Abstract
The purpose of the study was to examine the determinant factors that influence the intention to revisit retailer omnichannel in Indonesia with Mean-End Chain Approach. The internet economy growth drives the use of the omnichannel strategy in Indonesia retail companies. The past research showed that there was inconsistency in the understanding of omnichannel strategy channel integration. The Means-End Chain Theory believes can help to enlighten this integration by understanding the attribute, consequences, and values of customer omnichannel strategy acceptances. The research is cross-sectional quantitative research with sample of 248 respondents that gathered using convenience sampling. The Disjoint Two-Stage Approach Partial Least Square Structural Equation Model (PLS-SEM) was used to analyse the high order construct model. The finding shows that both customer satisfaction and shopping value were the mediating variables between channel integration and intention to revisit. The integrated order fulfilment, integrated transaction information, integrated customer service, and integrated customer service influenced channel integration were identified as the dominant dimensions to the channel integration. The managerial implication emphasized on the various channel integration optimization and integration during pre and post customer shopping journey. This study enriches the customer behavior studies, especially in the importance of channel integration with Mean-End Chain Theory approach.
1. Introduction
Customer experience can be developed through the channel where it can be used to raise awareness among customers of the product and services, it helps customers evaluate a company value proposition, it allows customer to purchase and get support but also allows for a company to deliver it value proposition. As consumer behavior is changing, proliferation of digital technology, and highly competitive markets drive the emerge of omnichannel strategies. Indonesia’s internet economy is expected to reach $146 billion by 2025 (SEADS, Citation2021), with predominantly market based in Java (Statistik, Citation2022). Therefore, understanding online customer shopping is important especially their decision-making process that allow marketer to tailor their marketing strategies (Grewal & Roggeveen, Citation2020).
Omnichannel shopping integrates channels, such as physical stores, e-commerce platforms, social media, and mobile apps to ensure a consistent purchasing experience (Lee et al., Citation2019). Consumer shopping behavior from omnichannel is very diverse. Retailers must enhance digital platforms (Leão & da Silva, Citation2021), incorporate user-generated content (Khattak et al., Citation2022), and provide customer protection to achieve success (Limanseto, Citation2022). In Indonesia, the implementation of omnichannel marketing is gaining traction during COVID-19 pandemic because the customer has reduced their mobility for shopping (Cai et al., Citation2023; Sugiat et al., Citation2023). Omnichannel consumers spend 4% more time in physical stores and 10% in online stores compared to consumers who only interact with brands through one channel (Sirclo, Citation2020). Therefore, the large-scale business increases their investment in omnichannel marketing infrastructure by integrating their online and offline channel (Saghiri & Mirzabeiki, Citation2021), security technology (Ameen et al., Citation2020), augmented reality technology (Utomo et al., Citation2023), and increasing customer awareness for seamless customer experience in their online shopping journey (Lazaris et al., Citation2021).
Omnichannel integration can be said to be O2O (Offline to Online or Online to Offline) which is the basic concept of omnichannel that pays attention to the convenience, convenience, and shopping experience of consumers and removes the boundaries between offline and online (Lazaris et al., Citation2021). The omnichannel strategy in Indonesia began to be recognized in 2018 and the implementation of the omnichannel system is still relatively small due to the lack of knowledge and ability to manage omnichannel. Good omnichannel implementation needs to be based on a dynamic consumer needs orientation. The challenges faced by management are knowing when and the right way to communicate to consumers, how to take advantage of store services and guarantees both offline and online for a smooth consumer shopping experience, to consider the best way to maintain long-term relationships with consumers (Villanova et al., Citation2021). In addition, the effects of the COVID-19 pandemic also seem to be lingering and may signal a ‘new normal’, including a less complicated needs assessment phase that focuses on fewer needs, such as those related to product safety and functionality, reliability, pricing, availability via home delivery or pick up (Roggeveen & Sethuraman, Citation2020).
The previous research attempt to examine the channel integration using unidimensional approach (Cotarelo et al., Citation2021) and multidimensional approach (Gao et al., Citation2021; Lee et al., Citation2019; Zhang et al., Citation2018). The implementation of click and collect system in Spain that integrate their product price information and price, promotion offering, variety of product, and channel switching are positively affecting the shopping value and customer satisfaction (Cotarelo et al., Citation2021). However, the customer engagement was positively affected by omnichannel integration dimensions (range of channel service option, standardization of content, operation alignment, and transparency of channel setup) (Lee et al., Citation2019). omnichannel integration dimensions (integrated order fulfilment, integrated customer service, integrated product & price, and integrated information access) were positively affect customer empowerment of omni-channel retailing in China (Zhang et al., Citation2018). However, omnichannel integration dimension (integrated transaction information, integrated order fulfilment, integrated promotion, integrated product and price, and integrated information access) positively affect cognitive customer experience of omni-channel retailing in China (Gao et al., Citation2021). The researchers determined the inconsistency of channel integration in omnichannel setting. Therefore, the researchers consider channel integration as multidimensional variable with omnichannel retail setting in Indonesia.
The previous studies on omnichannel are limited since the previous researchers don not consider the omnichannel in new normal context. The impact of COVID-19 pandemic has changed consumer behaviour in seeking information and shopping. Previous study has concentrated on the influence of omnichannel integration and shopping value on the customer experience in Pakistan fashion retail (Ahmed & Syed, Citation2021; Riaz et al., Citation2021). However, another previous study found that omnichannel shopping value positively affect customer satisfaction in the retail context in Spain. Moreover, those studied did not consider the effect of customer experience on the continuance usage intention (Lee et al., Citation2019). The researchers concerned that research is testing channel integration relationships, shopping value, and satisfaction in omnichannel setting still limited. Therefore, it is important to examine the mediating effect of shopping value and customer satisfaction between omnichannel integration and revisit intention.
This research will focus on Ace Hardware consumers as research subjects because the company has implemented an omnichannel strategy through Ruparupa. The research provides views and suggestions for Ace Hardware and companies in similar industries regarding what characteristics must be considered when implementing an omnichannel strategy. The urgency of this research is how an integrated customer experience study can provide input to the management team by measuring and predicting the behavior of omnichannel customers through physical stores and online channels.
2. Literature review
2.1. Means-end chain theory
The current study deals with omnichannel integration, shopping value, customer satisfaction, and behavioral intention in omnichannel retailing. ‘Means-end theory’ is the leading theory that can explain the relationship between quality, value, and behavior and the fundamental relationship between consumers and products/services (Olson & Reynolds, Citation1983). The means-end chain theory can explore the motivational and informational aspects underlying product evaluation, which can be considered pivotal points for more complicated post-purchase evaluations (Gardial et al., Citation1994). Previous literature suggests that the central thesis of means-end theory is that people are goal-oriented and use various product attributes to assume goals or outcomes (Gutman, Citation1982). More specifically, previous studies have shown that customers evaluate the satisfaction of a complex retail omnichannel experience from the perspective of the shopping value dimension in the consumer goods category (Rahman, Carlson, Gudergan, et al., Citation2022). Shopping value is a highly desirable goal, evaluation of the shopping experience on omnichannel can be received positively by customers. Research using the means-end chain theory has increased quite sharply over the last two decades, and this theory can explain value as one of the leading causes of repurchase intention (Borgardt, Citation2020) Therefore, means-end is the suitable method to categorize the basic pattern of relationships with product or service quality that can increase customer shopping value.
Previous research used a means-end chain theory to explain the relationship between quality–value–behavior in the context of a water park (Jin et al., Citation2015). The results showed a positive relationship between the quality of experience, image, perceived value, satisfaction, and behavioral intention. In addition, Xiao, Guo, et al. (Citation2019) use a means-end chain theory approach to understand customer segments in online and offline commerce so that management gains insight from consumer behavior. The results of this study present the influence of company profiles and network externalities on the level of trust in customer groups that are vulnerable to risk and the influence of product variations on optimal choices in customer groups that are sensitive to returns using a qualitative approach.
Develop a model from means-end chain theory that only focuses on value and consequence to understand the desire to buy back with a structural equation modeling approach Kim et al. (Citation2020). Moreover, Other researchers use means-end chain theory to explain online-based student service experiences to answer the complexity of user demand for service excellence (Liang et al., Citation2022). These findings prove that the student service experience instrument can measure student responsibility towards university service performance socially and constructively.
The mean-end chain theory application for explaining the customer satisfaction through shopping value in e-commerce has been conducted in Japan (Moriuchi & Takahashi, Citation2023), India (Kumar & Ayodeji, Citation2021), and Taiwan (Huang & Hung, Citation2023) in the context of C2C e-commerce setting, the previous research utilized the means-end chain theory to explore the relationship between perceived value and satisfaction using quantitative approach (Moriuchi & Takahashi, Citation2023). The findings show that customer trust’s impact on satisfaction varies based on perceived functional and emotional. The previous research attempts to apply the means-end chain theory to examine the customer activation and retention in Indian e-retailers setting (Kumar & Ayodeji, Citation2021). The findings show that e-retailer success (system quality, information quality, and service quality) influenced the customer satisfaction through utilitarian and hedonistic values. Another study investigated the effect of group intention and shopping value in the context of online shopping day using means-end chain theory (Huang & Hung, Citation2023). The study found that major type of shopping value, group norm, social identity, and anticipated emotion increased we-intention, consumers’ desire, and participation behavior.
Finally, the means-end chain theory has been applied for investigating the customer experience in omnichannel retailing using qualitative approach (Rahman, Carlson, et al., Citation2022) and mixed method (Rahman, Carlson, Gudergan, et al., Citation2022) approach. Using laddering technique, the researchers attempt to examine the safety factors in omnichannel shopping as perceived by customers and their impact on customer experience evaluation in post-pandemic setting (Rahman, Carlson, et al., Citation2022). This study has identified fourteen safety aspects in omnichannel shopping and has proposed a new paradigm for safety and security in post-pandemic retail service contexts. Contradictory, the researchers attempt to propose comprehensive measurement for omnichannel customers experience by incorporating means-end chain theory using mixed method approach (Rahman, Carlson, Gudergan, et al., Citation2022). The findings show that the proposed measurement model empirically confirm and predict the customer behavior in the consumer goods omnichannel retail setting. The measurement models consist of shopping value, social communication, personalization, product availability, prices across channel, delivery, product return, and loyalty programs.
Although means-end chain theory is a prominent theory for understanding and predicting consumer behavior in various industries, there are challenges in measuring the value of the means-end chair to analyze diverse consumer behavior. Therefore, we position channel integration as an attribute of shopping value and customer satisfaction that drives revisit intentions. Ultimately, the value of MEC theory provides the principles and direction underlying this research.
2.2. Omnichannel and customer journey
Omnichannel customer journeys in the retail industry refer to the seamless, integrated experiences that customers have across multiple channels when interacting with retailers (Hickman et al., Citation2020). This approach recognizes that customers can engage with brands through multiple touchpoints, such as physical stores, websites, mobile apps, and social media platforms, live streaming channels, and digital kiosks (Roggeveen & Sethuraman, Citation2020). The goal of an omnichannel strategy is to deliver a consistent and personalized experience throughout the customer journey across multiple channels (Lehrer & Trenz, Citation2022). This approach goes beyond traditional multichannel or cross-channel approaches by emphasizing the importance of channel integration and synchronization to create a cohesive and satisfying experience for customers (Gao et al., Citation2021).
Retailers implementing effective omnichannel customer journeys will get the benefits. First, the omnichannel concept leads to a better customer experience by eliminating friction points and providing seamless transitions between channels (Rahman, Carlson, Gudergan, et al., Citation2022). The omnichannel concept allows customers to switch between online and offline channels with ease, increasing convenience and accessibility. Second, omnichannel strategies increase customer engagement by providing personalized recommendations, offers, and content based on individual preferences and previous interactions (Mosquera et al., Citation2017). The personalized approach fosters stronger relationships with customers and encourages them to be honest with the brand. Lastly, a well-executed omnichannel strategy can result in higher sales and revenue for retailers (Lehrer & Trenz, Citation2022). By providing a consistent experience across channels, retailers can capture customer attention, inspire purchases, and even drive cross-channel sales as customers seamlessly move from one touchpoint to another (Kopot & Cude, Citation2021).
The implementation of omnichannel customer journeys in the retail industry has its own challenges. Retailers need to overcome barriers, such as data integration and infrastructure, ensuring that customer data is accurately synchronized across channels (Grewal & Roggeveen, Citation2020). Organizational alignment is another important factor, as different departments within a company must work together to provide a consistent experience (Kopot & Cude, Citation2021). Retailers must invest in technology and communications to communicate and share data barriers so as to maintain consistency and personalization of increasingly complex channels (Thaichon et al., Citation2023). Retailers must address talent and skills gaps within their organizations to execute omnichannel strategies effectively. Therefore, addressing these challenges is critical for retailers to deliver a unified and exceptional customer experience across all touchpoints and maximize the benefits of the omnichannel customer journey.
2.3. Channel integration
Organizations will gain a competitive advantage over their competitors if they successfully integrate various channels (Rusanen, Citation2018). Channel integration can be defined as the extent to which different channels interact with each other. Physical channels facilitate digital information sharing through offline-online integration (Xiao, Guo, et al., Citation2019). The urgency of channel integration has been studied by previous researchers (Gao et al., Citation2021; Lee et al., Citation2019; Zhang et al., Citation2018). Previous research has examined the impact of channel integration on customer experience (Gao et al., Citation2021), customer interaction (Lee et al., Citation2019), shopping value (Cotarelo et al., Citation2021), customer satisfaction (Lazaris et al., Citation2021), and customer empowerment (Zhang et al., Citation2018). Researchers concluded that the importance of conducting research that examines channel integration in the context of omnichannel retailing needs to be revisited. Omnichannel retailing requires the integration of customer touchpoints for a seamless customer experience resulting in sales transactions.
Integrated product and price are the integration of product information and pricing on physical stores, websites, mobile applications, and other platforms (Zhang et al., Citation2018). Customers can know consistent product information, stock, and pricing so that customers can choose the most convenient channel and according to their preferences. While integrated information access is an integration of product description and stock information, product videos, customer reviews, and recommendations from various sales channels (Gao et al., Citation2021). Such information can help customers in taking better buying decisions based on a comprehensive understanding of the products of interest. Integrated promotion is the integration of promotions and special offers through various sales channels (Lee et al., Citation2019). This provides an opportunity for customers to take advantage of relevant offers and increase the value of their shopping.
Integrated order fulfillment is the integration of the process of ordering, payment, and picking up products through physical stores, courier delivery, or delivery through third parties (Ryu et al., Citation2023). Customers can access up-to-date shipping information and track order status through various channels so that orders can be received on time. Integrated transaction information is the integration and ease of accessing customer transaction history through physical stores and online platforms (Wang & Jiang, Citation2022). This can help the system to provide product recommendations that are in accordance with customer transaction behavior. Integrated customer service is the integration of customer service through various channels consistently (Saghiri & Mirzabeiki, Citation2021). Customers can contact the service support team via phone, email, live chat, or Instagram to get solutions to problems encountered during the shopping trip.
In this study, researchers adopted the channel integration dimension of research conducted by Zhang et al. (Citation2018). The results of testing with a second-order formative construct approach show that only integrated product and price, integrated information access, integrated order fulfillment, and integrated customer service are statistically proven to have a significant effect on channel integration (Zhang et al., Citation2018). Although integrated promotion and integrated transaction information proved to have no effect on previous research (Zhang et al., Citation2018). However, integrated promotion and integrated transaction information affect customer satisfaction when tested using the first order construct approach (Cattapan & Pongsakornrungsilp, Citation2022). Researchers observe inconsistencies in approaches in assessing channel integration separately and collectively. The six dimensions are conceptually different from each other to understand channel integration, but researchers believe that they all explain the customer journey in the context of omnichannel retailing.
2.4. Hypothesis development
2.4.1. Channel integration and customer satisfaction
Previous research has established omnichannel integration positively affect customer satisfaction (Cattapan & Pongsakornrungsilp, Citation2022; Gao et al., Citation2021; Lazaris et al., Citation2021; Wang & Jiang, Citation2022; Zhang et al., Citation2018; Zhu et al., Citation2022). Traditional retail models often involve fragmented experiences, such as inconsistent pricing, intermittent inventory management, and limited product availability across channels (Mukherjee & Wood, Citation2021). By implementing omnichannel integration, retailers can address these challenges and provide customers with a unified and cohesive experience (Lim et al., Citation2022). Customers expect a seamless shopping experience that allows them to interact with retailers through multiple channels, such as physical stores, online platforms, and mobile apps (Riaz et al., Citation2021; Zhang et al., Citation2018). Customers have easy access to products and services using click-and-collect, in-store returns for online purchases, and more personalized real-time inventory visibility and other features so they’ll feel comfortable and satisfied (Cattapan & Pongsakornrungsilp, Citation2022; Saghiri & Mirzabeiki, Citation2021). Consumers will be more satisfied if they are given wider control with the implementation of omnichannel strategies when they want to shop through online and offline channels (Wang & Jiang, Citation2022; Zhang et al., Citation2018). Therefore, the researcher formulated the hypothesis statement as follows:
H1:
Channel integration positively affect customer satisfaction.
2.4.2. Channel integration and shopping value
Previous research has proven that omnichannel integration can have a positive influence on shopping value (Asare et al., Citation2022; Cotarelo et al., Citation2021; Gao et al., Citation2021; Wang & Jiang, Citation2022). With the ability to collect and analyze customer data from online and offline channels, retailers can gain valuable insights into channel preferences for evaluating products, shopping, shipping, after-sales service (Cotarelo et al., Citation2021; Gunawan et al., Citation2023). Armed with this information, retailers can provide personalized recommendations through online channels, compile promotional catalogs on offline channels, develop product knowledge materials digitally, improve product knowledge from salesmen, evaluate payment and delivery performance and identify opportunities, and provide more appropriate solutions when customers need aftersales assistance (Gao et al., Citation2021; Timoumi et al., Citation2022). By providing relevant and meaningful interactions, retailers enhance customers’ perceptions of value, making them feel understood and valued as individuals (Chang & Geng, Citation2022). Therefore, perceived shopping value is a crucial factor influencing consumers’ purchasing decisions, as it encompasses the benefits customers perceive in relation to the costs, time, and effort incurred (Asare et al., Citation2022; Wang & Jiang, Citation2022). Therefore, the researcher formulated the hypothesis statement as follows:
H2:
Channel integration positively affects shopping value.
2.4.3. Customer satisfaction and intention to revisit
Previous research has examined that shopping value positively affects the intention to revisit an omnichannel retail platform (Cattapan & Pongsakornrungsilp, Citation2022; Chang & Geng, Citation2022; Gao et al., Citation2021). Customers will get more value if they buy the right product at a reasonable price when shopping through retailers that have implemented omnichannel (Rahman, Carlson, Gudergan, et al., Citation2022). Customers can take advantage of the online and offline channels to obtain information, compare prices, order, and payment, choose the appropriate shipping method or in-store pick up, and get after-sales service (Gao et al., Citation2021). Retailers should concentrate on providing high quality product, cost saving, and convenience to the customer during online shopping in online and offline channel (Cattapan & Pongsakornrungsilp, Citation2022). In addition, retailers who have developed technology that facilitates both retailer-customer and customer-customer relationships will improve accuracy in data-driven decision making (Tripathi et al., Citation2021). Retailers can determine online discounts, omnichannel promotions, purchase online, return in-store, and other methods to guarantee product quality based on channel characteristic for improving accessibility, searchability, and convenience (Cattapan & Pongsakornrungsilp, Citation2022). Customers who perceive a higher shopping value from their previous omnichannel shopping experiences tend to show higher omnichannel revisit intention (Chang & Geng, Citation2022). Therefore, the researcher formulated the hypothesis statement as follows:
H3:
Customer satisfaction positively affects intention to revisit.
2.4.4. Shopping value and intention to revisit
Previous research investigated that shopping value positively affects the intention to revisit an omnichannel retail environment (Chang & Geng, Citation2022; Pereira et al., Citation2023; Rahman, Carlson, Gudergan, et al., Citation2022; Song & Jo, Citation2023). Shopping value can be defined as the benefits and advantages that consumers feel during their shopping journey across various channels and touchpoints offered by retailers (Cotarelo et al., Citation2021; Huré et al., Citation2017). Shopping value is one of the most important dimensions of customer satisfaction and loyalty (Rahman, Carlson, Gudergan, et al., Citation2022). During the purchase planning phase, retailer must provide adequate information through omnichannel channel and benefits to encourage customer to purchase their products (Chang & Geng, Citation2022). Therefore, customers feel the convenience, personalization, and efficiency positively affect their intention to revisit the omnichannel retail environment (Ameen et al., Citation2020) The higher shopping value during shopping journey will increase the intention to revisit for next purchase (Blom et al., Citation2021). Therefore, the researcher formulated the hypothesis statement as follows:
H4:
Shopping value positively affects intention to revisit.
2.4.5. Mediation effect of shopping value and customer satisfaction
When the customers feel the omnichannel integration fulfills their needs during online shopping, they will perceive higher shopping value in the omnichannel environment and likely feel satisfied with their interaction (Cotarelo et al., Citation2021). Consequently, this heightened satisfaction and perceived shopping value is expected to positively influence their intention to revisit the retailer who adopts omnichannel strategy. Customers who have a purpose-based shopping orientation will feel more satisfied if retailers who have implemented integrated omnichannel (Blom et al., Citation2021). Management synergy in utilizing technology to integrate online channels and physical stores to ensure customers get an optimal shopping experience (Lazaris et al., Citation2021). Retailer who already adopt omnichannel encourage their customer to empower the strength of online and offline channel for fulfilling their daily needs (Pereira et al., Citation2023). Understanding the mediating effect of customer satisfaction and shopping value between channel integration and intention to revisit will provide valuable insights for retailer. Therefore, the researcher formulated the hypothesis statement as follows:
H5:
Shopping value positively mediate between channel integration and intention to revisit.
H6:
Customer satisfaction positively mediates between channel integration and intention to revisit.
shows the proposed conceptual model. The authors proposed the model based on the hypothesis development stated in the literature review section. Channel integration is an exogenous variable, shopping value, and customer satisfaction are mediating variables, and intention to revisit is endogenous variable.
3. Research methods
The research design used a quantitative method with cross-sectional study. The data collection method is using an online questionnaire. The researchers used partial least squares structural equation modeling (PLS-SEM) for data analysis because the research model is complex (Lim et al., Citation2022). PLS-SEM was primarily used to develop theories in exploratory research. This was done by focusing on explaining the variables in the model (Hair et al., Citation2017). The data analysis software used SmartPLS 4.0.8.5 (Lim et al., Citation2022).
The researchers cannot determine the population size due to limited access on number of customers of ACE hardware. The sample size determination used ten times the number of indicators and our research employ 23 indicators. Therefore, the sample size was a minimum of 230 (Ali Memon et al., Citation2020). The sampling method is using convenient sampling. Researchers distributed the questionnaire through social media (Instagram, Twitter, and Facebook) and Online Messaging Applications (Line, WhatsApp, and Telegram) to circles of friends, family, communities, and some relatives. The researchers gathered 248 sample within 2 months. The criteria are ACE hardware customer who purchased product through online or offline channels.
The researchers collect the data by using online questionnaire through Google Form. The questionnaire item measured using 6-point Likert scale (1 is strongly disagree and 6 is strongly agree. The questionnaire contained two parts, the first part comprised seven items to gather demographic and psychographic profile of respondent who used omnichannel in retail company. The indicators of variables were adapted from previous study. Moreover, the second part consist of the variables in the study, such as integrated information access (Cattapan & Pongsakornrungsilp, Citation2022), integrated order fulfillment (Cattapan & Pongsakornrungsilp, Citation2022), integrated promotion (Gao et al., Citation2021), integrated product and price information (Gao et al., Citation2021), integrated transaction information (Gao et al., Citation2021), shopping value (Cotarelo et al., Citation2021), customer satisfaction (Cotarelo et al., Citation2021), and intention to revisit (Lazaris et al., Citation2021). Finally, the researchers written the consent at the beginning of the questionnaire by stating the research objective, researchers identity, privacy and data security storage protocol, respondents right, data usage, and approval request.
The researchers proposed the conceptual model using a reflective-formative model (Sarstedt et al., Citation2019). Therefore, the data will be assessed using individual indicator reliability, internal consistency reliability, convergent validity analysis, and discriminant validity in the first stage of data analysis (Sarstedt, Hair, Pick, et al., Citation2022). After completing the lower order construct analysis, the researchers perform the higher order formative construct analysis by examining the measurement model and structural model analysis (Sarstedt, Hair, Pick, et al., Citation2022). Finally, the researchers ran the PLS-Predict to assess the predictive power based on the proposed model (Shmueli et al., Citation2019). The detailed protocol will be explained in the results and discussions.
4. Results
is the result of descriptive data processing of respondent profiles. Based on the results of data processing, most respondents are female, aged 20–39 years, the last education is undergraduate, works as a student/student and private employee, visits physical or online stores 1–2 times in the last 3 months, and physical stores are a favourite channel to find and buy products.
Table 1. Descriptive analysis of respondent profile.
4.1. Non-response common method bias
Researchers used the same respondent and self-reported questionnaire approach in distributing the questionnaire. Therefore, there is a potential common method bias that needs to be considered (Hsia et al., Citation2020; Podsakoff & Organ, Citation1986). By employing the CMB approach, Researchers need to ensure the accuracy and reliability of research results by ensuring robustness. The researchers employed the full collinearity test using Smart PLS and used cut-offs value lower than 3.3 (Kock, Citation2015; Kock & Lynn, Citation2012; Yao et al., Citation2023). The researchers create dummy variable and regress all variables with dummy variable. presents the full collinearity test results. The inner VIF results show between 1.224 and 1.382. Hence, common method bias was not a significant problem that could affect the results.
Table 2. Full collinearity estimates.
The disjoint two-stage approach is a method to analyse higher-order construct on structural equation modelling (Sarstedt et al., Citation2019). This approach is useful for studying variable constructs consisting of several dimensions. Higher order constructs describe broader concepts or latent variables whereas lower order dimensions describe important aspects of constructs. Moreover, reduction of measurement error, and conceptual clarity (Utomo & Kurniasari, Citation2023). The first stage is to test the reflective measurement model, researchers perform individual indicator reliability, internal consistency reliability, convergent validity analysis, and discriminant validity. First, researchers perform individual reliability indicators using cut-off values for a loading factor of at least 0.5 (Bagozzi & Yi, Citation2012). This criterion is often used in complex models and can be used with the condition that the AVE value is at least 0.5 although it generally uses a cut-off value of 0.7 (Sarstedt, Hair, Pick, et al., Citation2022). Second, researchers use a cut-off value composite reliability of at least 0.7 to test internal consistency reliability (Hair et al., Citation2019; Sarstedt, Hair, Pick, et al., Citation2022). Third, in convergent validity testing, researchers use cut-off value average variance extracted at least 0.5 (Bagozzi & Yi, Citation2012; Hair et al., Citation2019; Sarstedt, Hair, Pick, et al., Citation2022). presents the results of convergent validity, internal consistency reliability. The results of individual indicator reliability testing show that the loading factor value for all indicators meets predetermined criteria and is categorized as acceptable. The results of internal consistency reliability testing show that the composite reliability value has met the criteria and is categorized as acceptable. The results of convergent validity testing show that the AVE value has met the criteria and is categorized as acceptable.
Table 3. Convergent validity result.
Furthermore, researchers conducted a discriminant validity analysis using HTMT criterion. The use of HTMT criterion was introduced in 2015 and various studies confirm its reliability (Henseler et al., Citation2015; Sarstedt, Hair, Pick, et al., Citation2022). presents discriminant validity values using the HTMT criterion. Researchers used cut-off values of HTMT of 0.9 and 0.85 to determine variable similarities and differences (Hair et al., Citation2021; Sarstedt, Hair, Pick, et al., Citation2022). In this study, customer satisfaction, integrated customer service, integrated information access, integrated order fulfillment, integrated product and price information, integrated promotion, integrated transaction information, intention to revisit, shopping value. As a result, the study’s model has no problems with discriminant validity show results that match the criteria.
Table 4. Discriminant validity results—HTMT criterion.
After testing the validity and reliability of the lower-order reflective construct measurement model is completed, the researcher stores latent variable scores from the variables integrated customer service, integrated information access, integrated order fulfillment, integrated product and price information, integrated promotion, integrated transaction information for use in the second stage of the disjoint two-stage approach. The second stage examines second-order formative constructs to develop measurement models and structural model analysis (Sarstedt, Hair, Pick, et al., Citation2022). Researchers evaluated the variance inflation factor (VIF) value to identify the phenomenon of multicollinearity among predictor variables with a cut-off value of VIF below 3.3 (Sarstedt, Hair, et al., Citation2022). The VIF value in this study is below 2, indicating that the outcome is so accurate that the symptoms of multicollinearity are not visible. Next, researchers evaluated outer weight, t-statistic, p-value, and outer loading to explain the importance of relevant indicators in the formative model using bootstrapping techniques (Hair et al., Citation2021). It can be concluded that channel integration is influenced by integrated order fulfillment, integrated transaction information, integrated customer service, and integrated information access. Integrated promotion and integrated product and price information do not affect channel integration.
To evaluate the structural model assessment, researchers conduct hypothesis testing, coefficient of determination (R2), dan predictive power. shows the structural model assessment. The bootstrapping procedure is as follows: subsample is 5.000, confidence interval method is Bias-corrected and accelerated (Bca) bootstrap, test type: one tailed, significance level: 0.025. The f2 statistic measures the contribution of exogenous factors to endogenous variables, and the intervals are as follows: high effect (f2 > 0.350), medium effect (f2 > 0.150), and small effect (f2 > 0.020) (Cohen, Citation1988). The researchers also used a combination of criteria, such as p-values and effect sizes to better understand the findings (Uzir et al., Citation2021). shows the hypothesis testing result and there are four of five supported hypotheses. Shopping value (β = 0.291; p-value < 0.001; small effect; BCI LL = 0.165; BCI UL = 0.394) and customer satisfaction (β = 0.299; p-value < 0.001; small effect; BCI LL = 0.174; BCI UL = 0.405) positively affect intention to revisit. Channel integration positively affect customer satisfaction (β = 0.484; p-value < 0.001; moderate effect; BCI LL = 0.331; BCI UL = 0.593) and shopping value (β = 0.481; p-value < 0.001; moderate effect; BCI LL = 0.351; BCI UL = 0.566). Shopping values were not found to be significant for customer satisfaction (β = 0.016; p-value = 0.408; BCI LL = −0.101; BCI UL = 0.156).
Table 5. Outcome of formative measurement model.
Table 6. Hypothesis test result.
In the next stage, the researchers introduced the control variables and checked the structural model results again (see ). The researchers used gender, occupation, and age group as control variable. The results were almost similar, the Δ of β value between H3 (CS-IR) without control variable and H3 with control variable is −0.030 and the Δ of β value between H4 without control variable and H4 with control variable is 0.006. The relationship of gender (β = 0.082; p-value = 0.249; BCI LL = −0.101; BCI UL = 0.156), occupation (β = 0.034; p-value = 0.318; BCI LL = −0.098; BCI UL = 0.172), education (β = −0.071; p-value = 0.118; BCI LL = −0.184; BCI UL = 0.043), and age group (β = −0.124; p = value = 0.064; BCI LL = −0.277; BCI UL = 0.0.29) on intention to revisit is not significant.
Furthermore, the beta value of gender is 0.082 and the researchers concluded that the results are supportive for woman rather than male. Similarly, the beta value of occupation is 0.034 and the researchers conclude that the characteristic of occupation shows a different effect on intention to revisit omnichannel. Contradictory, the beta value of education is −0.071 and the researchers conclude that the higher education background means the intention to revisit omnichannel increased. Finally, the beta value of age group is −0.124 and the researchers concluded that different age group have different preferences for intention to revisit.
Moreover, this study also tested the mediation effect of customer satisfaction and shopping value between channel integration and intention to revisit. The researchers examine the significance of indirect effect and the Bca bootstrap to assess the mediation effect (Hair et al., Citation2021). The significance of indirect effect cut-off value at t-values > 1.645 and p-value < 0.025. illustrates that customer satisfaction mediated the effect of channel integration on intention to revisit (β = 0.145; p < 0.001; BCI LL = 0.075; BCI UL = 0.216) and shopping value mediated the effect of channel integration on intention to revisit (β = 0.140; p < 0.001; BCI LL = 0.069; BCI UL = 0.208). Contrary to the researcher’s expectation, shopping value and customer satisfaction did not mediate the effect of channel integration on intention to revisit (β = 0.002; p = 0.415; BCI LL = −0.015; BCI UL = 0.026). the researchers adopt the Preacher and Hayes approach for mediation effect test by bootstrapping the indirect effect (Preacher & Hayes, Citation2008).
Table 7. Hypothesis testing of indirect effect.
This study used three recommended levels to evaluate coefficient of determination (R2) value; 0.75 considered as substantial predictive power, 0.5 as moderate, and 0.25 as weak (Hair et al., Citation2017). R2 value of customer satisfaction is 0.242, shopping value is 0.231, and intention to revisit is 0.217. therefore, all constructs modeled explain 21.7% of revisit intention and can be considered weak. The final step in the structural model assessment is assessing the predictive power using PLS-Predict (Shmueli et al., Citation2019). This method is determined using 10-folds with ten repetitions as the setting. To demonstrate good predictive potential, the PLS mean absolute error (MAE) value should be lower than the LM (linear regression model) MAE. If all of indicators of PLS_MAE < LM_MAE, then the model has high predictive power; if most indicators of PLS_MAE < LM_MAE, then the model has moderate predictive power; if the minority of indicators of PLS_MAE < LM_MAE, then the model has low predictive power. presents the PLS predict result. The researchers identify that IRO1 and IRO3 have PLS_MAE < LM_MAE then the model has moderate predictive power.
Table 8. PLS predict result.
5. Discussions
Our study attempted to examine the determinant factors affecting the revisit intention of retailer that implement omnichannel strategy. This study employed Means-End Chain Theory as the main theory for understanding the revisit intention. the researchers position the omnichannel integration as an attribute of shopping value and customer satisfaction that drives revisit intentions.
Theoretically, the research framework proposed in this research was adapted from Means-End Chain Theory developed by Gutman (Citation1982). Based on the literature review findings, the researchers managed to identify various studies that applied MEC using qualitative or quantitative approach in water park (Jin et al., Citation2015), e-commerce (Huang & Hung, Citation2023; Kumar & Ayodeji, Citation2021; Moriuchi & Takahashi, Citation2023), online student service centre (Liang et al., Citation2022), and omnichannel retailing (Kim et al., Citation2020; Rahman, Carlson, et al., Citation2022; Rahman, Carlson, Gudergan, et al., Citation2022; Xiao, Zhang, et al., Citation2019). However, three studies related to consumer behavior in the omnichannel retail setting that applied MEC Theory. The researchers attempted to examine the effect of channel integration on the intention to revisit omnichannel retailer that mediated by customer satisfaction and shopping value. As an extension, our research seeks to apply the MEC Theory in the context of omnichannel retailing in Indonesia.
First, the researchers position channel integration as an attribute of shopping value. Since the researchers identify inconsistency finding from previous research to examine the effect of channel integration in different omnichannel retail context. Therefore, the researchers consider the integrated customer service, integrated information access, integrated product and price, integrated transaction information, and integrated promotion as dimension of channel integration. Furthermore, the results show that the integrated promotion and integrated product and price did not influence channel integration. The researchers conclude that this research contributes to the existing literature by performing a multidimensional analysis to describe omnichannel integration and its effect on customer journey.
Second, our study applied customer satisfaction and shopping value as mediating variable. The findings proved that customer satisfaction showed slightly higher effect compared to shopping value. In the context of omnichannel retailing environment, customer satisfaction and shopping value represent as mediating variable between channel integration and intention to visit in different path. Moreover, there is no relationship between shopping value and customer satisfaction. Third, our study enriches the understanding of channel integration to increase customer satisfaction and provide added value for customers in Indonesia. Finally, this study provides academic significance that it supported and enrich the usage of Means-End Chain Theory in user experience research in the context of omnichannel retailing.
The analysis showed that intention to revisit positively affected by customer satisfaction and shopping value. These findings supported the previous research findings that examined the relationship between customer satisfaction (Cattapan & Pongsakornrungsilp, Citation2022; Chang & Geng, Citation2022; Gao et al., Citation2021) and shopping value (Chang & Geng, Citation2022; Pereira et al., Citation2023; Rahman, Carlson, Gudergan, et al., Citation2022; Song & Jo, Citation2023) on intention to revisit. Based on the findings, the researchers concluded that higher customer satisfaction and shopping value will cause higher intention to revisit retailer that implement omnichannel strategy. If the customer who realized that shopping in retailer that implement omnichannel strategy are efficient and effective, they will feel satisfied and more value when shopping, their intention to revisit will increase. However, the effect of customer satisfaction is slightly higher than shopping value. Therefore, the retailer should design seamless and smooth shopping experience by integrating their online and offline channel for ensuring the customer experienced best and superior services. Finally, the retailer should not neglect the importance of shopping value. The retailer should offer and highlight the value added of omnichannel strategy. When the customer realized that shopping in omnichannel retailer will save their time, cost, and effort for searching and buying, they willing to revisit or recommend to others.
The study findings revealed that channel integration positively effect on shopping value in the context of omnichannel retailer. The findings supported the previous research findings that examined the channel integration affect shopping value positively (Asare et al., Citation2022; Cotarelo et al., Citation2021; Gao et al., Citation2021; Wang & Jiang, Citation2022). The researchers concluded that the higher of omnichannel integration will cause higher shopping value from customer perspective. The implementation of omnichannel integration strategy can be considered as competitive advantage for the company to compete in uncertain environment. Therefore, omnichannel integration can improve the customer shopping experience and the customer will realize the value added. When the customer in the pre-purchase stage, the retailer should be able to provide a personalized product recommendation in the customer preferred online channel based on the integration of transaction information. The branch manager also can utilize the customer purchase transaction for identifying potential product to be promoted in their monthly newsletter and develop digital content related to product demo based on the customer inquiry from customer service.
The analysis found that channel integration positively effect on customer satisfaction. The findings supported the previous research findings that examined the channel integration on customer satisfaction (Cattapan & Pongsakornrungsilp, Citation2022; Gao et al., Citation2021; Lazaris et al., Citation2021; Wang & Jiang, Citation2022; Zhang et al., Citation2018; Zhu et al., Citation2022). Customers feel satisfied during their shopping journey because the retailer manages to integrate their online channel and physical store. When the retailer gives customers the flexibility to order, pay, and get the products they need, customer satisfaction increases. Therefore, it is important for retailers to design procedures, policies, and information systems that can allow customers to order products and pay for orders through online or offline channels, obtain products through in-store pick up or use internal or third-party delivery services, and get customer service support online or offline.
In this study, the researchers also introduce customer satisfaction and shopping value as mediator variable for explaining complex customer experience in omnichannel retail context. Customer satisfaction and shopping value can be considered as important factors for managing customer journey in omnichannel retail environment. These findings supported the previous research finding that examined the role of customer satisfaction and shopping value as mediator between channel integration and intention to revisit (Cotarelo et al., Citation2021; Lazaris et al., Citation2021). The retailer who is concerned about the customer satisfaction after shopping on integrated online and offline channels can increase their customer intention to revisit. Retailers who regularly evaluate the omnichannel customer journey and improve the quality of channel integrations can slowly identify customer shopping value. Therefore, the retailer should focus on integrating the order fulfillment, transaction information, customer service, and information access to increase customer shopping value and satisfaction.
The empirical findings showed that shopping value does not affect customer satisfaction. The finding is contrary to the previous study that found that shopping value positively affects customer satisfaction (Cotarelo et al., Citation2021). Customers have not considered convenience; time and cost efficiency can increase their satisfaction in shopping at retailers that implement an omnichannel strategy. The contradictory findings could be related to the demographic profile of respondents. Most respondents only visit physical stores 1–2 times and online stores, and physical stores are favorite channels to find and buy products. This can affect their assessment in assessing shopping value and satisfaction.
6. Managerial implication
This study presents several managerial implications. First, the hypothesis testing results reveal that channel integration improve the customer satisfaction and shopping value. Therefore, the management must concern in customer satisfaction survey and identify novel mechanism to encourage the customer participate in the survey. In addition, exhibiting regular focus group discussions for identifying shopping value after using online and offline channel. Second, the insight from higher order model analysis show that customer in Indonesia consider integration of order fulfillment and customer service is important for their shopping journey. The management must ensure the flexibility in customer order and inquiry for after sales support when they adopt new online channel, collaborating with emerging third party logistic, or opening new physical store. Finally, integration of transaction information and information access is essential for pre and post-shopping journey. The customer can recall their history of purchase transaction when they want to repurchase the item, claim the warranty, or need assistance for using the product. The retailer that applies transaction information and information access integration will not require the customer to keep the transaction receipt. Moreover, they can access all the information through the physical store system or mobile application.
7. Conclusion
In summary, the findings showed that a well-integrated omnichannel retail environment for order fulfillment, transaction information, customer service, and information access will increase the customer satisfaction and shopping value. The customer will dissatisfy when the information scattered and different across the channel. Furthermore, customer satisfaction and shopping value will increase the intention to revisit.
There are several limitations in this research and the researchers also present the future direction. First, our research did not consider the characteristics of product displayed on online and offline channels. The shopping journey in omnichannel may be different based on the characteristics of the product. In the future, it is important to investigate the omnichannel customer by accommodating the product type. Second, the current study examined only users in ACE hardware store from Indonesia. To improve the generalization of the findings, future studies should investigate the customer from several retailers that implement omnichannel strategies in Indonesia. Third, the researchers employ convenience sampling in current research due to COVID-19 pandemic. The sampling selection has limitations for generalization of findings. Therefore, the future research should apply probability sampling for finding generalization. Forth, perceived value used in this research focus on utilitarian value. Since the perceived value is complex in consumer behaviours. The future research should apply Schwartz theory of basic value for better understanding of shopping value. Lastly, the researchers treat the dimensions of omnichannel integration as one construct may not be able to specifically explain the effect size of each dimension on shopping value and customer satisfaction. The future research should treat the dimensions of omnichannel as five constructs.
Author contributions
Conceptualization, A.M.S., D.M., and G.R.H.; methodology, A.M.S., N.S.P., and G.R.H.; software, A.M.S. and D.M.; validation, A.M.S., P.U., and N.S.P.; formal analysis, A.M.S. and D.M.; investigation, D.M., G.R.H., and N.S.P.; resources, A.M.S., D.M., and N.S.P.; data curation, D.M., N.S.P., and A.M.S.; writing—original draft preparation, D.M., G.R.H., and N.S.P.; writing—review and editing, A.M.S. and P.U.; visualization, D.M., G.R.H., and N.S.P.; supervision, A.M.S. and P.U.; project administration, D.M., G.R.H., and N.S.P.; funding acquisition, N/A. All authors have read and agreed to the published version of the manuscript.
Disclosure statement
No potential conflict of interest was reported by the author(s).
Data availability statement
Data available on request from the authors.
Additional information
Funding
Notes on contributors
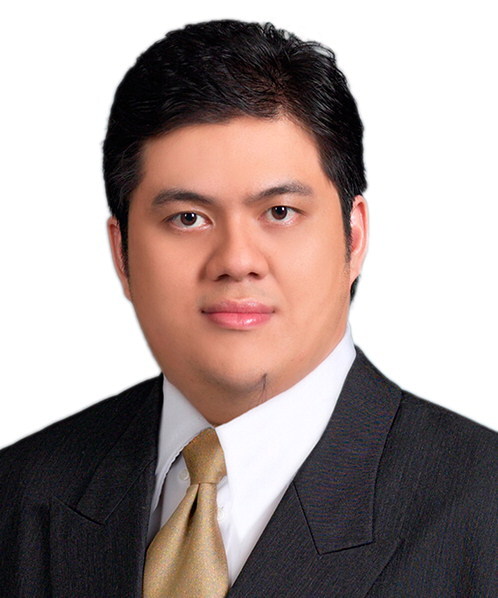
Arta Moro Sundjaja
Arta Moro Sundjaja is an associate professor of Management Information System at Bina Nusantara University. His research interest are technology adoption, consumer behaviour, and IS strategic management.
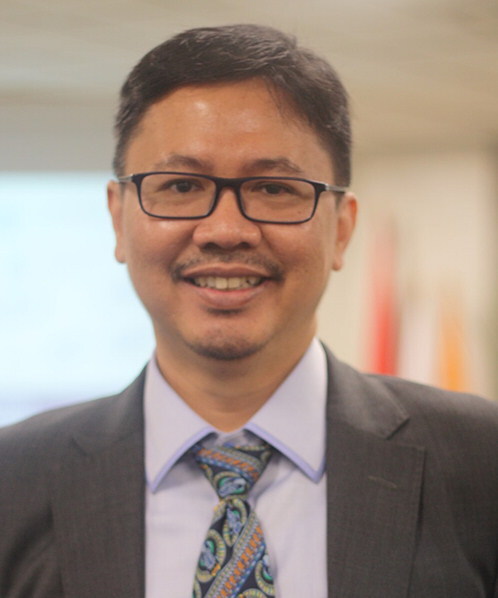
Prio Utomo
Prio Utomo is an associate professor of Technology Management at Universitas Multimedia Nusantara. His research interest are behavioural strategy, technology adoption, strategic management.
Darrell Matthew
Darrell Matthew is a master student of Binus Business School Master Program, Bina Nusantara University.
Garry Reverio Hellianto
Garry Reverio Hellianto is a master student of Binus Business School Master Program, Bina Nusantara University.
Niko Sutanto Putra
Niko Sutanto Putra is a master student of Binus Business School Master Program, Bina Nusantara University.
References
- Ahmed, H., & Syed, S.-U.-H. (2021). Omnichannel customer experience in the context of omnichannel shopping value: The moderating role of omnichannel intensity (a focus on fashion retail in Pakistan). KASBIT Business Journal, 14(1), 1–19. https://kasbitoric.com/index.php/kbj/article/view/149
- Ali Memon, M., Ting, H., Cheah, J.-H., Thurasamy, R., Chuah, F., & Huei Cham, T. (2020). Sample size for survey research: Review and recommendations. Journal of Applied Structural Equation Modeling, 4(2), i–xx. https://doi.org/10.47263/JASEM.4(2)01
- Ameen, N., Tarhini, A., Shah, M., & Madichie, N. O. (2020). Going with the flow: Smart shopping malls and omnichannel retailing. Journal of Services Marketing, 35(3), 325–348. https://doi.org/10.1108/JSM-02-2020-0066
- Asare, C., Majeed, M., & Cole, N. A. (2022). Omnichannel integration quality, perceived value, and brand loyalty in the consumer electronics market: The mediating effect of consumer personality. Lecture Notes in Networks and Systems, 392, 29–45. https://doi.org/10.1007/978-981-19-0619-0_4
- Bagozzi, R. P., & Yi, Y. (2012). Specification, evaluation, and interpretation of structural equation models. Journal of the Academy of Marketing Science, 40(1), 8–34. https://doi.org/10.1007/s11747-011-0278-x
- Blom, A., Lange, F., & Hess, R. L. (2021). Omnichannel promotions and their effect on customer satisfaction. European Journal of Marketing, 55(13), 177–201. https://doi.org/10.1108/EJM-12-2018-0866/FULL/PDF
- Borgardt, E. (2020). Means-end chain theory: A critical review of literature. Prace Naukowe Uniwersytetu Ekonomicznego We Wrocławiu, 64(3), 141–160. https://doi.org/10.15611/pn.2020.3.12
- Cai, L., Yuen, K. F., Fang, M., & Wang, X. (2023). A literature review on the impact of the COVID-19 pandemic on consumer behaviour: Implications for consumer-centric logistics. Asia Pacific Journal of Marketing and Logistics, 35(11), 2682–2703. https://doi.org/10.1108/APJML-08-2022-0731/FULL/XML
- Cattapan, T., & Pongsakornrungsilp, S. (2022). Impact of omnichannel integration on millennials’ purchase intention for fashion retailer. Cogent Business & Management, 9(1), 1–26. https://doi.org/10.1080/23311975.2022.2087460
- Chang, Y., & Geng, L. (2022). Planned or unplanned purchases? The effects of perceived values on omnichannel continuance intention. International Journal of Retail & Distribution Management, 50(12), 1535–1551. https://doi.org/10.1108/IJRDM-01-2021-0012
- Cohen, J. (1988). Statistical power analysis for the behavioral sciences (2nd ed.). L. Erlbaum Associates.
- Cotarelo, M., Fayos, T., Calderón, H., & Mollá, A. (2021). Omni-channel intensity and shopping value as key drivers of customer satisfaction and loyalty. Sustainability, 13(11), 5961. https://doi.org/10.3390/su13115961
- Gao, W., Fan, H., Li, W., & Wang, H. (2021). Crafting the customer experience in omnichannel contexts: The role of channel integration. Journal of Business Research, 126, 12–22. https://doi.org/10.1016/j.jbusres.2020.12.056
- Gardial, S. F., Clemons, D. S., Woodruff, R. B., Schumann, D. W., & Burns, M. J. (1994). Comparing consumers’ recall of prepurchase and postpurchase product evaluation experiences. Journal of Consumer Research, 20(4), 548–560. https://doi.org/10.1086/209369
- Grewal, D., & Roggeveen, A. L. (2020). Understanding retail experiences and customer journey management. Journal of Retailing, 96(1), 3–8. https://doi.org/10.1016/j.jretai.2020.02.002
- Gunawan, A. R., Ratnaningtyas, S., & Wibowo, S. A. (2023). Proposed omnichannel strategy to increase sales revenue in a fashion retail company (Case: La Omvi). International Journal of Current Science Research and Review, 6(6), 3729–3747. https://doi.org/10.47191/ijcsrr/V6-i6-63
- Gutman, J. (1982). A means-end chain model based on consumer categorization processes. Journal of Marketing, 46(2), 60–72. https://doi.org/10.1177/002224298204600207
- Hair, J. F., Black, W. C., Babin, B. J., & Anderson, R. E. (2019). Multivariate data analysis (8th ed.). Cengage. www.cengage.com/highered
- Hair, J. F., Hult, G. T. M., Ringle, C. M., & Sarstedt, M. (2021). A primer on partial least squares structural equation modeling (PLS-SEM). SAGE Publication. https://us.sagepub.com/en-us/nam/a-primer-on-partial-least-squares-structural-equation-modeling-pls-sem/book270548
- Hair, J., Hult, G. T., Ringle, C., & Sarstedt, M. (2017). A primer on partial least squares structural equation modeling (PLS-SEM). Sage.
- Henseler, J., Ringle, C. M., & Sarstedt, M. (2015). A new criterion for assessing discriminant validity in variance-based structural equation modeling. Journal of the Academy of Marketing Science, 43(1), 115–135. https://doi.org/10.1007/s11747-014-0403-8
- Hickman, E., Kharouf, H., & Sekhon, H. (2020). An omnichannel approach to retailing: demystifying and identifying the factors influencing an omnichannel experience. The International Review of Retail, Distribution and Consumer Research, 30(3), 266–288. https://doi.org/10.1080/09593969.2019.1694562
- Hsia, T. L., Wu, J. H., Xu, X., Li, Q., Peng, L., & Robinson, S. (2020). Omnichannel retailing: The role of situational involvement in facilitating consumer experiences. Information & Management, 57(8), 103390. https://doi.org/10.1016/j.im.2020.103390
- Huang, S. L., & Hung, M. S. (2023). Exploring factors influencing consumers’ participation in online shopping days: Using double 11 day as an example. Journal of Organizational Computing and Electronic Commerce, 33(3–4), 133–161. https://doi.org/10.1080/10919392.2023.2261811
- Huré, E., Picot-Coupey, K., & Ackermann, C. L. (2017). Understanding omni-channel shopping value: A mixed-method study. Journal of Retailing and Consumer Services, 39, 314–330. https://doi.org/10.1016/j.jretconser.2017.08.011
- Jin, N. P., Lee, S., & Lee, H. (2015). The effect of experience quality on perceived value, satisfaction, image and behavioral intention of water park patrons: New versus repeat visitors. International Journal of Tourism Research, 17(1), 82–95. https://doi.org/10.1002/jtr.1968
- Khattak, A., Tabash, M. I., Yousaf, Z., Radulescu, M., Nassani, A. A., & Haffar, M. (2022). Towards innovation performance of SMEs: Investigating the role of digital platforms, innovation culture and frugal innovation in emerging economies. Journal of Entrepreneurship in Emerging Economies, 14(5), 796–811. https://doi.org/10.1108/JEEE-08-2021-0318
- Kim, C., Costello, F. J., & Lee, K. C. (2020). The unobserved heterogeneneous influence of gamification and novelty-seeking traits on consumers’ repurchase intention in the omnichannel retailing. Frontiers in Psychology, 11, 1664. https://doi.org/10.3389/fpsyg.2020.01664
- Kock, N. (2015). Common method bias in PLS-SEM: A full collinearity assessment approach. International Journal of e-Collaboration, 11(4), 1–10. https://doi.org/10.4018/ijec.2015100101
- Kock, N., & Lynn, G. S. (2012). Lateral collinearity and misleading results in variance-based SEM: An illustration and recommendations. Journal of the Association for Information Systems, 13(7), 546–580. https://doi.org/10.17705/1jais.00302
- Kopot, C., & Cude, B. J. (2021). Channel depth or consistency? A study on establishing a sustainable omnichannel strategy for fashion department store retailers. Sustainability, 13(13), 6993. https://doi.org/10.3390/su13136993
- Kumar, V., & Ayodeji, O. G. (2021). E-retail factors for customer activation and retention: An empirical study from Indian e-commerce customers. Journal of Retailing and Consumer Services, 59, 102399. https://doi.org/10.1016/j.jretconser.2020.102399
- Lazaris, C., Sarantopoulos, P., Vrechopoulos, A., & Doukidis, G. (2021). Effects of increased omnichannel integration on customer satisfaction and loyalty intentions. International Journal of Electronic Commerce, 25(4), 440–468. https://doi.org/10.1080/10864415.2021.1967005
- Leão, P., & da Silva, M. M. (2021). Impacts of digital transformation on firms’ competitive advantages: A systematic literature review. Strategic Change, 30(5), 421–441. https://doi.org/10.1002/jsc.2459
- Lee, Z. W. Y., Chan, T. K. H., Chong, A. Y. L., & Thadani, D. R. (2019). Customer engagement through omnichannel retailing: The effects of channel integration quality. Industrial Marketing Management, 77, 90–101. https://doi.org/10.1016/j.indmarman.2018.12.004
- Lehrer, C., & Trenz, M. (2022). Omnichannel business. Electronic Markets, 32(2), 687–699. https://doi.org/10.1007/s12525-021-00511-1
- Liang, Z. X., Lai, K. P., Chong, S. C., & Tan, C. L. (2022). Understanding Malaysian students online service experiences for achieving sustainability in private higher education institutions. Journal of Sustainability Science and Management, 17(4), 161–182. https://doi.org/10.46754/jssm.2022.4.013
- Lim, X. J., Cheah, J. H., Dwivedi, Y. K., & Richard, J. E. (2022). Does retail type matter? Consumer responses to channel integration in omni-channel retailing. Journal of Retailing and Consumer Services, 67, 102992. https://doi.org/10.1016/j.jretconser.2022.102992
- Limanseto, H. (2022, April 1). Akselerasi Ekonomi Digital pada e-Commerce dan Online Travel Menjadi Salah Satu Strategi Efektif Mendorong Kinerja Perekonomian Nasional. Biro Komunikasi, Layanan Informasi, Dan Persidangan Kementerian Koordinator Bidang Perekonomian. https://www.ekon.go.id/publikasi/detail/3978/akselerasi-ekonomi-digital-pada-e-commerce-dan-online-travelmenjadi-salah-satu-strategi-efektif-mendorong-kinerja-perekonomian-nasional
- Moriuchi, E., & Takahashi, I. (2023). An empirical study on repeat consumer’s shopping satisfaction on C2C e-commerce in Japan: The role of value, trust and engagement. Asia Pacific Journal of Marketing and Logistics, 35(3), 560–581. https://doi.org/10.1108/APJML-08-2021-0631
- Mosquera, A., Olarte Pascual, C., & Juaneda Ayensa, E. (2017). Understanding the customer experience in the age of omni-channel shopping. Revista ICONO14 Revista Científica de Comunicación y Tecnologías Emergentes, 15(2), 92–114. https://doi.org/10.7195/ri14.v15i2.1070
- Mukherjee, M., & Wood, J. (2021). Consolidating unorganised retail businesses through digital platforms: Implications for achieving the un sustainable development goals. Sustainability, 13(21), 12031. https://doi.org/10.3390/su132112031
- Olson, J. C., & Reynolds, T. J. (1983). Understanding consumers’ cognitive structures: Implications for advertising strategy. Advertising and Consumer Psychology, 1, 77–90.
- Pereira, M. L., de La Martinière Petroll, M., Soares, J. C., Matos, C. A., & Hernani-Merino, M. (2023). Impulse buying behaviour in omnichannel retail: an approach through the stimulus-organism-response theory. International Journal of Retail & Distribution Management, 51(1), 39–58. https://doi.org/10.1108/IJRDM-09-2021-0394
- Podsakoff, P. M., & Organ, D. W. (1986). Self-reports in organizational research: Problems and prospects. Journal of Management, 12(4), 531–544. https://doi.org/10.1177/014920638601200408
- Preacher, K. J., & Hayes, A. F. (2008). Asymptotic and resampling strategies for assessing and comparing indirect effects in multiple mediator models. Behavior Research Methods, 40(3), 879–891. https://doi.org/10.3758/BRM.40.3.879
- Rahman, S. M., Carlson, J., & Chowdhury, N. H. (2022). SafeCX: A framework for safe customer experience in omnichannel retailing. Journal of Services Marketing, 36(4), 499–529. https://doi.org/10.1108/JSM-04-2021-0114
- Rahman, S. M., Carlson, J., Gudergan, S. P., Wetzels, M., & Grewal, D. (2022). Perceived omnichannel customer experience (OCX): Concept, measurement, and impact. Journal of Retailing, 98, 611–632. https://doi.org/10.1016/j.jretai.2022.03.003
- Riaz, H., Baig, U., Meidute-Kavaliauskiene, I., & Ahmed, H. (2021). Factors effecting omnichannel customer experience: Evidence from fashion retail. Information, 13(1), 12. https://doi.org/10.3390/info13010012
- Roggeveen, A. L., & Sethuraman, R. (2020). Customer-interfacing retail technologies in 2020 & beyond: An integrative framework and research directions. Journal of Retailing, 96(3), 299–309. https://doi.org/10.1016/j.jretai.2020.08.001
- Rusanen, O. (2018). Crafting an omnichannel strategy: Identifying sources of competitive advantage and implementation barriers. In Piotrowicz, W., Cuthbertson, R. (Eds.), Exploring Omnichannel Retailing. (pp. 11–46). Cham: Springer. https://doi.org/10.1007/978-3-319-98273-1_2
- Ryu, J. S., Fortenberry, S., & Warrington, P. (2023). Understanding omnichannel shopping behaviors: Incorporating channel integration into the theory of reasoned action. Journal of Consumer Sciences, 8(1), 15–26. https://doi.org/10.29244/jcs.8.1.15-26
- Saghiri, S., & Mirzabeiki, V. (2021). Omni-channel integration: The matter of information and digital technology. International Journal of Operations & Production Management, 41(11), 1660–1710. https://doi.org/10.1108/IJOPM-04-2021-0262
- Sarstedt, M., Hair, J. F., & Ringle, C. M. (2022). “PLS-SEM: Indeed a silver bullet”–Retrospective observations and recent advances. Journal of Marketing Theory and Practice, 31(3), 261–275. https://doi.org/10.1080/10696679.2022.2056488
- Sarstedt, M., Hair, J. F., Cheah, J. H., Becker, J. M., & Ringle, C. M. (2019). How to specify, estimate, and validate higher-order constructs in PLS-SEM. Australasian Marketing Journal, 27(3), 197–211. https://doi.org/10.1016/j.ausmj.2019.05.003
- Sarstedt, M., Hair, J. F., Pick, M., Liengaard, B. D., Radomir, L., & Ringle, C. M. (2022). Progress in partial least squares structural equation modeling use in marketing research in the last decade. Psychology & Marketing, 39(5), 1035–1064. https://doi.org/10.1002/mar.21640
- SEADS (2021). Economy SEA 2021—Roaring 20s: The SEA Digital Decade. SEADS. https://seads.adb.org/report/e-conomy-sea-2021-roaring-20s-sea-digital-decade
- Shmueli, G., Sarstedt, M., Hair, J. F., Cheah, J. H., Ting, H., Vaithilingam, S., & Ringle, C. M. (2019). Predictive model assessment in PLS-SEM: Guidelines for using PLSpredict. European Journal of Marketing, 53(11), 2322–2347. https://doi.org/10.1108/EJM-02-2019-0189
- Sirclo (2020). Kenali Pelanggan Omnichannel E-Commerce dan Faktor Perilaku Berbelanjanya. SIRCLO. https://www.sirclo.com/blog/kenali-pelanggan-omnichannel-e-commerce-dan-faktor-perilaku-berbelanjanya/
- Song, H. G., & Jo, H. (2023). Understanding the continuance intention of omnichannel: combining TAM and TPB. Sustainability, 15(4), 3039. https://doi.org/10.3390/su15043039
- Statistik, D. (2022). Statistik E-commerce 2022. https://www.bps.go.id/publication/2022/12/19/d215899e13b89e516caa7a44/statistik-e-commerce-2022.html
- Sugiat, M., Saabira, N., & Witarsyah, D. (2023). Omni-channel service analysis of purchase intention. International Journal on Informatics Visualization, 7(4), 2543–2549. https://doi.org/10.30630/joiv.7.4.2442
- Thaichon, P., Quach, S., Barari, M., & Nguyen, M. (2023). Exploring the role of omnichannel retailing technologies: Future research directions. Australasian Marketing Journal. https://doi.org/10.1177/14413582231167664
- Timoumi, A., Gangwar, M., & Mantrala, M. K. (2022). Cross-channel effects of omnichannel retail marketing strategies: A review of extant data-driven research. Journal of Retailing, 98(1), 133–151. https://doi.org/10.1016/j.jretai.2022.02.008
- Tripathi, A., Bagga, T., Sharma, S., & Vishnoi, S. K. (2021). Big Data-driven marketing enabled business performance: A conceptual framework of information, strategy and customer lifetime value. 2021 11th International Conference on Cloud Computing, Data Science & Engineering (Confluence) (pp. 315–320). https://doi.org/10.1109/Confluence51648.2021.9377156
- Utomo, P., & Kurniasari, F. (2023). The dynamic capability and ambidexterity in the early-stage startups: A hierarchical component model approach. Eurasian Studies in Business and Economics, 25, 49–61. https://doi.org/10.1007/978-3-031-36286-6_3/COVER
- Utomo, P., Cham, T.-H., Pek, C.-K., & Mamatkulov, S. (2023). Deep dive into the augmented reality customer experience and adoption research: A bibliometric study. In M. A. Al-Sharafi, M. Al-Emran, G. W.-H. Tan, & K.-B. Ooi (Eds.), Current and future trends on intelligent technology adoption. Studies in computational intelligence (Vol. 1128, pp. 413–433). Springer Nature Switzerland. https://doi.org/10.1007/978-3-031-48397-4_21
- Uzir, M. U. H., Al Halbusi, H., Lim, R., Jerin, I., Abdul Hamid, A. B., Ramayah, T., & Haque, A. (2021). Applied artificial intelligence and user satisfaction: Smartwatch usage for healthcare in Bangladesh during COVID-19. Technology in Society, 67, 101780. https://doi.org/10.1016/j.techsoc.2021.101780
- Villanova, D., Bodapati, A. V., Puccinelli, N. M., Tsiros, M., Goodstein, R. C., Kushwaha, T., Suri, R., Ho, H., Brandon, R., & Hatfield, C. (2021). Retailer marketing communications in the digital age: Getting the right message to the right shopper at the right time. Journal of Retailing, 97(1), 116–132. https://doi.org/10.1016/J.JRETAI.2021.02.001
- Wang, J., & Jiang, X. (2022). The impact of omnichannel shopping experience and channel integration on customer retention: Empirical evidence from China. Journal of Asian Finance, 9(2), 229–242. https://doi.org/10.13106/jafeb.2022.vol9.no2.0229
- Xiao, L., Guo, Z., & D’Ambra, J. (2019). Benefit-based O2O commerce segmentation: A means-end chain approach. Electronic Commerce Research, 19(2), 409–449. https://doi.org/10.1007/s10660-017-9286-3
- Xiao, L., Zhang, Y., & Fu, B. (2019). Exploring the moderators and causal process of trust transfer in online-to-offline commerce. Journal of Business Research, 98, 214–226. https://doi.org/10.1016/j.jbusres.2019.01.069
- Yao, P., Sabri, M. F., Osman, S., Zainudin, N., & Li, Y. (2023). Consumers continued intention to use online-to-offline (O2O) services in omnichannel retail: Differences between to-shop and to-home models. Sustainability, 15(2), 945. https://doi.org/10.3390/su15020945
- Zhang, M., Ren, C., Wang, G. A., & He, Z. (2018). The impact of channel integration on consumer responses in omni-channel retailing: The mediating effect of consumer empowerment. Electronic Commerce Research and Applications, 28, 181–193. https://doi.org/10.1016/j.elerap.2018.02.002
- Zhu, Y., Li, Y., Liu, L., Liu, H., & Zhong, Q. (2022). Effect of online–offline informational integration on customer patronage: The mediating roles of customer satisfaction and perceived deception. Journal of Data, Information and Management, 4(3–4), 291–303. https://doi.org/10.1007/s42488-022-00081-7