citation2013). Basically, technology diversification is measured annually and its accumulated form is used for cases with multiple time periods (Figure ). One of the requirements for a TDI is that it should be capable of capturing either changes or variations. With this rule, the changes in applicant-level technology diversification can be observed while the overall technological information is secured. Under this condition, diversification indices are derived with the equations mentioned in the previous section.
4.2. Principal component analysis (PCA)
PCA is a statistical procedure for reducing the dimensions of a data set. Here, the dimensions of a data set refer to the measurement type. It uses orthogonal transformation to convert a set of possibly correlated variables into a new set of linearly uncorrelated variables. By doing so, the following goals can be achieved (Abdi & Williams, Citation2010). First of all, the most important information from the data table can be extracted. In addition, the size of the data can be compressed while retaining important information. Lastly, the patterns of similarity in the observations and variables can be analyzed. Given these advantages, PCA has been widely used in big data analysis and in index studies (Morris et al., Citation2014). One of the key conditions for PCA is the large sample size (Chao & Wu, Citation2017). For instance, if the number of observations is less than the number of variables, PCA can be influenced by the outliers in the database and the results will not be consistent (Hastie, Tibshirani, & Jerome, Citation2009). In this study, the result of PCA is reliable as it uses a large data sample with universally accessible patent data.
In index studies, PCA is used for two different purposes. The first is to directly propose a new index using the results of the PCA (Chao & Wu, Citation2017; Filmer & Pritchett, Citation2001; Vyas & Kumaranayake, Citation2006). The outcome of the PCA—the principal component—is a linear combination of variables where the coefficient of each variable indicates its importance or weight with respect to a given principal component. By summing the products of the input variables and the PCA coefficients, a PCA-based index is proposed. This is one of the data-driven procedures of index mining or a systematic search for optimal variable aggregation (Chao & Wu, Citation2017). However, a principal component has a limitation to be used as a new index. In general, a new index is proposed with a generalized equation and an explanation on how the equation is designed with scientific sense. The result of PCA, however, does not guarantee the consistent result for all time and it is not always plausible to explain the weight and direction of variables.
Secondly, PCA is used to provide a guideline for selecting indices taking into consideration their similarities and differences (Godshalk & Timothy, Citation1988; Morris et al., Citation2014). As indices that are designed for the specific purposes share a certain level of similarity, determining the difference among actual measurements can provide a considerable amount of information in selecting a diversification index. Compared to using a simple correlation test, PCA has the advantages of dealing with a large number of variables and comparing in hierarchical order with respect to the relative importance of principal components. In this sense, principal components obtained from PCA can address the problem of collinearity (Chao & Wu, Citation2017). With this approach, the unobserved properties of indices that are shared among them can be observed and it can be used as a reference for selecting a diversification index. In this study, therefore, PCA is used to select a diversification index by analyzing their similarities and differences.
4.3. Selecting technology diversification index
Once principal components are derived, diversification indices are classified into either PC1 or PC2. In previous studies, the importance value (IV) of each index is measured to determine which is best able to differentiate principal components (Wilsey, Chalcraft, Bowles, & Willig, Citation2005). Here, IV synthesizes information based on the importance of each principal component and generates a value representing the overall ability of each diversification index to distinguish principal components (Morris et al., Citation2014). Previous studies, however, proposed using at least two measures; they failed in deriving an ideal index (Heino, Mykrä, & Kotanen, Citation2008; Stirling & Wilsey, Citation2001; Whittaker, Citation1972) as selecting a proper index requires the consideration of diverse situations and conditions. Thus, a comparison between principal components precedes index selection.
Following previous studies, the comparison among principal components is limited to PC1 and PC2 which explain the majority of the data. Between PC1 and PC2, the absolute values of the coefficient of each diversification index are compared. The coefficient of each principal component’s variable is also regarded as the weight of the variable. In other words, the coefficient of a diversification index shows its level of importance with respect to that principal component. In this sense, the coefficients of a diversification index can be used to determine the principal component that diversification most affects. For instance, if the absolute value of PC1’s variety is greater than that of PC2, it is assumed that PC1 is more influenced by variety than PC2. In this manner, each diversification index can be classified. One of the most important features of an index is whether it can show differences well. Among the list of diversification indices for each principal component, the one with the largest standard deviation (SD) is selected as the representative index. Rather than using all usable indices with similar meanings, selecting a representative index is recommended like any other analysis using a huge data set and calculation. A larger SD of an index, a wider spread of values, implies more an index can show the variation of data. This selection criterion is promising as a variable with the largest SD can be regarded as the one that can explain the changes well compared to others.
5. Empirical studies
For each case, the summarized results are elaborated in Table –. Firstly, the absolute value of coefficients of indices was compared to distinguish each index into PC1 and PC2. For each index, the principal component which each index is more relying on is elaborated. Secondly, the normalized SD of each index are described in parentheses. Within each principal component group, an index with the biggest SDs is represented with the bold type. The descriptive statistics are included in the Appendix.
Table 5. Summarized result of case 1
Table 6. Summarized result of case 2
Table 7. Summarized result of case 3
5.1. Case 1: cross-section
In case 1, applicant-level technology diversifications in 2015 are measured and those of six different countries are compared. YU is excluded as there were no patent applications in 2015 due to their separation in 2006. The result is somewhat interesting because the results of the PCA among the six countries in each of the three groups are very alike. In this cross-sectional data set, the Gini-Simpson and the Rao-Stirling are shown to express the representative indices for PC1 and PC2, respectively. In the high-level patent application group, the 1/HHI is more affected by PC2 in CN. This may be due to the relatively higher average number of IPC sub-classes per applicant and active IPC sub-classes in CN, as the 1/HHI is more correlated to the Variety and the Rao-Stirling. Although the separation between PC1 and PC2 is apparent in the high-level patent application groups, the mid- and low-level patent application groups show few differences in their results as Variety is allocated to PC1 in BE, IT, the NL, the RU, the EA, the PH, and SI. However, these small differences do not influence the overall result as the representative indices are the same for all groups.
5.2. Case 2: single time periods
In case 2, applicant-level technology diversification between 1996 and 2015 is measured. Here, the PCA result is consistent to case 1; the same indices are allocated to the same principal components. In the case of the mid-level (low-level) patent application group, the result is almost similar except that the 1/HHI (Variety) of IT (the PH) is classified to PC2 (PC1). However, this can be regarded as a minor difference as the representative indices for each principal component remain the same. In summary, the PCA results of case 2 are similar to case 1; the Gini-Simpson and the Rao-Stirling are the most important indices for PC1 and PC2 (Table ). Once again, applicant-level technology diversification in single time periods can be conducted from two different perspectives.
5.3. Case 3: multiple time periods
In case 3, technology diversifications are measured across four different time periods. Among the 18 countries, the US, the NL, and the PH are selected. Similar to the previous cases, a clear distinction between PC1 and PC2 is observed. In most cases, the HHI, the Gini-Simpson, the 1/HHI, and Entropy are spread along PC1 while only Variety and the Rao-Stirling mainly change with PC2. This also shows the consistent result to the previous cases that for analyzing diversification within a single country with multiple time periods, two different aspects of diversification indices can be used.
6. Discussion
As a result, the interesting findings are those related to improving the understanding of technology diversification.
Firstly, a clear distinction among diversification indices is observed in all cases. As a result of the PCA, the diversification indices are separated into two groups based on principal components: the HHI, the Gini-Simpson, the 1/HHI, and Entropy for PC1 and Variety and the Rao-Stirling for PC2. This is somewhat consistent with Stirling’s (Stirling, Citation2007) framework as PC1 represents balance and PC2 represents both variety and disparity. Since theories or existing evidence supporting index outcomes are important (Chao & Wu, Citation2017), proposing two diversification perspectives is reliable and supported by Stirling’s (Stirling, Citation2007) framework. In this context, diversification can be explained using two perspectives of diversification: balance-centered and hetero-centered diversification (Figure ). Balance-centered diversification refers to the balance of diversification where the proportions of elements are the targets of interest. On the other hand, hetero-centered diversification refers to the variety and disparity of diversification, which focuses on the degree of differentiation among elements. In addition, the variables with the biggest SD are shown to be the Gini-Simpson and the Rao-Stirling. Since the bigger SD implies that the variations in the indices are more observable, the Gini-Simpson and the Rao-Stirling are recommended for observing PC1 and PC2.
To supplement the selection of these two indices, a comparison among diversification indices for each diversification perspective is conducted. Figure shows the time-series graph of balance-centered diversification in the US. Here, the size of each dot indicates the average number of IPC sub-classes used for patent applications. Although all these diversification indices show similar variations, the Gini-Simpson appears to accurately reflect diversification changes and reality. From 2000 to 2001, a great shift in technology diversification occurred, incrementing the use of numerous IPC sub-classes. Compared to other indices, the Gini-Simpson increased by a greater value, indicating that it detects diversification changes more clearly. Another interesting point is the period between 2008 and 2009 when the financial crisis occurred; the Gini-Simpson showed a more reasonable outcome as it shows the smallest changes in that time period.
In the case of hetero-centered diversification, Figure shows the time-series graph of hetero-centered diversification where the size of each dot indicates the total number of unique IPC sub-classes. Compared to Variety, the Rao-Stirling describes hetero-centered diversification more clearly. For instance, the Rao-Stirling experienced a greater increase between 2000 and 2001 and no changes between 2008 and 2009. Since Variety can only determine the number of IPC sub-classes used for a patent application, the level of heterogeneity for diversification is underestimated, a characteristic which is highlighted by the Rao-Stirling. In addition, although lesser or no diversification increment was expected in 2008 and 2009, Variety increased while the Rao-Stirling remained the same. This shows that the two representative diversification indices for each diversification perspective not only captures the variation well but also reflects reality in a more reasonable sense.
With these two perspectives of diversification indices, interesting findings can be obtained. If diversification is discussed with a single diversification index, only limited information about diversification is used. For instance, comparing the diversification of applications in each country only tells us the degree to which it either increases or decreases. Two perspectives of diversification clarify how the applicants in each country achieved diversification either in balance or heterogeneity. Figure shows the result of the empirical analysis based on two perspectives of diversification indices with an average of normalized diversification perspective for each five-year time period. Here, the size of each point indicates the average usage of IPC sub-classes per patent application. As diversification is measured with reference to each country, only comparisons of either increases or decreases in values are allowed.
Figure 5. Comparison among countries using two diversification perspectives (High-level patent application group).
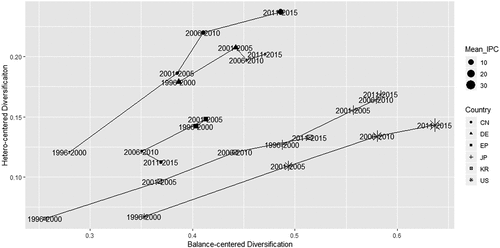
For the high-level patent application group, a clear increase in both diversification perspectives is observed. In the case of DE and EP, slight decreases in 2006–2010 and 2011–2015 are observed as a consequence of the financial crisis. The countries (all except CN) familiar with applying for numerous IPC sub-classes for patent applications tend to move more horizontally, seeking balance-centered diversification. On the other hand, CN’s technology diversification is more focused on hetero-centered diversification. This is a reasonable finding as CN is known to achieve dramatic increases in technological development in a very short period of time. From these findings, the differences in the trajectories of technology diversification regarding the maturity of technological application are observed.
7. Conclusion
7.1. Implications
In this study, the selection of a TDI has been empirically analyzed by covering the most frequently used cases and indices in previous studies. As a result, two diversification perspectives are proposed. It not only proves Stirling’s (Stirling, Citation2007) framework empirically but also provides generalized insights on technology diversification. Overall, the implications of this study for both practitioners and researchers in the field of technology management are as follows.
For academic research, this study provides a reliable resource for selecting TDI. Due to the difference in calculation and characteristic, it is important to select an appropriate diversification index to satisfy the researcher’s intended meaning of diversification and avoid the issue of subjective selection. The two technology diversification indices suggested from this study are already obtained content validity to be implemented in the field of technology management because they were derived through the efforts to generalize the result by adopting different cases and diversification indices. Given the absence of consensus on selecting a proper TDI, this study can be used as a reference for further relevant studies. This study can also be used as a reference for a more systematic mode of index selection, in particular for those interested in the quantitative aspects of science. More importantly, the two diversification perspectives proposed in this study can be used to drive a more extended discussion on technology diversification effect. Previously, technology diversification was only discussed with a single property of diversity. Instead, technology diversification can be discussed either with balance-centered or hetero-centered perspectives. Whether used separately or together, technology diversification measured by Gini-Simpson and Rao-Stirling allows the researchers to consider different diversity properties.
For the practitioners, the two technology diversification perspectives and representative indices are expected to be used for technology development strategy. Referring to the diversification index without an understanding of the diversity property may lead to the faulty decision. By noticing the difference between balance-centered and hetero-centered diversification, the decision makers can evaluate their own level of technology diversification more clearly and establish a more specific direction for their technology.
7.2. Limitations and directions for future research
While this study provides a guideline for selecting TDI, it has a couple of limitations. In this study, technology diversification was measured by patent’s sub-class IPCs. The level of IPC not only indicates the unit and meaning of technology but also affects the sample size and PCA result. Although using sub-class IPC is supported by previous studies, the difference caused by the level of IPC is better to be considered. In addition, technology and industry effect were ignored. The heterogeneity among different technology sectors and industries may lead to an additional discussion on diversification measurement. With relation to the limitation of this study, the additional examination on a selecting TDI with a different level of IPCs is needed. Moreover, a comparison of cases using different technologies and industries can be also discussed. Beside technology diversification, this also can be implemented to other studies or measurements used in the field of management.
Additional information
Funding
Notes on contributors
Keungoui Kim
Keungoui Kim is currently a post-doctoral research fellow at Réseaux Innovation Territoires et Mondialisation, Univ. Paris-Sud. His research interests include technology innovation, diversification strategy, and network analysis.
References
- Abdi, H., & Williams, L. J. (2010). Principal component analysis. Wiley Interdisciplinary Reviews: Computational Statistics, 2(4), 433–18. doi:10.1002/wics.101
- Argyres, N. (1996). Capabilities, technological diversification and divisionalization. Strategic Management Journal, 17(5), 395–410. doi:10.1002/(ISSN)1097-0266.
- Bas, C. L., & Patel, P. (2005). Does Internationalisation of Technology Determine Technological Diversification in Large Firms? An Empirical Study. Revue d’économie Industrielle Does, 110, 157–174. doi:10.3406/rei.2005.3077.
- Berry, C. H. (1971). Corporate growth and diversification. The Journal of Law & Economics, 14(2), 371–383. doi:10.1086/466714.
- Breschi, S., Lissoni, F., & Malerba, F. (2003). Knowledge-relatedness in firm technological diversification. Research Policy, 32(1), 69–87. Retrieved from doi:10.1016/S0048-7333(02)00004-5.
- Chao, Y. S., & Wu, C. J. (2017). Principal component-based weighted indices and a framework to evaluate indices: Results from the medical expenditure panel survey 1996 to 2011. PLoS ONE, 12(9). doi:10.1371/journal.pone.0183997.
- Chiu, Y.-C., Lai, H.-C., Lee, T.-Y., & Liaw, Y.-C. (2008). Technological diversification, complementary assets, and performance. Technological Forecasting and Social Change, 75(6), 875–892. doi:10.1016/j.techfore.2007.07.003.
- Cohendet, P., Llerena, P., & Sorge, A. (1992). Technological diversity and cohérence in Europe : An analytical overview. Revue d’économie Industrielle, 59(1), 9–26. doi:10.3406/rei.1992.1399.
- Fan, F., Li, B., & Yang, Y. (2017). Study of the impact of TMT characteristics on the technology diversification and performance relationship of high-tech enterprise. GSIS 2017. IEEE International Conference on Grey Systems and Intelligent Services (pp. 393–399). Retrieved from https://doi.org/10.1109/GSIS.2017.8077738
- Filmer, D., & Pritchett, L. H. (2001). Estimating wealth effects without expenditure data-or tears: An application to educational enrollments in States of India. Demography, 38(1), 115. doi:10.2307/3088292.
- Gambardella, A., & Torrisi, S. (1998). Does technological convergence imply convergence in markets? Evidence from the electronics industry. Research Policy, 27(5), 445–463. doi:10.1016/S0048-7333(98)00062-6
- Garcia-Vega, M. (2006). Does technological diversification promote innovation?: An empirical analysis for European firms. Research Policy, 35(2), 230–246. doi:10.1016/j.respol.2005.09.006.
- Geringer, J. M., Tallman, S., & Olsen, D. M. (2000). Product and international diversification among Japanese multinational firms. Strategic Management Journal, 21(1), 51–80. doi:10.1002/(SICI)1097-0266(200001)21:1<51::AID-SMJ77>3.0.CO;2-K.
- Godshalk, E. B., & Timothy, D. H. (1988). Factor and principal component analyses as alternatives to index selection. Theoretical and Applied Genetics, 76(3), 352–360. doi:10.1007/BF00265334.
- Hart, P. E. (1971). Entropy and other measures of concentration. Journal of the Royal Statistical Society. Series A (general), 134(1), 73–85. doi:10.2307/2343975
- Hastie, T., Tibshirani, R., & Jerome, F. (2009). The elements of statistical learning: Data mining, inference, and prediction. New York, NY: Springer.
- Heino, J., Mykrä, H., & Kotanen, J. (2008). Weak relationships between landscape characteristics and multiple facets of stream macroinvertebrate biodiversity in a boreal drainage basin. Landscape Ecology, 23(4), 417–426. doi:10.1007/s10980-008-9199-6.
- Jaffe, A. B. (1989). Characterizing the “Technological Position” of firms, with application to quantitative technological opportunity and research spillovers. Research Policy, 18(1), 984–1001. doi:10.1016/0048-7333(89)90007-3.
- Kauffman, S. A. (1992). Self-organization and selection in evolution. In Series on directions in condensed matter physics: origins of order (Vol. 6, pp. 61–100). New York, NY: Oxford University Press.
- Kim, H., Lim, H., & Park, Y. (2009). How should firms carry out technological diversification to improve their performance? An analysis of patenting of Korean firms. Economics of Innovation and New Technology, 18(8), 757–770. doi:10.1080/10438590902793315.
- Kim, J., Lee, C. Y., & Cho, Y. (2016). Technological diversification, core-technology competence, and firm growth. Research Policy, 45(1), 113–124. doi:10.1016/j.respol.2015.07.005.
- Kim, K., Jung, S., & Hwang, J. (2018). Technology convergence capability and firm innovation in the manufacturing sector: An approach based on patent network analysis. R&D Management, 1–12. doi:10.1111/radm.12350.
- Kim, K., Jung, S., Hwang, J., & Hong, A. (2018). A dynamic framework for analyzing technology standardisation using network analysis and game theory. Technology Analysis and Strategic Management, 30(5), 540–555. doi:10.1080/09537325.2017.1340639
- Kim, P. R. (2013). Characteristics of ICT-Based converging technologies. ETRI Journal, 35(6), 1134–1143. doi:10.4218/etrij.13.0113.0043.
- Kook, S. H., Kim, K. H., & Lee, C. (2017). Dynamic technological diversification and its impact on firms’ performance An empirical analysis of Korean IT firms. Sustainability, 9(7). doi:10.3390/su9071239.
- Kruskal, J. B. (1964). Nonmetric multidimensional scaling: A numerical method. Psychometrika, 29(2), 115–129. doi:10.1007/BF02289694.
- Lee, S. U., & Kang, J. (2015). Technological diversification through corporate venture capital investments: Creating various options to strengthen dynamic capabilities. Industry and Innovation, 22(5), 349–374. doi:10.1080/13662716.2015.1054128.
- Leten, B., Belderbos, R., & Van Looy, B. (2007). Technological diversification, coherence, and performance of firms. Journal of Product Innovation Management, 567–579. doi:10.1111/j.1540-5885.2007.00272.x.
- Lin, C., & Chang, C. C. (2015). The effect of technological diversification on organizational performance: An empirical study of S&P 500 manufacturing firms. Technological Forecasting and Social Change, 90(PB), 575–586. doi:10.1016/j.techfore.2014.02.014.
- Macarthur, R. A. (1965). Patterns of species diversity. Biology Reviews, 40, 510–533. doi:10.1111/j.1469-185X.1965.tb00815.x.
- Marfels, C. (1971). Absolute and relative measures of concentration reconsidered. Kyklos, 24(4), 753–766. doi:10.1111/kykl.1971.24.issue-4
- Morris, E. K., Caruso, T., Buscot, F., Fischer, M., Hancock, C., Maier, T. S., … Rillig, M. C. (2014). Choosing and using diversity indices: Insights for ecological applications from the German biodiversity exploratories. Ecology and Evolution, 4(18), 3514–3524. doi:10.1002/ece3.1155
- Nagendra, H. (2002). Opposite trends in response for the Shannon and Simpson indices of landscape diversity. Applied Geography, 22(2), 175–186. doi:10.1016/S0143-6228(02)00002-4
- Pavitt, K. (1988). Uses and Abuses of Patent Statistics. In A. F. J. B. T.-H. of Q. S. of S. and T. VAN RAAN (Ed.), Handbook of Quantitative Studies of Science and Technology (pp. 509–536). Amsterdam: Elsevier. doi:10.1016/B978-0-444-70537-2.50021-0
- Quintana-García, C., & Benavides-Velasco, C. A. (2008). Innovative competence, exploration and exploitation: The influence of technological diversification. Research Policy, 37(3), 492–507. doi:10.1016/j.respol.2007.12.002
- Robins, J., & Wiersema, M. (1995). Multibusiness Firm: Empirical analysis of corporate financial performance. Strategic Management Journal, 16, 277–299. doi:10.1002/smj.4250160403
- Robins, J., & Wiersema, M. (2003). The measurement of corporate portfolio strategy: Analysis of the content validity of related diversification indexes. Strategic Management Journal, 24(1), 39–59. doi:10.1002/smj.282
- Sambharya, R. B. (1995). The Combined Effect of International Diversification and Product Diversification Strategies on the Performance of U.S.-Based Multinational Corporations. MIR: Management International Review, 35(3), 197–218. Retrieved from http://www.jstor.org/stable/40228273
- Saviotti, P. P., & Mani, G. S. (1995). Competition, variety and technological evolution: A replicator dynamics model. Journal of Evolutionary Economics, 5(4), 369–392. doi:10.1007/BF01194367
- Schmoch, U. (1999). Patent Indicators. Research Evaluation, 8(2), 119–131. doi:10.3152/147154499781777540
- Shannon, C. E. (1948). A Mathematical Theory of Communication. Bell System Technical Journal, 27(April 1924), 623–656. doi:10.1002/j.1538-7305.1948.tb00917.x
- Singh, D. A., Gaur, A. S., & Schmid, F. P. (2010). Corporate diversification, TMT experience, and performance: Evidence from German SMEs. Management International Review, 50(1), 35–56. doi:10.1007/s11575-009-0025-4
- Solow, A., Polasky, S., & Broadus, J. (1993). On the Measurement of Biological Diversity. Journal of Environmental Economics and Management. doi:10.1016/S0003-9861(71)80064-4
- Stephan, M. (2002). An analysis of the relationship between product diversification, geographical diversification, and technological diversification. Discussion Paper on International Management and Innovation, No. 02-02. Stuttgart: Hohenheim University.
- Stirling, A. (1998). On the economics and analysis of diversity. Science Policy Research Unit (SPRU), Electronic Working Papers Series, 141.
- Stirling, A. (2007). A general framework for analysing diversity in science, technology and society. Journal of the Royal Society Interface, 4(15), 707–719. doi:10.1098/rsif.2007.0213
- Stirling, G., & Wilsey, B. (2001). Empirical Relationships between Species Richness, Evenness, and Proportional Diversity. The American Naturalist, 158(3), 286–299. doi:10.1086/321317
- Vyas, S., & Kumaranayake, L. (2006). Constructing socio-economic status indices: How to use principal components analysis. Health Policy and Planning, 21(6), 459–468. doi:10.1093/heapol/czl029
- Wang, Y., Ning, L., & Prevezer, M. (2015). Technological diversification in China from 1986 to 2011: Evidence from patent data. Technological Forecasting and Social Change, 99, 54–66. doi:10.1016/j.techfore.2015.06.019
- Whittaker, R. H. (1972). Evolution and measurement of species diversity. Taxon, 21(2), 213–251. doi:10.2307/1218190
- Wilsey, B. J., Chalcraft, D. R., Bowles, C. M., & Willig, M. R. (2005). Relationships among indices suggest that richness is an incomplete surrogate for grassland biodiversity. Ecology, 86(5), 1178–1184. doi:10.1890/04-0394
- Woerheide, W., & Persson, D. (1992). An index of portfolio diversification. Financial Services Review, 2(2), 73–85. doi:10.1016/1057-0810(92)90003-U
Appendix
Descriptive statistics of case 1 (High-level patent application group)
Descriptive statistics of case 1 (Mid-level patent application group)
Descriptive statistics of case 1 (Low-level patent application group)
Descriptive statistics of case 2 (High-level patent application group)
Descriptive statistics of case 2 (Mid-level patent application group)
Descriptive statistics of case 2 (Low-level patent application group)