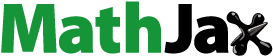
Abstract
The burgeoning panda tourism market in China is attracting an increasing number of domestic and international tourists. This study focuses on the Chengdu Research Base of Giant Panda Breeding as a case study and utilizes Latent Dirichlet Allocation (LDA) modeling and topic-based sentiment analysis to conduct text mining on online travel reviews in both English and Chinese languages. LDA modeling was employed to identify topics within online reviews, with a subsequent evaluation of the importance of each topic. Furthermore, topic-based sentiment analysis was conducted to assess the performance of different topics. Through importance-performance analysis, this study interprets the destination image disparities between English and Chinese reviews from a cross-cultural perspective. The research findings validate the effectiveness of destination image analysis methods, providing valuable insights for tailoring distinct destination marketing strategies that target tourists from diverse linguistic backgrounds.
1. Introduction
The global tourism market operates within a highly competitive landscape, characterized by destinations competing for the attention and patronage of tourists (Cronjé & Du Plessis, Citation2020). Destination image plays a pivotal role in tourists’ decision-making processes, underscoring the imperative need for effective destination image management in tourism marketing (VG et al., Citation2021). Successful image not only cultivates the uniqueness and brand identity of a destination but also amplifies its competitiveness while augmenting tourist experience and satisfaction (Baloglu et al., Citation2014; Kanwel et al., Citation2019). Destinations with positive images are better equipped to navigate crises or negative publicity and mitigate their adverse impacts (Rittichainuwat et al., Citation2020). Furthermore, by disseminating a favorable image emphasizing environmental preservation, cultural heritage, and social responsibility, destinations can safeguard their resources and environment, fostering sustainable development within the tourism industry (J. C. Zhang et al., Citation2020).
The advancement of digital technology has transformed tourists’ information acquisition and behavioral patterns, precipitating significant changes in the tourism market (Nikolskaya et al., Citation2021). The digital transformation of the tourism industry has had a profound impact on destination marketing (Sorokina et al., Citation2022). Destinations have to actively utilize digital channels such as online travel platforms, social media, and mobile applications to cultivate positive destination images, bolster online marketing efforts, and engage with tourists (Oliveira & Panyik, Citation2015). Besides, as consumer demands for personalized and diverse travel experiences escalate, destinations have to formulate differentiated marketing strategies tailored to the needs of distinct market segments (Xiao et al., Citation2022). In the context of tourism globalization, expanding into international markets requires attracting tourists from diverse cultural backgrounds, thus increasing the complexity of destination marketing and presenting significant challenges to destination image management (Lee & Park, Citation2023).
Understanding tourists’ perceptions and evaluations of a destination is fundamental to managing its image and formulating effective marketing strategies (Ferrer-Rosell & Marine-Roig, Citation2020). While traditional survey methods primarily employ questionnaires and interviews to gauge tourists’ opinions of destinations, the rise of digital media has made user-generated content (UGC) on online travel platforms and social media a crucial resource for destination image research (Wang et al., Citation2021). UGC encompasses a wide range of content, including shared travel experiences, travel-related concerns, and satisfaction ratings. It offers immediate, comprehensive, and easily accessible data that reflects the dynamic shifts in tourists’ preferences and behaviors (Arefieva et al., Citation2021). The analysis of such data constitutes a burgeoning focal point within destination image research (Khan et al., Citation2021).
Conducting UGC analysis from a cross-cultural perspective can offer essential support for both industry practices and policy formulation in managing destination image. In terms of tourism marketing practice, cross-cultural analysis aids in identifying potential market demands, breaking cultural barriers, developing targeted marketing strategies, and expanding the international markets (De La Hoz-Correa & Muñoz-Leiva, Citation2019). Regarding policy formulation, local governments can adjust policies and management measures based on the needs and preferences of diverse cultural groups, enhancing the international competitiveness of destinations and driving tourism development (Filimonau & Perez, Citation2018).
Emerging tourism markets present new opportunities and challenges for the tourism industry (Peng et al., Citation2022). China’s panda tourism market, as a vital component of the ecotourism landscape, emerges as a burgeoning sector with vast developmental potential. The Chengdu Research Base of Giant Panda Breeding, commonly referred to as the ‘Panda Base’, stands out as the premier attraction (Zou, Citation2020). Recognized as one of the top ten tourist attractions in China, this national 4 A-level site seamlessly combines giant panda research, education, breeding, conservation, and ecotourism (X. Li et al., Citation2023). Located in Chengdu City’s urban area, the facility houses over 200 giant pandas, accounting for a quarter of the world’s captive giant panda population. Positioned as an international panda tourism destination, the Panda Base currently attracts approximately 9 million tourists annually and aims to attract 21.3 million tourists annually by 2035 (Q. Li, Citation2020; X. Yan, Citation2022). However, enhancing the destination’s image to achieve a substantial increase in tourist numbers is an urgent issue that the Panda Base has to address.
This research aims to provide a theoretical framework to understand destination image through a cross-cultural analysis of UGC, while also offering practical insights into the development of differentiated destination marketing strategies. Specifically focusing on the Panda Base within the context of the emerging panda tourism market in China, this research endeavors to address the marketing challenges pertinent to this sector. With an intention to contribute to the scholarly discourse, this research is anticipated to serve as a valuable resource for public sector (e.g. governments) and private sector (e.g. destination marketing organizations). Ultimately, the findings of the research are potential to enrich the body of knowledge within the field of tourism and hospitality studies.
2. Literature review
2.1. Destination image research in a cross-cultural context
Destination image, recognized as a multifaceted construct, encompasses cognitive, affective, and conative dimensions (Gartner, Citation1994). The cognitive image pertains to tourists’ perceptions of destination attributes, the affective image encapsulates personal attitudes, and the conative image reflects travel intentions (Pike & Ryan, Citation2004). From a communication and marketing perspective, the concept of destination image is further classified into projected image and perceived image (Bramwell & Rawding, Citation1996).
The relationship between destination image and culture is intricate and pivotal. On one hand, the unique culture that a destination holds serves as a significant dimension of its overall image (Dong et al., Citation2023; Seo & Oh, Citation2017). On the other hand, the diverse cultural backgrounds of tourists play a crucial role in shaping the perceived image of a destination (Beerli & Martín, Citation2004).
Cultural orientations, such as preexisting stereotypes associated with specific cultures, give rise to expectations and preferences regarding various aspects of a destination (Stepchenkova et al., Citation2014). Furthermore, individuals from different backgrounds interpret symbols, signs, and experiences in unique ways, influencing word-of-mouth communication, particularly within cultural communities (Buzova et al., Citation2019; Reisinger & Turner, Citation1998). Tourists’ familiarity with cultural elements and behavioral norms directly impacts their comfort and satisfaction with the destination (Kozak et al., Citation2003; Tigre Moura et al., Citation2014).
Hence, the diverse array of cultural backgrounds among tourists exacerbates the challenge of navigating the diversity of meanings in destination image perception (MacKay & Fesenmaier, Citation2000). Understanding the varied perceived images through a cultural lens is crucial for tailoring marketing to diverse target markets, serving as a cornerstone in international destination marketing strategies (Lee & Park, Citation2023).
In cross-cultural investigations, nationality frequently serves as the primary criterion for categorizing tourists’ cultural backgrounds. Studies on destination images in Istanbul, Russia, and Beijing reveals noteworthy distinctions in the perceived images among tourists of diverse nationalities (Sahin & Baloglu, Citation2011; Stepchenkova et al., Citation2014; K. Zhang et al., Citation2019). Additionally, comparative investigations were conducted. For instance, international tourists exhibit elevated expectations and standards for services compared with their domestic counterparts, with a particular emphasis on environmental factors (Bonn et al., Citation2005). Japanese tourists prioritize emotional and novel experiences, whereas western tourists tend to emphasize the utilitarian aspect of value for money (Williams et al., Citation2017).
However, a notable limitation arises, as most of these studies rely on nationality to delineate the cultural characteristics of tourists, thereby overlooking the pivotal role of language as a cultural carrier. Language is a fundamental component of culture and plays a crucial role in encoding and decoding information, shaping a framework for interpreting the world (Kramsch, Citation2014). While there have been studies utilizing language as a cultural indicator to explore the travel behavior of various groups (Kay, Citation2013; Xi et al., Citation2022), the focus has predominantly been on investigating motivations for travel and factors influencing behavior, with limited attention given to destination image. Therefore, it is imperative to distinguish tourists’ cultural backgrounds by language and systematically examine the cross-cultural differences in destination images.
Within tourism research, Hofstede’s six-cultural dimension model (Hofstede, Citation2011), serves as the primary analytical framework for elucidating cross-cultural differences. In Hofstede’s framework, an analysis was conducted on responses obtained from 534 Internet users across six countries. Special attention was given to the moderating influence of cross-cultural differences, specifically those related to Individualism and Uncertainty Avoidance, in shaping destination image (De La Hoz-Correa & Muñoz-Leiva, Citation2019). Additionally, researchers have shed light on the distinctions in both projected and perceived images within a cross-cultural context (Q. Chen et al., Citation2023).
2.2. LDA modeling in destination image research
As UGC data garners have gained increased attention in tourism research, various text mining techniques have been employed. These techniques include topic modeling, sentiment analysis, social network analysis, and more (Albalawi et al., Citation2020). Among these, topic modeling is frequently applied to uncover prevalent themes within a body of text. Prominent techniques in topic modeling include Latent Semantic Analysis (LSA), Probabilistic Latent Semantic Analysis (PLSA), Non-Negative Matrix Factorization (NMF), Correlated Topic Model (CTM), Dynamic Topic Model (DTM) and Latent Dirichlet Allocation (LDA) (Vayansky & Kumar, Citation2020).
The LDA model has been extensively utilized to reveal concealed thematic structures, facilitate document classification, and provide insights into the content of extensive datasets of unstructured text (Jelodar et al., Citation2019). LDA modeling extends beyond natural language documents to support essential software engineering tasks by facilitating software textual retrieval and analysis (Panichella et al., Citation2013). In processing software text data, employing a search-based software engineering tool can effectively mitigate LDA topic instability (Agrawal et al., Citation2018). This three-level hierarchical Bayesian model posits that documents are viewed as mixtures of topics, with topics representing word distributions of words (Blei et al., Citation2003). Each topic generated by LDA serves as an attribute, and associated words play a pivotal role in characterizing these attributes (Poria et al., Citation2016).
Compared to traditional methods of topic extraction involving high-frequency word counting and manual classification, LDA topic modeling presents advantages in terms of objectivity, efficiency, and comprehensiveness. It enables a more precise elucidation of topic information within natural language text data (Kirilenko et al., Citation2021). The widespread popularity of the LDA model in tourism research is evident, proving invaluable in extracting topics that capture tourists’ attention within a destination (Q. Chen et al., Citation2023). This capability greatly aids in defining destination image attributes, enhancing reliability, and holding the potential to introduce new theoretical perspectives (X. Chen et al., Citation2021).
Scholars have employed LDA modeling to analyze destination images from both static and dynamic perspectives. From a static perspective, researchers have examined destination images without considering potential changes over time. For example, they identified the attributes of destination images or compared images from multiple destinations (Peng et al., Citation2022; Ren & Hong, Citation2017; Taecharungroj & Mathayomchan, Citation2019). Conversely, from a dynamic perspective, changes in destination images over time have been investigated in separate studies. These included examining public attitudes towards the post-earthquake recovery of the Indonesian islands, investigating shifts in international tourists’ perceptions of a South Korean island during two distinct periods, and comparing destination images before and after tourists traveled to Jiuzhaigou, China (K. Kim et al., Citation2019; Wang et al., Citation2021; Y. Yan et al., Citation2020).
2.3. Sentiment analysis in destination image research
Sentiment analysis has emerged as an active and pivotal research domain within the realm of text mining and constitutes a fundamental branch of natural language processing (Guerreiro & Rita, Citation2020). Its core function involves computing emotional nuances embedded in textual content, thereby facilitating the identification and comprehension of expressed sentiments (González-Rodríguez et al., Citation2016). A more nuanced definition of sentiment analysis involves discerning the overall contextual polarity, including positive, neutral, or negative orientations embedded in UGC (Alaei et al., Citation2019). The surge in online data highlights the methodological advantages of sentiment analysis, which provides both cost-effectiveness and heightened convenience over traditional manual analysis approaches (Mehraliyev et al., Citation2022).
Sentiment analysis encompasses two primary methods: machine-learning algorithms and lexicon-based algorithms (Mehraliyev et al., Citation2022). Machine-learning algorithms utilize manually annotated feature vectors and datasets for classifier training. Researchers have leveraged pre-trained algorithms trained on similar datasets in related domains (Kirilenko et al., Citation2018). Lexicon-based algorithms rely on pre-established sentiment dictionaries that contain emotion-related words and their corresponding sentiment polarities. The efficacy of these methods is contingent on the alignment between the domain of the analyzed data and the sentiment lexicon (Mehraliyev et al., Citation2022). In this approach, individual words are assigned sentiment scores, and the text’s cumulative score is calculated based on these scores. Unlike machine-learning algorithms, which require substantial annotated data for effective training and often face challenges in generalizing to new datasets, lexicon-based algorithms are becoming more prevalent in tourism research (González-Rodríguez et al., Citation2016).
Sentiment analysis is widely used to analyze online travel reviews, providing valuable insights into tourist satisfaction and forming affective destination images (Ali et al., Citation2021). Additionally, sentiment analysis has been combined with topic extraction to analyze destination images, with topics representing the cognitive image identified through the counting of high-frequency words and subsequent manual classification (Jiang et al., Citation2021). Compared to LDA modeling, this method of topic extraction is less efficient and more costly for processing large-scale text data, potentially overlooking certain topics.
Furthermore, J. Chen et al. (Citation2022) integrated cognitive and affective images in importance-performance analysis, demonstrating the effectiveness of this approach in fostering a comprehensive understanding of destination images. As highlighted by Steven Pike and Kotsi (Citation2018), an appealing destination is characterized by a positive image, shaped by attributes deemed importance by tourists. Analyzing the importance and performance of these attributes can offer valuable insights for destination marketing strategies.
2.4. Research gap
Firstly, despite being the predominant framework for cross-cultural analysis in tourism research, Hofstede’s theory is rarely utilized in the examination of cognitive and affective destination images. The viability of utilizing Hofstede’s theoretical framework to elucidate cross-cultural disparities in destination image remains unverified.
Secondly, LDA modeling and sentiment analysis methods have been employed separately to analyze UGC data but are seldom integrated. The topics extracted from the LDA model serve as destination image attributes, illustrating tourists’ cognitive perceptions (Peng et al., Citation2022). Based on these topics, sentiment analysis can probe tourists’ affective impressions of these attributes, leading to a deeper exploration of destination images. However, this domain has not been well researched yet.
Thirdly, the burgeoning China’s giant panda tourism market exhibits substantial growth potential, with the Panda Base emerging as the foremost attraction. Nevertheless, the absence of pertinent research poses significant challenges for destination managers in devising international marketing strategies. Furthermore, as most destination image studies concentrate on countries or cities, there is a pressing need to broaden research scope to include attractions (Y. Li et al., Citation2023).
This study endeavors to bridge these gaps by employing the Panda Base as a case study, integrating LDA modeling and sentiment analysis for UGC data mining, and examining destination image through a cross-cultural perspective. It aims to address three key questions:
Can LDA modeling and topic-based sentiment analysis effectively evaluate destination images at the attraction level?
Are significant cross-cultural disparities evident in destination images between English and Chinese reviews?
How can destination marketing strategies be customized to reach tourists with diverse linguistic backgrounds?
Gartner (Citation1994) outlined three distinct dimensions of destination images, among which conative images represent tourists’ behavioral tendencies and intentions toward the destination, including their decisions to choose a destination and their likelihood of revisiting it (Pike & Ryan, Citation2004). However, extracting such nuanced information directly from UGC poses significant challenges. Consequently, traditional research methods such as surveys conducted through questionnaires or interviews remain the primary means of investigating conative images (Gorji et al., Citation2023; Woosnam et al., Citation2020). UGC data mining proves effective in analyzing cognitive and affective images, acknowledged as the primary dimensions of the overall destination image (Jiang et al., Citation2021; Yang et al., Citation2022). Accordingly, this research will focus on exploring the cognitive and affective aspects of destination image.
3. Methodology
3.1. Research framework
The research framework of this study, outlined in , unfolds across four distinct phases.
In the initial stage, the original English and Chinese reviews from the Panda Base attraction page on designated websites were collected. Subsequently, all original Chinese reviews were translated into English. This translation process is a standard procedure for analyzing texts across various languages, especially for conducting comparative analyses and preparing research publications in English (Lee & Park, Citation2023; Xi et al., Citation2022). Pre-processing involved procedures including tokenization, removal of HTML tags, numbers, punctuation, and stop words, as well as lowercasing the letters.
For the second stage, the preprocessed data, categorized as English reviews (original text in English) and Chinese reviews (original text in Chinese), were subjected to the LDA model. Taking English reviews as an example, the LDA model generated both word-topic matrix and document-topic matrix. Subsequently, the word-topic matrix facilitated the labeling of each topic using the associated extracted words. The importance of each topic was then determined based on its probability in the document-topic matrix. In this context, the term document pertains to a review.
In the third stage, topic-based sentiment analysis was conducted using English reviews as an illustrative example. The sentiment scores for each review were computed. Because a review may be linked to multiple topics, a threshold was established based on the number of topics. If the probability of a topic corresponding to a review surpassed the threshold, the review was considered to contain that topic. The comprehensive sentiment score for each topic was then calculated based on the sentiment scores of all the reviews for each topic.
In the fourth stage, a comparative analysis was conducted between English and Chinese reviews. Initially, the authors compared the distribution and importance of topics, with the importance value determined by the topic intensity. Subsequently, the performance of each topic was computed based on the sentiment score. Finally, importance-performance analysis was applied to comprehensively contrast the destination image portrayed in English and Chinese reviews.
3.2. Data collection and pre-processing
This study employed a customized web crawler to systematically collect tourist reviews pertaining to the Panda Base from prominent online travel platforms, including TripAdvisor, Google Maps, and Ctrip. TripAdvisor, recognized as one of the world’s largest online travel agencies, facilitates users to share travel experiences, compose reviews, and provide ratings for a diverse range of entities, such as attractions, hotels, and restaurants (Ali et al., Citation2021). Google Maps, a mapping service offered by Google, provides valuable location-specific information. Although Google Maps is not exclusively tailored for travel, its extensive user base for mapping services generates a substantial volume of tourism-related review data (Munawir et al., Citation2019). Both TripAdvisor and Google Maps are widely used international online platforms for tourists, and their reviews can be accessible in multiple languages, enhancing the applicability of the data for tourism research. Ctrip, China’s foremost online travel agency boasting the country’s largest user base, plays a pivotal role as a prominent travel review platform in China (Ren & Hong, Citation2017). The reviews on Ctrip are exclusively composed in Chinese. Ctrip has emerged as a crucial online data source for numerous studies in the domain of destination research within China, and previous research underscores the reliability of Ctrip as a data source (Y. Li et al., Citation2023).
The reviews collected in this study were posted between January 1, 2011, and December 15, 2023. The collection period included the period of the Covid-19 pandemic. This timeframe witnessed a significant decline in both domestic and international tourist numbers owing to China’s stringent crisis prevention and management measures, resulting in a comparatively low volume of tourist reviews. Specifically, this study defined the period around the Covid-19 pandemic from December 31, 2019 (a viral pneumonia case reported on the official website of the Wuhan city government) to December 7, 2022 (when China’s State Council issued new COVID-19 prevention and control measures). For instance, on TripAdvisor during this period, there were 81 reviews about the Panda Base, accounting for only 1.24% of all reviews from January 1, 2011, to December 15, 2023. Furthermore, studies indicate that although tourists may harbor negative sentiments during external threats, their reviews seldom address these concerns directly. Instead, they predominantly focus on evaluating the destination’s services, attributed to a rational acknowledgment of external threats beyond the destination’s control (Hu et al., Citation2021; Zenker & Kock, Citation2020). Hence, this study retained online reviews posted during the Covid-19 pandemic.
This study classified tourist groups based on language rather than nationality. Tourists who contribute reviews in a specific language may be proficient in other languages; however, their primary cultural influence is derived from their predominant language (Perez-Arce, Citation1999). To streamline the identification of tourist groups based on language, those who submit reviews in English are referred to as English-speaking tourists, while those who post reviews in Chinese are denoted as Chinese-speaking tourists.
The English reviews, sourced from TripAdvisor and Google Maps, have a total of 5,942 reviews, with 4,763 from TripAdvisor and 1,179 from Google Maps, forming the review samples in English. Chinese reviews encompassing both traditional and simplified Chinese were collected from TripAdvisor, Google Maps, and Ctrip. Among these, 1,310 reviews were from TripAdvisor, 178 from Google Maps, and 4,571 from Ctrip, totaling 6,059 and constituting the review samples in Chinese. To ensure data quality, invalid and duplicate reviews were removed, resulting in 5,913 English and 6,026 Chinese reviews. For Chinese-to-English translation, Google Translate, a widely used machine translation service was employed, which is known for its reliability in text mining analytics (Kirilenko et al., Citation2021; Lee & Park, Citation2023). To improve the translation accuracy, 5% of the reviews were randomly selected for manual inspection by the authors, and translation errors in certain proper nouns were rectified. Upon identifying errors in the translation, all similar errors in the text data were corrected. For instance, ‘flower flower’ was corrected to the Chinese pinyin ‘huahua’, the name of a nationally renowned panda. Text pre-processing was performed using Python’s Natural Language Toolkit (NLTK) (Bird et al., Citation2009), involving tasks including tokenization, removal of numbers, punctuation, and stop words, as well as converting letters to lowercase. The effectiveness of NLTK in English text processing in the tourism industry has been validated (Q. Chen et al., Citation2023; Lin et al., Citation2021).
3.3. LDA modeling for topic extraction
Python Gensim Toolkit was employed to implement the LDA model for topic extraction, as depicted in . Each review, denoted as the m-th review, generates a topic probability distribution denoted as θm ∼ Dirichlet(α). Here, θm represents the topic probability distribution for the m-th review, and α is the Dirichlet prior parameter for the multinomial distribution of each topic. Similarly, each topic (indexed as k) generates a probability distribution of words, denoted by φk ∼ Dirichlet(β). In this context, φk signifies the probability of words for the k-th topic, and β acts as the Dirichlet prior for the multinomial distribution associated with words linked to a topic. The parameters for testing, Zm,n ∼ Multinomial(θ) and Wm,n ∼ Multinomial(φZm,n), signify the generated topics and words, respectively.
In this model, hyperparameter K, signifying the number of topics, plays a crucial role in shaping the ultimate output results. The optimal number of topics needs to be determined prior to initiating the LDA model. When considering the selection of hyperparameter K, perplexity emerges as a valuable metric, serving as a reference for assessing the model’s performance (Blei et al., Citation2003). Perplexity reflects the model’s predictive capability for unseen text data, indicating the difficulty the model faces in predicting new textual information given its trained parameters. Lower perplexity scores are often indicative of a model that generalizes well to unseen textual data (Cao et al., Citation2009). Typically, the perplexity score declines with an increase in the number of topics but may rise again at a certain point. This is because more topics lead to increased overfitting, reducing the predictive performance on unseen text (L. Huang et al., Citation2017).
Drawing from relevant literature (Eid et al., Citation2019; Pike, Citation2002), this study defined the range of the number of topics, K, as [1, 15], and calculated the perplexity score for different values of K. Subsequently, the perplexity curve was generated using the Matplotlib data visualization package in Python, facilitating the observation of perplexity trends. Building upon the insights provided by Tang et al. (Citation2014), Kirilenko et al. (Citation2021) and X. Chen et al. (Citation2021), the number of topics corresponding to the elbow-like point on the perplexity curve is suggested to represent the optimal number of topics.
Afterward, the optimal number of topics to be determined was substituted into the LDA model for testing. The PyLDAvis package in gensim was utilized to generate the topic distance map, visually representing the topic distribution. Within the topic distance map, each circle signifies a distinct topic, and the size of the circle corresponds to the popularity of each topic. The proximity on the map indicates similarity in topic distributions across the documents. When clear boundaries exhibit good independence and minimal overlap between topics, it suggests that the selection of the K value may be appropriate (Sievert & Shirley, Citation2014).
By integrating insights from the perplexity curve and topic distance map, the optimal number of topics was determined. Specifically, when processing English reviews, setting K to 7 revealed an elbow-like point on the perplexity curve and a clear differentiation between topics in the topic distance map. A comparable scenario arose when exploring the optimal K value for Chinese reviews. Thus, for both English and Chinese reviews, the optimal number of topics, K, was determined to be 7.
Subsequently, LDA modeling was performed, and the word-topic matrix and document-topic matrix were generated. The word-topic matrix illustrates the relationship between each topic and the words, representing the probability distribution of each word for each topic. Meanwhile, the document-topic matrix unveils the relationship between individual documents and topics, depicting the probability distribution of each topic within every document.
Based on the work by Maier et al. (Citation2021), the authors examined the list of keywords defining a latent topic. The semantic characteristics of these words were analyzed to identify the most appropriate name representing the topic’s content. Subsequently, the topic names were manually summarized and each topic was labeled accordingly.
The perplexity curves for English and Chinese reviews are depicted in and , respectively. Similarly, the topic distance maps for English and Chinese reviews are illustrated in and , respectively. In and , the top 30 words with the highest probabilities associated with a topic are shown, providing insight into the internal structure of that topic. In , Topic 2 attracts the highest level of attention among English-speaking tourists, followed by Topic 4, whereas Topics 5 and 6 receive the least popularity. The proximity between Topic 2, Topic 4, and Topic 7 suggests a higher similarity in the distribution of these three topics across the documents compared to other topics. In , Topic 6 and Topic 5 are particularly popular among Chinese-speaking tourists. Topic 3 and Topic 4 show close proximity and partial overlap, indicating a high degree of similarity in their distribution across the documents. The labeled names of the topics corresponding to the numbers in the figures are provided below each figure.
Figure 5. Topic distance map for English reviews (Notes: 1. Living Environment of Pandas, 2. Pandas and Breeding, 3. Crowd and Weather, 4. Location and Transportation, 5. Tour Guide Service, 6. Tickets, Souvenirs and Catering Service, 7. Information Service and Volunteer).
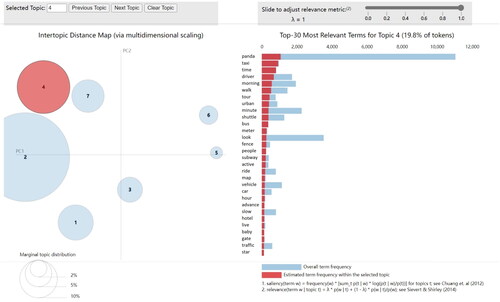
Figure 6. Topic distance map for Chinese reviews (Notes: 1. Parent-child Experience, 2. Tickets, Souvenirs and Catering Service, 3. Views and Travel Routes, 4. Location and Transportation, 5. Crowd and Weather, 6. Pandas and Breeding, 7. Tour Guide Service).
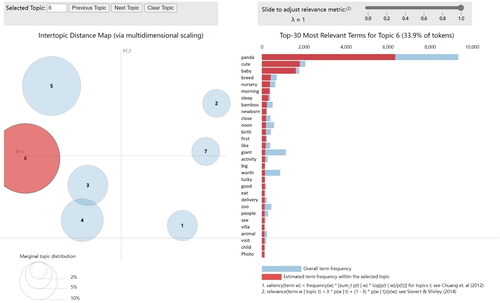
Upon completing the topic extraction process, the importance of each topic was assessed according to its probability in the document-topic matrix.
3.4. Sentiment analysis and topic assignment
This study employed the Valence Aware Dictionary and sEntiment Reasoner (VADER) from the NLTK library for sentiment analysis. VADER functions as both a sentiment lexicon and an analysis tool to assess the emotional tone of text and determine sentiment polarity (positive, neutral, or negative) (Elbagir & Yang, Citation2019). Utilizing lexicon-based analysis, rules, and contextual considerations, VADER ensures the accurate sentiment analysis of English text data. It is considered the gold standard for lexicon-based sentiment analysis across various text types (Ribeiro et al., Citation2016). Moreover, it excels in analyzing social media texts and online reviews, with its lexicon encompassing tourism-related vocabulary, making it appropriate for the tourism field (Alaei et al., Citation2019).
VADER calculates sentiment polarity scores for each review, ranging from −1 (negative) to 1 (positive). A higher absolute value indicates a stronger sentiment. Because reviews may relate to multiple topics, they are assigned to topics based on a defined threshold value (Ren & Hong, Citation2017). Establishing μ = 1/K (where K is the number of topics) determines the affiliation of a review with a particular topic. Based on the document-topic matrix, if the probability of a topic corresponding to a review is greater than or equal to μ, the review is allocated to that topic, thereby permitting multiple topic assignments. The average sentiment score of all reviews assigned to each topic was then calculated to provide a sentiment score for each topic.
The topics and sentiment scores from the reviews were analyzed. Based on these findings and the current management measures, targeted marketing strategies were proposed. This study received ethical approval from the Chengdu University Research Ethics Committee. Written informed consent was obtained from participants involved in this work.
4. Results
4.1. Topic analysis
4.1.1. Extracted topics in English and Chinese reviews
LDA modeling was conducted separately on English and Chinese reviews, resulting in the extraction of 7 topics in both datasets. To address the potential noise in the extracted topics, the authors performed a manual extraction process to pinpoint 10 topic keywords. This selection was based on the probability of words within a given topic and distinctiveness of words associated with other topics. These topic keywords serve as vital elements for interpreting the topic content, facilitating a nuanced characterization of destination image attributes.
The method proposed for assessing topic importance draws upon salience theory, which posits that individuals are inclined to allocate greater attention to specific stimuli or information based on their perceived relevance, significance, or prominence in a given context (Bordalo et al., Citation2013). This theory underscores the influence of perceived importance on individuals’ attention and memory. In the context of this study, the frequency of discussion among tourists regarding a particular topic reflects their level of concern, thereby indicating the perceived importance of that topic. In other words, topics that are more salient in the reviews are considered more important. This aligns with the findings of Steven Pike and Kotsi (Citation2018), who regarded destination image attributes that are more salient carry greater importance during importance-performance analyses.
To operationalize the evaluation of importance, the metric of topic intensity was introduced. According to X. Li et al. (Citation2023), topic intensity refers to the average probability value of a given topic across all documents. It serves as an indicator of the topic’s relative frequency in discussions within all documents. EquationEquation (1)(1)
(1) was used to calculate the topic intensity.
represents the intensity of topic z;
signifies the probability of topic z within document d; and D represents the total number of documents in the corpus.
(1)
(1)
Given that the importance scale must span from 0 to 5, with 5 indicating the highest importance (Lai & Hitchcock, Citation2015), EquationEq. (2)(2)
(2) was formulated to calibrate the scale. In this equation,
signifies the importance of topic z and
represents the maximum value of
.
(2)
(2)
The extracted topics, their corresponding topic keywords, topic intensity, and importance in both English and Chinese reviews are presented in and , respectively.
Table 1. Topics extracted from English reviews.
Table 2. Topics extracted from Chinese reviews.
4.1.2. Topic comparison between English and Chinese reviews
Seven topics are extracted from reviews in both languages, and five of them exhibit consistency, namely ‘Pandas and Breeding’, ‘Location and Transportation’, ‘Tickets, Souvenirs and Catering Service’, ‘Tour Guide Service’, and ‘Crowd and Weather’. Significantly, ‘Pandas and Breeding’ stands out as the most crucial topic in reviews in both languages. However, the importance of the other four topics varies between the two languages. In English reviews, the importance of ‘Location and Transportation’ surpasses that of ‘Crowd and Weather’, ‘Tickets, Souvenirs and Catering Service’, and ‘Tour Guide Service’. Conversely, in Chinese reviews, ‘Crowd and Weather’ garners significantly more attention than the other three topics. Irrespective of language, ‘Tickets, Souvenirs and Catering Service’ and ‘Tour Guide Service’ are consistently deemed the least important topics.
There is a high degree of similarity for the most crucial topic, indicating shared interest among tourists. In the ‘Location and Transportation’ topic, English reviews frequently mention concerns about taxi drivers using meters, emphasizing potential rip-offs, whereas Chinese reviews focus on location specifics such as parking lots, parking capacity, and bus transfers. This discrepancy may stem from English-speaking tourists predominantly relying on taxis, whereas Chinese-speaking tourists enjoy more flexible transportation choices. Regarding ‘Tickets, Souvenirs, and Catering Service’, English reviews prioritize payment methods, cafes, and Western food, while Chinese reviews emphasize the convenience of purchasing tickets and cost-effectiveness. In terms of ‘Tour Guide Service’, English reviews emphasize English services, panda films, and tour guide interactivity, whereas Chinese reviews focus on the professionalism of introductions and the communication of scientific knowledge. Regarding ‘Crowd and Weather’, both languages highlight the number of tourists and weather impact, with English reviews delving into crowd noise and local behavior, while Chinese reviews specifically address queuing chaos caused by a popular panda named Huahua in Chinese.
Two distinct topics emerged from English reviews: ‘Living Environment of Pandas’ and ‘Information Service and Volunteer’. English-speaking tourists express concerns about animal welfare, website updates, donations, and volunteer programs, emphasizing opportunities to care for and interact with pandas. In Chinese reviews, two unique topics were extracted: ‘Views and Travel Routes’ and ‘Parent-child Experience’. Chinese-speaking tourists tend to focus on natural landscapes, visiting routes, and clear signs. There is a notable emphasis on parent-child experiences, including stroller-friendliness and hygiene of associated facilities. The importance of family-oriented tourism is evident among Chinese-speaking tourists.
4.2. Topic-based sentiment analysis
In the LDA modeling framework, the number of topics is determined at K = 7, establishing a threshold of μ = 1/7 to determine the allocation of a review to a specific topic. Based on the document-topic matrix, a review is assigned to a particular topic if the associated probability is equal to or exceeds 1/7, thereby allowing multiple topic assignments for a single review. To compute the sentiment score for each topic, the authors employed EquationEq. (3)(3)
(3) to calculate the average sentiment score of all reviews assigned to that topic.
(3)
(3)
In the context of this study, variable i signifies each review within the corpus, whereas j represents each topic in the LDA model. Variable denotes the number of reviews assigned to the j-th topic, and
signifies the sentiment polarity score calculated by VADER for the i-th review within the j-th topic. Given that each review is associated with only one sentiment polarity score irrespective of the assigned topic, its value remains constant. Additionally,
signifies the average sentiment score for all reviews allocated to the j-th topic, representing the sentiment score specifically associated with topic j.
In this study, the assessment of topic performance was conducted by computing sentiment scores obtained from sentiment analysis. These sentiment scores, related to each topic, provide valuable insights into tourists’ satisfaction with various aspects of the destination image. As highlighted by J. Chen et al. (Citation2022), this approach indirectly assesses topic performance. Specifically, higher sentiment scores indicate more positive evaluations by tourists regarding the topic. Consequently, there exists a positive correlation between sentiment scores and topic performance, with higher sentiment scores reflecting better performance of the respective topic.
The sentiment polarity score typically spans from −1 to 1. However, aligning with the prevailing practice in the literature, where a 5-point scale is commonly employed for both importance and performance assessments (Lai & Hitchcock, Citation2015), the authors adjusted the performance scale to extend it from 0 to 5. This adjustment was executed using EquationEq. (4)(4)
(4) , where
represents the performance of topic j.
(4)
(4)
displays sentiment scores and compares topic performance between reviews in both languages. The average sentiment score from English reviews is higher than that from Chinese reviews, with only a 0.11 gap between the highest and lowest scores within English reviews. In contrast, the gap reaches 0.23 in Chinese reviews. This indicates that English-speaking tourists tend to have a higher satisfaction level and are more uniformly satisfied with each attribute of the destination image.
Table 3. Topic performance in English and Chinese reviews.
In English reviews, the topics ‘Pandas and Breeding’, ‘Location and Transportation’, and ‘Living Environment of Pandas’ represent the highest sentiment scores, while the topic ‘Information Service and Volunteer’ attains the lowest score. However, even with the lowest score, it still surpasses the overall average score of 0.68 for Chinese reviews. In Chinese reviews, the topic ‘Location and Transportation’ has the highest score, followed by ‘Parent-child Experience’ and ‘Tickets, Souvenirs, and Catering Service’. Conversely, the topic ‘Crowd and Weather’ receives the lowest score.
4.3. Importance-performance analysis
presents the topics extracted from the LDA model applied to English reviews, along with the associated keywords, intensity, and importance of each topic. Similarly, displays the topics obtained from processing Chinese reviews with the LDA model, including keywords, intensity, and importance. Following the LDA modeling results, sentiment analysis was conducted on all documents under each topic, and topic performance was assessed based on sentiment scores. To enable comparison, topics, sentiment scores, and topic performance from both English and Chinese reviews are consolidated into .
Leveraging the topic importance values from and alongside the topic performance values in , the importance-performance analysis framework was employed to depict the destination image reflected in both English and Chinese reviews. As previously mentioned, the topics derived from the LDA model can be interpreted as attributes of the destination image (Peng et al., Citation2022). The importance of each attribute represents the cognitive image of the destination, while the performance of each attribute reflects the affective image. Therefore, and illustrate the destination images as portrayed in English and Chinese reviews, respectively.
In and , the importance value of each attribute is plotted on the x-axis, and the performance value on the y-axis. The four quadrants in each figure have distinct implications (Martilla & James, Citation1977). For convenience, Quadrant 1 is labeled as Q1, Quadrant 2 as Q2, Quadrant 3 as Q3, and Quadrant 4 as Q4. Attributes situated in Q1 signify the destination’s competitive advantages, requiring continuous efforts for maintenance and reinforcement. Those in the Q2 indicate the destination’s potential, which should be given increased importance to further develop into a strength. Attributes in Q3 require relatively less attention, while those in Q4 highlight aspects that demand the destination’s immediate and utmost strengthening.
illustrates that ‘Pandas and Breeding’ and ‘Location and Transportation’ are two crucial attributes situated in Q1. Continual improvement of these two attributes is pivotal for maintaining a positive destination image. Moreover, ‘Pandas and Breeding’ demands special attention because of its substantial advantage, rendering it the most attractive for tourists. Attributes such as ‘Living Environment of Pandas’ and ‘Tickets, Souvenirs, and Catering Service’ are positioned in Q2. Strategic marketing adjustments may be required to raise awareness of these attributes among tourists. Among the three attributes in the Q3, special attention is warranted for ‘Information Service and Volunteer’ because of its relatively high importance but the poorest performance. Negative reviews of this attribute highlight issues with the destination’s English website information, ineffective responses to email or phone inquiries, and disappointment with canceled volunteer programs.
reveals that ‘Pandas and Breeding’ is in the Q1 but is in close proximity to Q4, suggesting that the destination might need to enhance the performance of this attribute. ‘Location and Transportation’, ‘Parent-child Experience’, and ‘Tickets, Souvenirs, and Catering Service’ are situated in the Q2. Strategic marketing adaptations may be necessary to enhance tourist awareness of these attributes and facilitate their movement to the Q1. Special attention is required for ‘Crowd and Weather’ in the Q4, signifying the need for urgent improvement.
5. Discussion
The data analysis results highlight disparities in the destination image perceived by predominantly English-speaking tourists compared to predominantly Chinese-speaking tourists. For tourism destinations venturing into international markets, conducting cross-cultural analyses is imperative to profoundly comprehend tourists’ characteristics (J. Li & Lu, Citation2016). English and Chinese exert substantial global influence, each intricately intertwined with its respective influential cultural backdrop (G. Zhang et al., Citation2022). This study employs Hofstede’s framework to elucidate variations in destination image among tourists from these two linguistic backgrounds.
Destination image attributes unique to English-speaking tourists encompass ‘Living Environment of Pandas’ and ‘Information Service and Volunteer’, while those unique to Chinese-speaking tourists include ‘Parent-child Experience’ and ‘Views and Travel Routes’. These findings can be interpreted through the lens of Hofstede’s ‘Individualism vs. Collectivism’ dimension. Given the inclination towards individualism in the culture, English-speaking tourists exhibit a heightened focus on the rights and interests of individuals (Manrai et al., Citation2019). Consequently, they are more inclined to view animal welfare and sustainable environmental practices as integral components of their personal values and responsibilities. Moreover, the emphasis on personal expression and self-presentation in English culture is reflected in their interest in volunteer programs, providing an avenue for expressing individual values and sense of responsibility. This observation is in line with the findings reported by C. Kim and Lee (Citation2020). In contrast, Chinese culture, with its collectivistic tendencies, prioritizes social relationships and interpersonal interactions, evident in the emphasis on family-oriented activities such as the ‘Parent-child Experience’ in travel. This is consistent with Confucian influences, underscoring the significance of family reunions and educational factors in influencing travel decisions (S. S. Huang & Wen, Citation2021).
In addition, English-speaking tourists express concern with information services, possibly due to the inconvenience of accessing English information about the destination or the perceived inadequacy of online and offline English services. Notably, while the Panda Base maintains an English website, its update frequency significantly lags behind that of the Chinese website. In contrast, Chinese-speaking tourists encounter no such obstacles, as they can effortlessly access a diverse array of information disseminated by the destination through WeChat public accounts.
The shared attributes of destination image for both English- and Chinese-speaking tourists can be explained by Hofstede’s ‘Uncertainty Avoidance Index’ and ‘Power Distance Index’. Notably, ‘Crowd and Weather’ ranks fifth in terms of the importance of destination image attributes for English-speaking tourists, while holding the second position among Chinese-speaking tourists. This discrepancy suggests a greater concern among Chinese-speaking tourists regarding the impact of ‘Crowd and Weather’ on their travel experiences compared to their English-speaking counterparts. In line with the findings of Quintal et al. (Citation2010) and Q. Chen et al. (Citation2023), the low Uncertainty Avoidance Index observed in English culture suggests that English-speaking tourists exhibit a greater acceptance of uncertainty, adaptability to change, and willingness to take risks. Conversely, the high Uncertainty Avoidance Index in Chinese culture highlights a preference for order, stability, and avoidance of uncertain situations. This inclination is also mirrored in the emphasis on travel routes evident in Chinese reviews.
Moreover, despite ‘Tour Guide Service’ ranking lowest in importance among both groups, English-speaking tourists assigned a significantly lower importance value (0.12) compared to Chinese-speaking tourists (0.82). This contrast is indicative of the low Power Distance Index in English culture, suggesting a tendency towards individual independence and autonomous decision-making, thereby diminishing reliance on tour guide services. Conversely, the high Power Distance Index in Chinese culture implies deference to authority, with Chinese-speaking tourists placing greater value on tour guide services for professional information and directional guidance. This observation aligns with the findings of Mok and Defranco (Citation2000).
The English-speaking tourists showed higher average sentiment scores for destination image attributes than Chinese-speaking tourists, indicating greater satisfaction with the Panda Base. This observation can potentially be elucidated through Hofstede’s cultural dimension of ‘Indulgence vs. Restraint’. English culture tends to lean towards ‘Indulgence’, where individuals actively pursue happiness and express positive emotions openly and enthusiastically (He & Filimonau, Citation2020). In contrast, Chinese culture often embodies ‘Restraint’, emphasizing moderation and expressing positive emotions more indirectly, cautiously, and conservatively. The cultural inclination towards implicitness and euphemism may consequently lead to a comparatively lower level of satisfaction expressed in Chinese reviews. This observation can also be interpreted through an alternative dimension. As outlined by De La Hoz-Correa and Muñoz-Leiva (Citation2019), tourists from cultures characterized by high Uncertainty Avoidance tend to hold a more positive affective image of destinations.
Differences in tourists’ travel expectations may also contribute to this variation (C.-F. Chen & Phou, Citation2013). Given the status of pandas as national treasures in China and their frequent portrayal in a favorable light by Chinese media, Chinese-speaking tourists might hold higher expectations for the destination compared to their English-speaking counterparts. When the gap between the actual travel experience and these heightened expectations is substantial, it can lead to a reduction in overall travel satisfaction.
However, this finding diverges from the conclusions drawn in Xi et al. (Citation2022), which suggests that Chinese-speaking tourists tend to be more positive than English-speaking tourists in their online reviews on Airbnb. The divergence in evaluations by the two groups of tourists may be attributed to the nature of the objects under evaluation. Unlike reviewing attractions, assessing accommodation involves evaluating a specific individual—the host. The communication between guests and hosts may foster emotional interactions, which could contribute to the amplification of positivity in reviews, reflecting Chinese culture’s emphasis on interpersonal relationships (Tam & Bond, Citation2002).
Moreover, Chinese tourists showed the lowest sentiment score with the destination image attribute ‘Crowd and Weather’. While tourists consider crowd levels as a crucial aspect, the destination’s performance seems to disappoint them. This may be attributed to the tendency of Chinese-speaking tourists to travel during long national holidays, resulting in heightened tourist congestion. The resulting overcrowding and prolonged queues can contribute to an unpleasant travel experience, exacerbated by unfavorable weather conditions, further diminishing tourist satisfaction. Topic keywords like ‘queue’, ‘hot’, ‘rain’, and ‘tired’ reflect tourists’ concerns. Without improvements by destination managers, this issue could continue to perpetuate a negative impact on the overall destination image.
6. Conclusions
6.1. Academic contributions
This study investigates the destination image of the Panda Base by employing text data mining of online travel reviews, addressing a research gap in the emerging panda tourism market. It showcases the adaptability and effectiveness of a methodology that combines LDA modeling with topic-based sentiment analysis for destination image research, thus advancing research methodologies in this domain. Moreover, an innovative approach is introduced by utilizing language as a cultural identifier to gauge differences in cognitive and affective destination images across distinct language groups, transcending conventional nationality classifications and offering a fresh perspective for cross-cultural research. The utilization of the importance-performance analysis framework enriches the understanding and comparison of destination images perceived by tourists with diverse cultural backgrounds, thereby providing reliable support for the formulation of differentiated destination marketing strategies.
This research offers a versatile approach applicable to diverse research contexts, allowing analysis of destination image perceptions across different tourism markets and geographical regions, while also providing a valuable framework for cross-cultural research in tourism. This study contributes to the literature on tourism and consumer behavior by enriching the body of destination image research. The majority of this study’s findings can be elucidated through Hofstede’s theory or by considering the social environment in conjunction with topic keywords extracted through text mining. In this regard, this study also broadens the research perspective of cross-cultural tourism marketing.
6.2. Practical implications
This study emphasizes the importance of extracting insights from online travel reviews to guide marketing strategies in the tourism sector, highlighting the growing significance of big data analytics in managing destination image. Amidst the digital transformation and globalization of the tourism industry, destination marketing in emerging tourism markets encounters significant challenges. By offering practical recommendations for enhancing destination image and cross-cultural marketing strategies, this research benefits not only the stakeholders of the Panda Base but also other destination marketing organizations and relevant government departments responsible for tourism planning and management. These insights serve as valuable references for a broader tourism industry, enriching the understanding of market dynamics and tourist preferences.
Both English-speaking and Chinese-speaking tourists share a predominant interest in pandas and the associated breeding process. Consequently, prioritizing and enhancing strengths in this domain is paramount for augmenting the overall image of the Panda Base. To achieve this, it is necessary to consider the expansion of offerings related to pandas and create additional avenues for tourists to gain comprehensive insights into the intricacies of the breeding process. Furthermore, tailored marketing strategies should be developed, leveraging an understanding of cultural nuances and varied interests among the two groups.
6.2.1. Marketing strategies targeting English-speaking tourists
Several crucial considerations should be considered when targeting English-speaking tourists. Firstly, it is crucial to acknowledge the substantial concern within this demographic regarding animal welfare and environmental practices. Actively prioritizing and promoting ethical and sustainable initiatives not only aligns with the values of this group, but also holds the potential to capture their attention and foster loyalty as responsible tourists. Facilitating opportunities for donations and volunteer programs further provides avenues for expressing personal values and responsibilities. Furthermore, it is essential to emphasize the destination’s commitment to ecological and environmental sustainability as well as the opportunities it offers tourists to engage in caring for pandas. According to the volunteer program manager at the Panda Base, only long-term volunteers are recruited every six months. Introducing a short-term volunteer program and prominently featuring it in various promotional materials could attract more English-speaking tourists.
Secondly, catering to English-speaking tourists entails considering their preference for personal independence. Addressing this aspect effectively involves prioritizing engaging self-guided travel services. Enhancing the efficiency of car rental agencies and bolstering safety measures for car rental services are crucial measures in this regard. Furthermore, providing comprehensive and user-friendly information services is imperative. While the Panda Base has offered human tour guide services in English, the number of tourists served remains limited. Introducing smart technology, such as English audio guides, voice assistants, or simultaneous interpretation enhanced by AI, can significantly break the language barriers and provide different experiences to the tourists. Optimizing the design of the English website to provide detailed and accurate information ensures tourists have the necessary resources for seamless journey planning. Regularly updating the website and enhancing its appeal with interactive multimedia elements are crucial steps. Additionally, ensuring swift, accurate, and efficient responses to inquiries contributes to fostering independent exploration and self-guided experiences.
Thirdly, addressing concerns about potential taxi driver fraud is noteworthy for English-speaking tourists who prefer taxis as their primary mode of transportation. In this context, destination marketing organizations play a pivotal role by advocating regulatory measures from transportation authorities to ensure transparency in the taxi service market. Additionally, in promotional materials targeting English-speaking tourists, it is significant to standardize operations of the taxi service as well as enhance the destination’s superior location and convenience in transportation. This approach bolsters tourists’ confidence in their transportation options and alleviates any apprehensions about fraudulent activities.
6.2.2. Marketing strategies targeting Chinese-speaking tourists
The following key strategies are recommended to address the preferences of Chinese-speaking tourists. Firstly, prioritizing family-oriented activities and fostering parent-child experiences emerges as a critical aspect. Enhancing facilities, services, and activities tailored to family groups is highly recommended to achieve this objective. Intensifying marketing communication efforts with a specific focus on family-oriented experiences is essential, and promotional materials should highlight features designed to engage families, such as convenient transportation options, easy accessibility, stroller-friendly paths, and facilities catering to children’s needs. This approach aims to attract more Chinese-speaking tourists seeking family centric travel experience. Simultaneously, considering the noise concerns raised by English-speaking tourists, it is imperative to fortify guidance for civilized tours aimed at mitigating noise associated with children. This initiative is essential for cultivating a harmonious and enjoyable environment for tourists.
Secondly, it is imperative to acknowledge and cater to the cultural inclinations of Chinese-speaking tourists, who highly value respecting authority and minimizing uncertainty. Addressing this cultural trait necessitates the prioritization of professional tour guide services. This involves not only providing customized guiding services, but also offering in-depth information about the destination, emphasizing the delivery of scientific knowledge. Additionally, it is essential to ensure well-defined tour routes, necessitating the enhancement of infrastructure, including the improvement of road signage for clarity and presentation of recommended tour route options. This effort aims to guarantee a seamless and organized experience for this group of tourists.
Thirdly, addressing the concern of crowd density necessitates the implementation of effective crowd-control measures. Currently, the Panda Base utilizes an online reservation and ticket purchasing system. Tourists are required to input their identity information and purchase tickets before visiting. If the daily reservation limit is reached, the system will indicate that no tickets are available. According to the ticket manager at the Panda Base, the daily reservation limit was reduced from 84,000 in 2021 to 60,000 in 2023. Further reducing this number could enhance the travel experience for Chinese-speaking tourists. As an initial step towards accommodating a larger daily influx of tourists, expanding the attraction’s physical footprint is recommended. Moreover, enhancing the online system to provide real-time reservation numbers and information about potential peak times can facilitate tourists’ planning and assist them in avoiding overcrowding.
Additionally, recognizing the impact of weather conditions on the experiences of Chinese-speaking tourists, there is a pressing need to enhance the accessibility of indoor venues. This could involve creating more sheltered spaces that are less weather-dependent and improving indoor facilities. Furthermore, promotional materials should emphasize the destination’s preparations for various weather conditions to alleviate tourists’ concerns about weather affecting their travel experiences. Given the urgency of this aspect, immediate attention is required and should be accorded the highest priority.
7. Limitations
The present research acknowledges its limitations as follows:
Firstly, the scope of the data collection was confined to three prominent websites, which may not fully represent the diversity of online platforms where travel reviews are shared. The reliance on this limited set of sources introduces potential bias to the findings, and some websites filtering or restricting data displays poses a risk of incomplete information.
The second constraint pertains to the accuracy of text data translation. While the utilization of Google Translate is a practical choice, it is important to recognize that machine translation may not always capture nuances accurately, leading to potential errors or misinterpretations.
The third limitation arises from the time span of data collection in this study, wherein the destination’s image may have changed over time. The study’s reliance on a specific time frame may not capture the fluctuations in the destination’s image, particularly in response to dynamic factors such as seasonal variations or evolving tourism trends.
8. Future studies
The limitations will be addressed in subsequent research.
Firstly, future studies should aim to broaden the scope of data collection, particularly focusing on investigating cultural differences. This expansion in data collection could involve integrating more online platforms to collect travel reviews, as well as incorporating a wider range of destinations as study cases. By doing so, researchers can enhance the validity and generalizability of the research method employed in this study.
Secondly, future research can enhance the translation accuracy by enhancing manual inspection or incorporating more manual translation services to rectify machine translation errors. Furthermore, an alternative approach could include translating English into Chinese, employing tokenization tools suitable for processing Chinese, utilizing lexicons and sentiment analysis tools designed for Chinese, and subsequently comparing the results with those obtained in this study.
Thirdly, researchers could conduct longitudinal studies to track changes in destination image over time, thereby providing a more comprehensive understanding of its evolution. For instance, employing annual data as corpus units for LDA modeling enables the observation of trends in destination image alterations. Continuous dynamic monitoring facilitates a more profound analysis of destination image, offering valuable insights for making timely adjustments to destination marketing and management.
Author contribution statement
Zuo Wang and Piyachat Udomwong contributed to the conception and design of this study. Zuo Wang conducted the analysis, interpreted the data, and drafted the paper. Jing Fu and Pintusorn Onpium provided critical revisions for intellectual content. Piyachat Udomwong gave final approval for the version to be published. All authors agree to be accountable for all aspects of the work.
Acknowledgments
The authors gratefully acknowledge the support of the Graduate School and International College of Digital Innovation at Chiang Mai University.
Conflicts of interest
The authors declare no conflict of interest.
Disclosure statement
No potential competing interest was reported by the authors.
Data availability statement
The data is available on request from the corresponding author.
Additional information
Notes on contributors
Zuo Wang
Ms. Zuo Wang, currently pursuing a PhD in Digital Innovation and Financial Technology at Chiang Mai University, concurrently holds a position as an associate professor at the School of Tourism and Culture Industry at Chengdu University. Her expertise lies in the domains of digital media innovation, as well as tourism destination marketing and management.
Piyachat Udomwong
Dr. Piyachat Udomwong is a lecturer at the International College of Digital Innovation at Chiang Mai University. She earned her Ph.D. in Biology (Bioinformatics) from the University of York, UK, with a background in mathematics, thriving in multidisciplinary settings. Her research interests include discovering insights from a massive amount of data coming from different fields by using data analytics, machine learning, and statistical learning techniques.
Jing Fu
Dr. Jing Fu is an associate professor in the School of Tourism and Culture Industry at Chengdu University, specializing in smart tourism and hospitality, as well as knowledge management in the field of tourism and hospitality. She earned her Ph. D. in Knowledge Management from Chiang Mai University in 2012. Subsequently, she was a visiting scholar at the University of Lyon 2, France, and undertook an executive development program at the University of New Hampshire, USA. She conducted post-doc research at the Department of Tourism and Hospitality Management, School of Business Administration, International Hellenic University, Greece, between 2013 and 2014. During her sabbatical year in 2018–2019, she served as program manager at the Hong Kong Polytechnic University’s School of Hotel and Tourism Management.
Pintusorn Onpium
Dr. Pintusorn Onpium, a lecturer at Chiang Mai University’s International College of Digital Innovation, conducts research in cultural tourism management, as well as tourism planning and development. She earned her Ph.D. in Integrated Tourism Management from the National Institute of Development Administration, Thailand. She actively collaborates with the local community, fostering their growth by generating revenue and creating jobs through Community-Based Tourism (CBT) and cultural capital initiatives.
References
- Agrawal, A., Fu, W., & Menzies, T. (2018). What is wrong with topic modeling? And how to fix it using search-based software engineering. Information and Software Technology, 98, 1–24. https://doi.org/10.1016/j.infsof.2018.02.005
- Alaei, A. R., Becken, S., & Stantic, B. (2019). Sentiment analysis in tourism: capitalizing on big data. Journal of Travel Research, 58(2), 175–191. https://doi.org/10.1177/0047287517747753
- Albalawi, R., Yeap, T. H., & Benyoucef, M. (2020). Using topic modeling methods for short-text data: A comparative analysis. Frontiers in Artificial Intelligence, 3, 42. https://doi.org/10.3389/frai.2020.00042
- Ali, T., Marc, B., Omar, B., Soulaimane, K., & Larbi, S. (2021). Exploring destination’s negative e-reputation using aspect based sentiment analysis approach: Case of Marrakech destination on TripAdvisor. Tourism Management Perspectives, 40, 100892. https://doi.org/10.1016/j.tmp.2021.100892
- Arefieva, V., Egger, R., & Yu, J. (2021). A machine learning approach to cluster destination image on Instagram. Tourism Management, 85, 104318. https://doi.org/10.1016/j.tourman.2021.104318
- Baloglu, S., Henthorne, T. L., & Sahin, S. (2014). Destination image and brand personality of Jamaica: A model of tourist behavior. Journal of Travel & Tourism Marketing, 31(8), 1057–1070. https://doi.org/10.1080/10548408.2014.892468
- Beerli, A., & Martín, J. D. (2004). Tourists’ characteristics and the perceived image of tourist destinations: a quantitative analysis—A case study of Lanzarote, Spain. Tourism Management, 25(5), 623–636. https://doi.org/10.1016/j.tourman.2003.06.004
- Bird, S., Klein, E., & Loper, E. (2009). Natural language processing with Python: Analyzing text with the natural language toolkit. O’Reilly Media, Inc.
- Blei, D. M., Ng, A. Y., & Jordan, M. I. (2003). Latent Dirichlet allocation. Journal of Machine Learning Research, 3(Jan), 993–1022.
- Bonn, M. A., Joseph, S. M., & Dai, M. (2005). International versus domestic visitors: An examination of destination image perceptions. Journal of Travel Research, 43(3), 294–301. https://doi.org/10.1177/0047287504272033
- Bordalo, P., Gennaioli, N., & Shleifer, A. (2013). Salience and consumer choice. Journal of Political Economy, 121(5), 803–843. https://doi.org/10.1086/673885
- Bramwell, B., & Rawding, L. (1996). Tourism marketing images of industrial cities. Annals of Tourism Research, 23(1), 201–221. https://doi.org/10.1016/0160-7383(95)00061-5
- Buzova, D., Sanz-Blas, S., & Cervera-Taulet, A. (2019). Does culture affect sentiments expressed in cruise tours’ eWOM? The Service Industries Journal, 39(2), 154–173. https://doi.org/10.1080/02642069.2018.1476497
- Cao, J., Xia, T., Li, J., Zhang, Y., & Tang, S. (2009). A density-based method for adaptive LDA model selection. Neurocomputing, 72(7-9), 1775–1781. https://doi.org/10.1016/j.neucom.2008.06.011
- Chen, J., Becken, S., & Stantic, B. (2022). Assessing destination satisfaction by social media: An innovative approach using importance-performance analysis. Annals of Tourism Research, 93, 103371. https://doi.org/10.1016/j.annals.2022.103371
- Chen, X., Li, J., Han, W., & Liu, S. (2021). Urban tourism destination image perception based on LDA integrating social network and emotion analysis: the example of Wuhan. Sustainability, 14(1), 12. https://doi.org/10.3390/su14010012
- Chen, C.-F., & Phou, S. (2013). A closer look at destination: Image, personality, relationship and loyalty. Tourism Management, 36, 269–278. https://doi.org/10.1016/j.tourman.2012.11.015
- Chen, Q., Xu, S., Liu, R., & Jiang, Q. (2023). Exploring the discrepancy between projected and perceived destination images: A cross-cultural and sustainable analysis using LDA modeling. Sustainability, 15(12), 9296. https://doi.org/10.3390/su15129296
- Cronjé, D. F., & Du Plessis, E. (2020). A review on tourism destination competitiveness. Journal of Hospitality and Tourism Management, 45, 256–265. https://doi.org/10.1016/j.jhtm.2020.06.012
- De La Hoz-Correa, A., & Muñoz-Leiva, F. (2019). The role of information sources and image on the intention to visit a medical tourism destination: a cross-cultural analysis. Journal of Travel & Tourism Marketing, 36(2), 204–219. https://doi.org/10.1080/10548408.2018.1507865
- Dong, X., Ma, J., Zhang, X., Shaalan, A., Chen, Q., & Jia, S. (2023). Revolution trend investigation of tourism destination image with machine learning. Journal of Vacation Marketing, 1–22. https://doi.org/10.1177/13567667231213152
- Eid, R., El-Kassrawy, Y. A., & Agag, G. (2019). Integrating destination attributes, political (in)stability, destination image, tourist satisfaction, and intention to recommend: A study of UAE. Journal of Hospitality & Tourism Research, 43(6), 839–866. https://doi.org/10.1177/1096348019837750
- Elbagir, S., & Yang, J. (2019 Twitter sentiment analysis using natural language toolkit and VADER sentiment [Paper presentation]. Proceedings of the International Multiconference of Engineers and Computer Scientists.
- Ferrer-Rosell, B., & Marine-Roig, E. (2020). Projected versus perceived destination image. Tourism Analysis, 25(2), 227–237. https://doi.org/10.3727/108354220X15758301241747
- Filimonau, V., & Perez, L. (2018). National culture and tourist destination choice in the UK and Venezuela: An exploratory and preliminary study. Tourism Geographies, 21(2), 235–260. https://doi.org/10.1080/14616688.2018.1490342
- Gartner, W. C. (1994). Image formation process. Journal of Travel & Tourism Marketing, 2(2-3), 191–216. https://doi.org/10.1300/J073v02n02_12
- González-Rodríguez, M. R., Martínez-Torres, R., & Toral, S. (2016). Post-visit and pre-visit tourist destination image through eWOM sentiment analysis and perceived helpfulness. International Journal of Contemporary Hospitality Management, 28(11), 2609–2627. https://doi.org/10.1108/IJCHM-02-2015-0057
- Gorji, A. S., Garcia, F. A., & Mercadé-Melé, P. (2023). Tourists’ perceived destination image and behavioral intentions towards a sanctioned destination: Comparing visitors and non-visitors. Tourism Management Perspectives, 45, 101062. https://doi.org/10.1016/j.tmp.2022.101062
- Guerreiro, J., & Rita, P. (2020). How to predict explicit recommendations in online reviews using text mining and sentiment analysis. Journal of Hospitality and Tourism Management, 43, 269–272. https://doi.org/10.1016/j.jhtm.2019.07.001
- He, L., & Filimonau, V. (2020). The effect of national culture on pro-environmental behavioural intentions of tourists in the UK and China. Tourism Management Perspectives, 35, 100716. https://doi.org/10.1016/j.tmp.2020.100716
- Hofstede, G. (2011). Dimensionalizing cultures: The Hofstede model in context. Online Readings in Psychology and Culture, 2(1), 8. https://doi.org/10.9707/2307-0919.1014
- Hu, F., Teichert, T., Deng, S., Liu, Y., & Zhou, G. (2021). Dealing with pandemics: An investigation of the effects of COVID-19 on customers’ evaluations of hospitality services. Tourism Management, 85, 104320. https://doi.org/10.1016/j.tourman.2021.104320
- Huang, S. S., & Wen, J. (2021). Developing and validating a Chinese cultural value scale in tourism. Tourism Management, 86, 104327. https://doi.org/10.1016/j.tourman.2021.104327
- Huang, L., Ma, J., & Chen, C. (2017 Topic detection from microblogs using T-LDA and perplexity [Paper presentation]. 2017 24th Asia-Pacific Software Engineering Conference Workshops (APSECW). https://doi.org/10.1109/APSECW.2017.11
- Jelodar, H., Wang, Y., Yuan, C., Feng, X., Jiang, X., Li, Y., & Zhao, L. (2019). Latent Dirichlet allocation (LDA) and topic modeling: Models, applications, a survey. Multimedia Tools and Applications, 78(11), 15169–15211. https://doi.org/10.1007/s11042-018-6894-4
- Jiang, Q., Chan, C.-S., Eichelberger, S., Ma, H., & Pikkemaat, B. (2021). Sentiment analysis of online destination image of Hong Kong held by mainland Chinese tourists. Current Issues in Tourism, 24(17), 2501–2522. https://doi.org/10.1080/13683500.2021.1874312
- Kanwel, S., Zhou, L. Q., Asif, M., Hwang, J., Hussain, A., & Jameel, A. (2019). The influence of destination image on tourist loyalty and intention to visit: Testing a multiple mediation approach. Sustainability, 11(22), 6401. https://doi.org/10.3390/su11226401
- Kay, P. L. (2013). Cultural experience tourist motives dimensionality: A cross-cultural study. In Marketing of tourism experiences (pp. 236–278). Routledge.
- Khan, A., Ashfaq, J., Bilal, M., Khan, M. H., & Shad, F. (2021). Destination image formation through user generated content (UGC). An updated literature review. Indian Journal of Economics and Business, 20(2), 1223–1238.
- Kim, C., & Lee, S. (2020). Understanding the cultural differences in tourist motivation between Anglo-American and Japanese tourists. In Japanese tourists. (pp. 153–170). Routledge.
- Kim, K., Park, O., Barr, J., & Yun, H. (2019). Tourists’ shifting perceptions of UNESCO heritage sites: Lessons from Jeju Island-South Korea. Tourism Review, 74(1), 20–29. https://doi.org/10.1108/TR-09-2017-0140
- Kirilenko, A. P., Stepchenkova, S. O., & Dai, X. (2021). Automated topic modeling of tourist reviews: Does the Anna Karenina principle apply? Tourism Management, 83, 104241. https://doi.org/10.1016/j.tourman.2020.104241
- Kirilenko, A. P., Stepchenkova, S. O., Kim, H., & Li, X. (. (2018). Automated sentiment analysis in tourism: Comparison of approaches. Journal of Travel Research, 57(8), 1012–1025. https://doi.org/10.1177/0047287517729757
- Kozak, M., Bigné, E., González, A. N. A., & Andreu, L. (2003). Cross-cultural behavior research in tourism: A case study on destination image. Tourism Analysis, 8(2), 253–257. https://doi.org/10.3727/108354203774077101
- Kramsch, C. (2014). Language and culture. AILA Review, 27(1), 30–55. https://doi.org/10.1075/aila.27.02kra
- Lai, I. K. W., & Hitchcock, M. (2015). Importance–performance analysis in tourism: A framework for researchers. Tourism Management, 48, 242–267. https://doi.org/10.1016/j.tourman.2014.11.008
- Lee, J.-S., & Park, S. (2023). A cross-cultural anatomy of destination image: An application of mixed-methods of UGC and survey. Tourism Management, 98, 104746. https://doi.org/10.1016/j.tourman.2023.104746
- Li, Y., He, Z., Li, Y., Huang, T., & Liu, Z. (2023). Keep it real: Assessing destination image congruence and its impact on tourist experience evaluations. Tourism Management, 97, 104736. https://doi.org/10.1016/j.tourman.2023.104736
- Li, J., & Lu, Y. (. (2016). Chinese culture in tourist research: a review and comparison of Chinese and English studies in 1993-2012. Tourism Review, 71(2), 118–134. https://doi.org/10.1108/TR-05-2015-0021
- Lin, M. S., Liang, Y., Xue, J. X., Pan, B., & Schroeder, A. (2021). Destination image through social media analytics and survey method. International Journal of Contemporary Hospitality Management, 33(6), 2219–2238. https://doi.org/10.1108/IJCHM-08-2020-0861
- Li, X., Zhang, Y., & Mei, L. (2023). Analyzing online reviews of foreign tourists to destination attractions in China: a novel text mining approach. Asia Pacific Journal of Tourism Research, 28(7), 647–666. https://doi.org/10.1080/10941665.2023.2255315
- Li, Q. (2020). Chengdu will build panda international tourism resort. Xinhua News Agency. https://m.gmw.cn/baijia/2020-06/02/1301256533.html
- MacKay, K. J., & Fesenmaier, D. R. (2000). An exploration of cross-cultural destination image assessment. Journal of Travel Research, 38(4), 417–423. https://doi.org/10.1177/004728750003800411
- Maier, D., Waldherr, A., Miltner, P., Wiedemann, G., Niekler, A., Keinert, A., Pfetsch, B., Heyer, G., Reber, U., & Häussler, T. (2021). Applying LDA topic modeling in communication research: Toward a valid and reliable methodology. In Computational methods for communication science (pp. 13–38). Routledge.
- Manrai, L. A., Manrai, A. K., Lascu, D., & Friedeborn, S. (2019). Determinants and effects of cultural context: A review, conceptual model, and propositions. Journal of Global Marketing, 32(2), 67–82. https://doi.org/10.1080/08911762.2018.1449599
- Martilla, J. A., & James, J. C. (1977). Importance-performance analysis. Journal of Marketing, 41(1), 77–79. https://doi.org/10.1177/002224297704100112
- Mehraliyev, F., Chan, I. C. C., & Kirilenko, A. P. (2022). Sentiment analysis in hospitality and tourism: A thematic and methodological review. International Journal of Contemporary Hospitality Management, 34(1), 46–77. https://doi.org/10.1108/IJCHM-02-2021-0132
- Mok, C., & Defranco, A. L. (2000). Chinese cultural values: Their implications for travel and tourism marketing. Journal of Travel & Tourism Marketing, 8(2), 99–114. https://doi.org/10.1300/J073v08n02_07
- Munawir, K., Koerniawan, M. D., & Dewancker, B. J. (2019). Visitor Perceptions and effectiveness of place branding strategies in thematic parks in Bandung City using text mining based on Google Maps user reviews. Sustainability, 11(7), 2123. https://doi.org/10.3390/su11072123
- Nikolskaya, E. Y., Zakharova, E. V., Galkin, D. V., Kovaleva, N. I., & Panova, N. A. (2021). The impact of digital technologies on the transformation of the tourism and hospitality industry. Revista Geintec-Gestao Inovacao e Tecnologias, 11(4), 623–632.
- Oliveira, E., & Panyik, E. (2015). Content, context and co-creation: Digital challenges in destination branding with references to Portugal as a tourist destination. Journal of Vacation Marketing, 21(1), 53–74. https://doi.org/10.1177/1356766714544235
- Panichella, A., Dit, B., Oliveto, R., Di Penta, M., Poshynanyk, D., & De Lucia, A. (2013 How to effectively use topic models for software engineering tasks? an approach based on genetic algorithms [Paper presentation]. 2013 35th International Conference on Software Engineering (ICSE). https://doi.org/10.1109/ICSE.2013.6606598
- Peng, Y., Yin, P., & Matzler, K. (2022). Analysis of destination images in the emerging ski market: The case study in the Host City of the 2022 Beijing Winter Olympic Games. Sustainability, 14(1), 555. https://doi.org/10.3390/su14010555
- Perez-Arce, P. (1999). The influence of culture on cognition. Archives of Clinical Neuropsychology, 14(7), 581–592. https://doi.org/10.1093/arclin/14.7.581
- Pike, S. (2002). Destination image analysis—A review of 142 papers from 1973 to 2000. Tourism Management, 23(5), 541–549. https://doi.org/10.1016/S0261-5177(02)00005-5
- Pike, S., & Kotsi, F. (2018). Stopover destination image – perceptions of Dubai, United Arab Emirates, among French and Australian travellers. Journal of Travel & Tourism Marketing, 35(9), 1160–1174. https://doi.org/10.1080/10548408.2018.1476303
- Pike, S., & Ryan, C. (2004). Destination positioning analysis through a comparison of cognitive, affective, and conative perceptions. Journal of Travel Research, 42(4), 333–342. https://doi.org/10.1177/0047287504263029
- Poria, S., Cambria, E., & Gelbukh, A. (2016). Aspect extraction for opinion mining with a deep convolutional neural network. Knowledge-Based Systems, 108, 42–49. https://doi.org/10.1016/j.knosys.2016.06.009
- Quintal, V. A., Lee, J. A., & Soutar, G. N. (2010). Tourists’ information search: the differential impact of risk and uncertainty avoidance. International Journal of Tourism Research, 12(4), 321–333. https://doi.org/10.1002/jtr.753
- Reisinger, Y., & Turner, L. (1998). Cross-cultural differences in tourism: A strategy for tourism marketers. Journal of Travel & Tourism Marketing, 7(4), 79–106. https://doi.org/10.1300/J073v07n04_05
- Ren, G., & Hong, T. (2017). Investigating online destination images using a topic-based sentiment analysis approach. Sustainability, 9(10), 18, Article 1765. https://doi.org/10.3390/su9101765
- Ribeiro, F. N., Araújo, M., Gonçalves, P., André Gonçalves, M., & Benevenuto, F. (2016). Sentibench-a benchmark comparison of state-of-the-practice sentiment analysis methods. EPJ Data Science, 5(1), 1–29. https://doi.org/10.1140/epjds/s13688-016-0085-1
- Rittichainuwat, B., Laws, E., Maunchontham, R., Rattanaphinanchai, S., Muttamara, S., Mouton, K., Lin, Y., & Suksai, C. (2020). Resilience to crises of Thai MICE stakeholders: A longitudinal study of the destination image of Thailand as a MICE destination. Tourism Management Perspectives, 35, 100704. https://doi.org/10.1016/j.tmp.2020.100704
- Sahin, S., & Baloglu, S. (2011). Brand personality and destination image of Istanbul. Anatolia, 22(1), 69–88. https://doi.org/10.1080/13032917.2011.556222
- Seo, J.-A., & Oh, I. K. (2017). Destination image analysis of Daegu using social network analysis: Social media big data. The Journal of the Korea Contents Association, 17(7), 443–454.
- Sievert, C., & Shirley, K. (2014 LDAvis: A method for visualizing and interpreting topics [Paper presentation]. Proceedings of the Workshop on Interactive Language Learning, Visualization, and Interfaces. https://doi.org/10.3115/v1/W14-3110
- Sorokina, E., Wang, Y., Fyall, A., Lugosi, P., Torres, E., & Jung, T. (2022). Constructing a smart destination framework: A destination marketing organization perspective. Journal of Destination Marketing & Management, 23, 100688. https://doi.org/10.1016/j.jdmm.2021.100688
- Stepchenkova, S., Kim, H., & Kirilenko, A. (2014). Cultural differences in pictorial destination images: Russia through the camera lenses of American and Korean tourists. Journal of Travel Research, 54(6), 758–773. https://doi.org/10.1177/0047287514535849
- Taecharungroj, V., & Mathayomchan, B. (2019). Analysing TripAdvisor reviews of tourist attractions in Phuket, Thailand. Tourism Management, 75, 550–568. https://doi.org/10.1016/j.tourman.2019.06.020
- Tam, B. K., & Bond, M. H. (2002). Interpersonal behaviors and friendship in a Chinese culture. Asian Journal of Social Psychology, 5(1), 63–74. https://doi.org/10.1111/1467-839X.00094
- Tang, J., Meng, Z., Nguyen, X., Mei, Q., & Zhang, M. (2014 Understanding the limiting factors of topic modeling via posterior contraction analysis [Paper presentation]. International Conference on Machine Learning.
- Tigre Moura, F., Gnoth, J., & Deans, K. R. (2014). Localizing cultural values on tourism destination websites: The effects on users’ willingness to travel and destination image. Journal of Travel Research, 54(4), 528–542. https://doi.org/10.1177/0047287514522873
- VG, G., Park, E., & Lee, C. -K. (2021). Testing the influence of destination source credibility, destination image, and destination fascination on the decision-making process: Case of the Cayman Islands. International Journal of Tourism Research, 23(4), 569–580. https://doi.org/10.1002/jtr.2427
- Vayansky, I., & Kumar, S. A. (2020). A review of topic modeling methods. Information Systems, 94, 101582. https://doi.org/10.1016/j.is.2020.101582
- Wang, J., Li, Y., Wu, B., & Wang, Y. (2021). Tourism destination image based on tourism user generated content on internet. Tourism Review, 76(1), 125–137. https://doi.org/10.1108/TR-04-2019-0132
- Williams, P., Soutar, G., Ashill, N. J., & Naumann, E. (2017). Value drivers and adventure tourism: A comparative analysis of Japanese and Western consumers. Journal of Service Theory and Practice, 27(1), 102–122. https://doi.org/10.1108/JSTP-05-2015-0116
- Woosnam, K. M., Stylidis, D., & Ivkov, M. (2020). Explaining conative destination image through cognitive and affective destination image and emotional solidarity with residents. Journal of Sustainable Tourism, 28(6), 917–935. https://doi.org/10.1080/09669582.2019.1708920
- Xi, Y., Ma, C., Yang, Q., & Jiang, Y. (2022). A cross-cultural analysis of tourists’ perceptions of Airbnb attributes. International Journal of Hospitality & Tourism Administration, 23(4), 754–787. https://doi.org/10.1080/15256480.2020.1862014
- Xiao, X., Fang, C., Lin, H., & Chen, J. (2022). A framework for quantitative analysis and differentiated marketing of tourism destination image based on visual content of photos. Tourism Management, 93, 104585. https://doi.org/10.1016/j.tourman.2022.104585
- Yan, X. (2022). Chenghua district, Chengdu: Strive to create a New Highland for the integrated development of culture, business, and tourism. Sichuan Economic Network. https://travel.sohu.com/a/592412866_120364846
- Yan, Y., Chen, J., & Wang, Z. (2020). Mining public sentiments and perspectives from geotagged social media data for appraising the post-earthquake recovery of tourism destinations. Applied Geography, 123, 102306. https://doi.org/10.1016/j.apgeog.2020.102306
- Yang, Y., Sha, C., Su, W., & Donkor, E. K. N. (2022). Research on online destination image of Zhenjiang section of the Grand Canal based on network content analysis. Sustainability, 14(5), 2731. https://doi.org/10.3390/su14052731
- Zenker, S., & Kock, F. (2020). The coronavirus pandemic–A critical discussion of a tourism research agenda. Tourism Management, 81, 104164. https://doi.org/10.1016/j.tourman.2020.104164
- Zhang, G., Cheng, M., & Zhang, J. (2022). A cross-cultural comparison of peer-to-peer accommodation experience: A mixed text mining approach. International Journal of Hospitality Management, 106, 103296. https://doi.org/10.1016/j.ijhm.2022.103296
- Zhang, K., Chen, Y., & Li, C. (2019). Discovering the tourists’ behaviors and perceptions in a tourism destination by analyzing photos’ visual content with a computer deep learning model: The case of Beijing. Tourism Management, 75, 595–608. https://doi.org/10.1016/j.tourman.2019.07.002
- Zhang, J. C., Xie, C. W., Morrison, A. M., & Zhang, K. (2020). Fostering resident pro-environmental behavior: The roles of destination image and Confucian culture. Sustainability, 12(2), 17, Article 597. https://doi.org/10.3390/su12020597
- Zou, Y. (2020). Ctrip releases data: Chengdu research base of giant panda breeding ranks third in China in terms of online bookings. Red Star News https://www.yangtse.com/zncontent/885834.html