Abstract
Ambiguity has always been a pain in the neck of Natural Language Processing (NLP). Despite enormous AI tools for human language processing, it remains a key concern for Language Technology Researchers to develop a linguistically intelligent tool that could effectively understand linguistic ambiguity and creativity possessed by human language. In this regard, the newly designed AI tool ChatGPT has dramatically attracted human attention due to its remarkable ability to answer human questions from a wide range of domains, which needs a reality check. This article scrutinises ChatGPT’s ability to answer and interpret neologisms, codemixing, and linguistically ambiguous sentences. For this, we have tested lexically, syntactically, and semantically ambiguous expressions, codemixed words, as well as a few language game instances. The findings show that ChatGPT still fails to understand linguistically complex sentences, specifically those common in everyday discourse or not part of any standard textbook. More specifically, semantically ambiguous sentences and language games remain an uphill task for ChatGPT to understand. This has implications for further improving the output of ChatGPT.
1. Introduction
Humans use language to communicate their complex ideas, thoughts, feelings, and so on to others. These messages may also contain linguistically ambiguous words or phrases, leading to the unpredictability and uncertainty of meaning. In everyday interaction, humans scramble with such linguistic ambiguity; therefore, it is safe to argue that AI tools and machines might also struggle to obtain linguistically precise interpretations for such instances (Ortega-Martín et al., Citation2023). In this regard, every Natural Language Processing (NLP) task seeks to do word sense disambiguation task at one of six linguistic levels of analysis, i.e. phonology, morphology, syntax, semantics, pragmatics, and discourse level in the development of intelligent language tools (Jurafsky and Martin, 2020, as cited in Ortega-Martín et al., Citation2023). It is necessary to reach a linguistically precise interpretation (deep-level analysis) of a given input sentence for an application/tool to produce reliable end-user output (Sag et al., Citation2002).
Since its inception, linguistic ambiguity has been a central concern for NLP researchers, significantly affecting the end-user output of NLP tools. Handling linguistic ambiguity is directly proportional to the degree of idiomaticity a sentence contains. To this end, researchers and developers have proposed several techniques to deal with ambiguity. However, so far, it has failed to produce comprehensive, satisfactory, and reliable results (Blodgett et al., Citation2020). Researchers currently developing intelligent language tools plan to employ a mix of techniques to deal with ambiguity effectively (Sag et al., Citation2002). In this respect, a sentence, “I saw him walking by the bank” (Fromkin et al., Citation2014), offers four possible interpretations (see Appendix A), which need disambiguation at all six linguistic levels (as mentioned above) in order to get satisfactory output.
We believe that ambiguity remains an uphill task for the recently launched AI tool, ChatGPT (Sohail et al., Citation2023; Zhong et al., Citation2023), whose output needs evaluation to understand how it handles/reacts to diverse types of ambiguity to propose a suggestion for its effective handling/processing (Lu et al., Citation2023; Peng et al., Citation2023). The growth in ChatGPT-related research is tremendous and more than 5000 articles can be found in the Scopus database (Sohail Citation2024). The research has contributed to many fields including healthcare (Farhat, Citation2023, Farhat et al., Citation2024), academia (Farhat et al., Citation2023), business, finance, marketing, etc.
Section 2 is dedicated to covering ChatGPT’s successes and failures concerning different kinds of linguistic ambiguity, codemixing, neologisms, and language games and their implications for ChatGPT. In Section 3, we present the findings and discussion. Finally, Section 4 presents the conclusion and identifies future research directions.
2. ChatGPT’s success and failures
In this section, we present the test cases of ChatGPT’s responses generated against the questions asked belonging to the categories of neologisms, linguistic ambiguity, creativity, and language games. We have purposely selected these categories, as they require the knowledge of strong analytical linguistic skills to decode the meaning. Notably, some of the demonstrated test cases of ChatGPT’s failures might improve in the future because its database is continuously improving. However, the findings of this study could play a crucial role in improving ChatGPT and other AI tools in generating desired end-user outputs.
2.1. Coinage, neologisms, and codemixing
Coinage is a popular word formation process, using which a new word, term, and phrase is created in a language (Qamar et al., Citation2022). Notably, using coinage a wide range of words, terminologies, and phrases can be created, which is yet to become part of the main vocabulary of human language. Yule (2020) defines it as “The word-formation type that allows humans to create a completely new lexical item either deliberately or accidentally.” Such a coinage might occur within one language (e.g., COVID-19) or by fusing items from two or more languages, leading to the codemixed coinage, e.g., virason (viruses).Codemixed words are lexical items that combine elements from two or more different languages within a single utterance or text e.g. doctaron (meaning doctors). The former is pure English coinage, while the latter ones are English + Hindi coinage example, where the “on” Hindi plural suffix is attached to the English root “virus” and “doctor” to make English-Hindi codemixed plural words (refer to Kathpalia, Citation2018; Qamar et al., Citation2022 detailed discussion on Hindi-English codemixed words and patterns). Codemixed words are becoming increasingly popular because they bridge the lexical gap between two linguistically different languages in ways that wouldn’t be possible within the confines of a single language. Codemixing presents a creative and intellectual challenge marked by ambiguity, requiring speakers and listeners to navigate and interpret multiple linguistic cues simultaneously, similar to solving a puzzle. This dynamic interplay of languages not only enriches communication but also showcases the complex identities and cultural interactions of multilingual speakers.
2.2. Ambiguity and some of its types
Ambiguity arises when a linguistic expression possesses more than one possible sense or inexactness and is ambiguous (Oxford Learner’s Dictionaries, Citation2023). According to the linguistic lens, ambiguity can be classified into lexical, syntactic, semantic, pragmatic, and discourse levels. Therefore, inevitably we encounter ambiguity at one or multiple linguistic levels in everyday conversation. Notably, in the development of NLP tools (Anas et al., Citation2024), advanced levels of ambiguity, such as pragmatic and discourse ambiguity, are still kept out of scope (Ortega-Martín et al., Citation2023). In this paper, we have also addressed pragmatic-level ambiguity in a preliminary manner (refer to ). However, the main focus remains on lexical, syntactic, semantic, and pragmatic ambiguities, as well as some wordplays that possess ambiguity. Lexical ambiguity may also arise at the phonological or phonetic level. In addition, syntactic and semantic ambiguities result because of structures posing more than one possible interpretation, and pragmatic ambiguity involves contextual interpretation. Notably, syntactic ambiguity occurs with high frequency. In the section below, we systematically present instances from each category, discuss challenges they pose due to their non-compositional behaviour, and propose solutions for handling them.
2.2.1. Lexical ambiguity
Lexical ambiguity refers to the notion when a word offers two or more possible interpretations/meanings/senses, alternatively also known as semantic ambiguity or homonymy. There are two main types of lexical ambiguity, i.e. homonymy and polysemy. Interestingly, homonymy is a phenomenon when two words have the same pronunciation and spelling or mean different things. Notably, lexical ambiguity is often employed to create Paronomasia and Puns, as exemplified below:
“Your logic is sound, nothing but sound” (Benjamin Franklin); in this example, the word sound is creating a pun. The word “sound” offers two meanings, as an adjective referring to the quality of “being logical or well-reasoned, and as a noun referring to “audible sound.” Suppose people are asked subjectively to respond to the question. In that case, people will quickly understand that the repetition of the word “sound” referring to two different things, hence creating a humoristic effect.
On the other hand, homonymy is synonymously used to refer to both homophones and homographs. Homophones are those linguistic expressions written in the same way or differently but pronounced in the same way and refer to different things—for example, two, to, and too. Further, homographs are words whose orthography and pronunciation remain the same but mean different things, e.g., bark (dog barking as well as the covering of a tree).
Furthermore, polysemous words are expressions that carry multiple senses, serving different communicative functions in other contexts. For instance, the different meaning of the word “sanction” denotes different things, as in “to sanction” means “to permit” and “put sanction on” means “do not permit.”
In short, homonymy refers to the phenomenon where two words bear the same orthography, whereas, in polysemy, a single word bearing the same form serves different meanings depending on the use and by the company it keeps.
2.2.2. Syntactic ambiguity
Structural or syntactic ambiguity occurs when a given sentence or structure carries two or more possible meanings. For instance, in “Flying plane is dangerous,” the interpretation greatly depends on whether one is inside or outside the plane, meaning that “Flying a plane is a dangerous activity,” “Flying by plane is dangerous,” or “Flying plane is dangerous to the objects (people, birds, and drones, etc.) around it.” Lastly, the intended meaning in case of syntactic ambiguity can, by and large, be determined based on its contextual use.
Moreover, in another sentence, “The professor said on Monday he would give an exam”. There are two sources of ambiguity: a) Monday as the day of the exam/Monday as the day when professor announced the exam, and b) the professor as the person himself will appear for his exam.
Therefore, resolving such ambiguities requires careful consideration of the context in which the sentence is used. By incorporating contextual analysis, AI tools like ChatGPT can significantly enhance their performance in handling syntactic ambiguity, leading to more accurate and reliable interpretations.
2.2.3. Semantic ambiguity
Typically, semantic ambiguity arises when there is a lack of context or one or more words refer to multiple senses. Therefore, understanding its meaning requires detecting all possible senses or its dictionary meaning against a word or phrase. However, notably, these measures are not adequate for all the semantic variation across the domains and contexts. Therefore, in some cases, world knowledge or subjective knowledge is required to detect the meaning. For instance, “She knew a little Greek”; in this case, without context, it is hard to predict whether the phrase “a little Greek” infers to the language and culture or a young male or female of Greek origin. In another case, “It is cold here,” the interpretation greatly depends on the fact that who is saying this to whom. If it is a father addressing to his daughter, he wants his daughter to close the window, but if the father says the sentence to his wife/girlfriend, it means he is asking his wife/girlfriend to come, sit next to him and cuddle. Therefore, semantic inexactness or ambiguity may vary from context to context, and the ambiguity could be more significant.
Since its inception, processing ambiguity effectively has been problematic for AI tools (Sag et al., Citation2002). This is also evident in ChatGPT’s generated response against our test cases of ambiguity belonging to different categories, such as lexical ambiguity, syntactic, and semantic ambiguity. Such inaccuracies in AI-generated outputs indicate the methods, stereotypes, and linguistic modelling used to train the system (Borji, Citation2023; Cai et al., Citation2023), leading to the generation of inaccurate, prejudiced, and culturally biased or sensitive outputs. This issue casts doubt on the reliability of AI tools, making it difficult for users to fully trust their responses. Consequently, this can lead to the AI’s inability to produce the output users are seeking.
In order to test ChatGPT’s output accuracy and comprehensibility, we tested lexically, syntactically, and semantically ambiguous sentences and noticed that it could handle ambiguity up to an extent. It understands ambiguous sentences easily, when they are part of standard textbooks, or widely used on digital platforms, especially lexically and syntactically ambiguous sentences. However, it surprisingly fails to handle instances concerning semantic ambiguity, which is evident in . For example, when given a prompt, “I don’t like doormats,” ChatGPT offers only one interpretation of this sentence, treating “doormats” as meaning a mat typically placed near a door/entrance. It does not consider the alternative meaning, which refers to a submissive or unassertive person (see ). We tested another sentence, “Lions eat more than deer”; the result was generation based on the parts of speech. Whereas the response would have been generated by focusing on the function of the phrase “more than deer,” which is a comparative expression that signifies something surpasses deer, ultimately referring to the notion that lions eat other animals besides deer, upon asking follow-up question that can you provided more appropriate interpretation to it. ChatGPT said, “It’s important to note that the most suitable interpretation would depend on the specific context in which the phrase is used and the intended meaning of the speaker or writer. Without further information about the context or intended message, these interpretations provide general possibilities for understanding the phrase ‘more than deer’.” The pipeline architecture of ChatGPT has been trained using a vast amount of data, containing a large number of information concerning human language, bias, interpretation, creativity, and socio-political context, to name a few. So this becomes an extremely uphill task for developers to figure out the point/module where a specific problem occurred during the processing of data, leading to the generation of inaccurate, biased, insufficient, and sensitive output. However, it is noteworthy to mention that the developers of ChatGPT are aware that the comprehension of meaning in human language is far more complex and often depends on both linguistic and extra-linguistic factors. So, we hope that future versions will get better at handling linguistic ambiguity, including advanced levels of ambiguity, such as pragmatic and discourse ambiguity, if the data is thoroughly examined and classified, taking into account socio-political information, linguistic knowledge, and so on.
2.3. Wordplays, puns, and satires
By and large, all these three keywords (wordplays, puns, and satire) are used to create a verbal wit or humorous effect. Yule (Citation2010, p. 121) says that understanding the polysemous nature of a word in a sentence is crucial to understand all the possible interpretations of a sentence. For instance, in “Mary had a little lamb,” we generally think of literal interpretation, i.e. little pet lamb, but the polysemous nature of the word ‘lamb’ offers us another interpretation, “flesh of a lamb.” Notably, people have used humour to amuse and entertain each other in society since time immemorial. It may appear in both verbal and non-verbal forms, and sometimes involves a high level of creativity. Also, noteworthy to mention that the same jokes may have completely different reactions from different audiences; therefore, they should be used carefully.
Our test data suggests that ChatGPT could understand the wordplay and humour up to a point. However, in some cases, it miserably failed to understand the polysemous behaviour of a word in a given sentence, leading to the dual interpretation of the whole sentence (see and ). In addition, it also failed to understand the political joke. It gave a general explanation of the prompt (see ), giving rise to the notion that it does not understand when linguistic creativity is high.
2.4. Language games
The concept of language game mainly relies on the philosophical notion of language use and how language is creatively organized, introduced by the German philosopher Ludwig Wittgenstein. The proponent advocated that the meaning of words or sentences can only be understood by the game’s rules. In this regard, based on the context (tone and speaker’s social status), the word “water” could be interpreted as order, command, or request. McCabe (Citation1992) defines a language game as “a spoken routine for two or more players, meant to be repeated many times.” This infers that mastery over language games enables the speakers to communicate effectively. Playing language games requires critical and analytical thinking, helping humans develop language and cognitive abilities. Those not well exposed to it will greatly fail to predict the pattern and language cues.
In this regard, ChatGPT produced inaccurate output concerning the language game, namely “identification of hidden animal names in different sentences.” Sometimes, it identified the animal’s name but not according to the given sentence. In a typical example, “If Roger comes, we will begin,” ChatGPT said “goat,” while the correct answer is “frog.” In fact, on several occasions, this happens due to the scarcity of creative data sets; however, Google correctly answers this question. This gives rise to the sense that ChatGPT fails to fetch information from open-source internet repository, resulting in creating either inaccurate results or saying that “I apologise for any confusion caused. Please note that the given input is a creative and playful interpretation and not a literal or grammatically correct analysis of the sentence” (see ). This clearly indicates that ChatGPT greatly fails to process creative and analytical language-related questions.
However, when asked, ChatGPT could explain the concept of language games, their history, proponent, and some of their types. Notably, humans are no exception, but comparatively, they can identify and predict patterns using natural intelligence.
3. Discussion
ChatGPT has surprised everyone because of its Large Language Models (LLMs) technique, enabling it to answer a wide range of human queries and giving it an edge over the earlier AI tools, which need thorough and systematic investigation. As the first step, LLMs initially break the given input into multiple tokens and decillions of such tokens have been used to train this system in a sense that which word will occur after which one in a linear order in a sentence (Cai et al., Citation2023). Even though ChatGPT has become immensely popular thanks to its exceptional ability to provide answers, a feat achieved through training the system with a vast dataset and employing a probabilistic model, it has reached a point where the developers could not also figure out how the system responds to a particular prompt given as an input. This raises the question of how the system interprets an idiosyncratic situation or instance, which is a new example or rarely used expression.
Therefore, we have examined ChatGPT’s efficacy, accuracy, and comprehensibility in answering questions concerning linguistic creativity (such as neologisms, codemixing, and language games) (see ) and ambiguity (lexical, syntactic, and semantic) (see ) to see whether it understands the language the way humans understand it or use it. Our findings revealed that of those above two broad categories, i.e. linguistic creativity and linguistic ambiguity, ChatGPT was able to understand standardised or textual examples of lexical and syntactic ambiguity far more easily as compared to the semantic ambiguity, neologisms, and language games (refer ). More specifically, even after providing the context for neologisms and language game-related queries, it failed to generate desired output (see ). More specifically, our creative examples (refer Appendix A) revealed that ChatGPT merely mimics the information based on the data available, signifying further that human cognition and meaning comprehension is still far more reached for ChatGPT. In particular, the problem occurred because ChatGPT treated all words individually and generated the semantic features in the given combination, which should be considered as a single token, despite having multiword in the given combination. For example, a) Kim made a face at policemen, and b) Kim made a face in pottery class. In example (a), ‘made a face’ should be treated as one token, while in example (b), it should be treated as three different tokens (made, a, and face). For this, a suitable linguistic analysis needs to be done, failing which system will either produce inaccurate or unreliable results, which is by and larger evident in the examples (please refer to the Appendix A). As opposed to humans, ChatGPT-generated answers infer that the system did not seem to be relying on contextual information in parsing lexically, syntactically, and semantically ambiguous sentences. Further, it mostly gives irrationally long answers instead of specific information required against a given prompt, requiring concise and shorter answers. These observations highlight the need for enhancing ChatGPT’s ability to leverage contextual information and generate more concise responses to improve its handling of ambiguous sentences and alignment with user expectations.
4. Conclusion and future directions
In this article, we have demonstrated that the efficacy of ChatGPT in answering queries concerning linguistic ambiguity, creativity, and codemixing (words or sentences composed of two or more linguistic items from different languages) remains a significant challenge, given the complexity, idiosyncrasy, and diversity of human linguistic behavior. Therefore, linguistic ambiguity and creativity are key concerns for ChatGPT, requiring enhanced linguistic precision in AI tools. The primary goal of this paper was to shed light on the linguistic creativity, variability, and productivity of human language causing pain in the neck of ChatGPT. In this regard, we tested demo sentences highlighting linguistic ambiguity and creativity, showcasing how humans often deviate from standard grammatical norms to meet their communicative needs. This is especially evident in code-mixing, which poses a significant challenge for ChatGPT in understanding its contextual use. Codemixing enables humans to fill the lexical gap between the two linguistically different languages and helps us communicate effectively. We have also tested ChatGPT’s output with such examples, which provides enough evidence of ChatGPT’s efficacy in meeting human language use, which still looks far from being achieved.
While the findings advance our understanding and suggest directions for further systematic and linguistic investigations into factors affecting ChatGPT’s outputs, there is a notable gap in addressing potential solutions or improvements. Therefore, to enhance the performance of ChatGPT in handling linguistic complexities, future research should not only continue to identify weaknesses but also actively explore and test potential solutions. This could include developing advanced algorithms that better understand and generate codemixed text, improving models’ training on diverse linguistic datasets, and integrating more sophisticated natural language understanding techniques. These techniques could significantly refine AI tools like ChatGPT’s output, making them more adept at handling the nuanced nature of human language.
Disclosure statement
No potential conflict of interest was reported by the author(s).
Additional information
Notes on contributors
Md. Tauseef Qamar
Md. Tauseef Qamar is an Assistant Professor of English Linguistics at the School of Advanced Sciences and Languages, VIT Bhopal University, Sehore, Madhya Pradesh, India. He received his doctorate in Computational Linguistics at Aligarh Muslim University, Aligarh, India, in 2019. Currently, he teaches undergraduate and graduate engineering and management major students Computational linguistics, Cross-cultural communication, and Soft skills courses. His academic and research area focuses on Multiword Expressions, Foreign Language Teaching Methodologies, Technology-enhanced Learning, English in the Workplace, and Indic Machine Translation.
Juhi Yasmeen
Juhi Yasmeen is an Assistant Professor of English Linguistics at the School of Advanced Sciences and Languages, VIT Bhopal University, Sehore, Madhya Pradesh, India. She received PhD in Applied Linguistics at Aligarh Muslim University, Aligarh, India, in 2017. She currently teaches undergraduate and graduate engineering and management students Cross-cultural communication, Technical Communication, and Soft skills courses. Her research interests are Translation Studies, English Language Teaching, Education and Technology, Syllabus Design, and Media Linguistics.
Sanket Kumar Pathak
Sanket Kumar Pathak is a Language Manager at Mihup Communications, Kolkata, West Bengal, India. He received his doctorate in linguistics at Lucknow University, India, in 2010. Currently, he handles a large team of linguists and engineers. Besides this, he has developed several Indic machine translation systems. His research focuses on Artificial Intelligence Language Technology such as ASR, TTS, NLU/NLG, MTs, etc.
Shahab Saquib Sohail
Shahab Saquib Sohail holds a master’s and doctorate from Aligarh Muslim University, majoring in computer science. His research interests include Social Networks and Privacy, Recommender Systems, Personally Identifiable Information (PII), and Users’ Online Behavior. Notably, he has developed a book recommender system tailored for computer science graduates in India. With over 70 publications in reputed journals and top-tier conferences, he serves on the editorial boards of numerous scientific journals.
Dag Øivind Madsen
Dag Øivind Madsen obtained his bachelor’s degree from the University of Bergen, Norway, followed by an M.Sc. degree from the London School of Economics in 2002 and a Ph.D. from the Norwegian School of Economics in 2011. Presently, he serves as a Professor at the University of South-Eastern Norway. His research focuses on Industry 4.0/5.0 and the Utilization of emerging technologies, such as Big Data and Artificial Intelligence, in various business and organizational settings.
Mithila Rangarajan
Mithila Rangarajan, a postgraduate student in English at St. Joseph’s University in Bengaluru, India, centres her academic interests on Translation Studies, Aesthetics, and Artificial Intelligence.
References
- Anas, M., Saiyeda, A., Sohail, S. S., Cambria, E., & Hussain, A. (2024). Can generative AI models extract deeper sentiments as compared to traditional deep learning algorithms? IEEE Intelligent Systems, 39(2), 1–17. https://doi.org/10.1109/MIS.2024.3374582
- Blodgett, S. L., Barocas, S., Daumé, H., III, & Wallach, H. (2020). Language (technology) is power: A critical survey of “bias” in NLP. In Proceedings of the 58th Annual Meeting of the Association for Computational Linguistics 2020 July. pp. 5454–5476.
- Borji, A. (2023). A categorical archive of ChatGPT failures. arXiv preprint arXiv:2302.03494.
- Cai, Z. G., Haslett, D. A., Duan, X., Wang, S., & Pickering, M. J. (2023). Does ChatGPT resemble humans in language use?. arXiv preprint arXiv:2303.08014.
- Farhat, F.,Sohail, S. S., &Madsen, D. (2023). How trustworthy is ChatGPT? The case of bibliometric analyses. Cogent Engineering. 2023 Dec. Cogent Engineering, 10(1), 1–8. https://doi.org/10.1080/23311916.2023.2222988.
- Farhat, F., Chaudhry, B. M., Nadeem, M., Sohail, S. S., & Madsen, D. Ø. (2024). Evaluating large language models for the national premedical exam in India: Comparative analysis of GPT-3.5, GPT-4, and Bard. JMIR Medical Education, 10(e51523), 01–12. https://doi.org/10.2196/51523.
- Farhat, F. (2023). ChatGPT as a complementary mental health resource: A boon or a bane. Annals of Biomedical Engineering, 52(2024), 1111–1114. https://doi.org/10.1007/s10439-023-03326-7.
- Fromkin, V., Rodman, R., & Hyams, N. (2014). An introduction to language. An introduction to language. Wadsworth.
- Kathpalia, S. S. (2018). Neologisms: Word creation processes in Hindi-English code-mixed words. English World-Wide. A Journal of Varieties of English, 39(1), 34–59. https://doi.org/10.1075/eww.00002.kat
- Lu, Q., Qiu, B., Ding, L., Xie, L., & Tao, D. (2023). Error analysis prompting enables human-like translation evaluation in large language models: A case study on chatgpt. https://doi.org/10.20944/preprints202303.0255.v1
- McCabe, A. (1992). Language games to play with your child. Da Capo Lifelong Books. Insight Books Plenum Press.
- Ortega-Martín, M., García-Sierra, Ó., Ardoiz, A., Álvarez, J., Armenteros, J. C., & Alonso, A. (2023). Linguistic ambiguity analysis in ChatGPT. arXiv preprint arXiv:2302.06426.
- Oxford Learner’s Dictionaries. (2023). Oxfordlearnersdictionaries.com dictionary. Retrieved Jun 14, 2023, from https://www.oxfordlearnersdictionaries.com/definition/english/ambiguity?q=ambiguity
- Peng, K., Ding, L., Zhong, Q., Shen, L., Liu, X., Zhang, M., Ouyang, Y., & Tao, D. (2023). Towards making the most of chatgpt for machine translation. arXiv preprint arXiv:2303.13780.
- Qamar, M. T., Yasmeen, J., Zeeshan, M. A., & Pathak, S. (2022). Coroneologisms and word formation processes in Hindi-English codemixed words. Acta Linguistica Asiatica, 12(1), 59–89. https://doi.org/10.4312/ala.12.1.59-89
- Sag, I. A., Baldwin, T., Bond, F., Copestake, A., & Flickinger, D. (2002). Multiword expressions: A pain in the neck for NLP. In Computational Linguistics and Intelligent Text Processing: Third International Conference, CICLing 2002 Mexico, 2002 Proceedings 3 (pp. 1-15). Berlin, Heidelberg: Springer. https://doi.org/10.1007/3‐540‐45715‐1_1
- Sohail, S. S., Farhat, F., Himeur, Y., Nadeem, M., Madsen, D. Ø., Singh, Y., Atalla, S., & Mansoor, W. (2023). Decoding ChatGPT: A taxonomy of existing research, current challenges, and possible future directions. Journal of King Saud University - Computer and Information Sciences, 35(8), 101675. https://doi.org/10.1016/j.jksuci.2023.101675
- Sohail, S. S. (2024). A promising start and not a panacea: ChatGPT’s early impact and potential in medical science and biomedical engineering research. Annals of Biomedical Engineering, 52(5), 1131–1135. https://doi.org/10.1007/s10439-023-03335-6
- Yule, G. (2010). The study of language. Cambridge university press.
- Zhong, Q., Ding, L., Liu, J., Du, B., & Tao, D. (2023). Can chatgpt understand too? A comparative study on chatgpt and fine-tuned bert. arXiv preprint arXiv:2302.10198.
Appendix A.
ChatGPT test results
Syntactic ambiguity
Example 2 “She wore a dress with pink dots and circles.”
Source: Tested on ChatGPT on 17 May 2023.
Note: ChatGPT was able to generate only one interpretation—that the dress had (a pattern of) pink dots and pink circles. The scope of ambiguity (does the word “pink” apply only to “dots” or to “dots and circles”) is not present in the interpretations provided by ChatGPT.
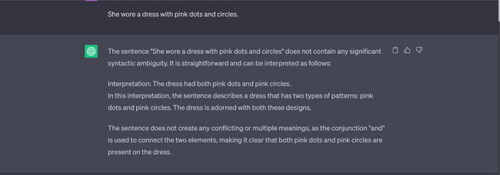
Example 3 “Man linked to stock market crashes.”
Source: Tested on ChatGPT on 17 May 2023.
Note: ChatGPT offers two interpretations, both of which are similar, since they treat “crashes” as modifying “stock market.” Another distinct interpretation is: “A man was linked to the stock market. This man has crashed.” ChatGPT’s two interpretations seem to revolve around semantic ambiguity in the word “linked,” rather than syntactic ambiguity relating to the word “crashes.”
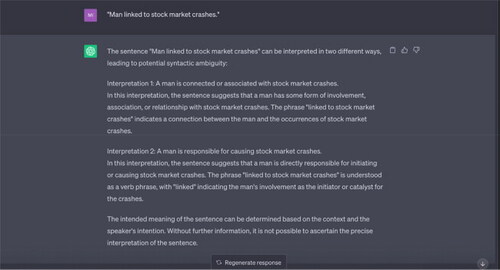
Example 4 “Lions eat more than deer.”
Source: Tested on ChatGPT on 17 May 2023.
Note: ChatGPT offers only one interpretation, that of a comparison between the amount eaten by lions and deer, and offers an explanation “that lions have a higher food intake or appetite compared to deer.” It does not consider that the sentence could be interpreted to mean that lions eat other animals, not just deer.
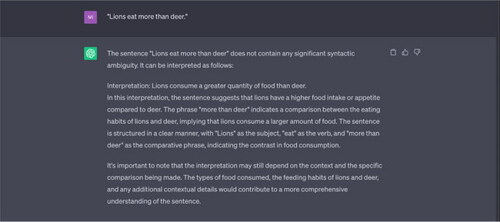
Example 5 “Irritating professors can cause problems for students.”
Source: Tested on ChatGPT on 17 May 2023.
Note: The word “irritating” here can be used as a verb or as an adjective. ChatGPT offers only one interpretation, i.e. it treats “irritating” as an adjective, treating it as part of the subject “irritating professors.” It doesn’t consider that the sentence might also mean: “[The act of] irritating professors can cause problems for students.”
In a previous input sentence, “Linguistics students are confusing people,” ChatGPT similarly offered only one interpretation (treating “confusing” as a part of the verb phrase, as part of the predicate, and ignoring the use of “confusing” as an adjective.
![Example 5 “Irritating professors can cause problems for students.”Source: Tested on ChatGPT on 17 May 2023.Note: The word “irritating” here can be used as a verb or as an adjective. ChatGPT offers only one interpretation, i.e. it treats “irritating” as an adjective, treating it as part of the subject “irritating professors.” It doesn’t consider that the sentence might also mean: “[The act of] irritating professors can cause problems for students.”In a previous input sentence, “Linguistics students are confusing people,” ChatGPT similarly offered only one interpretation (treating “confusing” as a part of the verb phrase, as part of the predicate, and ignoring the use of “confusing” as an adjective.](/cms/asset/5f447e32-0731-4de0-ba73-1cc42cb81ef1/oaah_a_2353984_a0004_c.jpg)
Example 6 “The old man the boat.”
Source: Tested on ChatGPT on 17 June 2023.
Note: This is quite a famous example of a syntactically ambiguous sentence, so it is surprising that ChatGPT was unable to parse it for ambiguity. The sentence only makes sense when “man” is treated as a verb form, giving us the meaning “The old (people) operate the boat.” However, ChatGPT is unable to identify and resolve this ambiguity, since it says the input sentence “lacks a clear verb,” “punctuation and clear syntactic clues.”
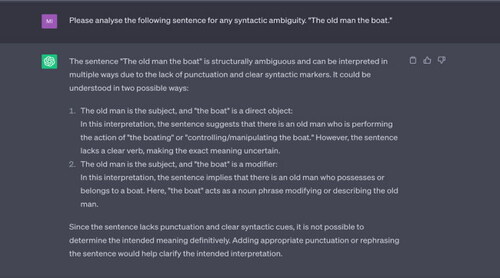
Example 7 “I would like to thank my parents, God and Jawaharlal Nehru/I would like to thank my parents, John and Jessica.”
Source: Tested on ChatGPT on 17 May 2023.
Note: ChatGPT only offers one interpretation of this sentence, which is that the speaker is thanking three separate entities: (their) parents, God, and Jawaharlal Nehru. It does not consider that the comma after “parents” might indicate that God and Jawaharlal Nehru (two separate entities) could be the “parents” referred to by the speaker. Even in the second example made simpler through the use of common Western names (John and Jessica) it does not interpret John and Jessica as the speaker’s parents but instead says the exact relationship between the speaker, John, and Jessica is unclear. It says the sentence is “structured in a clear manner, with a comma separating the different individuals being thanked.” However, there is no comma in the input sentences separating the last two entities (God and Jawaharlal Nehru; John and Jessica).
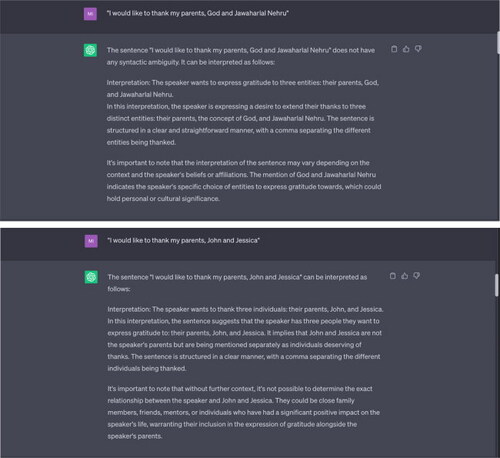
Example 8 “The teacher punished the student with a zero.”
Source: Tested on ChatGPT on 17 May 2023.
Note: ChatGPT does not consider the interpretation “The teacher punished the student who had scored a zero.” The phrase “with a zero” could indicate “the means or method of punishment used by the teacher,” as suggested by ChatGPT, or it could modify “the student,” describing the student who was punished. This latter interpretation is not offered by ChatGPT.
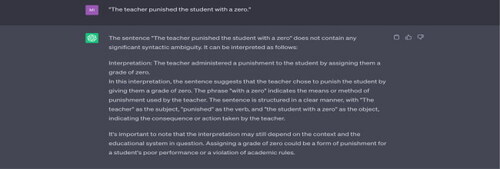
Example 9 “I’m glad I am healthy and so are you.”
Source: Tested on ChatGPT on 17 May 2023.
Note: ChatGPT offers only one interpretation, connecting the latter clause “so are you” to “healthy,” rather than “glad.” This would give us another interpretation: “I am glad I am healthy and you are also glad that I am healthy.”
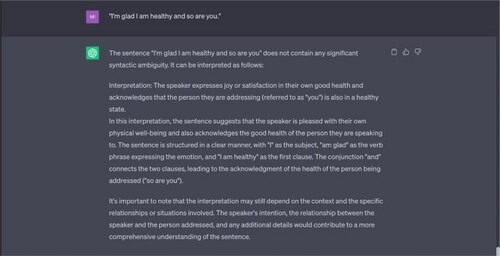
Semantic ambiguity
Example 1 “I don’t like doormats.”
Source: Tested on ChatGPT on 17 May 2023.
Note: ChatGPT offers only one interpretation of this sentence, treating “doormats” as meaning a mat typically placed near a door/entrance. It does not consider the alternative meaning, which refers to a submissive or unassertive person. The speaker might be declaring their dislike of meek or submissive people, rather than a mat placed near a door.
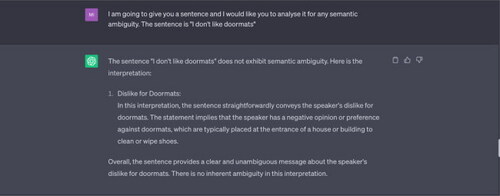
Example 2 “You cannot go fishing at the bank.”
Source: Tested on ChatGPT on 17 June 2023.
Note: In both times that this input sentence was tested, ChatGPT offers only one interpretation of “bank” each time, treating “bank” as a “financial institution” during the first test [17 May], and as “edge of a body of water” during the second test [17 June]. Clearly, it is aware of both meanings, but strangely does not offer both interpretations and insists that “there is no inherent ambiguity” [17 May] and “the sentence does not possess semantic ambiguity” [17 June].
![Example 2 “You cannot go fishing at the bank.”Source: Tested on ChatGPT on 17 June 2023.Note: In both times that this input sentence was tested, ChatGPT offers only one interpretation of “bank” each time, treating “bank” as a “financial institution” during the first test [17 May], and as “edge of a body of water” during the second test [17 June]. Clearly, it is aware of both meanings, but strangely does not offer both interpretations and insists that “there is no inherent ambiguity” [17 May] and “the sentence does not possess semantic ambiguity” [17 June].](/cms/asset/8c436e0e-5487-4e3c-a9f3-8a75f7924fa1/oaah_a_2353984_a0011_c.jpg)
Example 3 “John and his licence both expired last week.”
Source: Tested on ChatGPT on 17 May 2023.
Note: Although ChatGPT correctly interprets the sentence, it fails to explain the zeugma, or the different ways in which the word “expired” apply to John and his licence.
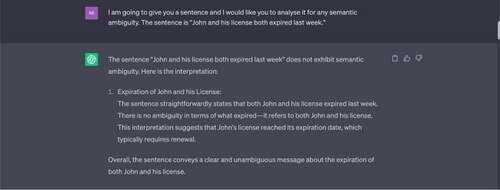
Example 4 He got a new mouse yesterday.”
Source: Tested on ChatGPT on 17 May 2023.
Note: ChatGPT does not recognise any semantic ambiguity in the input sentence. It offers only one interpretation of the word “mouse.” It does not consider that the speaker might have bought a new animal (a small rodent), as a pet mouse.
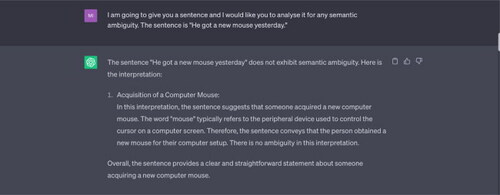
Example 5 “I am drawing a bath.”
Source: Tested on ChatGPT on 17 May 2023.
Note: ChatGPT does not recognise any semantic ambiguity in the input sentence. It offers only one interpretation of the word “drawing.” It does not consider that the speaker might be making a painting (“drawing”) of a bath. According to ChatGPT, “[t]he sentence is straightforward and does not present any ambiguity.”
![Example 5 “I am drawing a bath.”Source: Tested on ChatGPT on 17 May 2023.Note: ChatGPT does not recognise any semantic ambiguity in the input sentence. It offers only one interpretation of the word “drawing.” It does not consider that the speaker might be making a painting (“drawing”) of a bath. According to ChatGPT, “[t]he sentence is straightforward and does not present any ambiguity.”](/cms/asset/3657567a-856f-41e1-a815-911d649bc38a/oaah_a_2353984_a0014_c.jpg)
Example 6 “Fruit flies like bananas.”
Source: Tested on ChatGPT on 17 May 2023.
Note: This input sentence is part of a longer formulation: “Time flies like an arrow. Fruit flies like bananas.” ChatGPT does not offer the possibility of interpreting “flies” as the simple present tense of “fly” (to move through the air using wings). The resulting sentence, although syntactically correct, would be nonsensical, similar to “The car ate a sandwich for lunch” or “Colourless green ideas sleep furiously.” However, the purpose of this exercise is to test whether the LLM is able to generate these interpretations or not, not to see if these interpretations produce meaningful sentences.
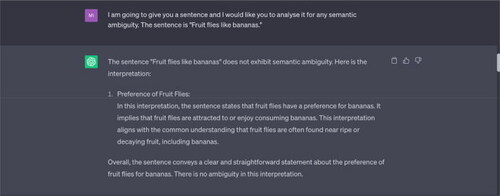
Example 7 “The bat flew overhead.”
Source: Tested on ChatGPT on 17 May 2023.
Note: ChatGPT does not recognise any semantic ambiguity in the input sentence. It offers only one interpretation of the word “bat,” i.e. a small flying mammal. It does not consider that the speaker might be describing a wooden sporting instrument (such as a cricket bat) moving swiftly through the air above the speaker’s head.
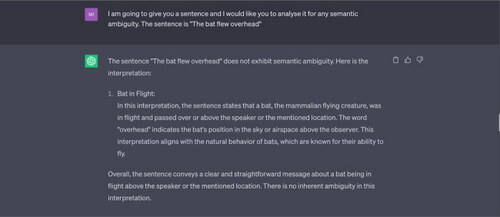
Example 8 “I prefer this date to the others.”
Source: Tested on ChatGPT on 17 May 2023.
Note: ChatGPT considers only one interpretation of “dates,” i.e. as referring to “a specific point in time or calendar day.” It does not consider all the other meanings that might be inferred, such as a small, dark fruit, or a romantic engagement/person with whom one has a romantic engagement.
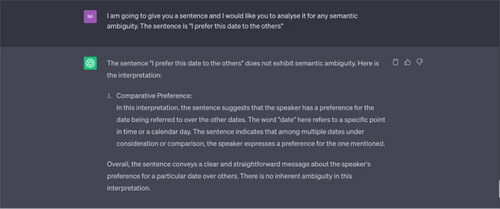
Example 9 “The plants are poisoning the river.”
Source: Tested on ChatGPT on 17 May 2023.
Note: ChatGPT does not provide a definite interpretation of the word “plants,” only stating that plants are “introducing toxins… into the water.” It is unclear if it reads this sentence as referring to polluting living organisms such as small trees or shrubs, or to industrial facilities.
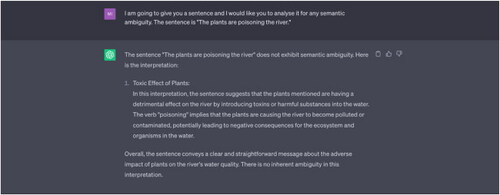