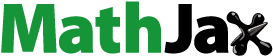
Abstract
Physical activity (PA) has been confirmed by a dozen of studies to be an effective treatment for many diseases. Unfortunately, the habit of regularly engaging in it is rare among Africans, especially those caught in the web of time-consuming sedentary jobs. To contribute to a remedy of this problem, this study identifies relevant socio-demographic factors that determine engagement in PA by workers. A cross-sectional quantitative research technique was employed in this study. Participants were workers in Koforidua Township in Ghana. A self-reported questionnaire was used to collect data from 686 volunteer participants. Ordinary least squares (OLS) regression analysis was used to present results. Findings show that education, gender, marital status, alternative employment, whether or not one currently has or previously had a chronic disease, and the sector of a person’s employment are some of the determinants of PA. All socio-demographic determinants account for 96.3% of the total variation, indicating a strong fit of the OLS model. While education and some other variables promote engagement in PA, age, income, and alternative employment do otherwise. Improving individuals’ education, especially physical education, is recommended as a step towards enabling workers to inculcate the habit of regularly participating in PA.
Public Interest Statement
Research has endorsed physical activity (PA) as a good treatment for depression. PA also positively impacts health-related quality of life and therefore contributes to controlling the global burden of disease and mortality. There is thus an urgent need for all people to embrace PA as a hobby.
Competing Interests
The author declares no competing interest.
1. Introduction
Physical activity (PA) is “any bodily movement produced by skeletal muscles that requires energy expenditure” (World Health Organization, [WHO], Citation2014). According to Webster (Citation2015), PA is becoming part and parcel of life in many developed countries owing to its positive impact on Health-Related Quality of Life (HRQoL). Empirical evidence also shows that an adequate level of PA reduces the risk of many diseases (Blake, Citation2012; Craft & Perna, Citation2004; Ströhle, Citation2009; Webster, Citation2015), particularly cardiovascular diseases, colon and breast cancer, diabetes and depression (Webster, Citation2015; WHO, Citation2014). Also worth mentioning is the fact that physical inactivity is known to be the fourth leading risk factor for worldwide mortality, causing about 3.2 million deaths globally (WHO, Citation2015). Individuals, governments and organizations have as a result begun to recognize the critical role of PA in the promotion of health.
The WHO in particular has provided cut-off points of PA level for various age groups through its “2010 Global Recommendations on Physical Activity for Health”. Within the framework of WHO’s recommendations, countries such as the United Kingdom have instituted a national PA guideline that includes a surveillance program aimed at promoting engagement in PA by citizens. WHO (Citation2015) has also used its “Steps to health: a framework for action in the WHO European Region” guiding document to influence many countries in Europe and other regions to develop and implement a national PA action policy and plan. As a consequence, willful engagement in PA in the United Kingdom and other European countries has improved considerably in the last decade (Josefsson, Lindwall, & Archer, Citation2014; Webster, Citation2015). The situation is however abysmally different in Africa.
WHO (Citation2014) reports that physical inactivity levels in Africa are generally consistent between different genders, ethnicities and income levels. It adds that half of all adults in Africa are inactive. Several studies (e.g. Dembe, Erickson, Delbos, & Banks, Citation2005; Micklesfield et al., Citation2014; Mukona, Munjanja, Zvinavashe, & Stray-Pederson, Citation2016; Oyeyemi et al., Citation2016) have also reported that the level of engagement in PA is lower in Africa than Europe and other developed countries. So unlike many Europeans and citizens of other developed countries, Africans are yet to have PA entrenched in their way of life. In other words, the majority of Africans are yet to inculcate the habit of regularly engaging in PA within the framework of WHO’s recommendations and guidelines.
Africans embracing PA as a habit would depend on their knowledge of basic facts about the impact of PA on health. Sadly this knowledge is currently low owing to a gross lack of PA research and data on Africa (Josefsson et al., Citation2014; Webster, Citation2015). More importantly, stakeholders need knowledge of socio-demographic variables (SDVs) that influence engagement in PA in African environments. Oliveira-Brochado, Oliveira-Brochado, and Brito (Citation2010) reasoned that this knowledge is needed by public health stakeholders, especially governments, to develop and implement national PA guidelines and programs. In addition, individuals would be better equipped to plan regular engagement in PA if they know how and the extent to which the SDVs influence engagement in PA.
Based on the systematic review of Webster (Citation2015) nonetheless, no identifiable study has identified the said SDVs in an African context, a situation that corroborates the general paucity of PA research in Africa (Josefsson et al., Citation2014; Webster, Citation2015). This study is therefore carried out in an attempt to contribute to a remedy of this problem, with the researcher’s attention focused on workers, who are increasingly susceptible to physical inactivity (Reichert, Barros, Domingues, & Hallal, Citation2007; Webster, Citation2015).
2. Methods
2.1. Study design
This study adopts a cross-sectional quantitative research technique to address the research problem. Ordinary least squares (OLS) regression analysis was used to test the effect of selected SDVs on PA. Though several SDVs have been conceptualized in the literature (e.g. Oliveira-Brochado et al., Citation2010) to determine PA, this study focused on those deemed to sufficiently relate to African settings, particularly this study’s participants.
2.2. Participants and sample
This study’s participants were volunteers who were working in various private and public sector organizations in Koforidua in the Eastern Region of Ghana. In selecting participants, the researcher took the list of all organizations in Koforidua from the Municipal Assembly. The researcher visited each of the listed organizations to identify, by means of a short interview, workers who were willing to participate. The numbers of participants reached for various sectors are shown as follows: banking/finance = 123; Healthcare = 115; Security (i.e. police, military, fire services) = 112; education = 103; and others (public sector) = 179. In all, 760 individuals were willing to participate. To provide a basis for detecting weak correlations and effects, all individuals in each group were made to participate in this study.
2.3. Instrumentation
PA was measured using the second version of WHO’s Global Physical Activity Questionnaire (GPAQ-2). The GPAQ-2 is the most recent and validated version of the GPAQ and is much oriented for measuring PA. It also includes items that capture PA in days and hours, making it robust and comprehensive. Its first six items (i.e. Q1–Q6) measure work-related PA whereas the next nine items (i.e. Q7–Q15) measure PA relating to leisure and recreation. The sixteenth item (Q16) measures sedentary behavior. The GPAQ-2 has five questions that are associated with two levels of response, namely “Yes” and “No”. Based on WHO (Citation2014), “Yes” and “No” were associated with the codes 1 and 2 respectively in this study. In addition, PA was computed using the following formula recommended by WHO (Citation2014):
where the constants 8 and 4 represent the metabolic equivalents of vigorous and moderate PA respectively, and P2, P3, P5, …, P15 are the questions measuring PA in days/week and hours/day in GPAQ-2. With respect to the above equation, the unit of measurement of PA is minutes per week. The GPAQ-2 specifies that the minimum recommended PA level is 600 min per week. The GPAQ-2 has no factor structure; hence there is no need to validate it (WHO, Citation2014). The researcher however deemed it necessary to compute its overall internal consistency, which is .818.
shows the SDVs selected for this study from the study of Oliveira-Brochado et al. (Citation2010). All SDVs were treated as quantitative/continuous variables in the analysis software in order to be able to express them as a linear combination of PA through OLS regression analysis. For this reason, the interest of the researcher is to only find out whether or not each SDV predicts PA. also shows the operational definition of each variable.
Table 1. SDVs, their definitions and coding scheme
2.4. Ethical statement and data gathering
Prior to data collection, the management of each organization from which participants were drawn endorsed the study. Each participant also willingly signed an informed consent form, which clearly spelt out the benefits and potential risks to which every respondent was exposed. Data was collected in about 7 weeks through hand delivery of the self-reported questionnaire. Out of 760 questionnaires administered, 706 were returned. However 20 were discarded because they contained major response errors. Therefore, 686 questionnaires were analyzed.
2.5. Statistical analysis
Data was analyzed using SPSS version 21, which was preferred because its results are consistent with the majority of quantitative analysis softwares. In data analysis, descriptive statistics were used to summarize the data. Normal distribution of the data was also tested and verified for the dependent variable (i.e. PA). Data associated with PA was also normally distributed on the basis of satisfying p > .05 criterion recommended by Williams, Grajales, and Kurkiewicz (Citation2013) in terms of Shapiro-Wilk test (statistic = 4.322, p = .563). Moreover, the z-score computed for each value of the dependent variable is within the generally recommended range of −3 to 3. Results of the study are presented in the next section. It must be borne in mind that assessment of interaction, mediation and moderation effects on PA is not of interest in this study.
3. Results
shows the number of participants at each level of the categorical variables. For each variable, there is substantial representation of participants at each level. For instance, about 19% of all participants (N = 129) came from partially public organizations, whereas about 25% of all participants came from public organizations (N = 172). About 56% of all participants (N = 385) came from the private sector. shows the correlation matrix of SDVs and PA.
Table 2. Summary descriptive statistics for categorical SDVs
Table 3. Descriptive statistics showing PA level for relevant SDVs
shows the level of PA associated with each level of relevant SDVs. In this table, males (Mean = 756.27, SD = 226.11) have a higher level of PA than females (Mean = 627.019, SD = 233.32); public (Mean = 1141.88, SD = 629.04) and partially public (Mean = 950.68, SD = 219.31) have PA scores higher than private (Mean = 389.350, SD = 218.53); those not married (Mean = 834.78, SD = 592.30) have higher PA scores than those married (Mean = 592.303, SD = 231.76); Ewes have the highest PA scores (Mean = 865.76, SD = 327.91), whereas Hausas have the lowest PA scores (Mean = 168.24, SD = 74.04); and those without a history of chronic disease (Mean = 772.291, SD = 131.59) have larger PA scores as compared to their counterparts (Mean = 569.337, SD = 127.71).
In , there is a negative correlation between PA and sector 1 (R = −.574, p = .000), income (R = −.178, p = .000), age (R = −.785, p = .000), marital status (R = −.245, p = .000), gender (R = −.133, p = .000), tenure (R = −.269, p = .000), number of dependents (R = −.549, p = .000), tribe (R = −.479, p = .000), and history of chronic disease (R = −.209, p = .000) at 1% significance level. Thus, PA changes with these variables (e.g. number of dependents, income). These results suggest that PA differs significantly across the groups associated with sector 1, marital status, gender, tribe and HCD
Table 4. Correlation matrix of SDVs and PA
In , the SDVs account for 96.3% of the total variance in PA, with the error term accounting for 3.7% of the variance. In view of the large variance accounted by the SDVs, the OLS model is strongly fitted. The Durbin-Watson value of this model is also approximately 2. Based on Williams et al. (Citation2013), the independence-of-errors assumption is satisfied when the Durbin-Watson value falls between 1.5 and 2.5. The independence-of-errors assumption is therefore met for the OLS model. Also worth mentioning is the fact that the ANOVA test is significant at 5% significance level [F (14, 671) = 12.47.91, p = .000].
Table 5. OLS model summary
shows the coefficients of the OLS model. It can be seen that all SDVs significantly predict PA at 5% significance level, except sector 1 (t = −.477, p = .633, β = −24.236). Among all the predictors, HCD accounts for the largest effect on PA (t = 14.26, p = .000, β = 473.019). It could also be observed from in relation to that some negative correlations have become positive effects on PA in the OLS regression analysis. Similarly, the insignificant correlation between PA and religion is much significant at the level of the OLS regression. These changes are as a result of interaction between the SDVs. Based on Williams et al. (Citation2013) however, the collinearity assumption is satisfied on the basis of the VIF value of each predictor being less than 10 in a model involving 10 or more predictors.
Table 6. OLS coefficients
4. Discussion
Data analysis shows that the sector in which an individual works determines his or her involvement in PA. In this vein, PA is lowest in the private sector, with organization having a public/government ownership status supporting higher levels of work-related PA. This finding is consistent with previous studies (e.g. Oliveira-Brochado et al., Citation2010; Sale, Guppy, & El-Sayed, Citation2000) and supports the fact that supervision and monitoring in the private sector in Ghana are more rigorous and stricter as compared to the public sector. This situation could result in workers in private organizations having little or no opportunity and time to engage in PA. In addition, the construction and manufacturing sectors better promote engagement in physical activities as compared to the services sector. This result supports some previous studies (e.g. Humphreys & Ruseski, Citation2006; Oliveira-Brochado et al., Citation2010; Reichert et al., Citation2007) conducted in western countries, including the United States. It also agrees with the argument that construction and manufacturing jobs generally require more frequent engagement in physical activities such as walking and climbing, unlike service sector jobs that require sitting and other forms of sedentary behavior. Private and service sector companies would therefore have to rethink job and work-environment design in order to encourage workers to engage in PA. For example, they can create gyms where employees can exercise during break time. In Ghana and possibly other African countries however, this step may have to be taken by companies in the light of a new organizational culture.
According to this study, alternative employment also determines and limits engagement in PA. Potentially, having alternative employment decreases PA since this condition increases the working time of an individual. As a result, it is less likely for the individual to engage in PA, especially if the alternative employment requires much of sitting and other forms of sedentary behavior. This result is supported by Dembe et al. (Citation2005), who observed that sedentary jobs associated with more working time do not encourage engagement in PA and often accompany occupational stress, fatigue and other psychological and health issues. It likewise reflects the need for individuals to limit the number of alternative employments or jobs they hold at a time.
Income, age and tenure also significantly predict engagement in PA. More precisely, PA decreases with increasing income, age and tenure. This result is also in harmony with the study of Oliveira-Brochado et al. (Citation2010) and others (e.g. Humphreys & Ruseski, Citation2006; Reichert et al., Citation2007). It is also strongly supported by Sale et al. (Citation2000) in terms of the impact of income. To explain, people with higher income are likely those who have spent more years on the job and are busier, resulting in their inability to engage in PA. Tenure is also likely to increase with age and income. This being the case, the elderly and people with more income are less likely to engage in PA.
In terms of the correlation coefficients, increased number of dependents and history of chronic disease decrease the likelihood of a person engaging in PA. At the level of OLS regression nevertheless, these variables positively predict PA. This positive impact, in terms of NDs, is obviously as a result of the influence of education and affiliation to sectors that support PA such as the public sector (please see the strong positive correlation between NDs and sector 1). So whiles having a larger number of dependents is expected to increase work burden and consequently lead to lower PA, working in a sector that promotes engagement in PA leads to the opposite situation. This result is again supported by Dembe et al. (Citation2005), who found that some jobs are associated with adequate PA regardless of the influence of other demographic variables.
This study also indicates that people without a history of chronic disease better engage in PA as compared to their counterparts. This result is supported by several studies (e.g. Johnson & Taliaferro, Citation2011; Sale et al., Citation2000) on the basis of the argument that people who currently have or had one or more chronic diseases less engage in PA as compared to those without a history of these diseases. Some studies (e.g. Humphreys & Ruseski, Citation2006; Johnson & Taliaferro, Citation2011) asserted that a lack of the habit of engaging in PA must have led to the development of chronic diseases, especially cardiovascular diseases, by people with HCD. To say the least, having a chronic disease condition could also prevent a person from engaging in PA.
Other determinants of PA confirmed in this study are marital status, gender, education, employee category, tribal affiliation, and religion. To explain, individuals who were not married better engaged in PA as compared to those who were married. Based on Oliveira-Brochado et al. (Citation2010), this result implies that majority of unmarried persons were younger, had a smaller number of dependents, and had more time to spend on recreation and leisure. To add, females engaged less in PA possibly owing to their domestic role, relative physical weakness and unfavorable conditions of pregnancy and childbirth. Data analysis also shows that education increases with PA. With respect to the argument of Oliveira-Brochado et al. (Citation2010), it is thus likely that knowledge of the importance of PA increases with education.
As the individual’s job rank increases, he or she becomes busier and is therefore less likely to engage in PA. This study however indicates that PA increases with job rank. This result is justifiable in view of the influence of high education and one’s affiliation to a sector that supports regular engagement in PA such as the public/government and construction sectors. Therefore promoting the formal education of individuals is potentially an effective way to make engagement in PA a habit in African localities. Last but not least, religion and tribal affiliation make a significant impact on PA. Though no identifiable study supports this finding empirically, a framework developed by Oliveira-Brochado et al. (Citation2010) recognizes cultural variables, including religious and tribal affiliation, as potential determinants of PA.
5. Conclusion
All the SDVs captured in this study are determinants of PA in the population of workers studied, though sector 1 (i.e. whether or not a person worked in a public, partially public or private organization) did not significantly predict PA. Sector 2 (i.e. whether or not one works in the services, manufacturing or construction sector), HCD, tribe and age are some of the strongest determinants of PA.
Moreover, access to alternative employment, income, age and tenure counter engagement in PA. By implication, people with alternative employments and those who have high income and job tenure less engage in PA. Ageing also limits engagement in PA, evidently because it is associated with the conditions of being married and having one or more chronic diseases, which both prevent participation in PA. Education, gender, marital status and HCD are some of the SDVs making a positive effect on PA.
Results of this study unveils two potentially effective ways for encouraging engagement in PA in African work environments. First, private and service companies could create recreation centers (e.g. gyms) where employees can exercise and recreate during lunch or break time. Such recreation centers can motivate employees to participate in PA regularly. Improving workers’ access to higher education can also enhance their knowledge of the relevance of PA to health. To improve occupational health and productivity therefore, organizations would have to introduce health-related training programs in which workers are educated on PA and its health implications.
6. Limitation of the study
The researcher admits that treating categorical variables (i.e. sector 1, sector 2, marital status, gender, employee category, tribe, religion and HCD) as quantitative/continuous variables resulted in only one regression coefficient for each of these variables. The fact that the categorical variables were not dummy-coded two or more times to yield more than one coefficient for each of them limits the scope of evidences provided in this study. The researcher recommends the execution of studies aimed at addressing this weakness.
Additional information
Funding
Notes on contributors
Nestor Asiamah
Nestor Asiamah is a Public Health Researcher and Scientist who seeks to use research to contribute to quality of life. He has published novel papers in the areas of healthcare, health management, quality of life, physical activity, ageing and health psychology. He is currently undertaking several research projects aimed at improving the quality of healthcare in developing African countries. He is also working on establishing the Africa Center for Epidemiology (ACE), a center for public health research excellence.
References
- Akhtar-Danesh, N., & Landeen, J. (2007). Relation between depression and sociodemographic factors. International Journal of Mental Health Systems, 1(4), 1–9. doi:10.1186/1752-4458-1-4
- Blake, H. (2012). Physical activity and exercise in the treatment of depression. Frontiers in Psychiatry, 3(2), 1–4.
- Craft, L. L., & Perna, F. M. (2004). The benefits of exercise for the clinically depressed. Journal of Clinical Psychiatric, 6, 104–113.
- Dembe, A. E., Erickson, J. B., Delbos, R. G., & Banks, S. M. (2005). The impact of overtime and long work hours on occupational injuries and illnesses: New evidence from the United States. Occupational & Environmental Medicine, 62, 588–597. doi:10.1136/oem.2004.016667
- Humphreys, B. R., & Ruseski, J. E. (2006). Economic determinants of participation in physical activity and sport, international association of sports economics (Working Paper Series, Paper No. 06-13). Illinois: International Association of Sports Economists.
- Johnson, K. E., & Taliaferro, L. A. (2011). Relationships between physical activity and depressive symptoms among middle and older adolescents: A review of the research literature. Journal for Specialists in Pediatric Nursing, 16, 235–251.10.1111/jspn.2011.16.issue-4
- Josefsson, T., Lindwall, M., & Archer, T. (2014). Physical exercise intervention in depressive disorders: Meta-analysis and systematic review. Scandinavian Journal of Medicine & Science in Sports, 24, 259–272.10.1111/sms.2014.24.issue-2
- Micklesfield, L. K., Pedro, T. M., Kahn, K., Kinsman, J., Pettifor, J. M., Tollman, S., & Norris, S. A. (2014). Physical activity and sedentary behavior among adolescents in rural South Africa: Levels, patterns and correlates. BMC Public Health, 14(40), 1–10.
- Mukona, D., Munjanja, S. P., Zvinavashe, M., & Stray-Pederson, B. (2016). Physical activity in pregnant women in Africa: A systematic review. International Journal of Nursing and Midwifery, 8, 28–34.
- Oliveira-Brochado, A., Oliveira-Brochado, F., & Brito, P. Q. (2010). Effects of personal, social and environmental factors on physical activity behavior among adults. Actividade Física, 28, 7–17.
- Oyeyemi, A. L., Ishaku, C. M., Oyekola, J., Wakawa, H. D., Lawan, A., Yakubu, S., & Oyeyemi, A. Y. (2016). Patterns and associated factors of physical activity among adolescents in Nigeria. Plos One, 11(2), 1–16.
- Reichert, F. F., Barros, A. J. D., Domingues, M. R., & Hallal, P. C. (2007). The role of perceived personal barriers to engagement in leisure-time physical activity. American Journal of Public Health, 97, 515–519.10.2105/AJPH.2005.070144
- Sale, C., Guppy, A., & El-Sayed, M. (2000). Individual differences, exercise and leisure activity in predicting affective well-being in young adults. Ergonomics, 43, 1689–1697.10.1080/001401300750004096
- Ströhle, A. (2009). Physical activity, exercise, depression and anxiety disorders. Journal of Neural Transmission, 116, 777–784. doi:10.1007/s00702-008-0092-x
- Webster, L. (2015). The effectiveness of physical activity as an intervention in the treatment of depression: A systematic review. Journal of Applied Psychology and Social Sciences, 1, 28–40.
- Williams, M. N., Grajales, C. A. G., & Kurkiewicz, D. (2013). Assumptions of multiple regression: Correcting two misconceptions. Practical Assessment, Research & Evaluation, 18(11), 1–14.
- World Health Organization. (2014). Global recommendations on physical activity for health. Retrieved October 15, 2016 from http://whqlibdoc.who.int/publications/2010/9789241599979_eng.pdf
- World Health Organization. (2015). Physical activity strategy for the WHO European Region 2016-2025. Retrieved October 15, 2016 from http://www.euro.who.int/__data/assets/pdf_file/0010/282961/65wd09e_PhysicalActivityStrategy_150474.pdf